An interval-parameter fuzzy nonlinear optimization model
国际自动化与计算杂志.英文版.
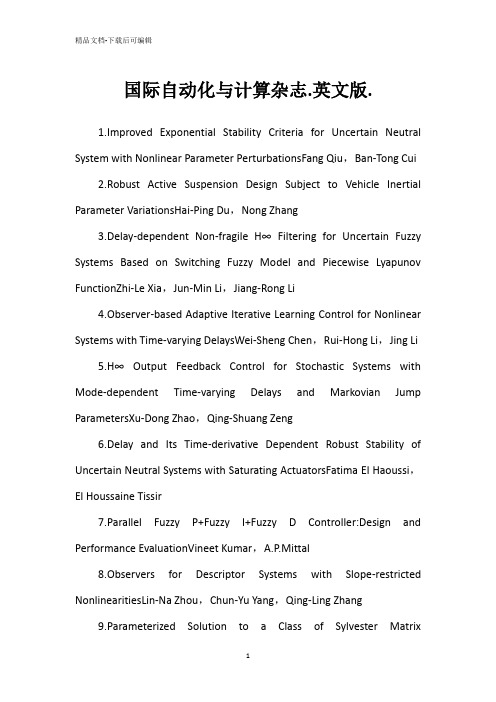
国际自动化与计算杂志.英文版.1.Improved Exponential Stability Criteria for Uncertain Neutral System with Nonlinear Parameter PerturbationsFang Qiu,Ban-Tong Cui2.Robust Active Suspension Design Subject to Vehicle Inertial Parameter VariationsHai-Ping Du,Nong Zhang3.Delay-dependent Non-fragile H∞ Filtering for Uncertain Fuzzy Systems Based on Switching Fuzzy Model and Piecewise Lyapunov FunctionZhi-Le Xia,Jun-Min Li,Jiang-Rong Li4.Observer-based Adaptive Iterative Learning Control for Nonlinear Systems with Time-varying DelaysWei-Sheng Chen,Rui-Hong Li,Jing Li5.H∞ Output Feedback Control for Stochastic Systems with Mode-dependent Time-varying Delays and Markovian Jump ParametersXu-Dong Zhao,Qing-Shuang Zeng6.Delay and Its Time-derivative Dependent Robust Stability of Uncertain Neutral Systems with Saturating ActuatorsFatima El Haoussi,El Houssaine Tissir7.Parallel Fuzzy P+Fuzzy I+Fuzzy D Controller:Design and Performance EvaluationVineet Kumar,A.P.Mittal8.Observers for Descriptor Systems with Slope-restricted NonlinearitiesLin-Na Zhou,Chun-Yu Yang,Qing-Ling Zhang9.Parameterized Solution to a Class of Sylvester MatrixEquationsYu-Peng Qiao,Hong-Sheng Qi,Dai-Zhan Cheng10.Indirect Adaptive Fuzzy and Impulsive Control of Nonlinear SystemsHai-Bo Jiang11.Robust Fuzzy Tracking Control for Nonlinear Networked Control Systems with Integral Quadratic ConstraintsZhi-Sheng Chen,Yong He,Min Wu12.A Power-and Coverage-aware Clustering Scheme for Wireless Sensor NetworksLiang Xue,Xin-Ping Guan,Zhi-Xin Liu,Qing-Chao Zheng13.Guaranteed Cost Active Fault-tolerant Control of Networked Control System with Packet Dropout and Transmission DelayXiao-Yuan Luo,Mei-Jie Shang,Cai-Lian Chen,Xin-Ping Guanparison of Two Novel MRAS Based Strategies for Identifying Parameters in Permanent Magnet Synchronous MotorsKan Liu,Qiao Zhang,Zi-Qiang Zhu,Jing Zhang,An-Wen Shen,Paul Stewart15.Modeling and Analysis of Scheduling for Distributed Real-time Embedded SystemsHai-Tao Zhang,Gui-Fang Wu16.Passive Steganalysis Based on Higher Order Image Statistics of Curvelet TransformS.Geetha,Siva S.Sivatha Sindhu,N.Kamaraj17.Movement Invariants-based Algorithm for Medical Image Tilt CorrectionMei-Sen Pan,Jing-Tian Tang,Xiao-Li Yang18.Target Tracking and Obstacle Avoidance for Multi-agent SystemsJing Yan,Xin-Ping Guan,Fu-Xiao Tan19.Automatic Generation of Optimally Rigid Formations Using Decentralized MethodsRui Ren,Yu-Yan Zhang,Xiao-Yuan Luo,Shao-Bao Li20.Semi-blind Adaptive Beamforming for High-throughput Quadrature Amplitude Modulation SystemsSheng Chen,Wang Yao,Lajos Hanzo21.Throughput Analysis of IEEE 802.11 Multirate WLANs with Collision Aware Rate Adaptation AlgorithmDhanasekaran Senthilkumar,A. Krishnan22.Innovative Product Design Based on Customer Requirement Weight Calculation ModelChen-Guang Guo,Yong-Xian Liu,Shou-Ming Hou,Wei Wang23.A Service Composition Approach Based on Sequence Mining for Migrating E-learning Legacy System to SOAZhuo Zhang,Dong-Dai Zhou,Hong-Ji Yang,Shao-Chun Zhong24.Modeling of Agile Intelligent Manufacturing-oriented Production Scheduling SystemZhong-Qi Sheng,Chang-Ping Tang,Ci-Xing Lv25.Estimation of Reliability and Cost Relationship for Architecture-based SoftwareHui Guan,Wei-Ru Chen,Ning Huang,Hong-Ji Yang1.A Computer-aided Design System for Framed-mould in Autoclave ProcessingTian-Guo Jin,Feng-Yang Bi2.Wear State Recognition of Drills Based on K-means Cluster and Radial Basis Function Neural NetworkXu Yang3.The Knee Joint Design and Control of Above-knee Intelligent Bionic Leg Based on Magneto-rheological DamperHua-Long Xie,Ze-Zhong Liang,Fei Li,Li-Xin Guo4.Modeling of Pneumatic Muscle with Shape Memory Alloy and Braided SleeveBin-Rui Wang,Ying-Lian Jin,Dong Wei5.Extended Object Model for Product Configuration DesignZhi-Wei Xu,Ze-Zhong Liang,Zhong-Qi Sheng6.Analysis of Sheet Metal Extrusion Process Using Finite Element MethodXin-Cun Zhuang,Hua Xiang,Zhen Zhao7.Implementation of Enterprises' Interoperation Based on OntologyXiao-Feng Di,Yu-Shun Fan8.Path Planning Approach in Unknown EnvironmentTing-Kai Wang,Quan Dang,Pei-Yuan Pan9.Sliding Mode Variable Structure Control for Visual Servoing SystemFei Li,Hua-Long Xie10.Correlation of Direct Piezoelectric Effect on EAPap under Ambient FactorsLi-Jie Zhao,Chang-Ping Tang,Peng Gong11.XML-based Data Processing in Network Supported Collaborative DesignQi Wang,Zhong-Wei Ren,Zhong-Feng Guo12.Production Management Modelling Based on MASLi He,Zheng-Hao Wang,Ke-Long Zhang13.Experimental Tests of Autonomous Ground Vehicles with PreviewCunjia Liu,Wen-Hua Chen,John Andrews14.Modelling and Remote Control of an ExcavatorYang Liu,Mohammad Shahidul Hasan,Hong-Nian Yu15.TOPSIS with Belief Structure for Group Belief Multiple Criteria Decision MakingJiang Jiang,Ying-Wu Chen,Da-Wei Tang,Yu-Wang Chen16.Video Analysis Based on Volumetric Event DetectionJing Wang,Zhi-Jie Xu17.Improving Decision Tree Performance by Exception HandlingAppavu Alias Balamurugan Subramanian,S.Pramala,B.Rajalakshmi,Ramasamy Rajaram18.Robustness Analysis of Discrete-time Indirect Model Reference Adaptive Control with Normalized Adaptive LawsQing-Zheng Gao,Xue-Jun Xie19.A Novel Lifecycle Model for Web-based Application Development in Small and Medium EnterprisesWei Huang,Ru Li,Carsten Maple,Hong-Ji Yang,David Foskett,Vince Cleaver20.Design of a Two-dimensional Recursive Filter Using the Bees AlgorithmD. T. Pham,Ebubekir Ko(c)21.Designing Genetic Regulatory Networks Using Fuzzy Petri Nets ApproachRaed I. Hamed,Syed I. Ahson,Rafat Parveen1.State of the Art and Emerging Trends in Operations and Maintenance of Offshore Oil and Gas Production Facilities: Some Experiences and ObservationsJayantha P.Liyanage2.Statistical Safety Analysis of Maintenance Management Process of Excavator UnitsLjubisa Papic,Milorad Pantelic,Joseph Aronov,Ajit Kumar Verma3.Improving Energy and Power Efficiency Using NComputing and Approaches for Predicting Reliability of Complex Computing SystemsHoang Pham,Hoang Pham Jr.4.Running Temperature and Mechanical Stability of Grease as Maintenance Parameters of Railway BearingsJan Lundberg,Aditya Parida,Peter S(o)derholm5.Subsea Maintenance Service Delivery: Mapping Factors Influencing Scheduled Service DurationEfosa Emmanuel Uyiomendo,Tore Markeset6.A Systemic Approach to Integrated E-maintenance of Large Engineering PlantsAjit Kumar Verma,A.Srividya,P.G.Ramesh7.Authentication and Access Control in RFID Based Logistics-customs Clearance Service PlatformHui-Fang Deng,Wen Deng,Han Li,Hong-Ji Yang8.Evolutionary Trajectory Planning for an Industrial RobotR.Saravanan,S.Ramabalan,C.Balamurugan,A.Subash9.Improved Exponential Stability Criteria for Recurrent Neural Networks with Time-varying Discrete and Distributed DelaysYuan-Yuan Wu,Tao Li,Yu-Qiang Wu10.An Improved Approach to Delay-dependent Robust Stabilization for Uncertain Singular Time-delay SystemsXin Sun,Qing-Ling Zhang,Chun-Yu Yang,Zhan Su,Yong-Yun Shao11.Robust Stability of Nonlinear Plants with a Non-symmetric Prandtl-Ishlinskii Hysteresis ModelChang-An Jiang,Ming-Cong Deng,Akira Inoue12.Stability Analysis of Discrete-time Systems with Additive Time-varying DelaysXian-Ming Tang,Jin-Shou Yu13.Delay-dependent Stability Analysis for Markovian Jump Systems with Interval Time-varying-delaysXu-Dong Zhao,Qing-Shuang Zeng14.H∞ Synchronization of Chaotic Systems via Delayed Feedback ControlLi Sheng,Hui-Zhong Yang15.Adaptive Fuzzy Observer Backstepping Control for a Class of Uncertain Nonlinear Systems with Unknown Time-delayShao-Cheng Tong,Ning Sheng16.Simulation-based Optimal Design of α-β-γ-δ FilterChun-Mu Wu,Paul P.Lin,Zhen-Yu Han,Shu-Rong Li17.Independent Cycle Time Assignment for Min-max SystemsWen-De Chen,Yue-Gang Tao,Hong-Nian Yu1.An Assessment Tool for Land Reuse with Artificial Intelligence MethodDieter D. Genske,Dongbin Huang,Ariane Ruff2.Interpolation of Images Using Discrete Wavelet Transform to Simulate Image Resizing as in Human VisionRohini S. Asamwar,Kishor M. Bhurchandi,Abhay S. Gandhi3.Watermarking of Digital Images in Frequency DomainSami E. I. Baba,Lala Z. Krikor,Thawar Arif,Zyad Shaaban4.An Effective Image Retrieval Mechanism Using Family-based Spatial Consistency Filtration with Object RegionJing Sun,Ying-Jie Xing5.Robust Object Tracking under Appearance Change ConditionsQi-Cong Wang,Yuan-Hao Gong,Chen-Hui Yang,Cui-Hua Li6.A Visual Attention Model for Robot Object TrackingJin-Kui Chu,Rong-Hua Li,Qing-Ying Li,Hong-Qing Wang7.SVM-based Identification and Un-calibrated Visual Servoing for Micro-manipulationXin-Han Huang,Xiang-Jin Zeng,Min Wang8.Action Control of Soccer Robots Based on Simulated Human IntelligenceTie-Jun Li,Gui-Qiang Chen,Gui-Fang Shao9.Emotional Gait Generation for a Humanoid RobotLun Xie,Zhi-Liang Wang,Wei Wang,Guo-Chen Yu10.Cultural Algorithm for Minimization of Binary Decision Diagram and Its Application in Crosstalk Fault DetectionZhong-Liang Pan,Ling Chen,Guang-Zhao Zhang11.A Novel Fuzzy Direct Torque Control System for Three-level Inverter-fed Induction MachineShu-Xi Liu,Ming-Yu Wang,Yu-Guang Chen,Shan Li12.Statistic Learning-based Defect Detection for Twill FabricsLi-Wei Han,De Xu13.Nonsaturation Throughput Enhancement of IEEE 802.11b Distributed Coordination Function for Heterogeneous Traffic under Noisy EnvironmentDhanasekaran Senthilkumar,A. Krishnan14.Structure and Dynamics of Artificial Regulatory Networks Evolved by Segmental Duplication and Divergence ModelXiang-Hong Lin,Tian-Wen Zhang15.Random Fuzzy Chance-constrained Programming Based on Adaptive Chaos Quantum Honey Bee Algorithm and Robustness AnalysisHan Xue,Xun Li,Hong-Xu Ma16.A Bit-level Text Compression Scheme Based on the ACW AlgorithmHussein A1-Bahadili,Shakir M. Hussain17.A Note on an Economic Lot-sizing Problem with Perishable Inventory and Economies of Scale Costs:Approximation Solutions and Worst Case AnalysisQing-Guo Bai,Yu-Zhong Zhang,Guang-Long Dong1.Virtual Reality: A State-of-the-Art SurveyNing-Ning Zhou,Yu-Long Deng2.Real-time Virtual Environment Signal Extraction and DenoisingUsing Programmable Graphics HardwareYang Su,Zhi-Jie Xu,Xiang-Qian Jiang3.Effective Virtual Reality Based Building Navigation Using Dynamic Loading and Path OptimizationQing-Jin Peng,Xiu-Mei Kang,Ting-Ting Zhao4.The Skin Deformation of a 3D Virtual HumanXiao-Jing Zhou,Zheng-Xu Zhao5.Technology for Simulating Crowd Evacuation BehaviorsWen-Hu Qin,Guo-Hui Su,Xiao-Na Li6.Research on Modelling Digital Paper-cut PreservationXiao-Fen Wang,Ying-Rui Liu,Wen-Sheng Zhang7.On Problems of Multicomponent System Maintenance ModellingTomasz Nowakowski,Sylwia Werbinka8.Soft Sensing Modelling Based on Optimal Selection of Secondary Variables and Its ApplicationQi Li,Cheng Shao9.Adaptive Fuzzy Dynamic Surface Control for Uncertain Nonlinear SystemsXiao-Yuan Luo,Zhi-Hao Zhu,Xin-Ping Guan10.Output Feedback for Stochastic Nonlinear Systems with Unmeasurable Inverse DynamicsXin Yu,Na Duan11.Kalman Filtering with Partial Markovian Packet LossesBao-Feng Wang,Ge Guo12.A Modified Projection Method for Linear FeasibilityProblemsYi-Ju Wang,Hong-Yu Zhang13.A Neuro-genetic Based Short-term Forecasting Framework for Network Intrusion Prediction SystemSiva S. Sivatha Sindhu,S. Geetha,M. Marikannan,A. Kannan14.New Delay-dependent Global Asymptotic Stability Condition for Hopfield Neural Networks with Time-varying DelaysGuang-Deng Zong,Jia Liu hHTTp://15.Crosscumulants Based Approaches for the Structure Identification of Volterra ModelsHouda Mathlouthi,Kamel Abederrahim,Faouzi Msahli,Gerard Favier1.Coalition Formation in Weighted Simple-majority Games under Proportional Payoff Allocation RulesZhi-Gang Cao,Xiao-Guang Yang2.Stability Analysis for Recurrent Neural Networks with Time-varying DelayYuan-Yuan Wu,Yu-Qiang Wu3.A New Type of Solution Method for the Generalized Linear Complementarity Problem over a Polyhedral ConeHong-Chun Sun,Yan-Liang Dong4.An Improved Control Algorithm for High-order Nonlinear Systems with Unmodelled DynamicsNa Duan,Fu-Nian Hu,Xin Yu5.Controller Design of High Order Nonholonomic System with Nonlinear DriftsXiu-Yun Zheng,Yu-Qiang Wu6.Directional Filter for SAR Images Based on NonsubsampledContourlet Transform and Immune Clonal SelectionXiao-Hui Yang,Li-Cheng Jiao,Deng-Feng Li7.Text Extraction and Enhancement of Binary Images Using Cellular AutomataG. Sahoo,Tapas Kumar,B.L. Rains,C.M. Bhatia8.GH2 Control for Uncertain Discrete-time-delay Fuzzy Systems Based on a Switching Fuzzy Model and Piecewise Lyapunov FunctionZhi-Le Xia,Jun-Min Li9.A New Energy Optimal Control Scheme for a Separately Excited DC Motor Based Incremental Motion DriveMilan A.Sheta,Vivek Agarwal,Paluri S.V.Nataraj10.Nonlinear Backstepping Ship Course ControllerAnna Witkowska,Roman Smierzchalski11.A New Method of Embedded Fourth Order with Four Stages to Study Raster CNN SimulationR. Ponalagusamy,S. Senthilkumar12.A Minimum-energy Path-preserving Topology Control Algorithm for Wireless Sensor NetworksJin-Zhao Lin,Xian Zhou,Yun Li13.Synchronization and Exponential Estimates of Complex Networks with Mixed Time-varying Coupling DelaysYang Dai,YunZe Cai,Xiao-Ming Xu14.Step-coordination Algorithm of Traffic Control Based on Multi-agent SystemHai-Tao Zhang,Fang Yu,Wen Li15.A Research of the Employment Problem on Common Job-seekersand GraduatesBai-Da Qu。
Fuzzy中英对照表
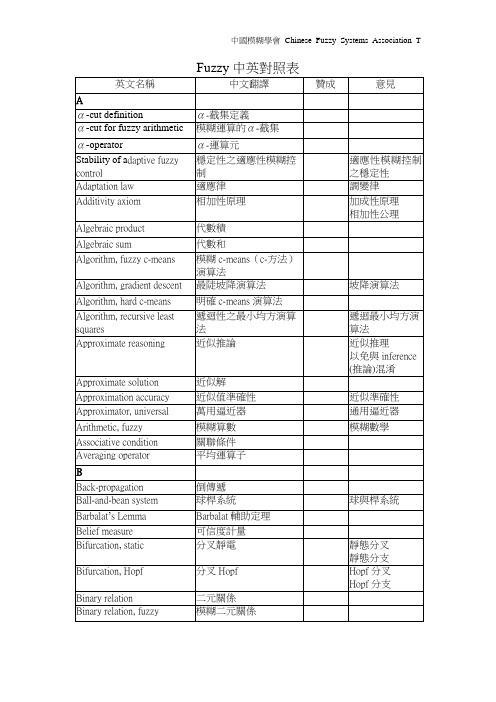
Composition of fuzzy relations
模糊關係之合成
Compositional rule of inference
推論之合成規則
合成規則推論法
Computing, soft
軟性運算
柔性運算或
柔性解算
Conditional possibility distribution
工業流程控制
Inference, composition based
組合式推論
Inference, individual-rule based
個別規則基礎的推論
個別規則式推論
Inference engine, Dienes-Rescher
Dienes-Rescher推論機制
Inference engine, Lukasiewicz
模糊關係方程式
Equilibrium
均衡
平衡
Extension principle
擴展法則
Feedforward network
前饋網路
Fuzzifier, Gaussian
高斯模糊化
高斯模糊化器
Fuzzifier, singleton
單點模糊化
單點模糊化器
Fuzzifier, triangular
Dombi類型之模糊交集
Intersection, fuzzy Dubois-Prade class
Dubois-Prade類型之模糊交集
Intersection Yager class
Yager類型之交集
Interval analysis
區間分析
Interval-valued function
基于NT降阶算法的区间二型模糊系统辨识
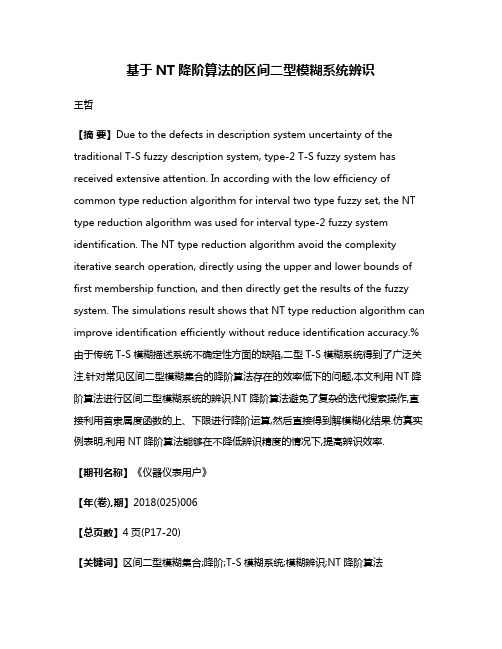
基于NT降阶算法的区间二型模糊系统辨识王哲【摘要】Due to the defects in description system uncertainty of the traditional T-S fuzzy description system, type-2 T-S fuzzy system has received extensive attention. In according with the low efficiency of common type reduction algorithm for interval two type fuzzy set, the NT type reduction algorithm was used for interval type-2 fuzzy system identification. The NT type reduction algorithm avoid the complexity iterative search operation, directly using the upper and lower bounds of first membership function, and then directly get the results of the fuzzy system. The simulations result shows that NT type reduction algorithm can improve identification efficiently without reduce identification accuracy.%由于传统T-S模糊描述系统不确定性方面的缺陷,二型T-S模糊系统得到了广泛关注.针对常见区间二型模糊集合的降阶算法存在的效率低下的问题,本文利用NT降阶算法进行区间二型模糊系统的辨识.NT降阶算法避免了复杂的迭代搜索操作,直接利用首隶属度函数的上、下限进行降阶运算,然后直接得到解模糊化结果.仿真实例表明,利用NT降阶算法能够在不降低辨识精度的情况下,提高辨识效率.【期刊名称】《仪器仪表用户》【年(卷),期】2018(025)006【总页数】4页(P17-20)【关键词】区间二型模糊集合;降阶;T-S模糊系统;模糊辨识;NT降阶算法【作者】王哲【作者单位】天津现代职业技术学院,天津 300350【正文语种】中文【中图分类】TP273+.40 引言近些年,T-S模糊模型在非线性系统辨识方面取得了很好的效果。
模糊认知图的学习

模糊认知图的学习此篇随笔仅⽤于记录学习内容⽅便以后查阅,主要参考学习了 迟亚雄. 模糊认知图智能学习算法与应⽤研究[D].基本理论与⽅法基于Hebbian的学习⽅法 核⼼思想:神经元的激活顺序和⽅式会影响权重的变化,若两神经元异步激活则降低权值,同步激活则增⾼权值。
特点:总是依赖专家知识。
⾮线性Hebbian学习算法[1](Nonlinear Hebbian Learning, NHL)的初始化需要专家对concept进⾏⼲预,⽐如建议各concept的模糊值,这些模糊值的取值范围以及各concept之间的因果关系等。
数据驱动型NHL[2](Data-driven Nonlinear Hebbian Learning, DDNHL),与NHL类似,但DDNHL利⽤了可观测到的数据进⾏学习来提⾼ FCMs 的模型质量。
集成学习与NHL相结合的学习算法[3],⽤NHL训练模型,再使⽤集成学习算法提⾼性能。
在学习模糊认知图的准确性⽅⾯由于DDNHL。
基于进化计算的学习⽅法 Hebbian的⽅法⽐较依赖专家知识,进化计算的⽬的是从数据中学习模糊认知图,搜索最优的模糊认知图。
进化算法是⼀种启发式算法,⽐较常见的启发式算法有:遗传算法,粒⼦群优化算法,模拟退⽕,蚁群优化等。
⾯临的问题 模糊认知图扩展到⼀定规模时,⾯临的问题是多维度优化问题,因为随着决策变量的增加,需要确定的权重关系的数量会呈指数增长,会造成维度灾难。
从优化的⾓度看,训练样本数量不变的情况下,决策变量的数量增加会导致过拟合。
当数据的维度到达⼀定⾼度,如果要找到最优解或者仅仅达到低维度的同等性能,则需要⼏何数量增长的数据。
真实模糊认知图的⽹络密度要⽐算法学习得到的⽹络模型的密度低的多。
基于神经⽹络和进化计算的模糊认知图学习Reference[1] Elpiniki Papageorgiou, Chrysostomos Stylios, Peter Groumpos. Fuzzy Cognitive Map Learning Based on Nonlinear Hebbian Rule[M]// AI 2003: Advances in Artificial Intelligence. Springer Berlin Heidelberg, 2003.[2] Stach W , Kurgan L A , Pedrycz W . Data-driven Nonlinear Hebbian Learning method for Fuzzy Cognitive Maps[C]// IEEE IEEE International Conference on Fuzzy Systems. IEEE, 2008.[3] Papageorgiou E I , Kannappan A . Fuzzy cognitive map ensemble learning paradigm to solve classification problems: Application to autism identification[J]. Applied Soft Computing Journal, 2012, 12(12):3798-3809.[] 迟亚雄. 模糊认知图智能学习算法与应⽤研究[D].。
带有饱和的电机伺服系统非奇异终端滑模funnel控制

带有饱和的电机伺服系统非奇异终端滑模funnel控制陈强;汤筱晴【摘要】本文提出一种非奇异终端滑模funnel控制(NTSMFC)方法,实现带有饱和输入电机伺服系统的指定性能跟踪控制.根据中值定理,非光滑饱和函数转化为放射形式,并且应用一个简单的神经网络进行逼近和补偿.为保证跟踪误差被限制在指定的界限内,同时为避免构建复杂的barrier李雅普诺夫函数或逆函数,本文采用一个新的限制变量.然后,构建非奇异终端滑模funnel控制器保证电机伺服系统的指定跟踪性能.该方法无需事先已知输入饱和函数的界限等先验知识,且基于李雅普诺夫函数设计可以保证位置跟踪误差的收敛性,最后给出仿真对比实例证明了该方法的有效性.【期刊名称】《控制理论与应用》【年(卷),期】2015(032)008【总页数】8页(P1064-1071)【关键词】funnel控制;非奇异终端滑模;神经网络;输入饱和【作者】陈强;汤筱晴【作者单位】浙江工业大学信息工程学院,浙江杭州310023;浙江工业大学信息工程学院,浙江杭州310023【正文语种】中文【中图分类】TP273Over the past decades,motor servo systems have been widely studied in motion control applications[1-3].The mechanical connection between servo motors and mechanical devices produces non-smooth nonlinear constraints on their outputs and/or inputs in the form of the physical stoppage,saturation,hysteresis,and dead-zone.During operation,violation of the constraints leads to performance degradation,hazards or system damage.For the output constraint,there are some effective methods for position,velocity,and force constraints existing in motor servo systems.By using the logarithmic function in the Lyapunov function design,a barrier Lyapunov function(BLF)is constructed,in which a symmetric or asymmetric constraint is utilized to constrain the state variable of the control system,so that the tracking errors can be indirectly constrained[4-6].However,the expression of BLF is complex, and extra efforts are needed to ensure the continuity and differentiability.In[7-9],a prescribed performance control(PPC)scheme is proposed,and the tracking error of a nonlinear system is transformed into a new error by constructing the inverse of the transformation function.Therefore,the prescribed tracking performance of the transient property and the steady-state error can be guaranteed.But PPC scheme may cause a singularity problem since the inverse transformation function includes a partial differential terms.Being a non-model-based(memory less)constraint technique,the funnel control is proposed to guarantee the prescribed transient behavior and asymptotic tracking of the system[10-13].This technique bypassesthe difficulties of identification and estimation of traditional high-gain adaptive control.Recently,a new error-constraint variable is designed as a virtual control variable in the backstep-ping design to ensure the prescribed transient and steadystate performance[14],and thus the aforementioned complex transformation function is not needed any more.In[15],a funnel dynamic surface control with prescribed performance is proposed to overcome the explosion of complexity problem in the backstepping technique,and the tracking performance of closed-loop system is guaranteed.Sliding mode control(SMC)is one of the most useful approaches to deal with system uncertainties and bounded disturbances,and has been widely applied in various fields[16-17].The traditional linear sliding mode control scheme can guarantee the asymptotical convergence of tracking errors when time goes to the infinity.Recently,many research works have focused on the finite-time convergence of tracking errors.Man and Yu[18]proposed a terminal sliding mode control(TSMC)scheme by introducing a nonlinear term in the SMC design and the tracking error can be guaranteed to converge within a finite time.However,there are two disadvantages of TSMC,i.e.,the singularity problem and requirement of the uncertainty bound.To overcome the singularity problem,Feng,et al.[19]and Yu,et al.[20]proposed nonsingular terminal sliding mode control(NTSMC)methods.Besides,Chen,et al [21]utilized a recurrent Hermite neural network(RHNN)to estimate the lumped uncertainty online when designing the nonsingular terminalsliding surface,and hence the lumped uncertainty bound is unnecessary. As one of the most important non-smooth nonlinearities,saturation on hardware dictates that the magnitude of the control signal is always constrained,which often severely limits system performance,giving rise to undesirable inaccuracy or leading instability[22].So far,many significant results on the control design for the systems with input saturation have been obtained[23-27].However,the lower and upper limits of the saturation constraints should be exactly known or estimated for controllers design.Recently,several research work has been investigated without using the prior knowledge of saturation bounds. Wen et al.[28]uses a smooth non-affine function of the control input signal to approximate the non-smooth saturation function,and a Nussbaum function is introduced to compensate for the nonlinear term arising from the input saturation.Based on the idea of[28],Wang et al.[29]transforms the non-affine function of the system into an affine form,and an adaptive control scheme is derived without requiring the prior knowledge of input saturation bounds.In this paper,we propose a nonsingular terminal sliding mode funnel control to achieve a prescribed tracking performance for motor servo systems with unknown input saturation.A smooth and affine function is used to solve the input saturation problem.Meanwhile,to avoid using the complex barrier Lyapunov function or inverse transformed function,a funnel constraint variable is utilized in constructingthenonsingularterminalslidingmodescheme to force thetracking error fall within prescribe boundaries. No prior knowledge of the input saturation bounds is required in the proposed method,and the effectiveness is demonstrated by simulation results.2.1 System descriptionThe mechanical dynamics of the motor servo system can be described as follows:where x=[x,]T∈R2,u(t)∈R,y∈R are state variables,the control input voltage to the motor and the output from the motor,respectively;x is the position,m is the inertia,k0is a positive control gain(the force constant),f(x,t)is the friction force;d(x,t)represents a bounded disturbance modeling nonlinear elastic forces generated by coupling and protective covers,measurement noise,power electronics disturbances and other uncertainties.v(u)∈R is the plant input subject to saturation nonlinearity described bywhere vmaxis an unknown parameter of input saturation.For convenience of the controller design,defining x1=x,x2=,the mechanical dynamics of the motor servo system can be transformed into Without loss of generality,two technical assumptions are made to pose the problem in a tractable manner.1)The desired position trajectory yd,the time derivative˙ydand¨ydare both bounded and smooth signals.2)The angular position and velocity,x1and x2,are measurable.2.2 Nonlinear saturation modelClearly,the relationship between the applied control v(t)and thecontrol input u(t)has a sharp corner when |u(t)|=vmax.As shown in Fig.1,the saturation is approximated by a smooth non-affine function defined asThen,v=sat(u)in(2)can be expressed in the following formwhere d1(u)=sat(u)-g(u)is a bounded function and its bound can be obtained aswhere D is the upper bound of|d1(u)|.According to the mean-value theorem[29],there exists a constant ξ with 0<ξ<1,such thatwhereand u0∈[0,u].By choosing u0=0,(6)can be rewritten in the following affine formSubstituting(7)and(5)into(3),we can obtainwhere2.3 Neural network approximationDue to good capabilities in function approximation,neural networks (NNs)are usually used for the approximation of nonlinear functions.The following neural network with a simple structure and a fast convergence property will be used to approximate the continuous functionwhere W∗∈Rn1×n2is the ideal weight matrix,φ(X)∈Rn1×1is the basis function of the neural network,ε is the neural network approximation error satisfying|ε|≤εN,φ(X)can be chosen as the commonly used sigmoid function,which is in the following formwith a,b,c and d being appropriate parameters.Remark 1 The employed neural network with sigmoid function representsa class of linearly parameterized approximation methods,and can be replaced by any other approximation approaches such as spline functions,RBF functions or fuzzy systems.However,the structure of the employed neural network in the this paper is simpler than the other neural networks that are commonly used in other works.There is no hidden layer in the employed NN,in which five inputs and one output are included and the corresponding weight matrix is 5×1.3.1 Funnel error variableFunnel control is a strategy that employs a timevarying gain ρ(t)to control systems of class S with a relative degree r=1 or 2,stable zero dynamics,and known high-frequency gains.The system S is governed by the funnel controller with the control inputwhere e(t)=y1-ydis the tracking error,ρ(·)denotes the control gain. As shown in Fig.2,evaluate the vertical distance at the actual time between the funnel boundary Fφ(t)and the Eucli dian error norm‖e (t)‖asThe funnel boundary is given by the reciprocal of an arbitrarily chosen bounded,continuous and positive function φ(t)>0 for all t≥0 with<∞.The funnel is defined asTo ensure that the error e(t)evolves inside the funnel Fφ(t),theexp ression of ρ(·)can be chosen asFrom(12)we can see that,when the gain ρ(t)increases,the error e (t)approaches the boundary Fφ,and when the gain ρ(t)decreases conversely,the error e(t)becomes small.A proper funnel boundary toprescribe the performance is selected aswhere δ0≥δ∞>0,inf Fφ(t),and|e(0)|<Fφ(0).According to(11)and(12),define a new funnel error variable s1(t)aswhere the funnel boundary Fφ(t)satisfies the condition given in (13).This variable will be employed to ensure the prescribed output performance.The derivative of(14)iswhereandwhere3.2 Controller designConsidering(15)and(16),the sliding mode manifold is designed as where α>0.Differentiating s2,we haveSubstituting(8)and(16)into(18)yieldswhere the nonlinear function κ isSince κ is not ea sy to be exactly known,the modelbased controllers cannot be applied directly.Hence,we adopt a neural network(9)to approximate the nonlinear function κ.Assume that there exists a constant ideal weight matrix W∗so that the nonlinear function κ can be expres sed aswhere the input vectorIn the following,a nonsingular terminal sliding mode neural funnel control approach is developed for tracking control of the motor servo system(8).To force s2converge to zero within a finite time,thenonsingular terminal sliding mode manifold is employed aswhere β>0,p and q are positive odd integers with p<q.Substituting(19)into(21)and using(20),the controller is designed aswhere b0is the lower bound of b;p and q are positive odd integers with p <q;is the estimate of the ideal weight W∗andµis the upper bound of sum of the neural network approximation error ε andTφ(X),where=W-is the weight estimation error of the neural network.The adaptive law ofis given bywhere K is a positive definite and diagonal matrix,and ν is a positiv e constant.Substituting(22)into(19)yieldsIn this section,a lemma and a theorem is provided to show the boundedness of all signals and the stability of the system(8)inboththereachingphaseandtheslidingphase,respectively.Lemma 1 Assume that there exists a continuous positive definite function V(t)satisfying the following inequality:where n>0,0<γ<1 are constants.Then,for any given t0,V(t)satisfies the following inequality:andwith tsgiven byTheorem 1 Consider the motor servo system(8)with unknown nonlinear saturation(2),nonsingular terminal sliding manifold(21),control law(22),and weight update law(23),then1)All signals of the closed-loop system are bounded.2)The nonsingular terminal sliding manifold s2can converge to zero in finite time by using controllers(22),if the design parameterµ>εN+‖Tφ(X)‖F.3)The tracking error e will fall into proscribed boundaries.Proof 1)Choose the following Lyapunov function candidatewhere km=FφΦF>0.Differentiating(26)with respect to time and using(24),we have Substituting(23)into(27)yieldsInequality(27)implies that both s2andare bounded.Meanwhile,considering(17)and the boundedness of W∗,we can conclude s1,˙s1,andare bounded,and thus from(22)and(14),we can obtain u,e andare all bounded.Furthermore,the boundedness of yd,˙ydand¨ydcan lead to the boundedness of s2according to(17).As a result,and˙s2is bounded due to the boundedness of guξ. Therefore,all signals of the closed loop system are bounded.From(26)-(28),the stability of the system(8)with control laws(22)and weight update law(23)has been proved.However,it is not necessary for the terminal sliding manifold s2to converge to zero in finite time.Therefore,further proof should be given to guarantee that the terminalslidingmanifolds2convergetozeroin finitetime.2)From(29),we can see that the sigmoid function φ(X)is boundedby 0<φi(X)<n0,i=1,···,n1, with n0=maxTherefore,φ(X) is bounded bywhere‖·‖denotestheEuclideannormofavector,φ(X)=[φ1(X)φ2(X)···φn1(X)]T.From the property of Forensics norm,it can be obtained thatSelect another Lyapunov function candidateDifferentiating(29)with respect to time and using(24),we have Sinceµ>εN+‖Tφ(X)‖F,(30)can be rewritten asThen,we can obtainAccording to Lemma 1,it can be concluded that the fast terminal sliding manifold s2can converge to the equilibrium point within a finite timet1given by3)Once the sliding surface s2=0 is achieved.the states of system(8)will remain on it and the system has the invariant properties.On the sliding surface s2=0,we can obtainConstructing the following Lyapunov candidateand differentiating V2along(34),we haveThen,we can conclude that the funnel error s2will converge to the equilibrium point.Thus,from(14),the tracking error e will fall into the prescribed boundaries.Remark 2 Since the discontinuous switching functionsgn(·)shown in (22)may result in the chattering phenomenon,the following continuousΔ(s)is employed instead in the simulation section:whereζis a positive constant defining the thickness of the boundary layer. When|s|≥ζ,the proof can be easily accomplished according to the proof of Theorem 1.When|s|<ζ,following the proof steps in[30],we can alsoobtainandActually,there exists a small positive constantϵsuch that ϵ≤(|s|+ζ).With this modified controller,the chattering can be eliminated and the finite-time convergence is guaranteed in the whole tracking process.In this section,the following three other control approaches are presented for the performance comparison with the proposed NTSMFC scheme.1)PID controlwhere kp=20,ki=0.05,and kd=4.2)Neural-network sliding mode control(SMC)[31]where s1=e,b0=6,α=2,k1=10,andµ=0.1.3)Neural-network sliding mode control(NTSMC)[21]where s1=e,α=2,β=0.2,k1=10,p=5,q=7,b0=6 andµ=0.1.For fair comparison,all control parameters are fixed for various reference signals.The initial states of the system are x1(0)=0,x2(0)=0.The NN parameters are K=0.1,a=2,b=10,c=1,d=-10.The parameters of funnel boundary(13)are chosen as δ0=100,δ∞=0.3 and a0=3.And the control law(22),where α=2,β=0.2,k1=10,p=5,q=7,b0=6 and µ=0.1.The system is select as h=0.2x2sinx2and b=6.The saturation bound vmax=1.In the following,three different cases are performed to compare four different controllers.Case 1 Sinusoidal wave.yd=0.5sint is employed as thereference.Simulation has been conducted,and two comparative resultsare shown in Fig.3.The tracking performance and tracking errors are depicted in Fig.3(a)and Fig.3(b),respectively.AsshowninFig.3(a),whentracking the sinusoidal wave,the NTSMFC and the NTSMC have the comparatively lower overshoot,while the PID control scheme has the largest overshoot.From Fig.3(b),we can see that the NTSMFC has the smallest tracking error and fastest convergence speed;NTSMC has the largest overshoot at the beginning,and the PID scheme has the largest steady tracking error.Case 2 Sinusoidal wave with harmonic.The trackingperformanceofthereferencesignal0.5(sint+sin0.5t)is shown in Fig.4.Among the four schemes,the NTSMFC has the smallest overshoot and tracking error with the fastest convergence speed.The SMC and NTSMC have a large overshoot at the beginning,and PID control has largest tracking error when time goes to the infinity.Obviously,NTSMFC has the best performance when tracking the the reference signal 0.5(sint+sin0.5t).Case 3 Step signal.To further justify the transient performance(e.g.,overshoot),a step signal with amplitude 1 rad is employed.Control parameters are set the same as those given before.As shown in Fig.5,we can see that PID control has comparatively larger overshoot.Besides,the proposed scheme can converge within 1 s,while PID control costs 1.5 s;SMC and NTSMC cost more than 4 s to achieve the same performance.Therefore,we can conclude that the proposed NTSMFC has the best transient performance.In order to show the comparison performance more convincingly,several indices are provided to evaluate the performance of the four controllers. 1),which is the integrated absolute value of the error to measure intermediate tracking result.2)which is the integral of the time multiplied by the absolute value of the error,and used to measure the tracking performance with time behaving as a factor to emphasize errors occurring late.3)which is the integrated square error and used to demonstrate the smoothness of the profile.The simulation results in terms of performance indices are provided by Tables 1-3.From Tables 1-2,we can see that when tracking sinusoidal waves,the proposed NTSMFC scheme has the smallest IAE,ITAE and ISDE,which means it performs best among four controllers.From Table 3,it can be concluded that when tracking the step signal,although PID controller has the smallest ISDE,it has the larger ITAE and IAE than NTSMFC,while SMC and NTSMC have relatively large IAE,ITAE and ISDE. Therefore,all the aforementioned simulation results clearly show that the proposed NTSMFC scheme can achieve the best tracking performance with respect to tracking errors and convergence speed.In this paper,a nonsingular terminal sliding mode funnel control (NTSMFC)scheme is proposed to achieve a prescribed tracking performance for motor servo systems with unknown input saturation.The non-smooth saturationistransformedintoanaffineformbydefiningasmoothnon-affine function and using the mean-value theorem.A new constraint variable is employed and the tracking error will be forced to fall into prescribe boundaries.By using a simple sigmoid neural network to approximate the unknown system nonlinearity,a nonsingular terminal sliding mode funnel control is developed for the prescribed tracking performance of the motor servo system.With the proposed scheme,no prior knowledge is required on the input saturation bound,and the convergence of the position tracking error is guaranteed via the Lyapunov synthesis.Our further work is to apply the proposed scheme to a practical motor servo system.References:[1]LI S H,LIU Z.Adaptive speed control for permanent-magnet synchronous motor system with variations of load inertia[J].IEEE Transactions on Industrial Electronics,2009,56(8):3050-3059.[2]MORAWIEC M.The adaptive backstepping control of permanent magnet synchronous motor supplied by current source inverter[J]. IEEE Transactions on Industrial Informatics,2013,9(2):1047-1055.[3]LIU G,CHEN L,ZHAO W,et al.Internal model control of permanent magnet synchronous motor using support vector machine generalized inverse[J].IEEE Transactions on Industrial Informatics,2013,9(2):890-898.[4]TEE K P,REN B B,GE S S.Control of nonlinear systems with time-varying output constraints[J].Automatica,2011,47(11):2511 -2516. [5]NIU B,ZHAO J.Barrier Lyapunov functions for the output trackingcontrol of constrained nonlinear switched systems[J].Systems& Control Letters,2013,62(10):963-971.[6]LI Y,LI T,JING X.Indirect adaptive fuzzy control for input and output constrained nonlinear systems using a barrier Lyapunov function [J].International Journal of Adaptive Control and Signal Processing,2014,28(2):184-199.[7]BECHLIOULIS C P,ROVITHAKIS G A.Robust partial-state feedback prescribed performance control of cascade systems with unknown nonlinearities[J].IEEE Transactions on Automatic Control,2011,56(9):2224-2230.[8]NA J.Adaptive prescribed performance control of nonlinear systems with unknown dead zone[J].International Journal of Adaptive Control and Signal Processing,2013,27(5):426-446.[9]NA J,CHEN Q,REN X M,et al.Adaptive prescribed performance motion control of servo mechanisms with friction compensation[J]. IEEE Transactions on Industrial Electronics,2014,61(1):486-494.[10]ILCHMANA,SCHUSTERH.Trackingcontrolwithprescribedtransient behavior degree[J].Systems&Control Letters,2006,55(5): 396-406. [11]ILCHMAN A,RYAN E P,TRENN S.PI-funnel control for two mass systems[J].IEEE Transactions on Affective Computing,2009,54(4): 918-923.[12]HACKL C M,ENDISCH C,SCHRODER D.Contribution to nonidentifier based adaptive control in mechatronics[J].Robotics and Autonomous Systems,2009,57(10):996-1005.[13]HACKL C M.High-gain position control[J].International Journal of Control,2011,84(10):1695-1716.[14]HAN S I,LEE J M.Recurrent fuzzy neural network backstepping control for the prescribed output tracking performance of nonlinear dynamic systems[J].ISA transactions,2014,53(1):33-43.[15]HAN S I,LEE J M.Fuzzy echo state neural networks and funnel dynamic surface control for prescribed performance of a nonlinear dynamic system[J].IEEE Transactions on Industrial Electronics,2014,61(2):1099-1112.[16]IMURA J,SUGIE T,YOSHIKAWA T.Adaptive robust control of robot manipulators-Theory and experiment[J].IEEE Transactions on Robotics and Automation,1994,10(5):705-710.[17]XU L,YAO B.Adaptive robust precision motion control of linear motors with negligible electrical dynamics:theory and experiments [J].IEEE Transactions on Mechatronics,2001,6(4):444-452.[18]MAN Z H,YU X H.Adaptive terminal sliding mode tracking control for rigid robotic manipulators with uncertain dynamics[J].International Journal of Mechanical Systems,Machine Elements,and Manufacturing,1997,40(3):493-502.[19]FENG Y,YU X H,MAN Z H.Non-singular terminal slidng mode control of rigid manipulators[J].Automatica,2002,38(12):2159-2167. [20]YU S,YU X H,SHIRINZADEHC B.Continuous finite-time control for robotic manipulators with terminal sliding mode[J].Automatica,2005,41(11):1957-1964.[21]CHEN S Y,LIN F J.Robust nonsingular terminal sliding-mode control for nonlinear magnetic bearing system[J].IEEE Transactions on Control Systems Technology,2011,19(3):636-643.[22]PEREZ-ARANCIBIA N O,TSAO T C,GIBSON J S.Saturationinduced instability and its avoidance in adaptive control of hard disk drives[J].IEEE Transactions on Control Systems Technology,2010,18(2):368-382.[23]GAOW Z,SELMIC R R.Neural networkcontrol of a class of nonlinear systems with actuator saturation[J].IEEE Transactions on Neural Networks,2006,17(1):147-156.[24]HU Q L,MA G F,XIE L H.Robust and adaptive variable structure output feedback control of uncertain systems with input nonlinearity [J].Automatica,2008,44(4):552-559.[25]CHEN M,GE S S,HOW B.Robust adaptive neural network control for a class of uncertain MIMO nonlinear systems with input nonlinearities [J].IEEE Transactions on Neural Networks,2010,21(5):796 -812. [26]CHEN M,GE S S,REN B B.Adaptive tracking control of uncertain MIMO nonlinear systems with input constraints[J].Automatica 2011,47(3):452-455.[27]ZHANG X,WANG M,ZHAO J.Stability analysis and antiwindup design of uncertain discrete-time switched linear systems subject to actuator saturation[J].Journal of Control Theory and Applications,2012,10(3):325-331.[28]WEN C Y,ZHOU J,LIU Z T,et al.Robust adaptive control ofuncertain nonlinear systems in the presence of input saturation and external disturbance[J].IEEE Transactions on Automatic Control,2011,56(7):1672-1678.[29]WANG H,CHEN B,LIU X,et al.Adaptive neural tracking control for stochastic nonlinear strict-feedback systems with unknown input saturation[J].Information Sciences,2014,269(6):300-315.[30]LIU H T,ZHANG T.Neural network-based robust finite-time control for robotic manipulators considering actuator dynamics[J].Robotics and Computer-Integrated Manufacturing,2013,29(2):301-308.[31]CHEN Q,NAN Y R,JIN Y.Neural sliding mode control for turntable servo system with unknown deadzone[C]//Proceedings of the 32nd Chinese Control Conference.Xi’an,China,2013.陈强(1984-),男,讲师,目前研究方向为交流伺服电机控制、非线性摩擦和死区补偿、自适应神经网络控制等,E-mail:****************.cn;汤筱晴(1993-),女,目前研究方向为滑模自适应控制,E-mail:**********************.。
英语翻译
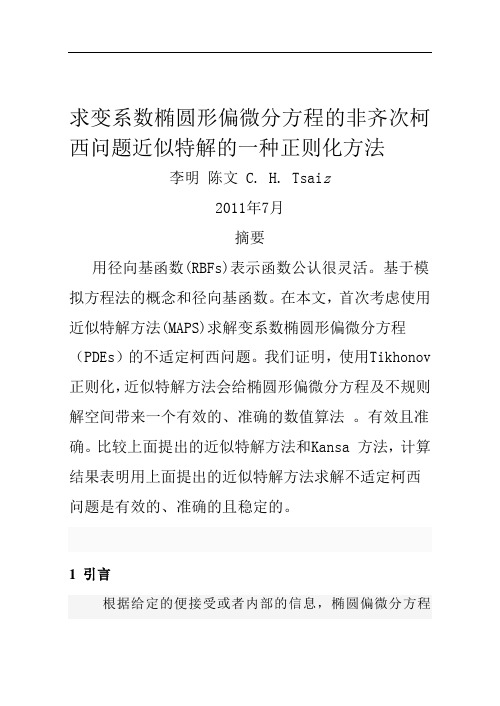
求变系数椭圆形偏微分方程的非齐次柯西问题近似特解的一种正则化方法李明陈文 C. H. Tsai z2011年7月摘要用径向基函数(RBFs)表示函数公认很灵活。
基于模拟方程法的概念和径向基函数。
在本文,首次考虑使用近似特解方法(MAPS)求解变系数椭圆形偏微分方程(PDEs)的不适定柯西问题。
我们证明,使用Tikhonov 正则化,近似特解方法会给椭圆形偏微分方程及不规则解空间带来一个有效的、准确的数值算法。
有效且准确。
比较上面提出的近似特解方法和Kansa 方法,计算结果表明用上面提出的近似特解方法求解不适定柯西问题是有效的、准确的且稳定的。
1 引言根据给定的便接受或者内部的信息,椭圆偏微分方程(PDEs) 大致可以分为以下五个主要问题:1.边界值问题[2,6]。
这些问题已经发展和应用很长一段时间。
例如:Dirichlet, Neumann 或者 Robin 混合问题。
2.Cauchy问题[23]。
这些问题也被称为边值的确定问题。
对于椭圆形Cauchy问题,Dirichlet和Neumann边界条件只在解空间边界的一部分给定。
3.边界的确定问题[11]。
这些是工程上典型的非破坏性测试问题。
这些问题确定解空间的未知边界。
4.系数的重建问题[26]。
5.潜在源的确定问题。
上述问题中。
只有第一个问题是适定的。
适定问题的定义是由Hadamard【7】给出的。
他认为,为某物理现象建立的合适的数学模型应具有以下三个属性:存在,唯一且稳定。
如若其中之一不满足,则建立在物理现象之上的这个数学模型就是不适定的。
然而,越来越多的不适定问题正出现于科学cs,中国香港特别行政区,香港城市大学相关作者,中国南京河海大学工程力学系型的逆问题,从Hadamard角度上来说,他们是不适定的,因为测量数据任何小误差都可能导致解的大差距。
偏微分方程的系数通常对应于问题的材料参数。
在非均匀介质中,材料参数可能会随位置而变化。
因此在非均匀介质的物理问题的控制方程中很可能会涉及到变系数。
AISSVOL5VOL1-AICIT
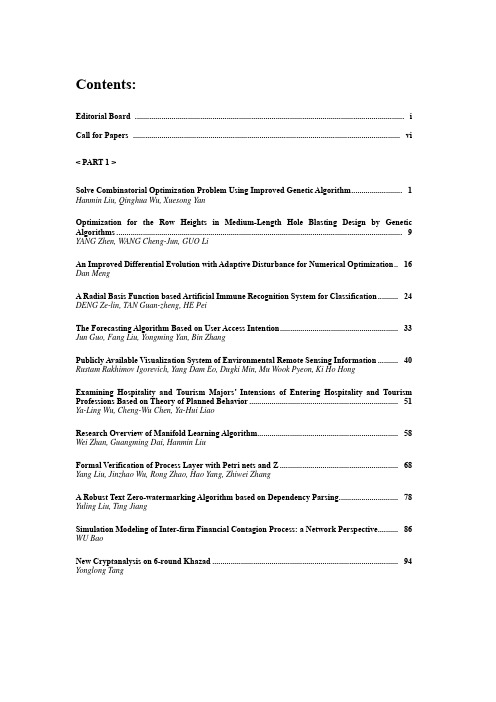
Contents:Editorial Board (i)Call for Papers (vi)< PART 1 >Solve Combinatorial Optimization Problem Using Improved Genetic Algorithm (1)Hanmin Liu, Qinghua Wu, Xuesong YanOptimization for the Row Heights in Medium-Length Hole Blasting Design by Genetic Algorithms (9)YANG Zhen, WANG Cheng-Jun, GUO LiAn Improved Differential Evolution with Adaptive Disturbance for Numerical Optimization .. 16 Dan MengA Radial Basis Function based Artificial Immune Recognition System for Classification (24)DENG Ze-lin, TAN Guan-zheng, HE PeiThe Forecasting Algorithm Based on User Access Intention (33)Jun Guo, Fang Liu, Yongming Yan, Bin ZhangPublicly Available Visualization System of Environmental Remote Sensing Information (40)Rustam Rakhimov Igorevich, Yang Dam Eo, Dugki Min, Mu Wook Pyeon, Ki Ho HongExamining Hospitality and Tourism Majors’ Intensions of Entering Hospitality and Tourism Professions Based on Theory of Planned Behavior (51)Ya-Ling Wu, Cheng-Wu Chen, Ya-Hui LiaoResearch Overview of Manifold Learning Algorithm (58)Wei Zhan, Guangming Dai, Hanmin LiuFormal Verification of Process Layer with Petri nets and Z (68)Yang Liu, Jinzhao Wu, Rong Zhao, Hao Yang, Zhiwei ZhangA Robust Text Zero-watermarking Algorithm based on Dependency Parsing (78)Yuling Liu, Ting JiangSimulation Modeling of Inter-firm Financial Contagion Process: a Network Perspective (86)WU BaoNew Cryptanalysis on 6-round Khazad (94)Yonglong TangA New Risk Assess Model for Urban Rail Transit Projects (104)Zhu Xiangdong, Xiao Xiang, Wu ChaoranAnt Colony Algorithm Optimized by Vaccination (111)He Haitao, Xin NingComputer Network Security and Precaution Evaluation based on Incremental Relevance Vector Machine Algorithm and ACO (120)Guangyuan SongRealization of an Embedded and Automated Performance Testing System for a MEMS Transducer (128)LIAO Hai-yang, XIONG Kui, WEN Zhi-yuMulti-level Cache Prediction and Partitioning Mechanism in CMP (135)Shuo Li, Gaochao Xu, Xiaozhong Geng, Xiaolin Qiao, Feng WuNew Classes of Sequences for Encryption Procedures in Symmetric Cryptography (145)Amparo Fuster-SabaterExplore and Analysis of Environmental Policy Based on Green Industry Development (152)Chunhong Zhu, Zhe Liu, Yue Zhou, Xuehua ZhangResearch on Service Encapsulation of Manufacturing Resources Based on SOOA (158)Lingjun Kong, Wensheng Xu, Nan Li, Jianzhong ChaA Twice Ant Colony Algorithm Based on Simulated Annealing for Solving Multi-constraints QoS Unicast Routing (167)Yongteng Lv, Yongshan Liu, Wei Chen, Xuehui Shang, Yuanyuan Han, Chang LiuA Two-phase Multi-Constraint Web Service Selection Approach for Web Service Composition (176)Zhongjun Liang, Hua Zou, Fangchun Yang, Rongheng LinAdvanced Coupled Map Lattice Model for the Cascading Failure on Urban Street Network . 186 ZHENG Li, SONG Rui, Xiao YunTransition Probability Matrix Based Tourists Flow Prediction (194)Yuting Hu, Rong Xie, Wenjun ZhangA Study on the Macro-Control Policy of China Real Estate Development (202)Lu ShiAn Effective Construction of a Class of Hyper-Bent Functions (212)Yu Lou, Feng Zhou, Chunming TangSpeaker-independent Recognition by Using Mel Frequency Cepstrum Coefficient and Multi-dimensional Space Bionic Pattern Recognition (221)Guanglin Xian, Guangming XianIntelligent Decision Support System (IDSS) for Cooling/Heating Sources Scheme Selection of City Buildings Based on AHP Method (228)Liu Ying, Jiang Kun, Jiang ShaModeling of Underwater Distributed Target Based on FDTD and Its Scale Characteristic Extraction (237)P AN Yu-Cheng, SHAO Jie, ZHAO Wei-Song, ZHONG Ya-QinMethod for Dynamic Multiple Attribute Decision Making under Interval Uncertainty and its Application to Supplier Selection (246)Xu JingStudy on Multi-Agent Information Retrieval Based on Concern Domain (254)Sun JianmingA New Method for Solving Numerical Solution of Fractional Differential Equations (263)Jianping Liu, Xia Li, HuiQuan Ma, XueZhi Mao Guoping ZhenAssessment and Analysis of Hierarchical and Progressive Bilingual English Education Based on Neuro-Fuzzy approach (269)Hao Xin< PART 2 >Computer-based Case Simulations in China: 2001-2011 (277)Tianming Zuo, Peng Wang, Baozhi Sun, Jin Shi, Yang Zhang, Hongran BiHybrid Monte Carlo Sampling Implementation of Bayesian Support Vector Machine (284)Zhou Yatong, Li Jin, Liu LongA Face Recognition Method based on PCA and GEP (291)WANG Xue-guang, CHEN Shu-hongResearch and Application of Higher Vocational College Library Personalized Information Service Based on Cloud Computing (298)Meiying Nie, XinJuan Zhou,Qingzhi WenA Calculation Model for the Rover’s Coverage Boundary on the Lunar Surface Based on Elevation (307)Hu Yasi, Meng Xin, Pan Zhongshi, Li Dalin, Liang Junmin, Yang YiAn Evaluation of firms’ Best Strategies with the ANP, AHP and Sensitivity Test Approaches 316 Catherine W. Kuo, Shun-Chiao ChangDesign of Embedded Vehicle Safety Monitoring System (326)Jing-Lian, Lin-Hui Li, Hu-Han, Ya-Fu Zhou, Feng-Hu, Ze-Quan ZengThe Approach to Obtain the Accurate TOA of VHF Lightning Signals Based on FastICA Algorithm (334)Xuquan Chen, Wenguang ZhaoIntegrating Augmented Reality into Consumer’ Tattoo Try-on Experience (341)Wen-Cheng Wang, Hao-Hsiang Ku, Yen-Wu TiResearch on Location for Emergency Logistics Center Based on Node Cost (348)Wang ShouqiangLogistics Terminal Facility Location Model Based on Customer Value in Competitive Environment (354)Han Shuang Wang XiaoxiaA Decision Support Model for Risk Analysis with Interval-valued Intuitionistic Fuzzy Information (362)Guoqing WuPeak to Average Power Ratio Reduction with Bacterial Foraging Algorithm for OFDM Systems (370)Jing Gao, Jinkuan Wang, Bin WangUsing Linear and Nonlinear Inversion Algorithm Combined with Simple Dislocation Model Inversion of Coal Mine Subsidence Mechanism (379)Yu-Feng ZHU,Qin-Wei WU,Tie-Ding Lu, Yan LuoApplication of Multi- media in Education of Schoolgirl’s Public P. E. in College and University—set Popular Aerobics as Example (388)Yuanchao zhouA Fuzzy Control System for Trailers Driven by Multiple Motors in Side Slipway to Launch and Pull Out Ships (395)Nyan Win Aung, Wei HaijunA Novel Image Encryption Algorithm based on Virtual Optical Imaging and Hyper-chaos .. 403 Wei Zhu , Geng Yang, Lei Chen, Zheng-Yu ChenThe Structure Character of Market Sale Price in the Coordinating Supply Chain (412)Jun Tian, Zhichao WangDesign Parameter Analysis for Inducers (419)Wei Li, Weidong Shi, Zhongyong Pan, Xiaoping Jiang, Ling ZhouAutomatic Recognition of Chinese Traffic Police Gesture Based on Max-Covering Scheme .. 428 Fan Guo, Jin Tang, Zixing CaiDetermination of Acrylamide Contents in Fried Potato Chips Based on Colour Measurement 437 Peng He, Xiao-Qing Wan, Zhen zhou, Cheng-Lin WangAn Efficient Method of Secure Startup and Recovery for Linux (446)Lili Wu, Jingchao Liu,Research on Life Signals Detection Based on Parallel Filter Bank and Higher Order Statistics (454)Jian-Jun LiResearch for Enterprise Logistics Dynamic Optimization Based on the Condition of Production Ability Limited (462)Guo QiangTechnological Progress in Macroeconomic Volatility and Employment Impact analysis - Based on Endogenous Labor RBC Model (469)Wang Qine, Hu honghaiResearch on Bottleneck Identification in Multiple Products Small lot Production Logistics of Manufacturing Enterprise Based on TOC (476)Jian Xu, Hongbo WangThe Spiral Driven and Control Method Research of the Pipe Cleaning Robot (484)Quanyu Yu, Jingyuan Yu, Jun Wang, Jie LiuHoisting Equipment of Coal mine Condition Monitoring and Early Warning Based on BP Neural Network (491)Shu-Fang Zhao, Li-Chao ChenSpatial Temporal Index-based Historic Closing Event Query or Moving Objects (497)Xianbiao Ji, Hong Mi, Zheping ShaoResearch on the Risk-sharing Mechanism of Energy Management Contract Project in Building Sector (510)CaiWeiguang, Ren Hong, Qin BeibeiFinancial Crisis and Financial Index Structure Break (516)Keng-Hsin Lo, Yen-Chang Chen, Yi -Wei ChuangThe Research of Cooling System for the High-Energy Storage Flywheel (522)Wang Wan, He LinNumerical Models and Seismic Design of Steel Frames Equipped with Supplemental Fluid Viscous Devices (528)Marco ValenteStudy on Positive and Dynamic Enterprise Crisis Management based on Sustainable Business Model Innovation (535)Shi-chang Fu, Hui-fen Wang, Dalen ChiangQuantitatively Study on the Mechanism of Cooperating Profit Distribution within Business Ecosystem (544)Bin HuStudy on the Landscape Design of Urban Commemorative Squares Based on Sustainable Development (552)Wenting Wu, Ying Li, Yi Ren< PART 3 >SRPMS: A web-based Project Management System for Scientific Research (559)Yanbao Ji, Xiaopeng Yun, Zhao Jun, Quanjiang Bai, Lingwang GaoEmpirical Study on Influence Factors of Carbon Dioxide Emissions in Liaoning Province based on PLS (567)Yu-xi Jiang, Su-yan He, Xiang-chao WeiHarmony Factor Considered Evaluation of Science Popularization Talents Based on Grey Relational Analysis Model (575)Li MingStudy on the Safety of High-Speed Trains under Crosswind (582)Xian-Liang Sun, Bin-Jie Wang, Ming Gong, San-San Ding, Ai-Qing TianControl Method of Giant Magnetostrictive Precise Actuator Based on the Preisach Hysteresis Theory (589)Yu Zhang, Huifang Liu, Feng SunDynamic Modeling and Characteristics Analysis of Rolls along Axial Direction for Four High Mill Based on Timoshenko Theory (602)Jian-Liang Sun, Yan PengConstruct on Maintenance Requirement Analysis Model of Pavement Management System 610 Xiu-shan Wang, Yun-fang YangAutomatically Generate Test Data Based on Intelligent Algorithms Method (617)Jian Ni, Ning-NingYangAnalysis of the Functions of a High-Speed Railway Station in China (623)Li-Juan Wang, Tian-Wei Zhang, Fan Wang, Qing-Dong ZhouEconometric Analysis of Expectation in Savings-to-Investment in Capital Market Converting Process (630)Wang Yantao , Yu Lihua ,Mao BeibeiAnalysis Model and Empirical Research on Product Innovation Process of Manufacturing Enterprises Based on Entropy-Topsis Method (637)Hang Yin, Bai-Zhou Li, Tao Guo, Jian-Xin ZhuThe Market Analysis and Prediction of Chinese Iron and Steel Industry (645)Li Xiaohan, Sun Qiubai , Li HuaSystem Dynamics Mode Construction and System Simulation in the Product Innovation Process (653)Jian-Xin Zhu, Jun DuResearch on Organization Innovation of Enterprise Based on Complexity Theory (664)Yu Zheng, Tao GuoThe Study on TFP of Iron and Steel Industry inChina Based on DEA-Malmquist Productivity Index Model (672)Xiaodong Dong, Yuzhi ShenResearch and Implementation of Energy Balance Control System in Metallurgy Industry (681)Qiu DongA Study of Opportunities and Threats in the Implementation of International Marketing for Production Design of Corporate Brand Licensing – A Case Study of POP 3D Co., Ltd. (689)Min-Wei Hsu,Tsai-Yun Lo,Liang,K.C.Research on Risk Forming Mechanism and Comprehensive Evaluation of the Enterprise Group (695)Dayong XUMode Construction of Dining Reform in Universities Based on Theory of Institutional Transformation (704)Li PingjinResearch on QR Decomposition and Algorithm of Linguistic Judgment Matrix (711)Lu YuanA Comparison of the Mahalanobis-Taguchi System to A Selective Naïve Bayesian Algorithm for Semiconductor Chemical Vapor Deposition Process (720)Jui-Chin Jiang, Tai-Ying LinStudy of Policy-making Model for Producer Service: Empirical Research in Harbin (730)Xin Xu, Yunlong DingKrein space H∞ filtering for initial alignment of SINS with large azimuth misalignment (738)Jin Feng, Fei Yu, 3Meikui Zou, Heming JiaInternet Word-Of-Mouth on Consumer Online Purchasing Behavior Analysis in China (747)Jie Gao, Weiling YeConvex Relaxation for Array Gain/Phase Calibration in ULAs and UCAs with Unknown Mutual Coupling (758)Shu CaiFinancial-Industrial Integration Risk Management Model of Listed Companies Base on Logistic (767)Ke WenBehavior Equilibrium Analysis for The Cross-Organizational Business Process Reengineering in Supply Chain (775)Jianfeng Li, Yan ChenA Secure Scheme with Precoding Approach in Wireless Sensor Networks (782)Bin Wang, Xiao Wang, Wangmei GuoA New Method on Fault Line Detection for Distribution Network (789)Bo LiManagers’ Power and Earnings Manipulating Preference (796)J. Sun, X. F. Ju, Y. M. Peng, Y. ChangStudy and Application of the Consistency of Distributed Heterogeneous Database Based on Mobile Agent (804)Zhongchun Fang, Hairong Li, Xuyan Tu。
工程常用英语词汇

目录1、电力设计基本术语2、给水排水设计基本术语3、水泵专业英语词汇4、阀门种类英汉术语对照5、阀门专用英语词汇6、照明术语7、工程结构设计基本术语电力设计基本术语abrasion-Proof component of burner 燃烧器耐磨件arm-brace 撑脚ash conditoner 调灰器basket removal panel 元件盒检修护板BDV blow down valve 疏水阀,排污阀blind 堵板blind flange 法兰堵板/盲板法兰(盖calling 催交campell diagram 叶片埃贝尔曲线dado 墙裙daily service fuel tank level switch 日用油缸液位掣damage 损毁damper 挡板damper linkage 风闸联动装置damper motor 风闸马达damping mat 阻尼垫dangerous earth potential 危险性对地电势dashpot 减震器data transmission 数据传输DC/AC converter 直流电/交流电转换器dead 不带电dead weight 自重decanter 沉淀分取器declaration of conformity 符合标准声明decommissioning 解除运作;停止运作decompression chamber 减压室decorative lighting 装饰照明;灯饰deep bore well pump 深钻井泵defect liability period 故障修理责任期;保用期defectograph 钢缆探伤仪;故障检查仪defence in depth 纵深防御definite sequence 固定次序deflection 偏转;挠度deflector sheave 折向轮;导向轮defrost timer 防霜时间掣defrost unit 溶雪组合dehumidifier 抽湿机deleterious substance 有害物质delivery and return air temperature 送风及回风温度delivery connection 出油接头delivery pressure 输出压力demand side management 用电需求管理demand side management agreement 用电需求管理协议demand side management programme 用电需求管理计划dent 凹痕dental instrument 牙科仪器dental scaler 洗牙具Departmental Administration Division [Electrical and Mechanical Services Department] 行政部〔机电工程署〕Departmental Safety Unit [Electrical and Mechanical Services Department] 部门安全组〔机电工程署〕deposition 沉积物depth measuring facility 深度测量装置derating factor 额定值降低因子derust 除锈descale 清除氧化皮design current 设计电流design parameter 设计参数designated employee 指定雇员detachable grip 可拆除的夹扣Details of Branch Offices of Registered Electrical Contractors 注册电业承办商分行详情申报deterioration 变质;变坏Deutsche Industrie Normen [DIN] 德国工业标准device 器件;装置dewatering 脱水;排水diaphragm 膜片;隔板dielectric strength test 电介质强度测试diesel fuel tank 柴油燃料缸diesel oil 柴油differential gasket 差速器衬垫differential lock 差速器锁differential oil 差速器机油diffuser 透光罩;扩散器dilute 稀释dim sum trolley 点心手推车dim transformer 光暗变压器diminution of value 减值dimmer 调光器;光暗掣;光暗器dip tube 液位探测管Diploma in Electrical Engineering 电机工程学文凭dipstick 量油尺direct current [DC] 直流电direct current control 直流控制direct current electric drive 直流电电力驱动direct current reactor 直流电抗器direct drive 直接驱动direct purging 直接驱气direct-acting lift 直接驱动升降机direct-fired vaporizer 明火直热式汽化器direction arrow 方向箭头direction arrow plate 方向指示板direction indicator 方向指示器Director of Electrical and Mechanical Services 机电工程署署长Directory of Accredited Laboratories 认可实验所名册Directory of Quality System Registration Bodies 品质系统注册团体指南disassemble 拆散discharge 放电;卸载discharge lamp 放电灯;放电管discharge lighting 放电照明设施discharge of electricity 释电;放电discharge valve 排水阀disciplinary board 纪律审裁委员会disciplinary board panel 纪律审裁委员团disciplinary tribunal 纪律审裁小组disciplinary tribunal panel 纪律审裁委员团;纪律审裁委员会discolouring 变色disconnection 截断;截离steam hamerring analysis 汽锤分析steam packing unloading valve 汽封卸载阀steam purity 蒸汽纯度steam seal diverting valve 汽封分流阀steam seal feed valve 汽封给水阀steam water mixture 汽水混合物steel bar 扁钢steel supporting 钢支架steel wire brush 钢丝轮steel works 钢结构step load change 负荷阶跃still air 蒸馏气体stirrup 镫形夹stoikiometric ratio 化学当量比stopper 制动器、塞子storage vessell 贮水箱stppage alarm 停转报警stranded copper cable 铜绞线电缆strength 强度strong backs 支撑stud bolt 柱头螺栓、双头螺栓sub cooling line 欠热管submerged arc welding 埋弧焊substation 配电装置substation island 电气岛superficial corrosion 表面腐蚀superheat 过热度supersaturation 过饱和supervisory instrument 监测装置supply transformer 供电变压器support trunnion 支撑端轴surfactant 表面活性剂surge 喘振suspended diode 中断二极管suspended particles 悬浮颗粒switch board 开关柜switch gear 开关柜sychronization 并网sychroscope 同步指示器、同步示波器T square 丁字尺T/G transformer 发变组tackling system 起吊系统tamped/compacted backfill 夯实回填土tanks and accessories 箱罐和附件taper land thrust bearing 斜面式推力轴承tar epoxy paint 柏油环氧漆tarpaulin 防水布temperature digital display meter 温度数显表tensile test 拉伸试验tension test 拉伸试验,张力试验tensioning rod 拉杆terminal box 接线盒terminal poit 接口termination flange 接口法兰tertiary air 三次风test connection 试验接头test permition 试验合格the expansion coordinate system 热膨胀系统theodilite\transit instrument 经纬仪thermal insulatiion for tuebine casing 汽缸保温thermo resistor 热电阻thermostat 恒温器、恒温调节器thinner 稀释剂threaded flange 螺纹法兰throudh type 直通式、穿入式through bolt 贯穿螺栓、双头螺栓thrust plate 推力板tier tube 间隔管tilting pad 可倾瓦块tilting pad bearing 可倾瓦块轴承tip shroud 围带、环形叶栅外柱面tip speed 叶顶速度toe board/plate (kick plate) 踢脚板top crown plate seal 高冠板式密封装置top girder 顶板top penthouse 顶部雨棚top plan view 俯视图torquemeter 扭矩测量仪totalnumber of welding 焊口总数trajectory 轨道、轨迹transducer board 变送器屏transfer pipe 引出管transition piece 过渡连接件transtion piece 过渡段transverse strength 弯曲强度、抗挠强度transverse stress 横向应力、弯曲应力transverse test 抗弯试验trapezoid corrugated plate seperater 梯形波形板分离器、顶帽travelling crab 小车起重机travelling hoist 移动卷扬机tread width 踏步宽度trestle 组合支架trim and grind the welding 修磨焊点trisector air preheater 三分仓空预器trunk cable pair 主电缆对trunnion air seal assembly 端轴空气密封tube exchanger 管式热交换器tubing stress analysis 管系应力分析turbidity analyser 浊度分析仪turbine lube oil and conditioning system 汽机润滑油及净化系统turning oil 循环油twisted pair conveyer 双绞线传送器undercut 坡口underflow 地流、潜流、下溢union 活接头、管节unit control 单元控制unloadding spout vent fan 卸料口通风风机unloading valve 卸载阀urgent need equipment 急需设备urgtented need equipment 急需设备u-shape hanger chains u形曲链片吊挂装置UT ultrasonic testing 超声波探伤UTS ultimate tensible strength 极限抗拉强度vacuum belt filter 皮带真空吸滤器valve opening chart at load rejection 甩负荷阀门开启阀valve seat body seat 阀座valve spindle 阀轴、阀杆valve stem 阀杆vapor proof 防水灯variable inlet guide vane centrifugal fan 进口可调导叶离心式风机variable moning blade axial flow fan 动叶可调轴流式风机variable moving blade double stage axial fan 动叶可调双级轴流式风机variable speed driver 变速马达variables 变量vent capacity 排放量vent line 放气管ventilator valve 通风阀vernier caliper 游标卡尺vertical deflection 垂直挠度vertical movement 垂直位移vertical spindle coal pulveriser 立式磨煤机vibration isolation 隔振装置viewing lamp 观察指示灯viscosity 粘滞度、内摩擦viscous fluid 粘性液体visual examination of coating 外观质量vlve body 阀体void 无效volatily 挥发分voltage class 电压等级vortex gasket 涡流垫片wall type and retractable soot blower 墙式、伸缩式吹灰器warm air curtain 热风幕rwarming line 加热管water balance 水平衡water induction prevent control 防进水控制water level gauge 水位计water stop flange 止水法兰water supply facility island 水工岛wear hardness 可抗磨能力wear template 防磨板wearing bush 防磨套wearing plate 防磨板、护板weigh feeder 重量计量进料器weld bolt 焊接螺栓weld contamination 焊接杂质weld groove 焊缝坡口weld pass 焊道weld penetration 熔深weld preparation 焊缝坡口加工weld with shop beveled ends 工厂加工坡口焊接welder helment 面罩welding line 焊缝welding plate flange 焊接板式法兰welding rod 焊条welding rods dryer barrel 焊条保温筒welding run 焊道welding seam 对接焊缝welding technological properties 焊接工艺性能welding tool 电焊钳welding torch 焊枪welding wire 焊丝welds counting quantity 焊口统计数量wellington boot 防水长统靴whirl plate 折流板wide column 宽立柱winding resistance 绕组电阻wire feed speed 送丝速度wire netting/metal mesh 铁丝网wire wool 擦洗用的)钢丝绒,百洁丝withstand voltage test 耐压试验working medium 工质worm hole (焊缝)条虫状气孔yield strength 屈服强度yoke 磁轭、人孔压板、座架联板firproof paint 防火漆manifold valve 汇集阀saw trace 锯痕tapping point 取样点bushing current transformer 套管式电流互感器light gauge plate/sheet 薄钢板notch 槽口、凹口holding strip 压板straight edge 校正装置trailing edge 后缘lance 喷枪lighting off 点火gaseous fuel 气体燃料entrain 夹带、传输combustion air 助燃风hot stand by 热备用行波travelling wave模糊神经网络fuzzy-neural network神经网络neural network模糊控制fuzzy control研究方向research direction副教授associate professor电力系统the electrical power system大容量发电机组large capacity generating set输电距离electricity transmission超高压输电线supervltage transmission power line 投运commissioning行波保护Traveling wave protection自适应控制方法adaptive control process动作速度speed of action行波信号travelling wave signal测量信号measurement signal暂态分量transient state component非线性系统nonlinear system高精度high accuracy自学习功能selflearning function抗干扰能力antijamming capability自适应系统adaptive system行波继电器travelling wave relay输电线路故障transmission line malfunction仿真simulation算法algorithm电位electric potential短路故障short trouble子系统subsystem大小相等,方向相反equal and opposite in direction 电压源voltage source故障点trouble spot等效于equivalent暂态行波transient state travelling wave偏移量side-play mount电压electric voltage附加系统add-ons system波形waveform工频power frequency延迟变换delayed transformation延迟时间delay time减法运算subtraction相减运算additive operation求和器summator模糊规则fuzzy rule参数值parameter values可靠动作action message等值波阻抗equivalent value wave impedance附加网络additional network修改的modified反传算法backpropagation algorithm隶属函数membership function模糊规则fuzzy rule模糊推理fuzzy reasoning样本集合sample set给定的given模糊推理矩阵fuzzy reasoning matrix采样周期sampling period三角形隶属度函数Triangle-shape grade of membership function 负荷状态load conditions区内故障troubles inside the sample space门槛值threshold level采样频率sampling frequency全面地all sidedly样本空间sample space误动作malfunction保护特性protection feature仿真数据simulation data灵敏性sensitivity小波变换wavelet transformation神经元neuron谐波电流harmonic current电力系统自动化power system automation继电保护relaying protection中国电力China Power学报journal初探primary exploration标准的机组数据显示(Standard Measurement And Display Data) 负载电流百分比显示Percentage of Current load(%)单相/三相电压Voltage by One/Three Phase (Volt.)每相电流Current by Phase (AMP)千伏安Apparent Power (KVA)中线电流Neutral Current (N Amp)功率因数Power Factor (PF)频率Frequency(HZ)千瓦Active Power (KW)千阀Reactive Power (KVAr)最高/低电压及电流Max/Min. Current and Voltage输出千瓦/兆瓦小时Output kWh/MWh运行转速Running RPM机组运行正常Normal Running超速故障停机Overspeed Shutdowns低油压故障停机Low Oil Pressure Shutdowns高水温故障停机High Coolant Temperature Shutdowns起动失败停机Fail to Start Shutdowns冷却水温度表Coolant Temperature Gauge机油油压表Oil Pressure Gauge电瓶电压表Battery Voltage Meter机组运行小时表Genset Running Hour Meter怠速-快速运行选择键Idle Run – Normal Run Selector Switch运行-停机-摇控启动选择键Local Run-Stop-Remote Starting Selector Switch其它故障显示及输入Other Common Fault Alarm Display and input给水排水设计基本术语一、通用术语给水排水工程的通用术语及其涵义应符合下列规定:1、给水工程water supply engineering 原水的取集和处理以及成品水输配的工程。
Adaptive Dynamic Surface Control for Uncertain Nonlinear Systems With Interval Type-2 Fuzzy Neural
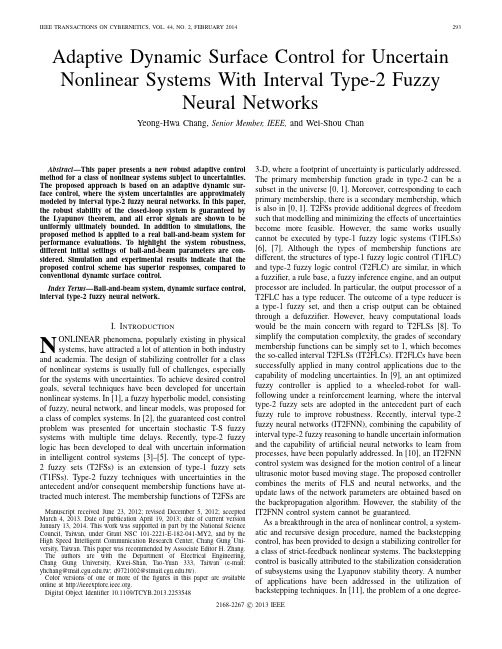
Adaptive Dynamic Surface Control for Uncertain Nonlinear Systems With Interval Type-2FuzzyNeural NetworksYeong-Hwa Chang,Senior Member,IEEE,and Wei-Shou ChanAbstract—This paper presents a new robust adaptive control method for a class of nonlinear systems subject to uncertainties. The proposed approach is based on an adaptive dynamic sur-face control,where the system uncertainties are approximately modeled by interval type-2fuzzy neural networks.In this paper, the robust stability of the closed-loop system is guaranteed by the Lyapunov theorem,and all error signals are shown to be uniformly ultimately bounded.In addition to simulations,the proposed method is applied to a real ball-and-beam system for performance evaluations.To highlight the system robustness, different initial settings of ball-and-beam parameters are con-sidered.Simulation and experimental results indicate that the proposed control scheme has superior responses,compared to conventional dynamic surface control.Index Terms—Ball-and-beam system,dynamic surface control, interval type-2fuzzy neural network.I.IntroductionN ONLINEAR phenomena,popularly existing in physical systems,have attracted a lot of attention in both industry and academia.The design of stabilizing controller for a class of nonlinear systems is usually full of challenges,especially for the systems with uncertainties.To achieve desired control goals,several techniques have been developed for uncertain nonlinear systems.In[1],a fuzzy hyperbolic model,consisting of fuzzy,neural network,and linear models,was proposed for a class of complex systems.In[2],the guaranteed cost control problem was presented for uncertain stochastic T-S fuzzy systems with multiple time delays.Recently,type-2fuzzy logic has been developed to deal with uncertain information in intelligent control systems[3]–[5].The concept of type-2fuzzy sets(T2FSs)is an extension of type-1fuzzy sets (T1FSs).Type-2fuzzy techniques with uncertainties in the antecedent and/or consequent membership functions have at-tracted much interest.The membership functions of T2FSs are Manuscript received June23,2012;revised December5,2012;accepted March4,2013.Date of publication April19,2013;date of current version January13,2014.This work was supported in part by the National Science Council,Taiwan,under Grant NSC101-2221-E-182-041-MY2,and by the High Speed Intelligent Communication Research Center,Chang Gung Uni-versity,Taiwan.This paper was recommended by Associate Editor H.Zhang. The authors are with the Department of Electrical Engineering, Chang Gung University,Kwei-Shan,Tao-Yuan333,Taiwan(e-mail: yhchang@.tw;d9721002@.tw).Color versions of one or more of thefigures in this paper are available online at .Digital Object Identifier10.1109/TCYB.2013.22535483-D,where a footprint of uncertainty is particularly addressed. The primary membership function grade in type-2can be a subset in the universe[0,1].Moreover,corresponding to each primary membership,there is a secondary membership,which is also in[0,1].T2FSs provide additional degrees of freedom such that modelling and minimizing the effects of uncertainties become more feasible.However,the same works usually cannot be executed by type-1fuzzy logic systems(T1FLSs) [6],[7].Although the types of membership functions are different,the structures of type-1fuzzy logic control(T1FLC) and type-2fuzzy logic control(T2FLC)are similar,in which a fuzzifier,a rule base,a fuzzy inference engine,and an output processor are included.In particular,the output processor of a T2FLC has a type reducer.The outcome of a type reducer is a type-1fuzzy set,and then a crisp output can be obtained through a defuzzifier.However,heavy computational loads would be the main concern with regard to T2FLSs[8].To simplify the computation complexity,the grades of secondary membership functions can be simply set to1,which becomes the so-called interval T2FLSs(IT2FLCs).IT2FLCs have been successfully applied in many control applications due to the capability of modeling uncertainties.In[9],an ant optimized fuzzy controller is applied to a wheeled-robot for wall-following under a reinforcement learning,where the interval type-2fuzzy sets are adopted in the antecedent part of each fuzzy rule to improve robustness.Recently,interval type-2 fuzzy neural networks(IT2FNN),combining the capability of interval type-2fuzzy reasoning to handle uncertain information and the capability of artificial neural networks to learn from processes,have been popularly addressed.In[10],an IT2FNN control system was designed for the motion control of a linear ultrasonic motor based moving stage.The proposed controller combines the merits of FLS and neural networks,and the update laws of the network parameters are obtained based on the backpropagation algorithm.However,the stability of the IT2FNN control system cannot be guaranteed.As a breakthrough in the area of nonlinear control,a system-atic and recursive design procedure,named the backstepping control,has been provided to design a stabilizing controller for a class of strict-feedback nonlinear systems.The backstepping control is basically attributed to the stabilization consideration of subsystems using the Lyapunov stability theory.A number of applications have been addressed in the utilization of backstepping techniques.In[11],the problem of a one degree-2168-2267c 2013IEEEFig.1.Structure of IT2FNN.of-freedom master-slave teleoperated device was discussed by the adaptive backstepping haptic control approach.The adaptive backstepping control was also applied to a plane-type 3-DOF precision positioning table,in which the unmodeled nonlinear effects and parameter uncertainties were considered [12].In[13],a backstepping controller design was investigated for the maneuvering of helicopters to autonomously track predefined trajectories.In[14],a voltage-controlled active magnetic bearing system was addressed,where a robust con-troller was designed to overcome unmodeled dynamics and parametric uncertainties by backstepping techniques.In[15], a backstepping oriented nonlinear controller was proposed to improve the shift quality of vehicles with clutch-to-clutch gearshifts.In[16],theflocking of multiple nonholonomic wheeled robots was studied,where distributed controllers were proposed with the backstepping techniques,graph theory and singular perturbation theory.The position control of a highly-nonlinear electrohydraulic system was considered,where an indirect adaptive backstepping was utilized to deal with param-eter variations[17].In[18],a neural network and backstepping output feedback controller was proposed for leader-follower-based multirobot formation control.In[19],an adaptive back-stepping approach was presented for the trajectory tracking problem of a closed-chain robot,where radial basis function neural networks were used to compensate complicated non-linear terms.In[20],an adaptive neural network backstepping output-feedback control was presented for a class of uncertain stochastic nonlinear strict-feedback systems with time-vary delays.Although backstepping control can be applied to a wide range of systems,however,there is a substantial drawback of the explosion of terms with conventional backstepping techniques.The dynamic surface control(DSC)has been proposed to overcome the“explosion of terms"problem, caused by repeated differentiations.Similar to the backstep-ping approach,DSC is also an iteratively systematic design procedure.The complexity arisen from the explosion terms in conventional backstepping control methods can be avoided by introducingfirst-order low passfilters[21]–[23].In[24], an integrator-backstepping-based DSC method for a two-axis piezoelectric micropositioning stage was proposed,where the robustness of tracking performance can be improved.In[25],a feedback linearization strategy including the dynamic surface control was developed to improve paper handling performance subject to noises.In[26]and[27],an adaptive DSC was adopted for mobile robots,where the exact parameters of robot kinematics and dynamics including actuator dynamics were assumed to be unknown a priori.In[28],a T-S fuzzy model based adaptive DSC was proposed for the balance control of a ball-and-beam system.Based on the T-S fuzzy modelling,the dynamic model of the ball-and-beam system was formulated as a strict feedback form with modelling errors.An observer-based adaptive robust controller was developed via DSC tech-niques for the purpose of achieving high-performance for servo mechanisms with unmeasurable states[29].A robust adaptive DSC approach was presented for a class of strict-feedback single-input-single-output nonlinear systems,where radial-basis-function neural networks were used to approximate those unknown system functions[30],[31].In[32],a novel-function approximator was constructed by combining a fuzzy-logic system with a Fourier series expansion to approximate the un-known system function depending nonlinearly on the periodic disturbances.Based on this approximator,an adaptive DSC was proposed for strict-feedback and periodically time-varyingCHANG AND CHAN:ADAPTIVE DYNAMIC SURFACE CONTROL FOR UNCERTAIN NONLINEAR SYSTEMS295systems with unknown control-gain functions.In[33]and [34],an adaptive fuzzy backstepping dynamic surface control approach was developed for a class of multiple-input multiple-output nonlinear systems and uncertain stochastic nonlinear strict-feedback systems,respectively.In[35],an adaptive DSC was proposed for a class of stochastic nonlinear systems with the standard output-feedback form,where neural networks were used to approximate the unknown system functions. In[36],a neural-network-based decentralized adaptive DSC control design method was proposed for a class of large-scale stochastic nonlinear systems,where neural network method was used to approximate the unknown nonlinear functions. In this paper,a robust adaptive control method is presented for a class of uncertain nonlinear systems.The proposed con-trol scheme is based on adaptive DSC techniques combining with IT2FNN learning(IT2FNNADSC).To achieve a desired tracking goal,a stabilizing control action can be systematically derived.Also,the uncertainty modelling can be attained using IT2FNNs.The IT2FNN has the ability to accurately approxi-mate nonlinear systems or functions with uncertainties based on the merits of both the type-2fuzzy logic and the neural network.From the Lyapunov stability theorem,it is shown that the closed-loop system is stable,and all error signals are uniformly ultimately bounded.In addition,a ball-and-beam system is utilized for performance validations,including both simulation and experimental results.Moreover,the form of strict-feedback systems is a special case of addressed general nonlinear systems.Thus,the proposed IT2FNNADSC control method can be easily applied to many applications of interest. The organization of this paper is as follows.In Section II, the dynamic characteristics of uncertain nonlinear systems are discussed.The structure and design philosophy of IT2FNNs and the design procedures of the adaptive DSC were intro-duced in Section III.The stability analysis is investigated in Section IV.In Section V,a ball-and-beam system is applied for performance validations,where both simulation and ex-perimental results are included.The concluding remarks are given in Section VI.II.PreliminariesA.Problem FormulationConsider a class of nonlinear systems as follows.˙x1=f1(x)+g1(x1)x2˙x2=f2(x)+g2(x1,x2)x3...˙x i=f i(x)+g i(x1,x2,...,x i)x i+1...˙x n=f n(x)+g n(x)u(t)(1)where i=1,2,...,n−1,x=[x1x2···x n]T∈R n is the state vector,u(t)∈R is the control input.The f i,g i, i=1,2,...,n are smooth system and virtual control-gain functions,respectively.In this paper,the state vector x is assumed to be in a compact set in R n.Definition1:System(1)is uniformly ultimately bounded with ultimate bound b if there exist positive constants band Fig.2.Interval type-2membership function with an uncertain mean. c,independent of t0≥0,and for every a∈(0,c),there is T=T(a,b)≥0,independent of t0such that[37]x(t0) ≤a⇒ x(t) ≤b,∀t≥t0+T.(2) With the consideration of system uncertainties,the perturbed model of(1)can be represented in˙x1=f1(x)+g1(x1)x2+ 1(x,t)˙x2=f2(x)+g2(x1,x2)x3+ 2(x,t)...˙x i=f i(x)+g i(x1,x2,...,x i)x i+1+ i(x,t)...˙x n=f n(x)+g n(x)u(t)+ n(x,t)(3)where i=1,2,...,n−1.The i(x,t),i=1,2,...,n are bounded uncertainties,consisting of unknown modelling errors,parameter uncertainties,and/or external disturbances. Denote x1d(t)as a control goal of x1(t).The control objective is to develop an adaptive controller such that the corresponding closed-loop system of(3)is stable,all errors are uniformly ultimately bounded,and the magnitude of the tracking error x1(t)−x1d(t)can be arbitrary small as t→∞. Assumption1:There exist some constantsγi>0,ψi>0, i=1,2,...,n such thatψi≤ g i(x1,x2,...,x i) ≤γi.(4) Assumption2:The reference command x1d(t)is a continu-ous and bounded function of degree2such that x1d,˙x1d,and ¨x1d are well defined.Remark1:In particular casesf i(x)=f i(x1,x2,...,x i),i=1,2,...,n(5) the representation of(1)turns out to be a class of nonlinear systems with a general strict-feedback form.B.Interval Type-2Fuzzy Neural NetworksRecently,interval type-2fuzzy neural networks handling uncertainty problems have been popularly addressed.The design procedures of IT2FNNs will be introduced in this section.First,the IF-THEN rules for an IT2FNN can be expressed asR j:IF x(1)1is˜F1j AND···AND x(1)n is˜F njTHEN y(5)is[w(4)jL w(4)jR],j=1,2,...,m(6)296IEEE TRANSACTIONS ON CYBERNETICS,VOL.44,NO.2,FEBRUARY2014 where˜F ij is an interval type-2fuzzy set of the antecedentpart,[w(4)jL w(4)jR]is a weighting interval set of the consequentpart,and x(1)i is the input of IT2FNN,i=1,2,...,n.Thenetwork structure of IT2FNN is shown in Fig.1,in whichthe superscript is used to identify the layer number.Thefunctionality of each layer of the IT2FNN will be introducedin sequence.1)Input Layer:In this layer,x(1)1,...,x(1)n,denoted as thestate variables of(3),are the inputs of IT2FNN.2)Membership Layer:In this layer,each node performsits work as an interval type-2fuzzy membership function.Asshown in Fig.2,the associated Gaussian membership functionregarding the i th input and j th nodes is represented asμ(2)˜F ij (x(1)i)=exp⎛⎝−12x(1)i−m(2)ijσ(2)ij2⎞⎠≡N(m(2)ij,σ(2)ij,x(1)i)(7)where m(2)ij∈[m(2)ijL m(2)ijR]is an uncertain mean,andσ(2)ij is a variance,i=1,...,n,j=1,...,m.In Fig.2,the upper mem-bership function(UMF)and the lower membership function(LMF)are denoted as¯μ(2)˜F ij (x(1)i)andμ(2)˜F ij(x(1)i),respectively.The upper and lower bounds of each type-2Gaussian membership function can be respectively represented as¯μ(2)˜F ij (x(1)i)=⎧⎪⎨⎪⎩N(m(2)ijL,σ(2)ij,x(1)i),x(1)i<m(2)ijL1,m(2)ijL≤x(1)i≤m(2)ijRN(m(2)ijR,σ(2)ij,x(1)i),x(1)i>m(2)ijR,(8)μ(2)˜F ij (x(1)i)=⎧⎨⎩N(m(2)ijL,σ(2)ij,x(1)i),x(1)i>m(2)ijL+m(2)ijR2N(m(2)ijR,σ(2)ij,x(1)i),x(1)i≤m(2)ijL+m(2)ijR2.(9)Therefore,the outputs of membership layer can be describedas a collection of intervals[μ(2)˜F ij (x(1)i)¯μ(2)˜F ij(x(1)i)],i=1,...,n,j=1,...,m.3)Rule Layer:The operation of this layer is to multiply the input signals and output the result of product.For example, the output of the j th node in this layer is calculated as[f(3)j ¯f(3)j]=n i=1μ(2)˜F ij(x(1)i) n i=1¯μ(2)˜F ij(x(1)i).(10)4)Type-Reduction Layer:Type-reducer has been applied to reduce a type-2fuzzy set to a type-reduced set[6],[38]. This type-reduced set is then defuzzified to derive a crisp output.To simplify the computation complexity,singleton output fuzzy sets with the center-of-sets type reduction method are considered such that the output y(4)=[y(4)L y(4)R]is an interval type-1set.Then,the output of the type-reduction layercan be expressed asy(4)= mj=1f(3)j w(4)jmj=1f(3)j(11)where w(4)j=[w(4)jL w(4)jR]is consequent interval weighting andf(3)j=[f(3)j ¯f(3)j]is the interval degree offiring.Withoutloss of generality,w(4)jR and w(4)jL are assumed to be assigned in ascending order,i.e.,w(4)1L≤w(4)2L≤···≤w(4)mL and w(4)1R≤w(4)2R≤···≤w(4)mR.With reference to[39]and[40],the calculation of y(4)L and y(4)R can be derived asy(4)L=lj=1¯f(3)jw(4)jL+mj=l+1f(3)jw(4)jLj=1f j+j=l+1f(3)j,(12) y(4)R=rj=1f(3)jw(4)jR+mj=r+1¯f(3)jw(4)jRrj=1f(3)j+mj=r+1¯f(3)j.(13)Obviously,the numbers l and r in(12)and(13)are essential in the switching between the lowerfiring strength f(3)jand the upperfiring strength¯f(3)j.In general,the values of l and r can be obtained from a sorting process.In[9],a simplified type of reduction was proposed as follows:ˆy(4)L=mj=1f(3)jw(4)jLmj=1f(3)j≡W T L F(14)ˆy(4)R=mj=1¯f(3)jw(4)jRmj=1¯f(3)j≡W T R¯F(15)where W L and W R are the weighting vectors connected between the rule layer and type-reduction layer,W T L=w(4)1L w(4)2L···w(4)mL,W T R=w(4)1R w(4)2R···w(4)mR;F and¯F are the weightedfiring strength vectorF=f(3)1mj=1f(3)jf(3)2mj=1f(3)j···f(3)mmj=1f(3)jT,¯F=¯f(3)1mj=1¯f(3)j¯f(3)2mj=1¯f(3)j···¯f(3)mmj=1¯f(3)jT.It is noted that only the lower and upper extremefiring-strengths are used in(14)and(15),respectively,such that the computation burden can be reduced.5)Output Layer:The output y(5)is determined as the average ofˆy(4)L andˆy(4)Ry(5)=ˆy(4)L+ˆy(4)R2.(16) Remark2:The results obtained so far are mainly on the structure description of IT2FNNs.It is more interesting to investigate how to model the uncertain terms in(3)with IT2FNNs.Moreover,the closed-loop stability affected by the approximation errors will be addressed later on.III.Design of IT2FNNADSCIn this paper,the dynamic surface control method is utilized to design a stabilizing controller for a class of uncertain nonlinear systems of(3).With IT2FNNs,uncertainty terms of(3)can be approximately modeled asi(x,t)=12W T i F i+εi(17) where W T i=W T iL W T iRis an ideal constant weights vector, F i=F T i¯F T iTis thefiring strength vector,andεi is the approximation error,i=1,2,...,n.In(17),there exist some unknown bounded constants W M andεM such that W i ≤CHANG AND CHAN:ADAPTIVE DYNAMIC SURFACE CONTROL FOR UNCERTAIN NONLINEAR SYSTEMS297 W M and εi ≤εM,respectively.Since there is no a prioriknowledge to determine exact W i s,an adaptive mechanismis applied such that the estimatedˆW i can asymptoticallyconverge to W i.Let the estimation error be defined as follows:˜Wi(t)=ˆW i(t)−W i,i=1,2,...,n.(18)For the aforementioned uncertain nonlinear systems,an adap-tive dynamic surface control(ADSC)will be proposed topreserve the closed-loop stability.The design procedures re-garding the ADSC are discussed in the following.To stayconcise,the notations i,i=1,2,...,n,will be used torepresent the uncertainties of(3).Step3.1:Defines1=x1−x1d.(19)It is desired to track the reference command x1d with giveninitial conditions.From(3)and(17),the derivative of(19)canbe obtained as˙s1=f1+g1x2+12W T1F1+ε1−˙x1d.(20)Let¯x2be a stabilizing function to be determined for(3)¯x2=g−11(−f1−12ˆW T1F1−k1s1+˙x1d)(21)where k1is a positive constant.An adaptive law is designated as˙ˆW 1=121F1s1−η 1ˆW1(22)with a symmetric positive definite matrix 1andη>0.Let x2d be the regulated output of¯x2through a low-passfilter τ2˙x2d(t)+x2d(t)=¯x2,x2d(0)=¯x2(0).(23) With a properly chosenτ2,the smoothed x2d can be equiva-lently considered the required¯x2.Step3.2:Analogous to the discussion in Step3.1,an error variable is defined ass2=x2−x2d.(24) From(3)and(17),the derivative of(24)can be obtained as ˙s2=f2+g2x3+12W T2F2+ε2−˙x2d.(25) Let¯x3be a stabilizing function to be determined for(3)¯x3=g−12(−f2−12ˆW T2F2−k2s2+˙x2d)(26)where k2is a positive constant.An adaptation law is designed as˙ˆW 2=122F2s2−η 2ˆW2(27)with a symmetric positive definite matrix 2.Let x3d be the smoothed variable of¯x3through a low-passfilterτ3˙x3d(t)+x3d(t)=¯x3,x3d(0)=¯x3(0).(28) With a properly chosenτ3,the smoothed x3d can be equiva-lently considered the required¯x3.Step3.i:The state tracking error of x i is defined ass i=x i−x id.(29) From(3)and(17),the derivative of(29)can be obtained as˙s i=f i+g i x i+1+12W T i F i+εi−˙x id.(30) Let¯x i+1be a stabilizing function to be determined for(3)¯x i+1=g−1i(−f i−12ˆW T i F i−k i s i+˙x id)(31) where k i is a positive constant.Similarly,an adaptive law is given as˙ˆWi=12i F i s i−η iˆW i(32) with a positive definite matrix i= T i.Similarly,let x i+1,d be the smoothed counterpart of¯x i+1through a low-passfilter τi+1˙x i+1,d(t)+x i+1,d(t)=¯x i+1,x i+1,d(0)=¯x i+1(0).(33) With a properly chosenτi+1,d,the smoothed x i+1,d can be equivalently considered the required¯x i+1.Step3.n:The state tracking error of x n is defined ass n=x n−x nd.(34) From(3)and(17),the derivative of(34)can be obtained as˙s n=f n+g n u(t)+12W T n F n+εn−˙x nd.(35) Let u(t)be a stabilizing function to be determined for(3) u(t)=g−1n(−f n−12ˆW T n F n−k n s n+˙x nd)(36) where k n is a positive constant.Analogous to the aforemen-tioned discussion,an adaptive law is given as˙ˆWn=12n F n s n−η nˆW n(37) where n is a symmetric matrix, n>0.Remark3:So far,only the design procedures,combining DSC approach and IT2FNN,are identified step by step.The derivation of control actions and learning rules in each step will be investigated later,where the closed-loop stability will also be discussed in detail.IV.Stability AnalysisIn this section,the overall stability of a class of uncertain nonlinear systems will be investigated,where a DSC controller with an IT2FNN adaption is adopted.In this paper,let 0and n be feasible sets of IT2FNNADSC such that0={(x1d,˙x1d,¨x1d):x1d+˙x1d+¨x1d≤p0}(38)n=(s i,˜W i,h i):12ni=1s2i+˜W T i −1i˜W i+12ni=2h2i≤p(39)where p0and p are positive constants.It is noticed that 0 and n are compact sets in R3and R(2m+2)n−1.298IEEE TRANSACTIONS ON CYBERNETICS,VOL.44,NO.2,FEBRUARY 2014Theorem 1:Consider a class of uncertain nonlinear systems of (3).With the DSC controller and IT2FNN adaptive laws designed as (19),(21)–(24),(26)–(29),(31)–(34),(36)–(37),the corresponding s i and ˜Wi are uniformly ultimately bounded,i =1,2,...,n ,where (38)and (39)are satisfied.Proo f :First,the errors between the stabilizing variables and the smoothed variables are defined ash i +1=x i +1,d −¯x i +1=x i +1,d −g −1i (−f i −12ˆW Ti F i −k i s i +˙x id )(40)where i =1,2,...,n −1.From (30),(31),and (40),the derivative of s i can be derived as˙s i=g i s i +1+g i h i +1−12˜W Ti F i +εi −k i s i(41)in which i =1,2,...,n −1.From (35)and (36),the derivativeof the tracking error s n can be obtained as˙s n =−k n s n −12˜W Tn F n +εn .(42)From (33)and (40),the dynamics of the smoothed variablex id can be formulated as˙x id (t )=¯x i −x id τi =−h iτi,i =2,3,...,n.(43)Therefore,from (40)and (43),the dynamic changes of h i +1can be derived in the following:˙h i +1=˙x i +1,d −˙¯x i +1=−h i +1τi +1+B i +1,i =1,2,...,n −1(44)in whichB i +1(s 1,...,s n ,h 2,...,h n ,ˆW T 1,...,ˆW T i ,x 1d ,˙x 1d ,¨x 1d )=g −2i [(˙f i +12˙ˆW T i F i +12ˆW T i ˙F i +k i ˙s i+˙h i τi )g i −˙g i (f i +12ˆW TiF i −˙x id +k i s i )].Choose a Lyapunov function candidate asV =12 n i =1 s 2i +˜W T i −1i ˜W i +12 n i =2h 2i .(45)The derivative of (45)can be obtained as˙V = n i =1 s i ˙s i +˜W T i −1i ˙ˆW i + n i =2h i ˙h i .(46)From (41),(42),(44),and (46),it leads to ˙V≤s 1(g 1s 2+g 1h 2−12˜W T1F 1+ε1−k 1s 1)+s 2(g 2s 3+g 2h 3−12˜W T2F 2+ε2−k 2s 2)+···+s i (g i s i +1−12˜W Ti F i +g i h i +1+εi −k i s i )+···+s n (−k n s n −12˜W Tn F n +εn )+ n i =1˜W T i −1i ˙ˆW i + n i =2 −h 2i τi + h i B i.(47)It is true thats i s i +1≤s 2i +s 2i +1,s i h i +1≤s 2i +h 2i +1,i =1,2,...,n −1(48)ands i εi ≤s 2i +ε2i ,i =1,2,...,n.(49)Therefore,the following inequality can be obtained by substi-tuting (4),(22),(27),(32),(37),and (48),(49)into (47)˙V ≤ n −1i =1(2γi s 2i +γi s 2i +1+γi h 2i +1)+ n i =1(−k i s 2i +ε2i +s 2i )+ n i =1(−η˜W T i ˆW i )+ n i =2 −h 2i τi+ h i B i .(50)Let α0>0be a constant such that k 1=2γ1+1+α0,k n =γn −1+1+α0,and k i =2γi +γi −1+1+α0,i =2,3,...,n −1,are satisfied.With reference to [30],using ˜Wi 2− W i 2≤2˜WT i ˆW i ,it gives ˙V ≤ ni =1(−α0s 2i +ε2i )+ n −1i =1(γi h 2i +1)+ n i =2 −h 2iτi + h i B i− n i =112η˜W i 2− W i 2 ≤ n i =1 −α0s 2i −η2λmax ( −1i )˜W T i −1i ˜W i+ n −1i =1(γi h 2i +1)+ n i =2 −h 2i τi + h i B i+ n i =1 ε2i +η2 W i 2.(51)Denoted ε2i +η2 W i 2=c i ,it leads to c i ≤ε2M +η2W 2M =c M ,i =1,2,...,n .From the positive definiteness of i ,a positive ηcan be determined such that η2λmax ( −1i)≥α0.From (51),itresults in˙V ≤ n i =1 −α0 s 2i +˜W T i −1i ˜W i +nc M + n −1i =1(γi h 2i +1)+ n i =2 −h 2iτi+ h i B i .(52)Therefore,from the assumptions of boundedness,smoothness,and compactness,B i is bounded on 0× n ,i.e.,there exists an M i >0such that B i ≤M i .Let1τi =γi −1+M 2i 2β+α0,i =2,3,...,n (53)where βis a positive number.Substituting (53)into (52),the following inequality can be obtained:˙V ≤ n i =1 −α0 s 2i +˜W T i −1i ˜W i +nc M + n i =2 − M 2i2β+α0 h 2i+ h i B i .(54)Notice that h i B i ≤h 2i B 2i 2β+ β2,i =2,3,...,n .Then,one can have˙V ≤ n i =1 −α0 s 2i +˜W T i −1i ˜W i +nc M +(n −1)β2+ n i =2 −α0h 2i −(M 2i −B 2i )h 2i 2β ≤ n i =1−α0 s 2i +˜W T i −1i ˜W i − n i =2(α0h 2i )+nc M +(n −1)β2=−2α0V +nc M +(n −1)β2.(55)Solving (55)leads toV (t )≤12α0 nc M +(n −1)β2+ V (0)−12α0nc M +(n −1)β2e (−2α0t ),∀t >0.(56)CHANG AND CHAN:ADAPTIVE DYNAMIC SURFACE CONTROL FOR UNCERTAIN NONLINEAR SYSTEMS299Fig.3.Experiment setup of the ball-and-beam system.From (56),it can be observed that V (t )is uniformly ultimately bounded,and the proof is completed.ٗRemark 4:By increasing the value of α0,the quantity of12α0(nc M +(n −1)β2)can be made arbitrarily small.Also,the convergence of V (t )implies that s i ,˜Wi ,and h i are uniformly ultimately bounded.Thus a large enough α0can make s 1=x 1−x 1d arbitrarily small.Equivalently,the stability of the proposed IT2FNNADSC control system can be guaranteed,and the purpose of tracking can be achieved in the boundness of steady-state errors.V .Example:Ball-and-Beam SystemTo verify the feasibility of the proposed IT2FNNADSC,anend-point driven ball-and-beam system is considered,where the scheme diagram is shown in Fig.3,and the associated dynamic equations are described as follows [41]:˙x 1=x 2+ 1(x ,t )˙x 2=Ax 1x 24−Agx 3+ 2(x ,t )˙x 3=x 4+ 3(x ,t )˙x 4=B cos x 3[C cos l b x 3d u −Dx 4cos l b x 3d−E −Hx 1]−BGx 1x 2x 4+ 4(x ,t )(57)where x (t )=[x 1(t )x 2(t )x 3(t )x 4(t )]T is the state vector,x 1is the ball position (m),x 2is the ball velocity (m/sec),x 3is the beam angle (rad),x 4is the angular velocity of the beam(rad/sec),A = 1+m −1B J B R −2 −1,B = J B +J b +m B x 21 −1,C =n g K b l b (R a d )−1,D = n g K b l b 2 R a d 2 −1,E =0.5l b m b g ,H =m B g ,G =2m B ,and u (t )is the input voltage of a DC motor.The physical parameters of the ball-and-beam system are listed in Table I.Remark 5:The representation of (57)can be fitted into the general form of (3).In consequence,the proposed IT2FNNADSC will be applied for the position control of a ball-and-beam system,where the ball is desired to be asymptotically balanced at a designated position.TABLE IParameters of the Ball-and-Beam System Symbol DefinitionValue m B Mass of the ball 0.0217kg m b Mass of the beam 0.334kg R Radius of the ball 0.00873m l b Beam length0.4m d Radius of the large gear 0.04mJ B Ball inertia 6.803×10−7kgm 2J b Beam inertia0.017813kgm 2K b Back-EMF constant 0.1491Nm/A R a Armature resistance 18.91 g Acceleration of gravity 9.8m /s 2n gGear ratio4.2TABLE IIParameters of ADSCi 1234k i 2840.887434.943727τi N/A0.0250.0250.025 i 100I 32α025η0.5A.Simulation ResultsFrom (21),(26),(31),and (57),stabilizing functions can be obtained as follows:¯x 2=−12ˆW T1F 1−k 1s 1+˙x 1d(58)¯x 3=−1Ag −12ˆW T 2F 2−k 2s 2−Ax 1x 24+˙x 2d (59)and¯x 4=−12ˆWT3F 3−k 3s 3+˙x 3d (60)where the employing neural networks are adaptively obtained from (32).From (36)and (57),the IT2FNNADSC based control action can be drived asu (t )=1BC cos x 3cos l b x 3d·[B cos x 3(Dx 4cos l b x 3d +E +Hx 1)+BGx 1x 2x 4+˙x4d −k 4s 4−12ˆW T4F 4].(61)The parameters for the conventional DSC and the IT2FNNADSC are initially given in Tables II and III,in which F 1=F 2=F 3=F 4=⎡⎣f (3)1 16j =1f (3)j···f (3)16 16j =1f (3)j¯f (3)1 16j =1¯f (3)j ···¯f (3)16 16j =1¯f(3)j ⎤⎦T ,[f (3)j¯f (3)j ]=[ 4i =1μ(2)˜F ij 4i =1¯μ(2)˜F ij ],j =1,2,...,16,and ˆW 1(0)=ˆW 2(0)=ˆW 3(0)=ˆW 4(0)=[ˆw(4)1L (0)···ˆw (4)16L (0)ˆw (4)1R (0)···ˆw (4)16R (0)]T .The rule table of an IT2FNN is shown in Table IV,where PS (positive small),PB (positive big),Neg (negative),Pos (positive),and Zero are interval type-2fuzzy sets.The input and output membership functions for the corresponding interval type-2fuzzy sets are shown in Fig.4.。
模糊推理
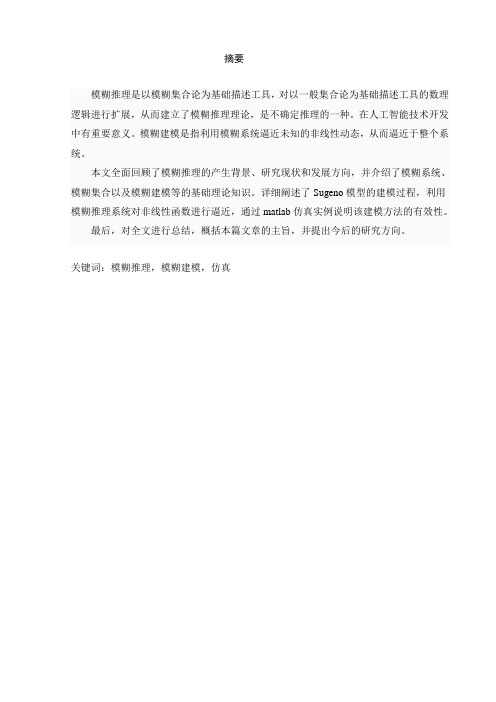
摘要模糊推理是以模糊集合论为基础描述工具,对以一般集合论为基础描述工具的数理逻辑进行扩展,从而建立了模糊推理理论,是不确定推理的一种。
在人工智能技术开发中有重要意义。
模糊建模是指利用模糊系统逼近未知的非线性动态,从而逼近于整个系统。
本文全面回顾了模糊推理的产生背景、研究现状和发展方向,并介绍了模糊系统、模糊集合以及模糊建模等的基础理论知识。
详细阐述了Sugeno模型的建模过程,利用模糊推理系统对非线性函数进行逼近,通过matlab仿真实例说明该建模方法的有效性。
最后,对全文进行总结,概括本篇文章的主旨,并提出今后的研究方向。
关键词:模糊推理,模糊建模,仿真AbstractFuzzy reasoning based on fuzzy sets theory to describe tool,it is based on general set theory of mathematical logic described tools, so as to establish the extended fuzzy reasoning theory,it is an uncertainty reasoning. It is important to the development of artificial intelligence technology. Fuzzy model is refered to the use of fuzzy system to approach unknown nonlinear dynamic,then approach the whole system.This paper reviews the background of fuzzy reasoning,research status and development direction,and introduces fuzzy system,the fuzzy set and the fuzzy model and basic theoretical knowledge. It also expounds the Sugeno modeling process,and use fuzzy inference system to approximate nonlinear function. Through matlab simulation example shows the effectiveness of the modeling methodFinally,the full text is summarized to express the purpose of this article, and puts forward the direction of future research.Keywords: fuzzy reasoning,fuzzy modeling,simulation目录1.绪论 (1)1.1 模糊思想的起源 (1)1.1.1 精确思维的缺陷 (1)1.1.2 逻辑推理与模糊性 (1)1.2 模糊推理理论研究的进展 (3)1.3 模糊推理的研究领域和成果 (4)1.3.1 模糊推理在模糊控制中的研究与应用 (4)1.3.2 模糊推理在人工智能中的研究与应用 (4)2.模糊系统基础 (6)2.1 模糊集 (6)2.2 模糊集的表示一隶属度函数 (6)2.3 If...then规则 (7)2.4 模糊推理 (8)2.5 模糊聚类 (10)3.模糊建模 (11)3.1 模糊模型建模过程 (11)3.2 非线性系统的T-S模糊模型 (12)3.3 T-S模型的参数辨识 (13)4.仿真实例 (16)4.1 仿真软件简介 (16)4.2 设计原理 (16)4.3 仿真实例 (18)4.4 结论 (22)结束语 (24)参考文献 (25)致谢 (26)1.绪论1.1 模糊思想的起源1.1.1 精确思维的缺陷迄今,经典逻辑和精确数学的成功推动了精确科学的迅速发展; 精确科学的巨大成就也造成了人类对“精确”的顶礼膜拜。
Fuzzy Logic and Systems
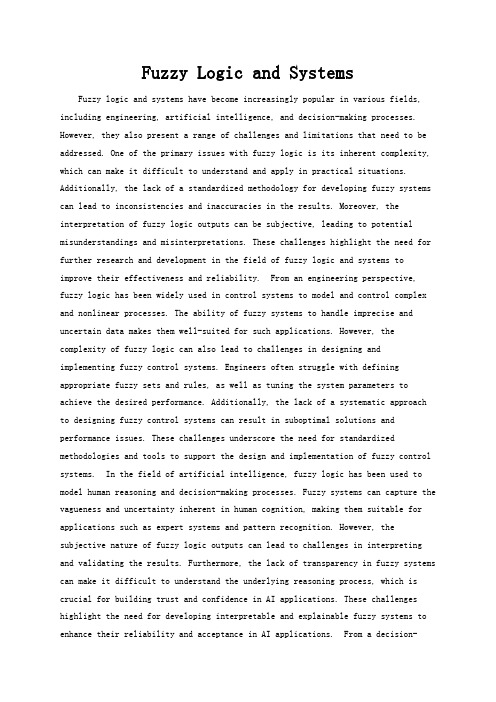
Fuzzy Logic and SystemsFuzzy logic and systems have become increasingly popular in various fields, including engineering, artificial intelligence, and decision-making processes. However, they also present a range of challenges and limitations that need to be addressed. One of the primary issues with fuzzy logic is its inherent complexity, which can make it difficult to understand and apply in practical situations. Additionally, the lack of a standardized methodology for developing fuzzy systems can lead to inconsistencies and inaccuracies in the results. Moreover, the interpretation of fuzzy logic outputs can be subjective, leading to potential misunderstandings and misinterpretations. These challenges highlight the need for further research and development in the field of fuzzy logic and systems to improve their effectiveness and reliability. From an engineering perspective, fuzzy logic has been widely used in control systems to model and control complex and nonlinear processes. The ability of fuzzy systems to handle imprecise and uncertain data makes them well-suited for such applications. However, the complexity of fuzzy logic can also lead to challenges in designing and implementing fuzzy control systems. Engineers often struggle with defining appropriate fuzzy sets and rules, as well as tuning the system parameters to achieve the desired performance. Additionally, the lack of a systematic approach to designing fuzzy control systems can result in suboptimal solutions and performance issues. These challenges underscore the need for standardized methodologies and tools to support the design and implementation of fuzzy control systems. In the field of artificial intelligence, fuzzy logic has been used to model human reasoning and decision-making processes. Fuzzy systems can capture the vagueness and uncertainty inherent in human cognition, making them suitable for applications such as expert systems and pattern recognition. However, the subjective nature of fuzzy logic outputs can lead to challenges in interpreting and validating the results. Furthermore, the lack of transparency in fuzzy systems can make it difficult to understand the underlying reasoning process, which is crucial for building trust and confidence in AI applications. These challenges highlight the need for developing interpretable and explainable fuzzy systems to enhance their reliability and acceptance in AI applications. From a decision-making perspective, fuzzy logic has been applied in various fields, including finance, marketing, and risk management. Fuzzy systems can handle imprecise and ambiguous information, making them valuable for making complex decisions under uncertainty. However, the lack of a standardized framework for developing fuzzy decision support systems can lead to inconsistencies and biases in the decision-making process. Additionally, the subjective nature of fuzzy logic outputs canlead to potential misunderstandings and misinterpretations, which can have significant implications for decision outcomes. These challenges emphasize the need for developing robust and transparent fuzzy decision support systems to improve their effectiveness and reliability in real-world applications. In conclusion, while fuzzy logic and systems offer valuable capabilities for handling imprecise and uncertain information, they also present a range of challenges and limitations that need to be addressed. From engineering, artificial intelligence, and decision-making perspectives, the complexity, lack of standardization, and subjective nature of fuzzy logic outputs can lead to inconsistencies, inaccuracies, and misunderstandings. To overcome these challenges, further research and development are needed to improve the effectiveness, reliability, and transparency of fuzzy logic and systems. By addressing these challenges, we can unlock the full potential of fuzzy logic and systems in various applications and enhance their value in addressing real-world problems.。
永磁同步电动机英文翻译

英文原文Research on Voltage Space-vector Control System of Synchronous Motor Vector control of field oriented control, the basic idea is: through coordinate transformation control method for simulation of DC motor to control the permanent magnet synchronous motor. Three-phase symmetrical windings in three-phase AC can produce a rotating magnetic motive force, two phase symmetrical windings into two symmetric alternating current can produce the same rotating magnet ometive force; therefore the three-phase symmetric winding can be replaced with two phase symmetrical windings equivalent independent of each other, equivalent principle is the constant magnetomotive force produced before and after transformation, transformation and total power constant.In oil field, the power factor was reduced and the reactive power consumption was increased because of the usage of the large number of asynchronous motor, and resulting in a huge waste of energy, which reduced the integrated cost-effective of field. The permanent magnet synchronous motor possess all the advantages of synchronous motor and it has high efficiency and higher power factor. For the advantages of permanent magnet synchronous,it will bring good energy saving results if it is used in pumping unit. As a result,the study on permanent magnet synchronous motor control system is important.In this paper the theory of vector control system on PMSM is first deeply studied,and the idea of coordinate transformation is used to build the mathematical model of PMSM. An in-depth theoretical analysis of voltage space vector control algorithm is done. Secondly,based on the mathematical model of permanent magnet synchronous motor and SVPWM theory,the model of PMSM vector control system is established by of Matlab/Simulink. The simulation result shows the possibility of using the control system.In the paper, the software and hardware of PMSM vector control system is designed core-based TI Company’s motor control DSP chip TMS320LF2407A. Hardware ncludes the main circuit,control circuit and its peripheral circuits;software contains the main program and SVPWM interrupt subroutine,it achieves the implementation of the dual closed-loop current. At last,the motor experiments are carried on under the laboratory,the experimental results verify the correctness of the hardware and control program.Permanent magnet synchronous motor with the advantages of simple structure,high efficiency,wide speed range,widely used in machining,aerospace and electric traction fields,this paper introduces the structure,control strategy of permanent magnet synchronous motor and its vector torque control research present situation and direction.Based on space vector principle,the three kind of coordinate systems as well as the transformation of them which usually used in motor’s speed control system areintroduced,then,the mathematic models on different coordinate systems are derived,be based on that,the principle of traditional direct torque control system as well as the direct torque control system based on SVPWM are analyzed detailed,meanwhile,the realization process of SVPWM algorithm is derived.Finally,the simulation model of convientional DTC Control system are established in MATLAB/Simulink.Control of permanent magnet synchronous motor mainly in the following1.1 vector controlThe core idea of vector control of three-phase current,voltage,the flux of the motor by coordinate transformation into the rotor flux oriented phase reference coordinate system, control idea according to DC motor, control motor torque.The advantages of the field oriented vector control is good torque response,precise speed control,zero speed can achieve full load.However,the vector control system needs to determine the rotor flux,to coordinate transformation,a large amount of calculation,but also consider the effect of changes in the rotor of the motor parameters,which makes the system more complex,this is the vector control deficiencies.1.2 direct torque controlIt is based on stator flux orientation,implementation of direct control of stator flux and torque.The control is based on the idea of amplitude real-time detection of motor torque and flux are given,and the torque and flux linkage value comparison,the torque and flux adjusting the appropriate stator voltage space vector selection table switch calculated directly from an offline,power switch and control of inverter state.Direct torque control does not need the vector coordinate transformation complex,the motor model is simplified,no pulse width modulation signal generator,control has the advantages of simple structure,motor parameter changes,can obtain good dynamic performance.But there are also some shortcomings,such as the inverter switching frequency is not fixed,large torque ripple current to realize digital control requires high sampling frequency.1.3 direct torque control based on space vector modulation(SVM-DTC)The SVM-DTC control is the vector control and direct torque control together,its theory foundation and DTC control theory,is based on torque angle control.According to the change of torque angle and flux vector position,get the flux of the next cycle position,which can be the reference voltage vector is required,then the reference voltage vector modulation,PWM wave inverter driving.The SVM-DTC control,the flux changes to determine the next position,so the accurate estimation of flux has great effect on the control system,and the flux estimation depends on motor parameters are stable.In addition,the electromagnetic torque and torque angle is a nonlinear relationship,but in the practical application is approximately linear,using PIregulation,performance so that the PI parameters can also affect the system.The model reference adaptive control(MRAS)The model system requirements of the control system with a model for the adaptive control,the output response model is ideal,this model is called the reference model.The system always tries to make dynamic consistency can bedynamic reference model and the adjustable model in operation.By comparing the output of reference model and actual process,and through the adaptive controller to adjust some parameters of the adjustable model or generate anauxiliary input,so that the output error between actual output and the reference model as small as possible.In practical application,usually used for speed estimation,to realize the speed sensor less operation.Therefore,the model reference adaptive depends mainly on the accuracy of the adjustable model,the stable operation of the system plays a decisive role in.In addition,the adaptive control law parameters tuning is a difficult problem,the control accuracy of the control system has a great impact.1.5The state observer based controlControl based on state observer is developed based on the modern control theory,observer based on the mathematical model of permanent magnet synchronous motor,used for each observation control system and the state,thus extracting speed control.It is also dependent on the accuracy of the motor model,the appearance of large error will run at low speed or increasing temperature leads to the variation of motor parameters,so as to bring large deviation to control.intelligent controlThe use of intelligent algorithms,intelligent control of the control system, such as fuzzy control,neural network control,self-tuning parameters and so on,through one or several times after the trial operation, automatic parameter tuning out,to realize the optimization control.Intelligent control has many advantages,especially in the motor is multi variable,nonlinear control system,however,control and its performance depends on the control object,that is to say not every control system can achieve good control,which require sexperience.At the same time,the large amount of computation,but also has certain requirements for the controller.Synchronous Motor because of having power factor higher run – time efficiency higher , stability good, the revolving speed settles to wait a merit, is extensively been applied to industrial production amid. The starting fault that acquaints with synchronous motor, and debugging in time, all have important meaning to the motor and the production systems . By way of energy in time, accurate debugging and transaction fault, have the familiar faultprogress of the synchronous motor in detail analytical!2 Familiar fault2.1 The synchro motor after switching on electricity the incapability startsThe synchro motor after starting the incapability run - time generally has the reason of severals as follows:(1)Power supply voltage over low.Because at the square of voltage, the starting torque direct proportion of synchro motor's the voltage of power supply over make low the starting torque of synchro motor significantly the droop is lower than load troque, can not start thus and want to raise vs this power supply voltage to enlarge the starting torque of dynamo.(2)The fault of motor. Check motor settle, the rotor winding had no short circuit, open circtui, open soldering and link bad etc. fault, these the faults will make the dynamo can not start to create starting of rating of intensity of magnetic field, make thus the dynamo can not start;Checking the motor bearing has already had no failure, the port cap has have no loose, if bearing failure port shroud loose, result in bearing's down sinking, mutually rub with stator iron core, result in thus dynamo's canning not start, vs settle the rotor fault can be shaken table with the low tension, gradually click to check to seek a fault condition and adopt homologous treatment;The countersgaft accepts and carries to shroud a loose condition and all wants a pan car before driving each time and sees motor rotor whether slewing is vivid, if bearing or shaft kiowatt damage and replace in time.(3)The control device breaks down.This kind of faults are mostly the d.c. output voltage of the windings of Li magnetic belt to adjust not appropriate or don't output, result in the stator current of motor over big, cause the motor conduct electricity the run make or the losing of dynamo magnetic belt run - time.Should check whether output voltage current and its waveform that the Li magnetic belt equips is normal at this time, the Rong breaks whether the machine Rong breaks, the contact is bad;Whether circuit board plug-in puts prison or alignment;Check loop resistance, put out whether crystal gate tube of magnet burns out or brokes through.(4)Mechanical trouble. Such as be dragged along a dynamic machinery to block, result in motor incapability's starting, the rotor that moves motor in response to the pan at this time sees whether the slewing is vivid, machinery burden whether existence fault2.2The synchro motor incapability leads long into synchronization.Synchro motor in common use law of nonsynchronous starting,throw in Li magnetic belt when the motor rotor revolving speed hits synchronous revolving speed of 95%, make it leads long into synchronization. The synchro motor incapability leads long into synchronous reason as follows:(1)The Li magnetic belt winding short circuit.Because the winding of Li magnetic belt, existence short circuit breaks down, as a result makes motor able to stabilize run - time but incapability and lead long into synchronization while being lower than synchronous revolving speed. Check to seek the Li magnetic belt winding short circuit, can open into low - tension(about the 30 Vs) in the rotor derivation on - line, put on the magnetic poles surface with a hand work steel saw, pursue inspection magnetic poles, if vibrating is violent, explain the magnetic poles to have no short circuit on steel saw of the magnetic poles' surface, if the vibrating of saw blade micro or don't flap, explain the magnetic poles short circuit. After unloading the magnetic poles, check the fault to click,is short-circuit degree, adopt local to mend or re- round to make.(2) Power supply voltage over low. Power supply voltage over low, result in the strong Li link of the device of Li magnetic belt incapability working, make the motor incapability lead long into synchronization thus, the concrete way is to raise power supply voltage appropriately.(3) The fault of Li magnetic belt device. Such as throw Li over speedy(namely throw in Li magnetic belt, motor rotor revolving speed over low), will make the motor can not lead long into synchronization, should check to throw if the Li link exists fault at this time. If Li magnetic belt device fault, the output's current is lower than a rating value, cause the electricity magnetic troque of dynamo over small but can not lead long into synchronization, at this time in response to scrutiny Li magnetic belt device of throw Li link and phase - shifting link, waveform use oscillo graph to check to throw Li link and phase - shifting link, should also check and put out magnetic belt link and put out crystal gate of magnetic belt whether tube discovers a question as usual, handle in time, if the incapability handles in time, by way of the energy quickly restore capacity, should replace to provide for use circuit board.2.3 Brush and compress tightly spring and gather to give or get an electric shock ring fault.The brush leads short and compresses tightly spring press scarcity and make brush and gather to give or get an electric shock ring of indirectly touch badly, thus generate spark or arc electric, arc electric or spark to on the other hand and easily spark short circuit, will make arc electric burn on the other hand shorter, spark open circtui thus, result in Li magnetic belt device only the Li magnetoelectricity press but have no Li magnetoelectricity streaming;Compress tightly spring ageing lapse, make brush and gather to give or get an electric shock ring of indirectly touch badly, effect the starting of motor thus;Gather to give or get an electric shock a ring surface to there is grease stain and scar or slot scar, will make brush and gather to give or get an electric shock ring of indirectly touch badly, generate spark, spark further burn gather to give or get an electric shock ring, will also make gnd short-circuit, the spark effects the starting of motor thus.For gather to give or get an electric shock ring superficial grease stain, can wipeto clean with the acetone; For thin trace, use many fettle shagging rings of sandpapers surface, is ring surface roughness to hit R1.6 ums, if the slot scar obviously needs to get on the car bed transform, truning, enter amount of knife to take every time 1 mm as proper, in the 1-1.5 ms/s, the truning speed control's roughness hits of the ums of R1.5-1.8 and becomes bad anti to finally polish with the sandpaper 2-3 times over the 0.05 mms.2.4 The damper winding breaks down.The damper winding of synchro motor rotor is provided for synchro motor starting to use and wipe - out run - time at the same time amid spark because of loading to change of out of step osc.Start the damper winding in the process to incise the magnetic field of stator revolution but induced very big starting current in the synchro motor, so the big current by all means will result in damping hair thermal expansion, under the normal condition because of starting time short, the damper winding starting is behind soon will cool off, but block up revolution in the motor, lack phase, start the super - in time to length ways wait a condition down, if don't shut down in time, will result in the damping take off soldering to split etc. condition.The damper winding is weaker link in the synchro motor parts, the damper winding familiar fault has:The damping takes off soldering and split, the damping ring discharges wildfire, damping ring the strain is serious.These faults will effect the starting of synchro motor. The damping takes off soldering and chooses silver actinium welding rod and adopts oxyacetylene welding to weld, the dynamo after taking out the core heats into rotor 200 Celsius degrees set rotor vertical in the oven, after taking out and adopt 750 Celsius degrees to or soly weld temperature, damping and the blind side of of damping ring complete solderings are full, clear a soldering dirt again, ;For split of the damping , after dismantling original damping, choose the material of material homology and adopt the above-mentioned method to weld after packing good damping.Damping ring the wildfire is mainly what damping ring indirectly touches bad or get in touch with area isn't enough to result in. Damping ring the strain seriously is mainly a damping to fix anticoincidence in the slot, the damping plugs into damping ring while welding hole falsely, appear additional stress after welding, at plus damping ring intensity not enough to, treatment is loose open all connectivity bolts of damping rings, vs strain anti big of damping ring, after oxyacetylene welding heating adjust with the exclusive use fixture even, vs strain serious replace a new damping of ring.3 ConclusionWhen the synchro motor appears fault, cautiously analytical possible reason, gradually expel, look into related data when it's necessary, absorb experience, propose corrective actions.Analytical the dynamo fault not only need to have firm theory knowledge and experience of prolific maintenance repairs, but also need to aim at concrete fault, deepconsideration, brave creative, the dynamo after ensuring to break down removal can stabilize run - time over a long period of time.中文翻译永磁同步电动机矢量控制系统〔中文对照〕矢量控制亦称磁场定向控制,其基本思路是:通过坐标变换实现模拟直流电机的控制方法来对永磁同步电机进行控制。
自动化专业英语
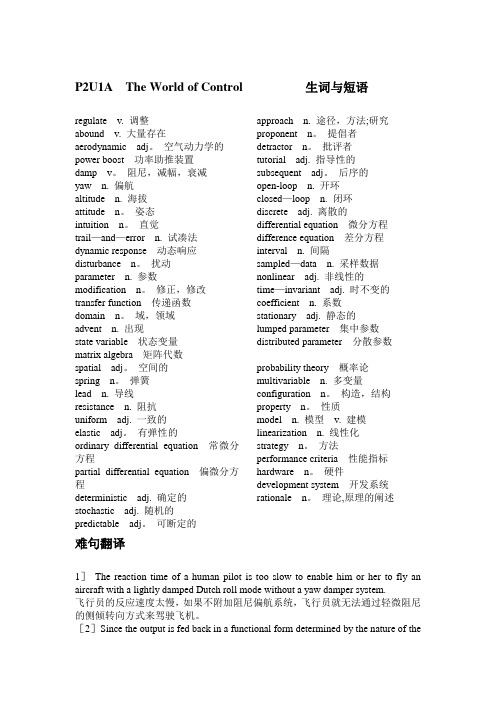
P2U1A The World of Control 生词与短语regulate v. 调整abound v. 大量存在aerodynamic adj。
空气动力学的power boost 功率助推装置damp v。
阻尼,减幅,衰减yaw n. 偏航altitude n. 海拔attitude n。
姿态intuition n。
直觉trail—and—error n. 试凑法dynamic response 动态响应disturbance n。
扰动parameter n. 参数modification n。
修正,修改transfer function 传递函数domain n。
域,领域advent n. 出现state variable 状态变量matrix algebra 矩阵代数approach n. 途径,方法;研究proponent n。
提倡者detractor n。
批评者tutorial adj. 指导性的subsequent adj。
后序的open-loop n. 开环closed—loop n. 闭环discrete adj. 离散的differential equation 微分方程difference equation 差分方程interval n. 间隔sampled—data n. 采样数据nonlinear adj. 非线性的time—invariant adj. 时不变的coefficient n. 系数stationary adj. 静态的lumped parameter 集中参数distributed parameter 分散参数spatial adj。
空间的spring n。
弹簧lead n. 导线resistance n. 阻抗uniform adj. 一致的elastic adj。
有弹性的ordinary differential equation 常微分方程partial differential equation 偏微分方程deterministic adj. 确定的stochastic adj. 随机的predictable adj。
数学英文论文
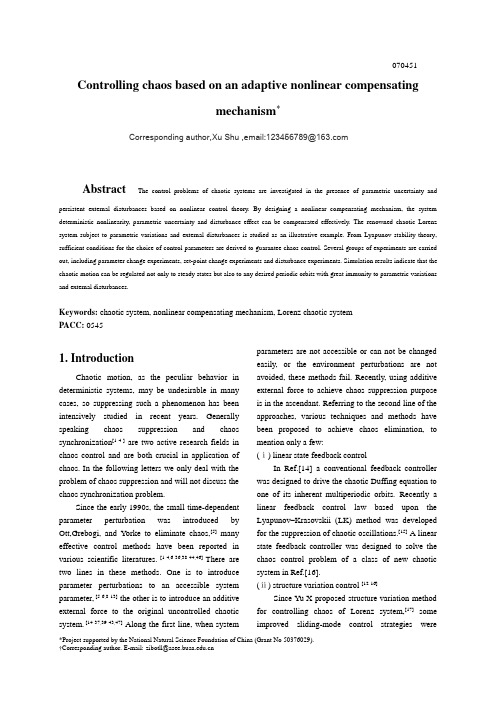
070451 Controlling chaos based on an adaptive nonlinear compensatingmechanism*Corresponding author,Xu Shu ,email:123456789@Abstract The control problems of chaotic systems are investigated in the presence of parametric u ncertainty and persistent external distu rbances based on nonlinear control theory. B y designing a nonlinear compensating mechanism, the system deterministic nonlinearity, parametric uncertainty and disturbance effect can be compensated effectively. The renowned chaotic Lorenz system subject to parametric variations and external disturbances is studied as an illustrative example. From Lyapu nov stability theory, sufficient conditions for the choice of control parameters are derived to guarantee chaos control. Several groups of experiments are carried out, including parameter change experiments, set-point change experiments and disturbance experiments. Simulation results indicate that the chaotic motion can be regulated not only to stead y states but also to any desired periodic orbits with great immunity to parametric variations and external distu rbances.Keywords: chaotic system, nonlinear compensating mechanism, Lorenz chaotic systemPACC: 05451. IntroductionChaotic motion, as the peculiar behavior in deterministic systems, may be undesirable in many cases, so suppressing such a phenomenon has been intensively studied in recent years. Generally speaking chaos suppression and chaos synchronization[1-4 ]are two active research fields in chaos control and are both crucial in application of chaos. In the following letters we only deal with the problem of chaos suppression and will not discuss the chaos synchronization problem.Since the early 1990s, the small time-dependent parameter perturbation was introduced by Ott,Grebogi, and Y orke to eliminate chaos,[5]many effective control methods have been reported in various scientific literatures.[1-4,6-36,38-44,46] There are two lines in these methods. One is to introduce parameter perturbations to an accessible system parameter, [5-6,8-13] the other is to introduce an additive external force to the original uncontrolled chaotic system. [14-37,39-43,47] Along the first line, when system parameters are not accessible or can not be changed easily, or the environment perturbations are not avoided, these methods fail. Recently, using additive external force to achieve chaos suppression purpose is in the ascendant. Referring to the second line of the approaches, various techniques and methods have been proposed to achieve chaos elimination, to mention only a few:(ⅰ) linear state feedback controlIn Ref.[14] a conventional feedback controller was designed to drive the chaotic Duffing equation to one of its inherent multiperiodic orbits.Recently a linear feedback control law based upon the Lyapunov–Krasovskii (LK) method was developed for the suppression of chaotic oscillations.[15]A linear state feedback controller was designed to solve the chaos control problem of a class of new chaotic system in Ref.[16].(ⅱ) structure variation control [12-16]Since Y u X proposed structure variation method for controlling chaos of Lorenz system,[17]some improved sliding-mode control strategies were*Project supported by the National Natural Science Foundation of C hina (Grant No 50376029). †Corresponding au thor. E-mail:zibotll@introduced in chaos control. In Ref.[18] the author used a newly developed sliding mode controller with a time-varying manifold dynamic to compensate the external excitation in chaotic systems. In Ref.[19] the design schemes of integration fuzzy sliding-mode control were addressed, in which the reaching law was proposed by a set of linguistic rules. A radial basis function sliding mode controller was introduced in Ref.[20] for chaos control.(ⅲ) nonlinear geometric controlNonlinear geometric control theory was introduced for chaos control in Ref.[22], in which a Lorenz system model slightly different from the original Lorenz system was studied considering only the Prandtl number variation and process noise. In Ref.[23] the state space exact linearization method was also used to stabilize the equilibrium of the Lorenz system with a controllable Rayleigh number. (ⅳ)intelligence control[24-27 ]An intelligent control method based on RBF neural network was proposed for chaos control in Ref.[24]. Liu H, Liu D and Ren H P suggested in Ref.[25] to use Least-Square Support V ector Machines to drive the chaotic system to desirable points. A switching static output-feedback fuzzy-model-based controller was studied in Ref.[27], which was capable of handling chaos.Other methods are also attentively studied such as entrainment and migration control, impulsive control method, optimal control method, stochastic control method, robust control method, adaptive control method, backstepping design method and so on. A detailed survey of recent publications on control of chaos can be referenced in Refs.[28-34] and the references therein.Among most of the existing control strategies, it is considered essentially to know the model parameters for the derivation of a controller and the control goal is often to stabilize the embedded unstable period orbits of chaotic systems or to control the system to its equilibrium points. In case of controlling the system to its equilibrium point, one general approach is to linearize the system in the given equilibrium point, then design a controller with local stability, which limits the use of the control scheme. Based on Machine Learning methods, such as neural network method[24]or support vector machine method,[25]the control performance often depends largely on the training samples, and sometimes better generalization capability can not be guaranteed.Chaos, as the special phenomenon of deterministic nonlinear system, nonlinearity is the essence. So if a nonlinear real-time compensator can eliminate the effect of the system nonlinearities, chaotic motion is expected to be suppressed. Consequently the chaotic system can be controlled to a desired state. Under the guidance of nonlinear control theory, the objective of this paper is to design a control system to drive the chaotic systems not only to steady states but also to periodic trajectories. In the next section the controller architecture is introduced. In section 3, a Lorenz system considering parametric uncertainties and external disturbances is studied as an illustrative example. Two control schemes are designed for the studied chaotic system. By constructing appropriate L yapunov functions, after rigorous analysis from L yapunov stability theory sufficient conditions for the choice of control parameters are deduced for each scheme. Then in section 4 we present the numerical simulation results to illustrate the effectiveness of the design techniques. Finally some conclusions are provided to close the text.2. Controller architectureSystem differential equation is only an approximate description of the actual plant due to various uncertainties and disturbances. Without loss of generality let us consider a nonlinear continuous dynamic system, which appears strange attractors under certain parameter conditions. With the relative degree r n(n is the dimension of the system), it can be directly described or transformed to the following normal form:121(,,)((,,)1)(,,,)(,,)r r r z z z z za z v wb z v u u d z v u u vc z v θθθθθθθθ-=⎧⎪⎪⎪=⎪=+∆+⎨⎪ ++∆-+⎪⎪ =+∆+⎪=+∆⎩ (1) 1y z =where θ is the parameter vector, θ∆ denotes parameter uncertainty, and w stands for the external disturbance, such that w M ≤with Mbeingpositive.In Eq.(1)1(,,)T r z z z = can be called external state variable vector,1(,,)T r n v v v += called internal state variable vector. As we can see from Eq.(1)(,,,,)(,,)((,,)1)d z v w u a z v w b z v uθθθθθθ+∆=+∆+ ++∆- (2)includes system nonlinearities, uncertainties, external disturbances and so on.According to the chaotic system (1), the following assumptions are introduced in order to establish the results concerned to the controller design (see more details in Ref.[38]).Assumption 1 The relative degree r of the chaotic system is finite and known.Assumption 2 The output variable y and its time derivatives i y up to order 1r -are measurable. Assumption 3 The zero dynamics of the systemis asymptotically stable, i.e.,(0,,)v c v θθ=+∆ is asymptotically stable.Assumption 4 The sign of function(,,)b z v θθ+∆is known such that it is always positive or negative.Since maybe not all the state vector is measurable, also (,,)a z v θθ+∆and (,,)b z v θθ+∆are not known, a controller with integral action is introduced to compensate theinfluenceof (,,,,)d z v w u θθ+∆. Namely,01121ˆr r u h z h z h z d------ (3) where110121112100ˆr i i i r r r r i i ii r i i d k z k k k z kz k uξξξ-+=----++-==⎧=+⎪⎪⎨⎪=----⎪⎩∑∑∑ (4)ˆdis the estimation to (,,,,)d z v w u θθ+∆. The controller parameters include ,0,,1i h i r =- and ,0,,1i k i r =- . Here011[,,,]Tr H h h h -= is Hurwitz vector, such that alleigenvalues of the polynomial121210()rr r P s s h sh s h s h --=+++++ (5)have negative real parts. The suitable positive constants ,0,,1i h i r =- can be chosen according to the expected dynamic characteristic. In most cases they are determined according to different designed requirements.Define 1((,,))r k sign b z v θμ-=, here μstands for a suitable positive constant, and the other parameters ,0,,2i k i r =- can be selected arbitrarily. After011[,,,]Tr H h h h -= is decided, we can tune ,0,,1i k i r =- toachievesatisfyingstaticperformances.Remark 1 In this section, we consider a n-dimensional nonlinear continuous dynamic system with strange attractors. By proper coordinate transformation, it can be represented to a normal form. Then a control system with a nonlinear compensator can be designed easily. In particular, the control parameters can be divided into two parts, which correspond to the dynamic characteristic and the static performance respectively (The theoretic analysis and more details about the controller can be referenced to Ref.[38]).3. Illustrative example-the Lorenz systemThe Lorenz system captures many of the features of chaotic dynamics, and many control methods have been tested on it.[17,20,22-23,27,30,32-35,42] However most of the existing methods is model-based and has not considered the influence ofpersistent external disturbances.The uncontrolled original Lorenz system can be described by112121132231233()()()()x P P x P P x w x R R x x x x w xx x b b x w =-+∆++∆+⎧⎪=+∆--+⎨⎪=-+∆+⎩ (6) where P and R are related to the Prendtl number and Rayleigh number respectively, and b is a geometric factor. P ∆, R ∆and b ∆denote the parametric variations respectively. The state variables, 1x ,2x and 3x represent measures of fluid velocity and the spatial temperature distribution in the fluid layer under gravity , and ,1,2,3i w i =represent external disturbance. In Lorenz system the desired response state variable is 1x . It is desired that 1x is regulated to 1r x , where 1r x is a given constant. In this section we consider two control schemes for system (6).3.1 Control schemes for Lorenz chaotic system3.1.1 Control scheme 1The control is acting at the right-side of the firstequation (1x), thus the controlled Lorenz system without disturbance can be depicted as1122113231231x Px Px u xRx x x x x x x bx y x =-++⎧⎪=--⎨⎪=-⎩= (7) By simple computation we know system (7) has relative degree 1 (i.e., the lowest ordertime-derivative of the output y which is directly related to the control u is 1), and can be rewritten as1122113231231z Pz Pv u vRz z v v v z v bv y z =-++⎧⎪=--⎨⎪=-⎩= (8) According to section 2, the following control strategy is introduced:01ˆu h z d=-- (9) 0120010ˆ-d k z k k z k uξξξ⎧=+⎪⎨=--⎪⎩ (10) Theorem 1 Under Assumptions 1 toAssumptions 4 there exists a constant value *0μ>, such that if *μμ>, then the closed-loop system (8), (9) and (10) is asymptotically stable.Proof Define 12d Pz Pv =-+, Eq.(8) can be easily rewritten as1211323123z d u v Rz z v v vz v bv =+⎧⎪=--⎨⎪=-⎩ (11) Substituting Eq.(9) into Eq.(11) yields101211323123ˆz h z d dv R z z v v v z v bv ⎧=-+-⎪=--⎨⎪=-⎩ (12) Computing the time derivative of d and ˆdand considering Eq.(12) yields12011132ˆ()()dPz Pv P h z d d P Rz z v v =-+ =--+- +-- (13) 0120010000100ˆ-()()ˆ=()d k z k k z k u k d u k d k z k d d k dξξξ=+ =--++ =-- - = (14)Defining ˆdd d =- , we have 011320ˆ()()dd d P h P R z P z v P v P k d=- =+- --+ (15) Then, we can obtain the following closed-loop system101211323123011320()()z h z dvRz z v v v z v bv d Ph PR z Pz v Pv P k d⎧=-+⎪=--⎪⎨=-⎪⎪=+---+⎩ (16) To stabilize the closed-loop system (16), a L yapunovfunction is defined by21()2V ςς=(17)where, ςdenotes state vector ()123,,,Tz v v d, isthe Euclidean norm. i.e.,22221231()()2V z v v dς=+++ (18) We define the following compact domain, which is constituted by all the points internal to the superball with radius .(){}2222123123,,,2U z v v d zv v dM +++≤(19)By taking the time derivative of ()V ςand replacing the system expressions, we have11223322*********01213()()(1)V z z v v v v dd h z v bv k P d R z v P R P h z d P v d P z v d ς=+++ =----++ +++-- (20) For any ()123,,,z v v d U ∈, we have: 222201230120123()()(1)V h z v b v k P dR z v PR Ph z d P v d d ς≤----+ ++++ ++ (21)Namely,12300()(1)22020V z v v dPR Ph R h R P ς⎡⎤≤- ⎣⎦++ - 0 - - 1 - 2⨯00123(1)()2Tb PR Ph P k P z v v d ⎡⎤⎢⎥⎢⎥⎢⎥⎢⎥⎢⎥⎢⎥0 ⎢⎥2⎢⎥++⎢⎥- - - +⎢⎥⎣22⎦⎡⎤⨯ ⎣⎦(22) So if the above symmetrical parameter matrix in Eq.(22) is positive definite, then V is negative and definite, which implies that system (16) is asymptotically stable based on L yapunov stability theory.By defining the principal minor determinants of symmetrical matrix in Eq.(22) as ,1,2,3,4i D i =, from the well-known Sylvester theorem it is straightforward to get the following inequations:100D h => (23)22004RD h =-> (24)23004R b D bh =-> (25)240302001()(1)(2)821[2(1)]08P M D k P D b PR Ph PR D Pb Ph R PR Ph =+-+++--+++>(26)After 0h is determined by solving Inequalities (23) to (25), undoubtedly, the Inequalities (26) can serve effectively as the constraints for the choice of 0k , i.e.20200031(1)(2)821[2(1)]8P M b PR Ph PR D Pb Ph R PR Ph k P D ++++ ++++>- (27)Here,20200*31(1)(2)821[2(1)]8P M b PR Ph PR D Pb Ph R PR Ph P D μ++++ ++++=-.Then the proof of the theorem 1 is completed. 3.1.2 Control scheme 2Adding the control signal on the secondequation (2x ), the system under control can be derived as112211323123x P x P x x R x x x x u xx x bx =-+⎧⎪=--+⎨⎪=-⎩ (28) From Eq.(28), for a target constant 11()r x t x =,then 1()0xt = , by solving the above differential equation, we get 21r r x x =. Moreover whent →∞,3r x converges to 12r x b . Since 1x and 2x havethe same equilibrium, then the measured state can also be chosen as 2x .To determine u , consider the coordinate transform:122133z x v x v x=⎧⎪=⎨⎪=⎩ and reformulate Eq.(28) into the following normal form:1223121231231zRv v v z u vPz Pv v z v bv y z =--+⎧⎪=-⎨⎪=-⎩= (29) thus the controller can be derived, which has the same expression as scheme 1.Theorem 2 Under Assumptions 1, 2, 3 and 4, there exists a constant value *0μ>, such that if *μμ>, then the closed-loop system (9), (10) and (29) is asymptotically stable.Proof In order to get compact analysis, Eq.(29) can be rewritten as12123123z d u v P z P v vz v bv =+⎧⎪=-⎨⎪=-⎩ (30) where 2231d Rv v v z =--Substituting Eq.(9) into Eq.(30),we obtain:1012123123ˆz h z d dv P z P v v z v bv ⎧=-+-⎪=-⎨⎪=-⎩ (31) Giving the following definition:ˆdd d =- (32) then we can get22323112123212301()()()()dRv v v v v z R Pz Pv Pz Pv v v z v bv h z d =--- =--- ----+ (33) 012001000ˆ-()d k z k k z k u k d u k dξξ=+ =--++ = (34) 121232123010ˆ()()()(1)dd d R Pz Pv Pz Pv v v z v bv h z k d=- =--- --+-+ (35)Thus the closed-loop system can be represented as the following compact form:1012123123121232123010()()()(1)zh z d v Pz Pv v z v bv d R Pz Pv Pz Pv v v z v bv h z k d⎧=-+⎪⎪=-⎪=-⎨⎪=---⎪⎪ --+-+⎩(36) The following quadratic L yapunov function is chosen:21()2V ςς=(37)where, ςdenotes state vector ()123,,,Tz v v d , is the Euclidean norm. i.e.,22221231()()2V z v v dς=+++ (38) We can also define the following compact domain, which is constituted by all the points internalto the super ball with radius .(){}2222123123,,,2U z v v d zv v dM =+++≤ (39)Differentiating V with respect to t and using Eq.(36) yields112233222201230011212322321312()(1)(1)()V z z v v v v dd h z P v bv k dP R h z d P z v z v v P b v v d P v d P z v d z v d ς=+++ =----+ +++++ ++--- (40)Similarly, for any ()123,,,z v v d U ∈, we have: 2222012300112133231()(1)(1)(2V h z P v b v k dPR h z d P z v v P b d P v d d M z dς≤----+ +++++ ++++ + (41)i.e.,12300()(12)22V z v v dPR M h P h P Pς⎡⎤≤- ⎣⎦+++ - -2 - 0 ⨯ 001230(12)(1)2TP b PR M h P k z v v d ⎡⎤⎢⎥⎢⎥⎢⎥ - ⎢⎥⎢⎥⎢⎥ ⎢⎥22⎢⎥⎢⎥ +++ - - -+⎢⎥⎣22⎦⎡⎤⨯ ⎣⎦(42) For brevity, Let1001(12)[(222)82(23)]P PR M h b PR P h M P b α=++++++ ++(43) 2201[(231)(13)]8P M P b b PR h α=+-+++ (44)230201(2)[2(12)8(2)(4)]PM P b P P PR M h P b Ph P α=++ +++ ++- (45)Based on Sylvester theorem the following inequations are obtained:100D h => (46)22004PD h P =-> (47)3202PMD bD =-> (48)403123(1)0D k D ααα=+---> (49)where,1,2,3,4i D i =are the principal minordeterminants of the symmetrical matrix in Eq.(42).*0k μ>*12331D αααμ++=- (50)The theorem 2 is then proved.Remark 2 In this section we give two control schemes for controlling chaos in Lorenz system. For each scheme the control depends on the observed variable only, and two control parameters are neededto be tuned, viz. 0h and 0k . According to L yapunov stability theory, after 0h is fixed, the sufficient condition for the choice of parameter 0k is also obtained.4. Simulation resultsChoosing 10P =,28R =, and 8/3b =, the uncontrolled Lorenz system exhibits chaotic behavior, as plotted in Fig.1. In simulation let the initial values of the state of thesystembe 123(0)10,(0)10,(0)10x x x ===.x1x 2x1x 3Fig.1. C haotic trajectories of Lorenz system (a) projected on12x x -plane, (b) projected on 13x x -plane4.1 Simulation results of control the trajectory to steady stateIn this section only the simulation results of control scheme 2 are depicted. The simulation results of control scheme 1 will be given in Appendix. For the first five seconds the control input is not active, at5t s =, control signal is input and the systemtrajectory is steered to set point2121(,,)(8.5,8.5,27.1)T Tr r r x x x b =, as can be seen inFig.2(a). The time history of the L yapunov function is illustrated in Fig.2(b).t/sx 1,x 2,x 3t/sL y a p u n o v f u n c t i o n VFig.2. (a) State responses under control, (b) Time history of the Lyapunov functionA. Simulation results in the presence ofparameters ’ changeAt 9t s =, system parameters are abruptly changed to 15P =,35R =, and 12/3b =. Accordingly the new equilibrium is changedto 2121(,,)(8.5,8.5,18.1)T Tr r r x x x b =. Obviously, aftervery short transient duration, system state converges to the new point, as shown in Fig.3(a). Fig.4(a) represents the evolution in time of the L yapunov function.B. Simulation results in the presence of set pointchangeAt 9t s =, the target is abruptly changedto 2121(,,)(12,12,54)T Tr r r x x x b =, then the responsesof the system state are shown in Fig.3(b). In Fig.4(b) the time history of the L yapunov function is expressed.t/sx 1,x 2,x 3t/sx 1,x 2,x 3Fig.3. State responses (a) in the presence of parameter variations, (b) in the presence of set point changet/sL y a p u n o v f u n c t i o n Vt/sL y a p u n o v f u n c t i o n VFig.4. Time history of the Lyapunov fu nction (a) in the presence of parameter variations, (b) in the presence of set point changeC. Simulation results in the presence ofdisturbanceIn Eq.(5)external periodic disturbance3cos(5),1,2,3i w t i π==is considered. The time responses of the system states are given in Fig.5. After control the steady-state phase plane trajectory describes a limit cycle, as shown in Fig.6.t/sx 1,x 2,x 3Fig.5. State responses in the presence of periodic disturbancex1x 3Fig.6. The state space trajectory at [10,12]t ∈in the presence ofperiodic disturbanceD. Simulation results in the presence of randomnoiseUnder the influence of random noise,112121132231233xPx Px x Rx x x x u xx x bx εδεδεδ=-++⎧⎪=--++⎨⎪=-+⎩ (51) where ,1,2,3i i δ= are normally distributed withmean value 0 and variance 0.5, and 5ε=. The results of the numerical simulation are depicted in Fig.7,which show that the steady responses are hardly affected by the perturbations.t/sx 1,x 2,x 3t/se 1,e 2,e 3Fig.7. Time responses in the presence of random noise (a) state responses, (b) state tracking error responses4.2 Simulation results of control the trajectory to periodic orbitIf the reference signal is periodic, then the system output will also track this signal. Figs.8(a) to (d) show time responses of 1()x t and the tracking trajectories for 3-Period and 4-period respectively.t/sx 1x1x 2t/sx 1x1x 2Fig.8. State responses and the tracking periodic orbits (a)&( b)3-period, (c)&(d) 4-periodRemark 3 The two controllers designed above solved the chaos control problems of Lorenz chaoticsystem, and the controller design method can also beextended to solve the chaos suppression problems of the whole Lorenz system family, namely the unified chaotic system.[44-46] The detail design process and close-loop system analysis can reference to the author ’s another paper.[47] In Ref.[47] according to different positions the scalar control input added,three controllers are designed to reject the chaotic behaviors of the unified chaotic system. Taking the first state 1x as the system output, by transforming system equation into the normal form firstly, the relative degree r (3r ≤) of the controlled systems i s known. Then we can design the controller with the expression as Eq.(3) and Eq.(4). Three effective adaptive nonlinear compensating mechanisms are derived to compensate the chaotic system nonlinearities and external disturbances. According toL yapunov stability theory sufficient conditions for the choice of control parameters are deduced so that designers can tune the design parameters in an explicit way to obtain the required closed loop behavior. By numeric simulation, it has been shown that the designed three controllers can successfully regulate the chaotic motion of the whole family of the system to a given point or make the output state to track a given bounded signal with great robustness.5. ConclusionsIn this letter we introduce a promising tool to design control system for chaotic system subject to persistent disturbances, whose entire dynamics is assumed unknown and the state variables are not completely measurable. By integral action the nonlinearities, including system structure nonlinearity, various disturbances, are compensated successfully. It can handle, therefore, a large class of chaotic systems, which satisfy four assumptions. Taking chaotic Lorenz system as an example, it has been shown that the designed control scheme is robust in the sense that the unmeasured states, parameter uncertainties and external disturbance effects are all compensated and chaos suppression is achieved. Some advantages of this control strategy can be summarized as follows: (1) It is not limited to stabilizing the embeddedperiodic orbits and can be any desired set points and multiperiodic orbits even when the desired trajectories are not located on the embedded orbits of the chaotic system.(2) The existence of parameter uncertainty andexternal disturbance are allowed. The controller can be designed according to the nominal system.(3) The dynamic characteristics of the controlledsystems are approximately linear and the transient responses can be regulated by the designer through controllerparameters ,0,,1i h i r =- .(4) From L yapunov stability theory sufficientconditions for the choice of control parameters can be derived easily.(5) The error converging speed is very fast evenwhen the initial state is far from the target one without waiting for the actual state to reach the neighborhood of the target state.AppendixSimulation results of control scheme 1.t/sx 1,x 2,x 3t/sL y a p u n o v f u n c t i o n VFig.A1. (a) State responses u nder control, (b) Time history of the Lyapunov functiont/sx 1,x 2,x 3t/sx 1,x 2,x 3Fig.A2. State responses (a) in the presence of parameter variations, (b) in the presence of set point changet/sL y a p u n o v f u n c t i o n Vt/sL y a p u n o v f u n c t i o n VFig.A3. Time history of the L yapu nov fu nction (a) in the presence of parameter variations, (b) in the presence of set point changet/sx 1,x 2,x 3Fig.A4. State responses in the presence of periodic disturbanceresponsest/sx 1,x 2,x 3Fig.A5. State responses in the presence of rand om noiset/sx 1x1x 2Fig.A6. State response and the tracking periodic orbits (4-period)References[1] Lü J H, Zhou T S, Zhang S C 2002 C haos Solitons Fractals 14 529[2] Yoshihiko Nagai, Hua X D, Lai Y C 2002 C haos Solitons Fractals 14 643[3] Li R H, Xu W , Li S 2007 C hin.phys.16 1591 [4]Xiao Y Z, Xu W 2007 C hin.phys.16 1597[5] Ott E ,Greb ogi C and Yorke J A 1990 Phys.Rev .Lett. 64 1196 [6]Yoshihiko Nagai, Hua X D, Lai Y C 1996 Phys.Rev.E 54 1190 [7] K.Pyragas, 1992 Phys. Lett. A 170 421 [8] Lima,R and Pettini,M 1990 Phys.Rev.A 41 726[9] Zhou Y F, Tse C K, Qiu S S and Chen J N 2005 C hin.phys. 14 0061[10] G .Cicog na, L.Fronzoni 1993 Phys.Rew .E 30 709 [11] Rakasekar,S. 1993 Pramana-J.Phys.41 295 [12] Gong L H 2005 Acta Phys.Sin.54 3502 (in C hinese) [13] Chen L,Wang D S 2007 Acta Phys.Sin.56 0091 (in C hinese) [14] C hen G R and Dong X N 1993 IEEE Trans.on Circuits andSystem-Ⅰ:Fundamental Theory and Applications 40 9 [15] J.L. Kuang, P.A. Meehan, A.Y.T. Leung 2006 C haos SolitonsFractals 27 1408[16] Li R H, Xu W, Li S 2006 Acta Phys.Sin.55 0598 (in C hinese) [17] Yu X 1996 Int.J.of Systems Science 27 355[18] Hsun-Heng Tsai, C hyu n-C hau Fuh and Chiang-Nan Chang2002 C haos,Solitons Fractals 14 627[19] Her-Terng Yau and C hieh-Li C hen 2006 C hao ,SolitonsFractal 30 709[20] Guo H J, Liu J H, 2004 Acta Phys.Sin.53 4080 (in C hinese) [21] Yu D C, Wu A G , Yang C P 2005 Chin.phys.14 0914 [22] C hyu n-C hau Fuh and Pi-Cheng Tu ng 1995 Phys.Rev .Lett.752952[23] Chen L Q, Liu Y Z 1998 Applied Math.Mech. 19 63[24] Liu D, R en H P, Kong Z Q 2003 Acta Phys.Sin.52 0531 (inChinese)[25] Liu H, Liu D and Ren H P 2005 Acta Phys.Sin.54 4019 (inChinese)[26] C hang W , Park JB, Joo YH, C hen GR 2002 Inform Sci 151227[27] Gao X, Liu X W 2007 Acta Phys.Sin. 56 0084 (in C hinese) [28] Chen S H, Liu J, Lu J 2002 C hin.phys.10 233 [29] Lu J H, Zhang S. 2001 Phys. Lett. A 286 145[30] Liu J, Chen S H, Xie J. 2003 C haos Solitons Fractals 15 643 [31] Wang J, Wang J, Li H Y 2005 C haos Solitons Fractals 251057[32] Wu X Q, Lu JA, C hi K. Tse, Wang J J, Liu J 2007 ChaoSolitons Fractals 31 631[33] A.L.Fradkov , R .J.Evans, 2002 Preprints of 15th IF AC W orldCongress on Automatic Control 143[34] Zhang H G 2003 C ontrol theory of chaotic systems (Shenyang:Northeastern University) P38 (in C hinese)[35] Yu-Chu Tian, Moses O.Tadé, David Levy 2002Phys.Lett.A.296 87[36] Jose A R , Gilberto E P, Hector P, 2003 Phys. Lett. A 316 196 [37] Liao X X, Yu P 2006 Chaos Solitons Fractals 29 91[38] Tornambe A, V aligi P.A 1994 Measurement, and C ontrol 116293[39] Andrew Y.T.Leung, Liu Z R 2004 Int.J.Bifurc.C haos 14 2955 [40] Qu Z L, Hu,G .,Yang,G J, Qin,G R 1995 Phys.Rev .Lett.74 1736 [41] Y ang J Z, Qu Z L, Hu G 1996 Phys.Rev.E.53 4402[42] Shyi-Kae Yang, C hieh-Li Chen, Her-Terng Yau 2002 C haosSolitons Fractals 13 767。
电力翻译词汇[1]
![电力翻译词汇[1]](https://img.taocdn.com/s3/m/de01050c844769eae009edd6.png)
Apparent Power (KVA)
中线电流 Neutral Current (N Amp)
功率因数 Power Factor (PF)
频率
Frequency(HZ)
千瓦 Active Power (KW)
千阀
Reactive Power (KVAr)
电力词汇
标准的机组数据显示
(Standard Measurement And Display Data)
负载电流百分比显示 Percentage of Current load(%)
单相/三相电压
Voltage by One/Three Phase (Volt.)
每相电流 Current by Phase (AMP)
抗干扰能力
antijamming capability
自适应系统
adaptive system
行波继电器
travelling wave relay
输电线路故障
transmission line malfunction
仿真
simulation
算法
algorithm
超高压输电线
supervltage transmission power line
投运
commissioning
行波保护
Traveling wave protection
自适应控制方法
adaptive control process
动作速度
speed of action
机组运行小时表
Genset Running Hour Meter
六旋翼植保无人机模糊自适应PID控制

六旋翼植保无人机模糊自适应PID控制李永伟;王红飞【摘要】六旋翼植保无人机在作业过程中自身载荷变化将引起飞行控制性能下降、抗扰动能力降低等问题.为了提高六旋翼植保无人机的可控性,通过对六旋翼植保无人机在喷洒农药过程中进行分析和建模,推导出植保无人机时变动力学模型,提出了一种模糊自适应PID控制算法,模糊自适应PID算法适应性强,参数整定简单,提高了系统动态响应和稳态性能.将各个传感器的测量参数输入到模糊自适应PID算法中,可以得到对应的控制量,实现飞行器稳定运行.通过使用Matlab软件对飞行系统进行仿真,并结合实验平台实际飞行控制表明,系统的动态性能和稳定性得到了有效提高.【期刊名称】《河北科技大学学报》【年(卷),期】2017(038)001【总页数】7页(P59-65)【关键词】自动控制理论;模糊PID;植保无人机;飞行控制;飞行器建模【作者】李永伟;王红飞【作者单位】河北科技大学电气工程学院,河北石家庄 050018;河北科技大学电气工程学院,河北石家庄 050018【正文语种】中文【中图分类】TP273;V249.121李永伟, 王红飞.六旋翼植保无人机模糊自适应PID控制[J].河北科技大学学报,2017,38(1):59-65. LI Yongwei, WANG Hongfei.Fuzzy adaptive PID control for six rotor eppo UAV [J].Journal of Hebei University of Science and Technology,2017,38(1):59-65.六旋翼植保无人机是一种典型的多变量欠驱动时变系统,且系统具有强耦合性的特点[1-3]。
植保无人机与其他无人飞行器有很多不同点,植保无人机的主要特点是靠近地面低空飞行,并且无人机在喷散农药作业时,很容易受到地面地势的变化、局部气流的变化、电池电量变化和自身质量变化的影响。
电力专业英语词汇-详全
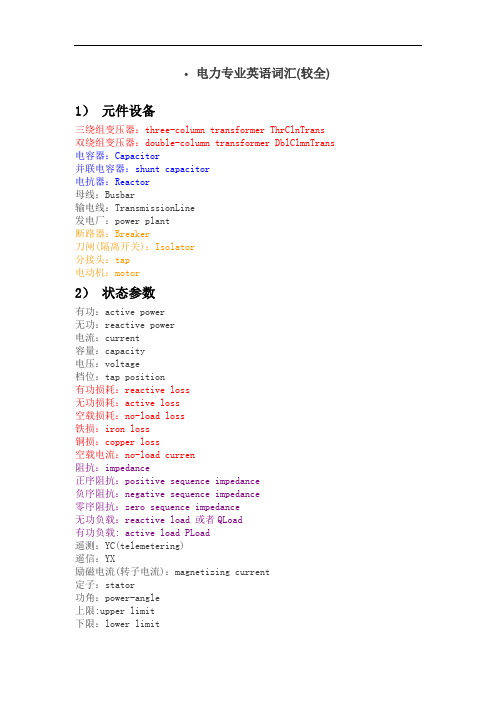
电力专业英语词汇(较全)1)元件设备三绕组变压器:three-column transformer ThrClnTrans 双绕组变压器:double-column transformer DblClmnTrans 电容器:Capacitor并联电容器:shunt capacitor电抗器:Reactor母线:Busbar输电线:TransmissionLine发电厂:power plant断路器:Breaker刀闸(隔离开关):Isolator分接头:tap电动机:motor2)状态参数有功:active power无功:reactive power电流:current容量:capacity电压:voltage档位:tap position有功损耗:reactive loss无功损耗:active loss空载损耗:no-load loss铁损:iron loss铜损:copper loss空载电流:no-load curren阻抗:impedance正序阻抗:positive sequence impedance负序阻抗:negative sequence impedance零序阻抗:zero sequence impedance无功负载:reactive load 或者QLoad有功负载: active load PLoad遥测:YC(telemetering)遥信:YX励磁电流(转子电流):magnetizing current定子:stator功角:power-angle上限:upper limit下限:lower limit并列的:apposable高压: high voltage低压:low voltage中压:middle voltage电力系统 power system发电机 generator励磁 excitation励磁器 excitor电压 voltage电流 current母线 bus变压器 transformer升压变压器 step-up transformer高压侧 high side输电系统 power transmission system输电线 transmission line固定串联电容补偿fixed series capacitor compensation 稳定 stability电压稳定 voltage stability功角稳定 angle stability暂态稳定 transient stability电厂 power plant能量输送 power transfer交流 AC装机容量 installed capacity电网 power system落点 drop point开关站 switch station双回同杆并架 double-circuit lines on the same tower 变电站 transformer substation补偿度 degree of compensation高抗 high voltage shunt reactor无功补偿 reactive power compensation故障 fault调节 regulation裕度 magin三相故障 three phase fault故障切除时间 fault clearing time极限切除时间 critical clearing time切机 generator triping高顶值 high limited value强行励磁 reinforced excitation线路补偿器 LDC(line drop compensation)机端 generator terminal静态 static (state)动态 dynamic (state)单机无穷大系统 one machine - infinity bus system 机端电压控制 AVR功角 power angle有功(功率) active power无功(功率) reactive power功率因数 power factor无功电流 reactive current下降特性 droop characteristics斜率 slope额定 rating变比 ratio参考值 reference value电压互感器 PT分接头 tap下降率 droop rate仿真分析 simulation analysis传递函数 transfer function框图 block diagram受端 receive-side裕度 margin同步 synchronization失去同步 loss of synchronization阻尼 damping摇摆 swing保护断路器 circuit breaker电阻:resistance电抗:reactance阻抗:impedance电导:conductance电纳:susceptance导纳:admittance电感:inductance电容: capacitanceAGC Automatic Generation Control自动发电控制AMR Automatic Message Recording 自动抄表ASS Automatic Synchronized System 自动准同期装置ATS Automatic Transform System 厂用电源快速切换装置AVR Automatic Voltage Regulator 自动电压调节器BCS Burner Control System 燃烧器控制系统BMS Burner Management System 燃烧器管理系统CCS Coordinated Control System 协调控制系统CRMS Control Room Management System 控制室管理系统CRT Cathode Ray Tube 阴极射线管DAS Data Acquisition System 数据采集与处理系统DCS Distributed Control System 分散控制系统DDC Direct Digital Control 直接数字控制(系统)DEH Digital Electronic Hydraulic Control 数字电液(调节系统)DPU Distributed Processing Unit 分布式处理单元EMS Energy Management System 能量管理系统ETS Emergency Trip System 汽轮机紧急跳闸系统EWS Engineering Working Station 工程师工作站FA Feeder Automation 馈线自动化FCS Field bus Control System 现场总线控制系统FSS Fuel Safety System 燃料安全系统FSSS Furnace Safeguard Supervisory System 炉膛安全监控系统GIS Gas Insulated Switchgear 气体绝缘开关设备GPS Global Position System 全球定位系统HCS Hierarchical Control System 分级控制系统LCD Liquid Crystal Display 液晶显示屏LCP Local Control Panel 就地控制柜MCC Motor Control Center (电动机)马达控制中心MCS Modulating Control System 模拟量控制系统MEH Micro Electro Hydraulic Control System 给水泵汽轮机电液控制系统MIS Management Information System 管理信息系统NCS Net Control System 网络监控系统OIS Operator Interface Station 操作员接口站OMS Outage Management System 停电管理系统PID Proportion Integration Differentiation 比例积分微分PIO Process inputOutput 过程输入输出(通道)PLC Programmable Logical Controller 可编程逻辑控制器PSS Power System Stabilizator 电力系统稳定器SCADA Supervisory Control And Data Acquisition 数据采集与监控系统SCC Supervisory Computer Control 监督控制系统SCS Sequence Control System 顺序(程序)控制系统SIS Supervisory Information System 监控信息系统TDCS(TDC)Total Direct Digital Control 集散控制系统TSI Turbine Supervisory Instrumentation 汽轮机监测仪表UPS Uninterrupted Power Supply 不间断供电专业英语(电力词汇)标准的机组数据显示 (Standard Measurement And Display Data) 负载电流百分比显示 Percentage of Current load(%)单相/三相电压 Voltage by One/Three Phase (Volt.)每相电流 Current by Phase (AMP)千伏安Apparent Power (KVA)中线电流 Neutral Current (N Amp)功率因数 Power Factor (PF)频率 Frequency(HZ)千瓦 Active Power (KW)千阀 Reactive Power (KVAr)最高/低电压及电流 Max/Min. Current and Voltage输出千瓦/兆瓦小时 Output kWh/MWh运行转速 Running RPM机组运行正常 Normal Running超速故障停机 Overspeed Shutdowns低油压故障停机 Low Oil Pressure Shutdowns高水温故障停机 High Coolant Temperature Shutdowns起动失败停机 Fail to Start Shutdowns冷却水温度表 Coolant Temperature Gauge机油油压表 Oil Pressure Gauge电瓶电压表 Battery Voltage Meter机组运行小时表 Genset Running Hour Meter怠速-快速运行选择键 Idle Run – Normal Run Selector Switch运行-停机-摇控启动选择键 Local Run-Stop-Remote Starting Selector Switch 其它故障显示及输入 Other Common Fault Alarm Display and电力行波词汇行波travelling wave模糊神经网络fuzzy-neural network神经网络neural network模糊控制fuzzy control研究方向 research direction副教授associate professor电力系统the electrical power system大容量发电机组large capacity generating set输电距离electricity transmission超高压输电线super voltage transmission power line投运commissioning行波保护Traveling wave protection自适应控制方法adaptive control process动作速度speed of action行波信号travelling wave signal测量信号measurement signal暂态分量transient state component非线性系统nonlinear system高精度high accuracy自学习功能self-learning function抗干扰能力anti-jamming capability自适应系统adaptive system行波继电器travelling wave relay输电线路故障transmission line malfunction仿真simulation算法algorithm电位electric potential短路故障short trouble子系统subsystem大小相等,方向相反equal and opposite in direction电压源voltage source故障点trouble spot等效于equivalent暂态行波transient state travelling wave偏移量side-play mount电压electric voltage附加系统add-ons system波形waveform工频power frequency延迟变换delayed transformation延迟时间delay time减法运算subtraction相减运算additive operation求和器summator模糊规则fuzzy rule参数值parameter values可靠动作action message等值波阻抗equivalent value wave impedance附加网络additional network修改的modified反传算法backpropagation algorithm隶属函数membership function模糊规则fuzzy rule模糊推理fuzzy reasoning模糊推理矩阵fuzzy reasoning matrix样本集合 sample set给定的given采样周期sampling period三角形隶属度函数Triangle-shape grade of membership function 负荷状态load conditions区内故障troubles inside the sample space门槛值threshold level采样频率sampling frequency全面地all sidedly样本空间sample space误动作malfunction保护特性protection feature仿真数据simulation data灵敏性sensitivity小波变换wavelet transformation神经元neuron谐波电流harmonic current电力系统自动化power system automation继电保护relaying protection中国电力 China Power学报 journal初探primary exploration电机学 electrical machinery自动控制理论 automatic control theory电磁场 electromagnetic field电磁场与电磁波& Magnetic Waves微机原理 principle of microcomputer电工学 electrotechnics principle of circuits电力系统稳态分析 steady-state analysis of power system电力系统暂态分析 transient-state analysis of power system电力系统继电保护原理 principle of electrical system's relay protection 电力系统元件保护原理 protection principle of power system 's element 电力系统内部过电压 past voltage within power system模拟电子技术基础 basis of analogue electronic technique数字电子技术 digital electrical technique电路原理实验lab. of principle of circuits电气工程讲座 lectures on electrical power production电力电子基础basic fundamentals of power electronics高电压工程high voltage engineering电子专题实践topics on experimental project of electronics 电气工程概论introduction to electrical engineering电子电机集成系统electronic machine system电力传动与控制electrical drive and control电力电子电路Power Electronic Circuit电力电子电器Power Electronic Equipment电力电子器件Power Electronic Devices电力电子学Powe r Electronics电力工程Electrical Power Engineering电力生产技术Technology of Electrical Power Generation电力生产优化管理Optimal Management of Electrical Power Generation电力拖动基础Fundamentals for Electrical Towage电力拖动控制系统Electrical Towage Control Systems电力系统Power Systems电力系统电源最优化规划Optimal Planning of Power Source in a Power System 电力系统短路Power System Shortcuts电力系统分析Power System Analysis电力系统规划Power System Planning电力系统过电压Hyper-Voltage of Power Systems电力系统继电保护原理Power System Relay Protection电力系统经济分析Economical Analysis of Power Systems电力系统经济运行Economical Operation of Power Systems电力系统可靠性Power System Reliability电力系统可靠性分析Power System Reliability Analysis电力系统无功补偿及应用Non-Work Compensation in Power Systems & Applicati 电力系统谐波Harmonious Waves in Power Systems电力系统优化技术Optimal Technology of Power Systems电力系统优化设计Optimal Designing of Power Systems电力系统远动Operation of Electric Systems电力系统远动技术Operation Technique of Electric Systems电力系统运行Operation of Electric Systems电力系统自动化Automation of Electric Systems电力系统自动装置Power System Automation Equipment电路测试技术Circuit Measurement Technology电路测试技术基础Fundamentals of Circuit Measurement Technology感应定律law of induction励磁 excitation 励磁器 magnetizing exciter励磁器 exciter 恒定励磁器constant exciter励磁器激振器exciter励磁电流:magnetizing current 强行励磁reinforced excitation励磁调节器excitation regulator无功伏安volt-ampere reactive无功伏安时volt-ampere-hour reactive稳态控制homeostatic control; stable control a steady-state control 水电厂hydroelectric station落点 drop point 调节 regulation调节器conditioner 励磁调节器excitation regulator调速器regulator, governor ;speed regulator ;(正规)speed governor 高抗 high voltage shunt reactor并列的: apposite; paratactic 同步 synchronization系统解列system splitting( trip)失去同步loss of synchronization 分接头:tap 裕度 margin 档位:tap position故障 fault 三相故障 three phase fault 切机 generator triping故障切除时间fault clearing ti me高顶值 high limited value静态 static (state) 动态 dynamic (state) 暂态transient机端电压控制 avr电动机:motor有功负载: active load/pload 无功负载:reactive load电压互感器pt (potential /voltage transformer )参考值 reference value 单机无穷大系统one machine - infinity bus system 仿真分析 simulation analysis 下降率 droop rate传递函数 transfer function 框图 block diagram受端 receive-side 同步 synchronization保护断路器 circuit breaker阻尼 damping无刷直流电机:brusless dc motor永磁电动机permanent-magnet current motor机端 generator terminal永磁同步电机:permanent-magnet synchronism motor异步电机:asynchronous motor三绕组变压器:three-column transformer thrclntransthree winding transformer双绕组变压器:double-column transformer dblclmntranstwo-circuit transformer; two-winding transformer固定串联电容补偿fixed series capacitor compensation双回同杆并架 double-circuit lines on the same tower单机无穷大系统 one machine - infinity bus system偿度 degree of compensation电磁场失去同步electromagnetic fields loss of synchronization装机容量 installed capacity无功补偿 reactive power compensation故障切除时间 fault clearing time极限切除时间 critical clearing time强行励磁 reinforced excitation并联电容器:shunt capacitor下降特性 droop characteristics线路补偿器 ldc(line drop compensation)。
基于Holt双参数指数平滑的图像椒盐噪声滤除
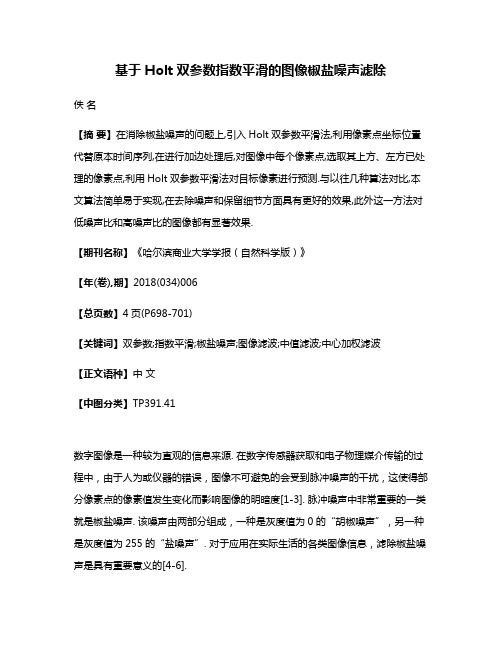
基于Holt双参数指数平滑的图像椒盐噪声滤除佚名【摘要】在消除椒盐噪声的问题上,引入Holt双参数平滑法,利用像素点坐标位置代替原本时间序列,在进行加边处理后,对图像中每个像素点,选取其上方、左方已处理的像素点,利用Holt双参数平滑法对目标像素进行预测.与以往几种算法对比,本文算法简单易于实现,在去除噪声和保留细节方面具有更好的效果,此外这一方法对低噪声比和高噪声比的图像都有显著效果.【期刊名称】《哈尔滨商业大学学报(自然科学版)》【年(卷),期】2018(034)006【总页数】4页(P698-701)【关键词】双参数;指数平滑;椒盐噪声;图像滤波;中值滤波;中心加权滤波【正文语种】中文【中图分类】TP391.41数字图像是一种较为直观的信息来源. 在数字传感器获取和电子物理媒介传输的过程中,由于人为或仪器的错误,图像不可避免的会受到脉冲噪声的干扰,这使得部分像素点的像素值发生变化而影响图像的明暗度[1-3]. 脉冲噪声中非常重要的一类就是椒盐噪声. 该噪声由两部分组成,一种是灰度值为0的“胡椒噪声”,另一种是灰度值为255的“盐噪声”. 对于应用在实际生活的各类图像信息,滤除椒盐噪声是具有重要意义的[4-6].为了去除椒盐噪声并保证图像质量,传统中值滤波(MF)被提出[7]. 中值滤波作为一种非线性滤波,其去噪效果远优于线性滤波,这使得中值滤波成为目前针对椒盐噪声去噪所使用的最广泛的滤波方法之一. 中值滤波去噪主要依靠滤波窗口尺寸的选择,窗口越小,去噪能力越弱,但保留细节的能力越强. 反之,窗口越大,去噪能力越强,但保留细节的能力越弱,图像越模糊[8-10].为了在噪声消除与细节保留中取舍,Ko和Lee提出了中心加权滤波(CWM)[11].他们采用权重平衡中心点或在滑动窗口中原本的像素点. 实验表明,在细节保留上,使用更大中心点权值的中心加权滤波效果要优于采用较小的,而在噪声消除上结果相反.在消除脉冲噪声时,只对噪声点进行滤波消除而非噪声点保持不变,能更好地保留图像细节. 所以,噪声消除时使用选择滤波器会具有更好的视觉效果. 三态中值滤波(TSM)在噪声检测过程中使用SMF与CWM滤波的输出结果,将其与原像素值或中心像素值进行比较来做出三态选择,从而在高效消除噪声的同时仍能够保留较多的图像细节,但也保留了SMF和CWM的缺点[12].细节保留与噪声消除的制约关系存在于各种中值滤波中,为进一步改善这种情况,本文引入Holt双参数指数平滑的方法,构建一种能够修匀具有线性趋势序列的算法(Holt′s Two-Parameter Smoothing Filter ,简称HF)来对含有椒盐噪声的图像进行滤除修复.1 本文算法Holt双参数指数平滑法是一种线性指数平滑的方法[13]. 这种方法的主要操作是对原时间序列进行迭代,并通过预测得到未来几期的参数值. 由于其具有很大的灵活性与准确性而被广泛地使用在金融、环境、资源等领域. 该方法包括两个平滑公式和一个预测公式,两个平滑公式是分别对时间和趋势的平滑:St=αyt+(1-α)(St-bt-1)(1)bt=γ(St-St-1 )+(1-γ)bt-1(2)预测公式为:(3)其中:α、γ为平滑参数,yt为实际参考值,T为预测期数.本文创新性地将Holt双参数指数平滑法引入到数字图像处理领域. 利用Holt双参数指数平滑法对含有椒盐噪声且像素值变化具有一定的线性趋势的图像进行修匀平滑. 对含有椒盐噪声的图像,取定一个像素点,向上方取若干个像素点作为参考依据,用从下到上的像素值排列次序替换原本平滑法中的时间序列进行处理. 经实验得知,步长为4时,去噪效果较优,故下述算法均带入四个参考点进行计算. 由于仅需要预测一期的数据,所以将T设定为1. 根据噪声点的个数分成下列两种情况进行讨论:当参考点不全为噪声点时,用像素点坐标位置代替原公式的时间序列,取定中心像素点,将其上方的参考点按距离中心像素点由远及近的排列顺序代入到Holt双参数指数平滑法进行迭代,得到预测值并取代该点像素值:img(i,j)=HF(img(i-1,j),img(i-2,j),img(i-3,j),img(i-4,j))(4)当参考点全部为噪声点时,由于滤波扫描方向为从左到右,从上而下,所以仅有左侧像素点具有参考价值,故以左方参考点像素值取代该点像素值:img(i,j)=img(i,j-1)(5)1.1 噪声检测阶段设置噪声标记矩阵Sig,假设噪声图像img在坐标(i,j)点处的灰度值为img (i,j). 若img (i,j)为0或255,则认为是噪声点并进行标记[14]:(6)其中:Sig(i,j)=1代表该点为噪声点,Sig(i,j)=0代表该点为信号点,以此为参照依据进行滤除操作.1.2 噪声消除阶段本算法以噪声图像左上角像素点作为起始点,从左到右,从上至下进行扫描滤除. 经多次实验,设定平滑参数α=0.65, β=0.5, T=1. 对img(i,j)进行下述操作:步骤1 选取img(i,j)正上方4个像素点作为参考,若其中有不超过3个噪声点,即Sig(i-1,j)+Sig(i-2,j)+Sig(i-3,j)+Sig(i-4,j)≤3则进行步骤2,否则进行步骤3.步骤2 将4个参考值代入计算函数中进行迭代运算,其迭代公式为:Sn=αSn-1+(1-α)(Sn-1+Bn-1)Bn=β(Sn-Sn-1)+(1-β)Sn-1其中:n=2,3,4,α,β为平滑参数.为求解迭代方程需设定两项初值条件:S1=img(i-4,j) ,B1=img(i-4,j)+img(i-3,j)得到最终预测值:选用最终预测值来替代img(i,j):步骤3 选择用左侧像素点的灰度值来代替img(i,j):img(i,j)=img(i,j+1)步骤4 判断是否结束扫描,如果结束进行步骤5,否则进行步骤1.步骤5 将修正后的图像输出.2 仿真实验与结果分析本文通过实验验证了HF算法的去噪能力和细节保留能力. 实验过程中选用分辨率大小为512×512的Lena图像作为测试图像,对标准中值滤波(MF)、中心加权滤波(CWM)、三态中值滤波(TSM)和Holt双参数指数平滑滤波(HF)进行比较. 其中MF的窗口尺寸分为3×3和5×5;CWM的参数W_C设置为3 ;TSM的阈值T设置为20.实验环境为Windows10(64位)操作系统,Intel© CoreTM i7-6700HQ处理器(2.6GHz),8GB内存. 仿真软件为Matlab R2016a.本文采用峰值信噪比(Peak Signal to Noise Ratio , RPSN)作为客观评价标准,其定义如下[15]:其中:O(i,j)和R(i,j)分别代表原始图像和噪声修复图像在(i,j)处的像素值.在图1中,可以看出MF在50%噪声浓度下,窗口尺寸的增大可以增强去噪能力,但是细节保留能力也会迅速下降,导致图像模糊. CWM的去噪效果明显弱于MF,噪声漏检率较大. TSM既包括以上两种算法优点,同时也保留了它们的缺点,所以在较高噪声比时实验效果介于二者之间. 上述算法共同之处是都留有大面积的噪声块而本文算法(HF)能有效消除噪声块,并较为完整地保留细节.如图2所示,HF算法在10%噪声浓度内处理效果略低于TSM而优于其余几种算法,但在20%到80%噪声浓度内,随着噪声浓度逐渐增大,HF相较于其他几种算法在信噪比等方面处理效果更好. 当浓度大于50%时,由于HF能够避免在检测和去噪过程中使用噪声点像素值,并且在迭代中有效地放大了同一方向上像素点的像素值之间的联系,从而在处理效果上将大幅度领先于以上算法. 随着噪声的浓度增加,处理效果会受到影响,但下降幅度相比其他算法要小很多.图1 四种算法的去噪效果对比图2 五种算法在Lena图像(50%噪声浓度)上的RPSN对比3 结语本文创新性地将经济学领域的Holt双参数指数平滑法改进并应用于图像处理,使之成为一种具有可行性的新型算法,并通过大量的实验验证了HF可以有效地去除噪声,能够较好地还原图像边缘,并保留原图像细节. 但是由于当像素值不具备参考价值时选择了用左侧像素取代目标像素,导致了输出图像一定程度上的模糊. 进一步研究的方向应为如何选取更具价值的参考点、取得更加灵活的平滑参数、改善左侧取代所带来的弊端等. 本算法还有许多值得开发与改进的空间.参考文献:【相关文献】[1] VIJAYKUMAR V R, SANTHANA G M,EBENEZER D. Fast switching based median-mean filter for high density salt and pepper noise removal[J]. AEUE - International Journal of Electronics and Communications,2014,68(12):1145-1155.[2] ANATHI V P, BALASUBRAMANIAM. A new image denoising method using interval-valued intuitionistic fuzzy sets for the removal of impulse noise[J]. Signal Processing,2016(10):121.[3] DAWOOD H, GUO P. Generalization of Impulse Noise Removal[J]. International Arab Journal of Information Technology, 2017,14(5):698-706.[4] SYAMALA J P, PARU R, PRADEEP K, et al. A fast novel algorithm for salt and pepper image noise cancellation using cardinal B-splines[J]. Signal, Image and Video Processing,2013,7(6):698-706.[5] ALI S A, ABBOOD C E A, KADHM C S A. Salt and pepper noise removal using resizable window and Gaussian estimation function[J]. International Journal of Electrical and Computer Engineering, 2016,6(5):2219-2224.[6] OSAMA S F, HANI M I. Adaptive switching weighted median filter framework for suppressing salt-and-pepper noise[J]. AEUE - International Journal of Electronics and Communications, 2016,70(8):1034-1040.[7] PITAS I, VENETSANOPOULOS A N.Nonlinear Digital Filters: Principles and Applications[M]. Boston: MA: Kluwer, 1990.[8] 张航,曹瞻.基于局部均值与方差的图像中值滤波方法[J].中南大学学报:自然科学版,2013,44(S2):381-384.[9] VIVEK S B, DIBYENDU G, ABUL H S. A new approach for high density saturated impulse noise removal using decision-based coupled window median filter[J]. Signal, Image and Video Processing,2014,8(1):71-84.[10] WEN X, DENG Z, XUE H. An improved median filtering image de-noising algorithm[J]. Revista Tecnica de la Facultad de Ingenieria Universidad del Zulia, 2016,39(5):293-298. [11] KO S J, LEE Y H. Center weighted median filters and their applications to image enhancement[J]. IEEE Trans.,1991,38: 984-993.[12] CHEN T, MA K K, CHEN L H.Tri-State Median Filter for Image Denoising[J].IEEE Transactions on Image Processing,1999,12(8):1834-1838.[13] 吴小明.Excel在霍尔特指数平滑法参数优选中的应用[J].无锡商业职业技术学院学报,2008,8(3):49-50.[14] CHARLES C.Holt frocasting seasonals and trends by exponentially weighted moving averages[J].International Journal of Forecasting,2004,20(1) :5-10.[15] 张新明,康强,程金凤,等.采用自适应四点窗中点滤波的高椒盐噪声滤除方法[J].计算机应用,2017, 37(3) : 832-838.。
一种非线性模糊综合评价模型
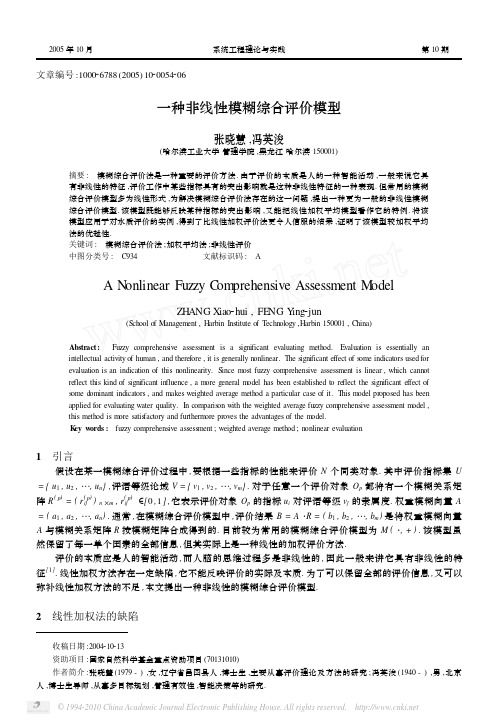
1 引言
假设在某一模糊综合评价过程中 , 要根据一些指标的性能来评价 N 个同类对象 . 其中评价指标集 U
= { u1 , u2 , …, u n} , 评语等级论域 V = { v1 , v2 , …, vm } . 对于任意一个评价对象 O p 都将有一个模糊关系矩
阵 R ( p) = ( r (ijp) )
A 与模糊关系矩阵 R 按模糊矩阵合成得到的 . 目前较为常用的模糊综合评价模型为 M ( ・ , + ) . 该模型虽
然保留了每一单个因素的全部信息 , 但其实际上是一种线性的加权评价方法 . 评价的本质应是人的智能活动 , 而人脑的思维过程多是非线性的 , 因此一般来讲它具有非线性的特 征
[1 ]
具体的说当被评对象某个指标值很高而其他指标值相对较低时实际情况下可以认为其是优秀的或不良的但应用加权平均法后由于权重影响的不足这个指标的突出影响就无法体现而使整体的评价结果与实际相悖
2005 年 10 月
系统工程理论与实践
第 10 期
文章编号 :100026788 ( 2005) 1020054206
一种非线性模糊综合评价模型
张晓慧 ,冯英浚
( 哈尔滨工业大学 管理学院 ,黑龙江 哈尔滨 150001)
摘要 : 模糊综合评价法是一种重要的评价方法 . 由于评价的本质是人的一种智能活动 , 一般来说它具 有非线性的特征 ,评价工作中某些指标具有的突出影响就是这种非线性特征的一种表现 . 但常用的模糊 综合评价模型多为线性形式 ,为解决模糊综合评价法存在的这一问题 ,提出一种更为一般的非线性模糊 综合评价模型 . 该模型既能够反映某种指标的突出影响 , 又能把线性加权平均模型看作它的特例 . 将该 模型应用于对水质评价的实例 ,得到了比线性加权评价法更令人信服的结果 ,证明了该模型较加权平均 法的优越性 . 关键词 : 模糊综合评价法 ; 加权平均法 ; 非线性评价 中图分类号 : C934 文献标识码 : A
- 1、下载文档前请自行甄别文档内容的完整性,平台不提供额外的编辑、内容补充、找答案等附加服务。
- 2、"仅部分预览"的文档,不可在线预览部分如存在完整性等问题,可反馈申请退款(可完整预览的文档不适用该条件!)。
- 3、如文档侵犯您的权益,请联系客服反馈,我们会尽快为您处理(人工客服工作时间:9:00-18:30)。
O.R.ApplicationsAn interval-parameter fuzzy nonlinear optimization model for stream water quality management under uncertaintyX.S.Qina,b,G.H.Huanga,b,*,G.M.Zeng c ,A.Chakma d ,Y.F.HuangeaSino-Canada Center of Energy and Environmental Research,North China Electric Power University,Beijing 102206,ChinabFaculty of Engineering,University of Regina,Regina,Saskatchewan,Canada S4S 0A2cDepartment of Environmental Science and Engineering,Hunan University,Changsha 410082,China dDepartment of Chemical Engineering,University of Waterloo,Waterloo,Ont.,Canada N2L 3G1eInstitute of River and Coastal Engineering,Tsinghua University,Beijing 100084,ChinaReceived 3December 2004;accepted 24March 2006Available online 30June 2006AbstractPlanning for water quality management systems is complicated by a variety of uncertainties and nonlinearities,where difficulties in formulating and solving the resulting inexact nonlinear optimization problems exist.With the purpose of tackling such difficulties,this paper presents the development of an interval-fuzzy nonlinear programming (IFNP)model for water quality management under uncertainty.Methods of interval and fuzzy programming were integrated within a general framework to address uncertainties in the left-and right-hand sides of the nonlinear constraints.Uncertainties in water quality,pollutant loading,and the system objective were reflected through the developed IFNP model.The method of piecewise linearization was developed for dealing with the nonlinearity of the objective function.A case study for water quality management planning in the Changsha section of the Xiangjiang River was then conducted for demon-strating applicability of the developed IFNP model.The results demonstrated that the accuracy of solutions through lin-earized method normally rises positively with the increase of linearization levels.It was also indicated that the proposed linearization method was effective in dealing with IFNP problems;uncertainties can be communicated into optimization process and generate reliable solutions for decision variables and objectives;the decision alternatives can be obtained by adjusting different combinations of the decision variables within their solution intervals.It also suggested that the linear-ized method should be used under detailed error analysis in tackling IFNP problems.Ó2006Elsevier B.V.All rights reserved.Keywords:Environment;Interval programming;Fuzzy programming;Nonlinear optimization;Uncertainty;Linear programming0377-2217/$-see front matter Ó2006Elsevier B.V.All rights reserved.doi:10.1016/j.ejor.2006.03.053*Corresponding author.Address:Faculty of Engineering,University of Regina,Regina,Saskatchewan,Canada S4S 0A2.Tel.:+13065854095;fax:+13065854855.E-mail address:huang@ (G.H.Huang).European Journal of Operational Research 180(2007)1331–13571332X.S.Qin et al./European Journal of Operational Research180(2007)1331–13571.IntroductionEffective planning for water quality management is important for facilitating sustainable socio-economic development in watershed systems.However,such a planning effort is complicated with a variety of uncertain-ties and nonlinearities.For example,dynamic interactions exist between pollutant loadings and receiving waters(Orlob,1983;Lamb and Hull,1985;Hoppe et al.,2004;Huang and Chang,2003),associated with a variety of uncertainties.Moreover,representation of system costs for water quality management involves a number of nonlinear functions for projecting environmental–economic interrelationships.These complexities lead to difficulties in formulating and solving the resulting nonlinear optimization problems.In the past decades,efforts were made in dealing with the uncertainties and nonlinearities in water quality management through interval,stochastic and fuzzy programs(Huang,1998;Huang and Loucks,2000;Huang et al.,2001;Huang et al.,2002).Interval nonlinear optimization methods present uncertainties of real-world systems as intervals.For instance,Zeng et al.(1994)proposed a linearization method to solve interval non-linear programming problems.Xia and Zhang(1993)introduced several methods for solving interval nonlin-ear programming problems based on an extended Kuhn–Tucker theorem,and applied them to water pollution control planning.More recently,Chen and Huang(2001)developed a series of solution algorithms for solving interval quadratic programs and applied them to environmental management under uncertainty.Stochastic programming is another measure to address uncertainties in nonlinear systems.The most com-monly used approach is Monte Carlo simulation(Smith,1994).Burn and Mcbean(1985)studied optimization modeling of water quality management in an uncertain environment through probabilistic analysis.Huang and Chen(1991)developed a stochastic nonlinear optimization method for water quality management,fol-lowed with environmental risk assessment based on the optimization results.In these models,the objective functions were exponential with several modeling parameters being uncertain and presented as probability density functions.By means of Monte Carlo simulation,the problems could be converted into large amounts of deterministic submodels and then solved by traditional nonlinear programming methods.Pereira and Pinto (1991)presented a method for solving multistage stochastic programming problems based on the approxima-tion of the expected-cost-to-go functions by piecewise linear programs obtained from the dual solutions at dif-ferent stages,and applied it to the planning of energy generation within a reservoir system.Another approach for optimization under uncertainty in water quality management is based on fuzzy set theory.It is a method that facilitates the analysis of systems with uncertainties being derived from vagueness or‘‘fuzziness’’rather than randomness alone.It is suitable for situations when the uncertainties cannot be expressed as probability density functions(PDFs),such that adoption of fuzzy membership functions becomes an attractive alternative(Huang et al.,1995).Previously,Huang et al.(1993,1995)proposed several fuzzy-optimization methods and applied them to environmental management problems.More recently,Guo et al.(1999)proposed a fuzzy multiobjective linear programming model and applied it to land-use planning for forestation and agricultural activities in the Erhai River Basin.Research for similar optimization problems under uncertainty and nonlinearity can also be found in other fields such as solid waste management,water resources management,and urban planning(Yeomans and Huang,2003).Taguchi et al.(2003)developed a genetic algorithm(GA)approach for solving nonlinear goal programming problems with interval coefficients.Gen et al.(1997)and Tang et al.(1998)presented several GA approaches to solve fuzzy nonlinear-objective programming problems.More recently,Chen and Huang (2001)developed a derived algorithm for solving interval quadratic program and applied it to environmental decision making under uncertainty.Wu(2000)developed a differentiated algorithm for tackling interval qua-dratic programming,and applied it to solid waste management.Yeomans et al.(2003)developed an evolution-ary algorithm for optimal planning of municipal solid waste management and applied it in the regional municipality of Hamilton–Wentworth.Generally,the above methods have advantages in their effectiveness in dealing with uncertainties.However, the stochastic methods are associated with difficulties in acquiring PDFs for a number of modeling parameters (Marr and Canale,1988;Guo et al.,2003).The GA-based nonlinear optimization methods are ineffective when the parameters are very uncertain;also,they are non-analytical approaches and have high computa-tional requirements(Qin and Zeng,2002;Zeng et al.,2004).Based on Huang et al.(2001),the interval pro-grams can deal with uncertainties that exist in left-hand side coefficients,but have difficulties when the model’sright-hand sides are highly uncertain;Huang et al.(1993)also indicated that fuzzy programming approach would be more applicable when right sides of the constraints have large intervals.The two methods have var-ied strengths and weaknesses,with a potential for compensating each other when they are integrated within a general framework.Therefore,the main objective of this research is to develop an interval-parameter fuzzy nonlinear programming(IFNP)model for water quality management under uncertainty and explore a linear-ization method for model solutions.This objective entails:(a)Integration of interval and fuzzy programming to address uncertainties in the left-and right-hand sidesof the nonlinear models.(b)Linearization of the nonlinear objective function through piecewise conversions.(c)Solution of the nonlinear models through IFLP solution method.(d)A case study for water quality management planning in the Changsha section of the Xiangjiang Riverfor demonstrating applicability of the proposed IFNP model and linearization method.(e)Comparison of the linearization method and a direct nonlinear method to assess effectiveness of the pro-posed methodology.2.Water quality simulationWater quality models were used extensively for supporting environmental management in river basins.Sev-eral water quality models were developed in the past decades,such as the Streeter–Phelps,O’Conner,Dobbins, and Thomas models(Rauch et al.,1998).In this study,the Streeter–Phelps model is used for supporting quan-tification of water quality constraints related to biological oxygen demand(BOD)and dissolved oxygen(DO) discharges as well as their concentrations in river waters(Streeter and Phelps,1925).Afirst-order degradation reaction of BOD can be written as:d Ld t¼ÀK d L;ð1Þwhere L is the ultimate BOD concentration,t is the meanflow time,and k d is thefirst-order deoxygenation rate constant.For dissolved oxygen(DO),a mass balance equation can be written as follows:d Od t¼Àk d Lþk aðO sÀOÞ;ð2Þwhere O is the DO concentration,O s is the saturated DO concentration,and k a is thefirst-order reaeration rate constant.The solutions of Eqs.(1)and(2)are(Thomann and Mueller,1987):L¼L0eÀk d t;ð3aÞO¼O sÀðO sÀO0ÞeÀk d tÀk d L0k aÀk dðeÀk d tÀeÀk a tÞ;ð3bÞwhere L0and O0are BOD and DO concentrations at the starting point of the river system,respectively.Segmentation is necessary since a number of wastewater discharge outlets scatter along the river,with tem-poral and spatial variations of their loadings.Water quality at each section is affected by various sources from the upper stream(Cheng and Cheng,1990).Fig.1shows a conceptual description of stream segmentation that is related to wastewater discharge and water utilization.According to Fig.1,the mass balance,flow continuity,flow rate,and BOD equilibrium can be written as follows:Q2i¼Q1iÀQ3iþQ i;ð4aÞQ1i¼Q2;iÀ1;ð4bÞL2i Q2i ¼L1iðQ1iÀQ3iÞþL i Q i:ð4cÞX.S.Qin et al./European Journal of Operational Research180(2007)1331–13571333From Eq.(3a),the BOD degradation process can be written as:L1i¼L2;iÀ1eÀk d;iÀ1t iÀ1:ð5ÞDefine a i¼eÀk d i t i.Substituting the corresponding item in Eq.(5)with a iÀ1,we have:L1i¼a iÀ1L2;iÀ1:ð6ÞFrom Eqs.(4c)and(6),we have:L2i¼L2;iÀ1a iÀ1ðQ1iÀQ3iÞQ2iþQiQ2iL i:ð7ÞDefine:a iÀ1¼a iÀ1ðQ1iÀQ3iÞQ2i;b i¼QiQ2i:A matrix expression can thus be used to replace Eq.(7):A~L2¼B~Lþ~g;ð8Þwhere A and B are n·n matrixes,and~g is an n-dimensional vector.From Eq.(8),we have: ~L2¼AÀ1B~LþAÀ1~g:ð9ÞEq.(9)defines the relationship between BOD input and output at each river section,where~L2is the simulated BOD level,and~L is the observed BOD discharge concentration.However,when Eq.(9)is applied in optimi-zation models,~L2generally represents as the desired BOD-discharge criteria,and~L is the variable that needs to be determined.The DO equilibrium can be written as follows:O2i Q2i¼O1iðQ1iÀQ3iÞþO i Q i:ð10ÞAccording to Eq.(3b),the DO level can be given by:O1i¼O2;iÀ1eÀk a;iÀ1t iÀ1Àk d;iÀ1L2;iÀ1k a;iÀ1Àk d;iÀ1ðeÀk d;iÀ1t iÀ1ÀeÀk a;iÀ1t iÀ1ÞþO sð1ÀeÀk a;iÀ1t iÀ1Þ:ð11ÞDefine c i¼eÀk a i t i;b i¼k d iða iÀc iÞk a iÀk d iand d i=O s(1Àc i).Then function(10)becomes:O2i¼Q1iÀQ3iQ2iðQ2;iÀ1c iÀ1ÀL2;iÀ1b iÀ1þd iÀ1ÞþQiQ2iO i:ð12Þ1334X.S.Qin et al./European Journal of Operational Research180(2007)1331–1357Definec iÀ1¼Q1iÀQ3iQ2ic iÀ1;d iÀ1¼Q1iÀQ3iQ2ib iÀ1and fiÀ1¼Q1iÀQ3iQ2id iÀ1:Then we have:O2i¼c i O2;iÀ1Àd iÀ1L2;iÀ1þf iÀ1þb i O i:ð13ÞA matrix function could be written as follows:C~O2¼ÀD~L2þB~Oþ~fþ~h:ð14ÞEq.(14)can be further converted into:~O2¼ÀCÀ1DAÀ1B~LþCÀ1B~OþCÀ1ð~fþ~hÞÀCÀ1DAÀ1~g:ð15ÞThus,Eqs.(9)and(15)can be used for predicting water quality at each river segment.3.Optimization of water quality management system3.1.Deterministic modeling formulationBased on the definition of b i,if the volumeflow of the wastewater is negligible compared with the volume flow of the river(i.e.Q i(Q2i such that b i!0),then Eq.(15)becomes:~O2¼ÀCÀ1DAÀ1B~LþCÀ1ð~fþ~hÞÀCÀ1DAÀ1~g:ð16ÞThus,the BOD and DO models can be simplified into:~L2¼U~Lþ~m;ð17aÞ~O2¼V~Lþ~n;ð17bÞwhere U and V are coefficient matrixes defined by Eqs.(9)and(16).The main objective of the water quality management is to minimize net operating cost of wastewater treatment under the constraints of water quality requirements and river conditions.The operational cost function for each wastewater treatment unit can be written as(Cheng and Cheng,1990):C¼k1Q k2þk3Q k2g k4;ð18Þwhere C is the treatment cost for a specific pollutant,Q is the wastewaterflow rate,g is the treatment effi-ciency,k1and k3are the cost-function coefficients,k2is the economy-of-scale index of the treatment cost, and k4is the scale index of treatment efficiency(k1,k3>0,0<k2<1,and k4>1).The operating cost functions for handling different types of wastewater generally imply the relevant eco-nomical consequences in terms of both scale and efficiency.They were normally expressed in various empirical formulations by researchers from different countries(Thomann,1972;Rinaldi et al.,1979;Lee and Wen, 1997).In China,the most widely used expression is written as Eq.(18).When the treatment efficiency is con-sidered as a constant,the equation becomes C=aQ k2,where a=K1+K3g K4.The equation of C=aQ k2can also be written in a differential style as d C/d Q=ak2Q k2À1.According to the results from a number of researchers,K2ranges from0.7to0.8(Fu and Cheng,1985;Cheng and Cheng,1990).Thus,the unit operating cost(d C/d Q)will decrease with the increase of the treatment scale(Q).Such a relationship between opera-tional cost and treatment scale is normally called economy-of-scale for wastewater treatment.This reveals X.S.Qin et al./European Journal of Operational Research180(2007)1331–13571335the economic advantages of large-scale wastewater treatment.When the scale of treatment(Q)is a constant, only the treatment efficiency becomes a variable.Since K4in Eq.(18)is always greater than1(Cheng and Cheng,1990;Qin and Zeng,2000),the treatment cost per unit of pollutant loading will increase with the treat-ment efficiency.Such a relationship is normally named economy-of-efficiency.The optimization model for water quality management can thus be established as follows: Min f¼X ni¼1C iðgÞ;ð19aÞs:t:U~Lþ~m6~L c;V~Lþ~n P~O c;ð19bÞ~L P0;g1 i 6gi6g2i;where C i(g i)is the wastewater treatment cost at segment i;g i is the treatment efficiency at segment i;U and V are the relational matrixes of BOD and DO;~L c and~O c are the environmental criteria of BOD and DO con-centrations,respectively;~m and~n are the constant vectors as delineated in(17);g1i and g2iare the technicalconstraints of treatment efficiencies.The relationship between the pollutant concentration in dischargedflow (L i)and the treatment efficiency(g i)can be given by:g i ¼1ÀL iL0i;ð20Þwhere L0iis the initial BOD concentration at segment i.3.2.Modeling formation under uncertaintyKothandaraman and Ewing(1969)proposed that both deoxygenation and reaeration rates in river systems have uncertain and dynamic characteristics.The uncertainties characteristics of k a and k d can be represented as intervals since their distributional information is often unavailable.Thus,Eqs.(3a)and(3b)become:LƼL0eÀkÆd t;ð21aÞOƼO sÀðO sÀO0ÞeÀkÆd tÀkÆdL0kaÀkdðeÀkÆd tÀeÀkÆa tÞ:ð21bÞTherefore,Eqs.(17a)and(17b)can be transformed into:~LÆ2¼UÆ~LÆþ~mÆ;ð22aÞ~OÆ2¼VÆ~LÆþ~nÆ:ð22bÞUncertainties of wastewater discharge rates can also be presented as intervals,leading to the following cost function as converted from Eq.(18):CƼk1QÆk2þk3QÆk2gÆk4:ð23ÞGenerally,pollutant concentrations would increase under low stream-flow rates.This occurs typically in win-ter for rivers in the central-south of China.This is due to the low dilution from the streamflow.Thus the low-estflow rate is applied in formulating the optimization model since it represents the most serious pollution conditions.Generally,since the data of the lower and upper bounds of water quality related parameters are easier to be estimated,uncertainties associated with water quality simulations are represented as intervals. The water quality criteria that present as the constraint right-hand sides are quantified as fuzzy sets due to its 1336X.S.Qin et al./European Journal of Operational Research180(2007)1331–1357effectiveness in avoiding or mitigating violation of model solutions.These uncertainty-manipulation settings result in an interval-parameter fuzzy nonlinear programming(IPFNP)model as follows:Min fƼX ni¼1CÆiðgÆÞ;ð24aÞs:t:UÆ~LÆP$~LcÀ~mÆ;ð24bÞVÆ~LÆP$~OcÀ~nÆ;ð24cÞ~LÆP0;ð24dÞg1 i 6gÆi6g2i;ð24eÞgÆi ¼1ÀLÆiL0i;ð24fÞwhere CÆi ðgÆiÞis the wastewater treatment cost at the segment i;the symbol P$represents that the right-hand-side of the constraint is treated as fuzzy-valued parameters.From the cost function as shown in Eq.(23),the objective function(24a)is mostly nonlinear.4.Solution method4.1.Interval-fuzzy linear programmingConsider an interval linear programming(ILP)problem as follows:Min fƼCÆXÆ;ð25aÞSubject to AÆXÆ6BÆ;ð25bÞXÆP0;where A±2{R±}m·n,B±2{R±}m·1,C±2{R±}1·n and X±2{R±}n·1(R±denotes a set of interval parameters).When the system’s goal and constraints are fuzzy,model(1)can be converted into an interval fuzzy linear programming(IFLP)problem,through incorporating the fuzzy-programming concept within the ILP frame-work(Huang et al.,1993,1995).The IFLP model can be formulated as follows(Huang et al.,1993): Max k;ð26aÞSubject to CÆXÆ6fþopt1ÀkÁ½fþopt1ÀfÀopt1;AÆXÆ6BÆÀkÁ½BþÀBÀ ;ð26bÞ06k61;XÆP0;where fÀopt1and fþopt1denote the least and most desirable system objectives,respectively;k is a control variablethat corresponds to the degree(membership grade)to which the model’s solution fulfills the fuzzy goal or con-straints.Specifically,flexibility in the constraints and fuzziness in the system objective,which are represented by fuzzy sets and denoted as‘fuzzy constraints’and a‘fuzzy goal’respectively,are expressed as a membership grade(k)corresponding to the degrees of satisfaction for the constraints and the objective.An interactive two-step solution algorithm was provided by Huang et al.(1993)for solving model Eqs. (26a)and(26b).In detail,thefirst submodel that corresponds to the desired lower-bound objective functionvalue can be formulated as follows(in water quality planning problems,the water quality criteria bÆi >0,andthe cost f±>0):X.S.Qin et al./European Journal of Operational Research180(2007)1331–13571337Max k;ð27aÞSubject toX k1j¼1cÀjxÀjþX nj¼k1þ1cÀjxþj6fþÀkðfþÀfÀÞ;X k1 j¼1j a ij jþSignðaþijÞxÀjþX nj¼k1þ1j a ij jÀSignðaÀijÞxþj6bþiÀkðbþiÀbÀiÞ;8ið27bÞ06k61;XÆjP0;8j;where j a ij j is the absolute value of a ij,and SignðaÆij Þis the sign of a ij.Thus,the solutions of xÀj optðj¼1;2;...;k1Þand xþj opt ðj¼k1þ1;k1þ2;...;nÞcan be obtained through submodel(27);fÀoptcan then be obtained from(25a).In the second step,the following submodel corresponding to the upper-bound objective function value can then be formulated:Max k;ð28aÞSubject toX k1j¼1cþjxþjþX nj¼k1þ1cþjxÀj6fþÀkðfþÀfÀÞ;X k1 j¼1j a ij jÀSignðaÀijÞxþjþX nj¼k1þ1j a ij jþSignðaþijÞxÀj6bþiÀkðbþiÀbÀiÞ;8ið28bÞ06k61;XÆjP0;8j;xþj P xÀj opt;j¼1;2;...;k1;xÀj 6xþj opt;j¼k1þ1;k2þ2;...;n:The solutions of xþj opt ðj¼1;2;...;k1Þ,xÀj optðj¼k1þ1;k1þ2;...;nÞand fþoptcan thus be obtained.Theresulting solution can be presented as xÆj opt ¼½xÀj opt;xþj opt,and fÆj opt¼½fÀj opt;fþj opt.4.2.Linearization of nonlinear cost functionThe cost function as shown in Eq.(18)is nonlinear(exponential).A linearization approach is proposed to solve such a problem.According to Cheng and Cheng(1990),cost function(18)could be simplified into: C¼aþb g k4;ð29Þwhere g is the treatment efficiency of BOD as defined in Eq.(20).The presentation of this equation is shown in pared with Eq.(18),we have a=K1Q k2and b=K3Q k2.When the scale of treatment(Q)is a con-stant,a and b become constant coefficients.In such a case,there will be no effect of scale economy.According to Cheng and Cheng(1990)and Fu and Cheng(1985)(1990)and Fu and Cheng(1985),the exponent index k4 in Eq.(29)is greater than1.Thus,Eq.(20)is always presented in a convex shape.The basic idea of the linearization is to cut the nonlinear function into several segments,and then use linear functions formulations to approximate them.The obtained linear models can thus be solved through the tra-ditional ILP method(Huang et al.,1993,1995).4.2.1.Piecewise conversion of the nonlinear function into multiple segmentsAssume that there exist t0segments within the interval of06g<1.The segmental values are g¼0;g 1;g2;...;gt0,and the relevant slopes are s1;s2;...;s t(Fig.2).In each segment,the linearized functions couldbe written as follows:1338X.S.Qin et al./European Journal of Operational Research180(2007)1331–1357C 1¼a þs 1ðg Àg 0Þ;g 06g <g 1;C 2¼a þs 1ðg 1Àg 0Þþs 2ðg Àg 1Þ;g 16g <g 2;...C t 0¼a þX i À1j ¼1s j ðg j Àg j À1Þþs t 0ðg Àg t 0À1Þ;g t 0À16g <g t 0:4.2.2.Minimization of linearization errorsThe sum of squared errors at all segments should be minimized (Fig.2):Min Z g i g i À1d E ¼Z g i g i À1a þX i À1j ¼1s j ðg j Àg j À1Þþs i ðg Àg i À1ÞÀða þb g k 4Þ"#2d g ;ð30ÞwhereR g ig i À1d E represents the integration approximation of errors in segmentsg i À1to g i .4.2.3.Slope at each segment To minimize R g i g i À1d E ,let d R g ig i À1d E =d s i ¼0.Thus,we can derive the slope of each linearized function as follows:s i ¼b 4g k 4þ2i Àg k 4þ2i À1ÀÁÀb g i À14g k 4þ1i Àg k 4þ1i À1ÀÁÀðg i Àg i À1Þ2P i À1j ¼1s j ðg j Àg j À1Þ13ðg i Àg i À1Þ:ð31Þ4.2.4.Formulation of piecewise linear objective functionThrough the linearization effort,Eq.(29)becomes:C ¼a þX t 0j ¼1s j g j :ð32ÞThus,cost function (19a)could be converted into:Min f ¼X n i ¼1a i þXt 0j ¼1s ij g ij "#;ð33Þwhere f is the total cost,n is the number of polluting sources,and t 0is the number of linearizedsegments.X.S.Qin et al./European Journal of Operational Research 180(2007)1331–135713394.3.Solution through linearizationBased on Zeng et al.(1994),the inexact water quality management problems can be generalized into:Min fƼX nj¼1½pÆjþcÆjðyÆjÞk j ;ð34aÞs:t:AÆi YÆ6$B;AÆi2AÆ;i¼1;2;...;m;ð34bÞwhere y j is the treatment efficiency,y i2Y.Suppose that the defined range of y j is cut into t0segments.Then, we have additional technical constraints as follows:yðtÞj6yjt 6yðtþ1Þj;t¼1;2;...;t0:ð35ÞThrough the linearization effort,we have:pÆj þcÆjðxÆjÞk j¼pÆjþX t0t¼1sÆjtðxÆjtÀxðtÞjÞ:ð36ÞBased on Section4.2,we have:s jt¼c jÁu jtðyð0Þj;yð1Þj;...;yðtÞjÞ¼c jÁu jtðÁÞ;ð37Þwhere u jt(Æ)is defined in Eq.(31).Therefore,we have:pÆj þcÆjðxÆjÞk j¼pÆjþcÆjX t0t¼1u jtðÁÞðxÆjtÀxðtÞjÞ:ð38ÞBecause the cost function is monotonically increasing(Fig.2),we have:ðyÆj Þk j¼X t0t¼1u jtðÁÞðyÆjtÀyðtÞjÞP0;ð39Þwhere u jt(Æ)>0when g0P0.The u jt(Æ)is also a monotonically increasing function.Based on the definition of yÆj,we then have:yÆj ¼X t0t¼1ðyÆjtÀyðtÞjÞ:ð40ÞFor the sake of simplification,define zÆjt ¼yÆjtÀyðtÞj within the following scope:06zÆjt 6yðtþ1ÞjÀyðtÞj;t¼1;2;...;t0:ð41ÞThus,model(34a)can be written as:Min fƼX nj¼1pÆjþcÆjX t0t¼1u jtðÁÞzÆjt"#;ð42Þs:t:X nj¼1aÆijÁX t0t¼1zÆjt6$b i;i¼1;2;...;m;ð43Þwhere zÆjt P0and u jt(Æ)P0.This is a conventional IFLP problem that can be solved through the followingtwo submodels(Huang et al.,1993):Max kð44aÞs:t:X nj¼1pþjþcþjX t0t¼1ujtðÁÞzÀjt"#þkðfþÀfÀÞ6fþ;ð44bÞ1340X.S.Qin et al./European Journal of Operational Research180(2007)1331–1357。