Improved Gene Selection for Classification of Microarrays
蛋白质修饰位点预测

蛋白质修饰位点预测
蛋白质修饰位点预测是生物信息学领域的一个重要研究方向。
蛋白质修饰是一种在蛋白质翻译后发生的化学变化,对蛋白质的功能和活性产生重要影响。
目前,许多生物信息学方法已经被开发用于预测蛋白质修饰位点,主要包括以下几种:
1. 基于机器学习的方法:这类方法通过训练一个分类器(如支持向量机(SVM)、神经网络等)来预测蛋白质修饰位点。
这类方法通常需要大量的已知修饰位点和非修饰位点的蛋白质序列作为训练数据。
例如,研究人员针对水稻蛋白质磷酸化位点开发了一种基于SVM的预测工具[1]。
2. 基于氨基酸序列特征的方法:这类方法通过分析蛋白质序列中的氨基酸特征(如氨基酸频率、组成等)来预测修饰位点。
这类方法不需要依赖蛋白质结构信息,仅通过序列信息进行预测。
例如,研究人员利用氨基酸频率计算方法来进行特征提取,并结合SVM算法构建了一种针对水稻蛋白质磷酸化位点的预测工具[2]。
3. 基于结构的方法:这类方法通过分析蛋白质三维结构来预测修饰位点。
由于蛋白质结构与功能密切相关,这类方法具有较高的预测准确性。
然而,结构信息通常不易获取,且计算成本较高。
4. 集成学习方法:这类方法将多个预测模型进行集成,以提高预测准确性。
例如,研究人员将多个基于机器学习的预测模型进行集成,构建了一种针对蛋白质翻译后修饰位点的预测工具[3]。
总之,蛋白质修饰位点预测是一个具有挑战性的课题。
随着生物信息学技术的发展,未来可能会出现更多高效、准确的预测方法。
同时,蛋白质修饰位点预测在生物学研究中的应用也将越来越广泛,有助于揭示蛋白质功能和调控机制。
临床检验中的分子诊断技术进展考核试卷
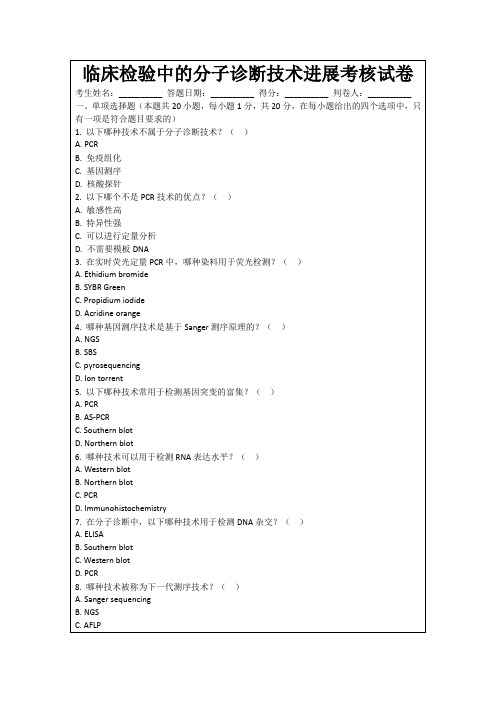
C. Western blot
D. Southern blot
11. 哪种技术可以用于检测单个细胞中的基因表达?( )
A. RNA-Seq
B. Single-cell PCR
C. qPCR
D. Northern blot
12. 以下哪种技术不是基于DNA芯片的检测方法?( )
A. Microarray
B. SNP array
C. CGH array
D. Western blot
13. 在分子诊断中,以下哪种技术用于检测基因重排?( )
A. PCR
B. FISH
C. Southern blot
D. Northern blot
14. 哪种技术可用于检测病原微生物的快速诊断?( )
D. PCR
8. 哪种技术被称为下一代测序技术?( )
A. Sanger sequencing
B. NGS
C. AFLP
D. RFLP
9. 以下哪个不是NGS技术的优点?( )
A. 高通量
B. 成本低
C. 准确性高
D. 速度快
10. 在分子诊断中,以下哪种技术用于检测DNA甲基化?( )
A. PCR
C. Western blot
D. Northern blot
19. 哪种技术可以用于检测miRNA的表达?( )
A. PCR
B. Northern blot
C. RNA-Seq
D. Western blot
20. 在分子诊断中,以下哪种技术用于检测病毒载量?( )
A. PCR
B. ELISA
C. Western blot
二代测序在临床决策的影响
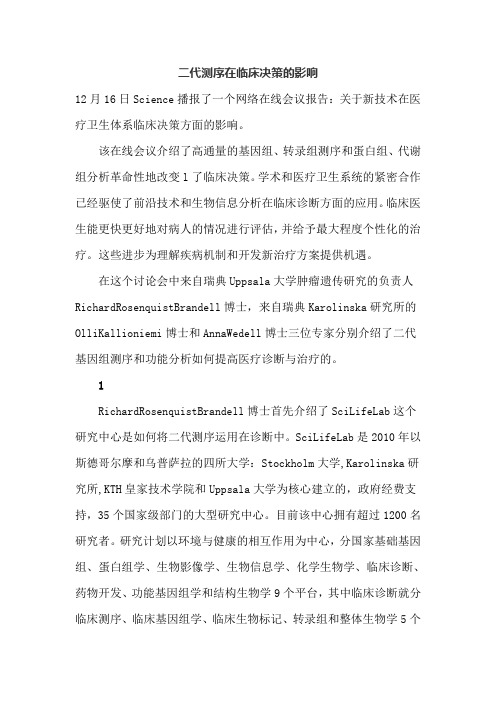
二代测序在临床决策的影响12月16日Science播报了一个网络在线会议报告:关于新技术在医疗卫生体系临床决策方面的影响。
该在线会议介绍了高通量的基因组、转录组测序和蛋白组、代谢组分析革命性地改变l了临床决策。
学术和医疗卫生系统的紧密合作已经驱使了前沿技术和生物信息分析在临床诊断方面的应用。
临床医生能更快更好地对病人的情况进行评估,并给予最大程度个性化的治疗。
这些进步为理解疾病机制和开发新治疗方案提供机遇。
在这个讨论会中来自瑞典Uppsala大学肿瘤遗传研究的负责人RichardRosenquistBrandell博士,来自瑞典Karolinska研究所的OlliKallioniemi博士和AnnaWedell博士三位专家分别介绍了二代基因组测序和功能分析如何提高医疗诊断与治疗的。
1RichardRosenquistBrandell博士首先介绍了SciLifeLab这个研究中心是如何将二代测序运用在诊断中。
SciLifeLab是2010年以斯德哥尔摩和乌普萨拉的四所大学:Stockholm大学,Karolinska研究所,KTH皇家技术学院和Uppsala大学为核心建立的,政府经费支持,35个国家级部门的大型研究中心。
目前该中心拥有超过1200名研究者。
研究计划以环境与健康的相互作用为中心,分国家基础基因组、蛋白组学、生物影像学、生物信息学、化学生物学、临床诊断、药物开发、功能基因组学和结构生物学9个平台,其中临床诊断就分临床测序、临床基因组学、临床生物标记、转录组和整体生物学5个部门。
临床测序中包括遗传学家、生物信息人员和医生,为提供“从实验室到病床边”的服务,为临床评估传递检测到的所有差异。
整合了大学、研究中心、医院在分子病理、临床遗传学方面不同平台的力量来做出决策是项目成功的关键。
主要分析目标是固体肿瘤,白血病和遗传性疾病。
癌症基因的临床测序主要是针对肺癌、结肠癌、黑色素瘤等多种癌症,运用靶向捕获技术对15-32个基因、50-250bp长度片段的诊断相关与治疗反应的突变进行检测。
genecards筛选score标准

一、简介Genecards是一个用于基因和蛋白质信息的集成数据库,提供了基因、蛋白质、药物和疾病等相关信息。
在Genecards中,基因的筛选score是一个重要的标准,用于评估基因的相关性和重要性,本文将对Genecards筛选score标准进行分析和总结。
二、Genecards筛选score的定义1. Genecards筛选score是指Genecards数据库根据一系列模型和算法对基因进行综合评估,得出的一个分数。
2. 这个分数是基于多种数据源和文献信息,包括基因表达水平、突变情况、蛋白质互作关系、药物靶点信息等。
3. Genecards筛选score越高,表示该基因在生物学功能和临床疾病中的重要性和相关性越高。
三、Genecards筛选score的应用1. 在基因功能研究中,研究人员可以利用Genecards筛选score来确定基因的重要性,优先选择研究重要性较高的基因。
2. 在药物靶点预测中,Genecards筛选score可以帮助研究人员筛选出潜在的药物靶点,加速药物研发过程。
3. 在临床转化研究中,基于Genecards筛选score的基因筛选可以帮助医生和研究人员发现与疾病相关的潜在生物标志物。
四、Genecards筛选score的影响因素1. 数据来源:Genecards整合了来自多个公共数据库和文献,对于数据覆盖范围和质量的要求较高。
2. 算法模型:Genecards筛选score的计算依赖于复杂的算法模型,不同模型的选择和权重设置会影响最终的得分结果。
3. 参数调整:Genecards对于不同基因类别和研究领域可能会进行参数调整,以保证评估结果的客观性和准确性。
五、Genecards筛选score的局限性1. 数据偏差:由于数据来源的限制,Genecards筛选score可能受到数据偏差的影响,特定基因的得分可能会受到一些偏差。
2. 算法局限:基于算法模型的限制,Genecards筛选score可能无法完全反映基因的所有相关信息和重要性。
基因修饰菌株的筛选和优化
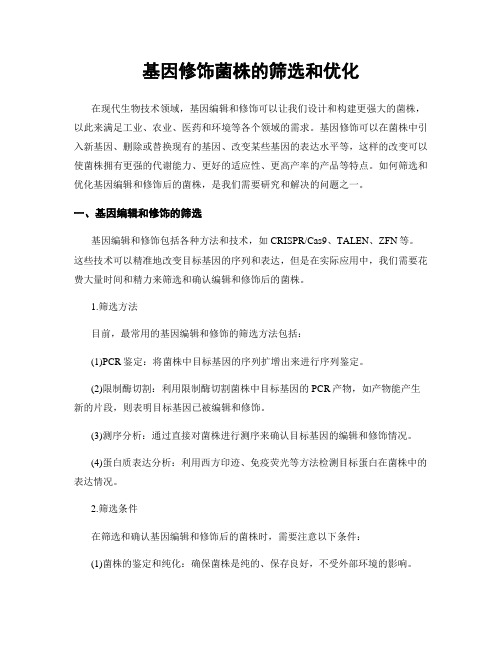
基因修饰菌株的筛选和优化在现代生物技术领域,基因编辑和修饰可以让我们设计和构建更强大的菌株,以此来满足工业、农业、医药和环境等各个领域的需求。
基因修饰可以在菌株中引入新基因、删除或替换现有的基因、改变某些基因的表达水平等,这样的改变可以使菌株拥有更强的代谢能力、更好的适应性、更高产率的产品等特点。
如何筛选和优化基因编辑和修饰后的菌株,是我们需要研究和解决的问题之一。
一、基因编辑和修饰的筛选基因编辑和修饰包括各种方法和技术,如CRISPR/Cas9、TALEN、ZFN等。
这些技术可以精准地改变目标基因的序列和表达,但是在实际应用中,我们需要花费大量时间和精力来筛选和确认编辑和修饰后的菌株。
1.筛选方法目前,最常用的基因编辑和修饰的筛选方法包括:(1)PCR鉴定:将菌株中目标基因的序列扩增出来进行序列鉴定。
(2)限制酶切割:利用限制酶切割菌株中目标基因的PCR产物,如产物能产生新的片段,则表明目标基因已被编辑和修饰。
(3)测序分析:通过直接对菌株进行测序来确认目标基因的编辑和修饰情况。
(4)蛋白质表达分析:利用西方印迹、免疫荧光等方法检测目标蛋白在菌株中的表达情况。
2.筛选条件在筛选和确认基因编辑和修饰后的菌株时,需要注意以下条件:(1)菌株的鉴定和纯化:确保菌株是纯的、保存良好,不受外部环境的影响。
(2)优化PCR扩增条件:优化PCR扩增的温度、时长和引物浓度等条件,确保PCR扩增的正确性和灵敏度。
(3)选择适当的限制酶:根据目标基因的序列,选择适当的限制酶,保证酶切产物的剪切正确性。
(4)测序单元长度:在进行测序时需要选择适当的单元长度,长单元长度可以提高测序的准确性和信噪比。
二、基因编辑和修饰的优化基因编辑和修饰后的菌株虽然拥有了更强的代谢能力和适应性,但是还存在一些问题,如产率不高、产品质量不稳定等。
因此,我们需要对基因编辑和修饰后的菌株进行优化,以便更好地满足应用需求。
1.基因表达优化基因表达的优化是指通过调整目标基因的表达水平,来获得更好的产率和产品质量。
自适应修改权重参数的果蝇优化算法
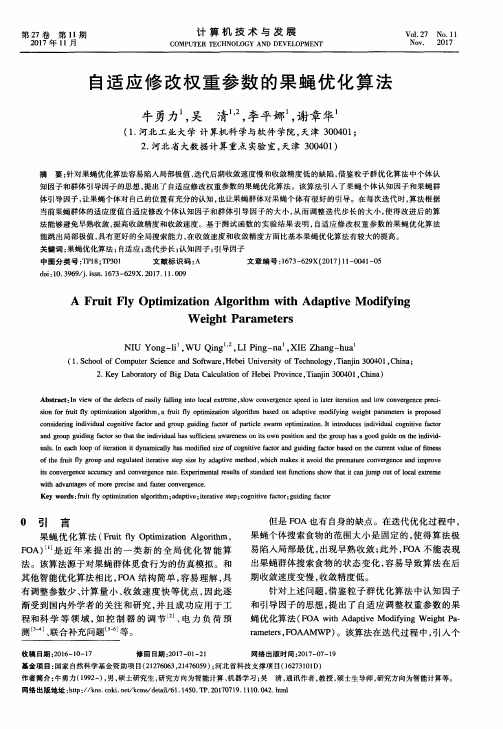
( 1 . 河北 工业 大学 计算 机科 学与 软件 学 院 , 天津 3 0 0 4 0 1 ; 2 . 河北省 大数 据计 算重 点 实验 室 , 天津 3 0 0 4 0 1 )
摘 要: 针 对 果蝇优 化算 法容 易陷入 局部 极值 、 迭代 后期 收敛 速度慢 和 收敛精 度低 的缺 陷 , 借 鉴粒 子群 优化 算 法 中个体 认
法 能够 避免 早熟 收敛 , 提高 收敛精 度和 收敛 速度 。基 于测试 函数 的 实验 结 果表 明 , 自适应 修 改权 重 参 数 的果 蝇优 化 算法
能跳出局部极值 , 具有更好的全局搜索能力, 在收敛速度和收敛精度方面比基本果蝇优化算法有较大的提高。
关键 词 : 果 蝇优 化算法 ; 自适 应 ; 迭代步 长 ; 认 知 因子 ; 引导 因子
第2 7卷 第 1 1 期 2 0 1 7发 展
C 0MPU TER I ECHNOL OGY AND DEVEL OPME NT
Vo 1 . 27 No .1 1 NO V. 2 Ol 7
自适应 修 改权 重 参 数 的 果蝇 优 化 算 法
Abs t r a c t : I n v i e w of t h e d e f e c t s o f e a s i l y f a l l i n g i n t o l o c a l e x t r e me , s l o w c o n v e r g e n c e s p e e d i n l a t e r i t e r a io t n a n d l o w c o n v e r g e n c e p r e c i - s i o n f o r f r u i t ly f o p t i mi z a i t o n a l g o r i hm , t a f r ui t ly f o p t i mi z a t i o n a l g o r i t h m b a s e d o n a d a p t i v e mo d i f y i n g we i g h t p a r a me t e r s i s p op r o s e d c o n s i d e r i n g i n d i v i d u a l c o g n i i t v e f a c t o r nd a g r o u p g u i in d g f a c t o r o f p a r t i c l e s wa r l T l o p t i mi z a i t o n . I t i n t r o d u c e s i n iv d i d u l a c o g n i iv t e f a c t o r nd a g r o u p g u i d i n gf a c t o r S Ot ha t h ei t n iv d i d u lh a a s s u ic f i e n t a wa r e n e s s o ni t s o wnpo s i t i o na n dt h e g r o u p h a s a g o o d g u i d e o nt h ei n iv d i d — u ls a . I n e ac h l o o p o f i t e r a i t o n i t d y n a mi c ll a y h a s mo di ie f d s i z e o f c o g n i iv t e f a c t o r nd a g u i d i n g f a c t o r b a s e d o n t h e c u r r e n t v lu a e o f it f n e s s o f he t f r u i t ly f g r o u p a n d r e g u l a t e d i t e r a i t v e s t e p s i z e b y a d a p t i v e me t h d , o wh i c h ma k e s i t a v o i d he t p r e ma t u r e c o n v e r g e n c e nd a i mp r o v e
《2024年基于机器学习挖掘益生菌基因组分子标记及构建可视化筛选预测平台》范文

《基于机器学习挖掘益生菌基因组分子标记及构建可视化筛选预测平台》篇一一、引言随着现代生物技术的飞速发展,益生菌作为一种具有重要生理功能的微生物,在人类健康领域的应用越来越广泛。
为了更好地研究和开发益生菌,对其基因组分子标记的挖掘和筛选显得尤为重要。
本文旨在介绍一种基于机器学习的方法,挖掘益生菌基因组分子标记,并构建可视化筛选预测平台,以期为益生菌的研究和开发提供新的思路和方法。
二、益生菌基因组分子标记的挖掘2.1 数据收集与预处理首先,我们需要收集大量益生菌的基因组数据,包括其基因序列、表达水平、功能注释等信息。
然后,对这些数据进行预处理,包括数据清洗、质量控制、标准化等步骤,以保证数据的可靠性和准确性。
2.2 特征提取与选择在预处理后的数据基础上,我们利用机器学习算法提取出与益生菌功能相关的特征,如基因序列中的保守区域、表达水平的差异等。
同时,通过特征选择算法,筛选出对预测益生菌功能具有重要影响的特征。
2.3 机器学习模型的构建与优化我们采用监督学习的方法,构建机器学习模型。
模型包括分类、聚类、回归等多种类型,根据具体需求选择合适的模型。
在模型构建过程中,我们通过交叉验证、参数调优等方法,优化模型的性能,提高其预测准确率。
三、可视化筛选预测平台的构建3.1 平台架构设计可视化筛选预测平台采用模块化设计,包括数据管理模块、特征提取与选择模块、机器学习模型模块、可视化展示模块等。
各个模块之间通过接口进行数据传输和交互。
3.2 数据管理与交互平台提供数据上传、下载、查询、删除等功能,方便用户管理和使用数据。
同时,平台支持用户自定义特征、模型和参数,以满足不同研究需求。
3.3 可视化展示平台采用图表、热图、散点图等多种方式,将复杂的数据分析结果直观地展示给用户。
用户可以通过鼠标操作,轻松地查看和分析数据,提高工作效率。
四、平台应用与效果评估4.1 平台应用我们利用构建的可视化筛选预测平台,对大量益生菌进行筛选和预测。
1种用于检测抑制基因功能的酵母株系的建立(英文)
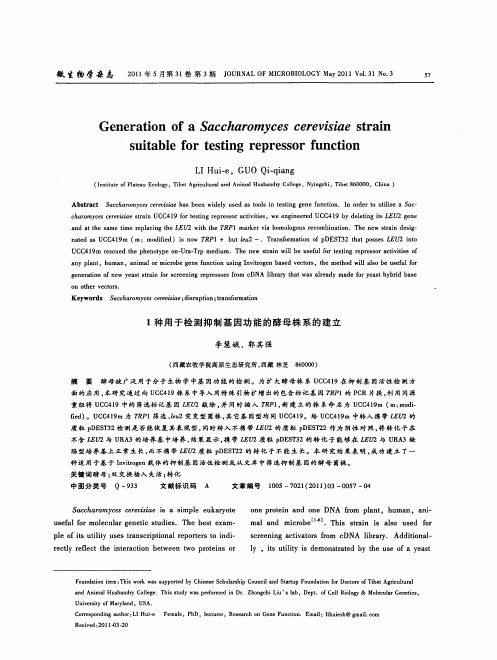
L u— ,G O Q -i g I i H e U i a qn
( n tueo l eu E ooy T b t giutrl n nma Hub n r o ee y g h ,Tb t 6 0 0,C ia) I s tt fPa a clg , ie A r l a a d A i l s a dy C l g ,N i c i ie 0 0 i t c u l n 8 hn
1种 用 于检 测 抑 制 基 因功 能 的 酵 母 株 系 的建 立
李 慧娥 ,郭其 强
( 藏农牧学院高原生态研究所, 藏 林芝 西 西 800 6 00)
摘
要
酵 母 被 广 泛 用 于分 子 生 物 学 中基 因功 能 的 检 测 。 为 扩 大 酵 母 株 系 U C 1 C 4 9在 抑 制 基 因活 性 检 测 方
A bsr c S c a o c sc rvsa a e n wie y u e s tos i e tn e u c in. I r e o u iie a S c ta t ac h r my e ee ii e h s b e d l s d a o l n t si g g ne f n to n o d rt tlz a — c a o c sc rvsa tan UCC41 o esi g r p e s ra tv te h r my e ee ii e sr i 9 frt tn e r s o ciiis,we e ie r d UCC41 ltn t EU2 g n ngn e e 9 bydeei g isL ee a tte s me tme r p a i g t e L nd a h a i e l cn h EU2 wih t 豫 P1 t he ma k rva ho lg u e o i ai n.Th e sr i e i— r e i moo o s r c mb n to e n w tan d sg
- 1、下载文档前请自行甄别文档内容的完整性,平台不提供额外的编辑、内容补充、找答案等附加服务。
- 2、"仅部分预览"的文档,不可在线预览部分如存在完整性等问题,可反馈申请退款(可完整预览的文档不适用该条件!)。
- 3、如文档侵犯您的权益,请联系客服反馈,我们会尽快为您处理(人工客服工作时间:9:00-18:30)。
IMPROVED GENE SELECTION FOR CLASSIFICATION OFMICROARRAYSJ. JAEGER*, R. SENGUPTA*†, W.L. RUZZO*†*Department of Computer Science & EngineeringUniversity of Washington114 Sieg Hall, Box 352350Seattle, WA 98195, USA†Department of Genome SciencesUniversity of Washington1705 NE Pacific St, Box 357730Seattle, WA 98195, USAIn this paper we derive a method for evaluating and improving techniques for selecting informative genes from microarray data. Genes of interest are typically selected by ranking genes according to a test-statistic and then choosing the top k genes. A problem with this approach is that many of these genes are highly correlated. For classification purposes it would be ideal to have distinct but still highly informative genes. We propose three different pre-filter methods — two based on clustering and one based on correlation — to retrieve groups of similar genes. For these groups we apply a test-statistic to finally select genes of interest. We show that this filtered set of genes can be used to significantly improve existing classifiers.1IntroductionEven though the human genome sequencing project is almost finished the analysis has just begun. Besides sequence information, microarrays are constantly delivering large amounts of data about the inner life of a cell. The new challenge is now to evaluate these gigantic data streams and extract useful information.Many genes are strongly regulated and only transcribed at certain times, in certain environmental conditions, and in certain cell types. Microarrays simultaneously measure the mRNA expression level of thousands of genes in a cell mixture. By comparing the expression profiles of different tissue types we might find the genes that best explain a perturbation or might even help clarify how cancer is developing.Given a series of microarray experiments for a specific tissue under different conditions we want to find the genes most likely differentially expressed under these conditions. In other words, we want to find the genes that best explain the effects of these conditions. This task is also called feature selection, a commonly addressed problem in machine learning, where one has class-labeled data and wants to figure out which features best discriminate among the classes. If the genes are the featuresdescribing the cell, the problem is to select the features that have the biggest impact on describing the results and to drop the features with little or no effect. These features can then be used to classify unknown data. Noisy or irrelevant attributes make the classification task more complicated, as they can contain random correlation. Therefore we want to filter out these features.Typically, informative genes are selected according to a test statistic or p-value rank according to a statistical test such as the t-test. The problem here is that we might end up with many highly correlated genes. Besides being an additional computational burden, it also can skew the results and lead to misclassifications. Additionally, if there is a limit on the number of genes to choose we might not be able to include all informative genes. Our approach is to first find similar genes, group them and then select informative genes from these groups to avoid redundancy.Besides t-like-statistics, there are many different techniques applicable. There are non-parametric tests like TNoM1 (which calculates a minimal error decision boundary and counts the number of misclassifications done with this boundary) or Wilcoxon rank sum/Mann-Whitney2 (which test statistic is identical3 to the Park4 score). It creates a minimal decision boundary too, but incorporates the distance from the boundary into the score. T-like statistics such as Fisher5 and Golub6 put different weights in the variance and number of samples. The Mutual Information score results from entropy and information theory, and the B-score7 comes from Bayesian decision theory. Vapnik8 describes an interesting method to optimize feature selection while generating support vector boundaries for SVMs.In this paper we will compare classification done with five different test statistics: Fisher,5 Golub,6 Wilcoxon,2 TNoM,1 and t-test2 on three different publicly available datasets, Golub,6 Notterman16 and Alon9. We will propose two algorithms based on clustering and one based on correlated groups to find similar genes.We then show that these prefiltering methods yield consistently better classification performance than standard methods using similar numbers of genes.The rest of the paper is organized as follows: Section 2 will review important methods needed for our proposed approach. Section 2.1 will introduce Microarrays. Section 2.2 describes a method for evaluating different feature selection sets using support vector machines and a technique called leave-one-out cross-validation. In section 2.3 we will review clustering techniques used in this paper. In section 2.4 we will discuss how reducing redundancy in a dataset can help with the final classification process and elucidate why redundancy can cause problems for classification tasks. In section 2.5 we propose new methods to select genes from clusters using correlation, clustering and statistical information about the genes. In section 3 we will present results using our proposed approach on three publicly available data sets. Section 4 contains conclusions and future research directions.2Methods and Approach2.1MicroarraysA typical microarray holds spots representing several thousand to several tens of thousands of genes or ESTs (expressed sequence tags). After hybridization the microarray will be scanned and converted into numerical data. Finally the data should be normalized. The purpose of this step is to counter systematic variation (e.g. difference in labeling efficiency for different dyes, compensation for signal spill over from neighboring spots10) and to allow a comparison between different microarrays11. The data we work with is already background-corrected and normalized, and we do not address these problems in this paper.2.2Validation, ClassificationAs we are proposing new gene selection schemes we want to measure their performance and allow a comparison. All schemes provide us with a set of informative genes that will be used for future classification. Assume we have n samples. Leave-one-out cross-validation (LOOCV) is a technique where the classifier is successively learned on n-1 samples and tested on the remaining one. This is repeated n times so that every sample was left out once. To build a classifier for the n-1 samples, we extract the most revealing genes for these samples, and use a machine learner. With this classifier we try to classify the remaining (left out) sample. Repeating this procedure n times gives us n classifiers in the end. Our error score is the number of mispredictions. We use support vector machines (SVMs12) as the classification method as these are very robust with sparse and noisy data.2.3Support Vector MachinesSupports Vector machines (SVMs) expect a training data set with positive and negative examples as input (i.e., a binary labeled training data set). Then they create a decision boundary (the maximal-margin separating hyperplane) between the two groups and select the most relevant examples involved in the decision process (the so-called support vectors). The construction of the hyperplane is always possible as long as the data is linearly separable. If this is not the case, SVMs can use ‘kernels’, which provide a nonlinear mapping into a higher dimensional feature space. If a separating hyperplane in this feature space is found, it can correspond to a nonlinear decision boundary in the input space. If there is noise or inconsistent data a perfectly separating hyperplane may not exist. Soft-margin SVMs12 attempt to separate the training set with a minimal number of errors. In this paper we used Bill Noble’s SVM implementation 1.3 beta now called gist13.2.4ClusteringCluster analysis is a technique for automatically grouping and finding structures in a dataset. Clustering methods partition the dataset into clusters, where similar data are assigned to the same cluster whereas dissimilar data should belong to different clusters. Fuzzy clustering14 deals with the problem that there is often no sharp boundary between clusters in real applications. Instead of assigning an element to one specific cluster there is a membership probability for each cluster. In doing so an element can be a member of several clusters. Fuzzy clustering can be seen as a generalization of k-means15 clustering. We used the FCMeans Clustering MATLAB Toolbox V2-0.2.5Reducing RedundancyNow that we have reviewed all the methods we need, we will return to the problem of feature selection. Table 1 shows a list of 7 genes from Notterman’s Adenoma16 dataset sorted by increasing p-value. For gene M18000 the expression value is generally higher in Adenoma than in Normals with the exception of Adenoma 1 and Normal 2. Looking at X62691 the same is true. Both genes have a very low p-value and would be pulled out by conventional methods, which focus on genes with the lowest p-values. Biologists are often interested in a small set of genes (for financial, personal workload or experimental reasons) that describes the perturbation as well as possible. Therefore we are limited in the number of genes to extract and e.g. assuming we could only extract 2 genes we would pull out the first two genes as they have the lowest p-value. However we would not get much additional information using the second gene as it shows the same overall pattern. It would be better to include a gene that provides us with extra information.Table 1:Expression values for 7 selected genes of Adenoma and normal tissues, sorted by p-value.Adenoma Normal AccessionNumber12341234t-test p-valueM18000705.411227.27959.35951.56359.83711.08485.33431.190.014 X62691387.91577.57578.45546.54227.26436.65306.94239.330.016 M8296291.8516.2712.6161.62187.4476.90181.38186.530.017 U374260.477.05 6.30 3.40-3.88 1.58-2.99-2.910.018 HG2564 2.330.54 1.58 3.82-2.91-2.11 1.00-2.910.019 Z5085335.4326.0351.4941.2227.6815.8012.4615.990.022 M32373-48.02-28.20-64.62-56.95-15.05-16.86-7.97-34.880.022Table 2: Correlation between Adenoma genes from table 1M18000X62691M82962U37426HG2564Z50853M32373 M18000 1.000X626910.961 1.000M82962-0.944-0.971 1.000U374260.9730.975-0.983 1.000HG25640.5920.653-0.5530.529 1.000Z508530.5140.616-0.6330.5970.614 1.000M32373-0.509-0.5900.602-0.580-0.619-0.874 1.000 Looking at the correlation values in table 2 we can see that the first four genes have an absolute correlation greater than 0.94. Not surprisingly highly correlated genes show the same misclassification pattern and in fact we find that the first four genes also have the same pattern of consistent outliers in Adenoma 1 and Normal 2. In order to increase the classification performance we propose to use more uncorrelated genes instead of just the top genes. We expect the phenomenon illustrated by this example to be a general one. By just using the k best ranking genes according to a test-statistic we would select highly correlated genes. Correlation can be a hint that the two genes belong to the same pathway, are coexpressed or are coming from the same chromosome. In general we expect high correlation to have a meaningful biological explanation. If, e.g., genes A and B are in the same pathway it could be that they have similar regulation and therefore similar expression profiles. If gene A has a good test score it is highly likely that gene B will, as well. Hence a typical feature selection scheme is likely to include both genes in a classifier, yet the pair of genes provides little additional information than either gene alone. Of course we could just select more genes in order to capture all relevant genes. But not only would more genes involve higher computational complexity for classification but it also can skew the result if we have a lot more genes from one pathway. Furthermore if there are several pathways involved in the perturbation but one pathway has the main influence, we will probably select all genes from this pathway. If we then have a limit for the number of genes we might end up with genes only from this pathway. If many genes are highly correlated we could describe this pathway with fewer genes and reach the same precision. Additionally, we could replace correlated genes from this pathway by genes from other pathways and possibly increase the prediction accuracy. The same issue might be true when selecting a lot of genes as well, but it is more compelling when we have a limited budget of genes and can only select a few genes.Our method for gene selection will therefore be to prefilter the gene set and drop genes that are very similar. For the remaining genes we will apply a common test statistic and pull out the highest-ranking genes. One way to find correlated genes would be to calculate the correlation between all genes. In our first method weselected from the best genes (best according to a test statistic) those that have a pair-wise correlation below a certain threshold. A simple greedy algorithm accomplishes this selection – the k-th gene selected is the gene with highest p-value among all genes whose correlation to each of the first k-1 is below the specified threshold. This method is called “Correlation” in the figures below. Greedy algorithms are of course simple, but often give results of poor overall quality due to their myopic decision-making. As an alternative allowing a more global view of the data, we also consider clustering algorithms. Clustering is very versatile as it can use different distancefunctions (Euclidean, Lk , Mahalanobis, and correlation), and different underlyingmodels, shapes and densities, which are not captured by just correlation. In this paper we compared clustering and correlation methods. We used a fuzzy clustering algorithm because it assigns a membership probability for a cluster for each gene and may therefore capture the fact that some genes are involved in several pathways. Although a cluster does not automatically correspond to a pathway it is a reasonable approximation that genes in the same cluster have something to do with each other or are directly or indirectly involved in the same pathway. Our basic approach is to cluster the genes, and then to select one or more representative genes from each cluster. The details how many genes from which cluster depend on the “quality” of each cluster and will be discussed below.2.6Assigning quality to clusterOnce we have done the clustering we know that genes in a cluster show similar expression profiles and might be involved in the same pathway. Since we want to have as many pathways as possible involved in our list of significant genes, we would like to sample from each cluster/pathway. But it would not be fair to treat each cluster and gene equally. The size of the clusters as well as the quality of a cluster play a role, i.e. how close together are the genes, how far away are they from the cluster center. If a cluster is very tight and dense it can be assumed that the members are very similar. On the other hand if a cluster has wide dispersion the members of the cluster are more heterogeneous. To capture the biggest possible variety of genes, it would therefore be favorable to take more genes from a cluster of bad quality than from a cluster with good quality. To determine the quality for the fuzzy clustering algorithm we used the membership probability for a gene. We said that an element belongs to the cluster to which it has the highest membership probability. The cluster quality is then assessed by looking at the average membership probability of its elements.A high cluster quality means low dispersion, and the closer the quality gets to 0 the more scattered the cluster becomes. In our first clustering algorithm we decided that no matter how bad the quality and how small the size of the cluster we should get at least one element from each cluster. Our reasoning is as follows. First, if thecluster size is very small but there is a very good gene in it, we do not want to miss that cluster. Second, by eliminating a cluster we lose all the information of that pathway, so getting at least one representative plays a role like pseudocounts. Third, if we have a cluster that is extremely correlated, we would have a very high quality score and therefore may not pick any gene from that cluster. But this one gene from that cluster might have had a very good contribution to the discrimination process.The drawback is that a cluster might represent a pathway that is totally unrelated to the discrimination we look for. If the cluster then has a bad quality we might pick a lot of genes from that cluster even though they are not informative. To counteract this problem we implemented the possibility to mask out and exclude clusters that have an average bad test statistic p-value (this method is called “Masked out Clustering” in the figures, whereas “Clustering” refers to the method where we look at all clusters and do not mask out any). Lastly we want to have genes that have a high discriminatory power, i.e. can explain the symptoms. This can be achieved by using an appropriate test statistic.3Results and DiscussionFor our experiments we selected three different publicly available microarray datasets, Alon9(40 Adenocarcinoma and 22 normal samples), Golub6(47 ALL and 25 AML leukemia samples) and Notterman16(18 tumor and 18 normal samples). We compared five different test statistics: Fisher5, Golub6, Park4, TNoM1, and t-test and ran our three different filtering algorithms described above: Correlation, Clustering, Masked out Clustering. The performance of the feature selection was calculated using SVM and LOOCV scores.For each possible combination of test statistic and clustering algorithm we evaluated the performance varying the number of clusters between 1 and 30 and the number of selected features between 2 and 100. We used the Euclidean distance metric and a fuzzy clustering softness of 1.2 (where 1 would be hard clustering and infinity is everything belonging to all clusters). For SVM we chose an RBF kernel function and used data normalization in the feature space.We also calculated the LOOCV performance using all available data (i.e. not reducing the number of genes but using all of them). The result was that LOOCV produced 6 false classifications in the Alon’s colon dataset, resulting in an error of 9.7%. In Golub’s leukemia dataset LOOCV produced 2 false classifications, resulting in an error of 2.8% and in Notterman’s carcinoma dataset LOOCV made 1 false classification, resulting in an error of 2.8%.Figure 1 shows a 3d plot of the LOOCV performance varying the number of clusters selected between 1 and 10 and the number of features chosen between 10 and 100 in steps of 10. The plot shows the clustering algorithm without masking out.Figure 1: LOOCV performance for Alon’s data set using clustering and conventional methodsThere are no values for 10 features and more than 10 clusters, as well as 20 features and more than 20 clusters, as one of our constraints is that each cluster has at least one member in the feature set. So we can never have more clusters than features selected.In the leftmost ribbon (starting in the lower left corner and going up to the top left corner) we can see the performance varying the number of features and using just one cluster (i.e. this is our standard comparison line, as this reflects just selecting features by test-statistic). The whole plot seems very flat once we have more than 30 features. But notice the darker spots in the middle (between 10 and 20 clusters), that reflect very low LOOCV scores. One reason that there is a high peak for less than 30 features is that the t-test selects highly correlated and therefore redundant genes, which makes it hard for the underlying SVM to learn a good classifier. The average correlation of the top 10 genes selected with the t-test is 0.85.Figure 2 compares different test statistics on a given data set using the first clustering algorithm. Notice that Fisher and Golub behave very similarly as do Park and TNoM, but t-test has (besides the big bulk at only a few features) a very flat and robust behavior. Fisher and Golub seem to have a higher variance in classification but their best classification performance is similar to t-test. They achieve their best results with 6-25 clusters. TNoM and Park achieve their best results for fewer clusters (in the range of 1-6) and in fact they seem not to benefit from the clusteringas much as t-test, Fisher or Golub do.Figure 2: Comparison of different test statisticsFor Notterman’s carcinoma dataset the standard classification process already achieves 0% error when using more than 10 features but we can still improve the classification when using 10 features or less. Using only that few features we still manage to have a 0% error with most of the test statistics. Due to space restrictions figures are not shown here but can be accessed online17.Now consider how the LOOCV performance of our clustered result compares to the conventional methods (depicted as normal). In figure 3 we plot the normal score, the clustered scores (the minimum error score over all trials with cluster size from 2 to 30), the clustered scores with masking out and the correlation scores for the LOOCV performance for each of the five test-statistics. Here we did not plot the number of errors (plots for this are available online17), that reflect the efficiency of the classification but a ROC18 (receiver operator curves) score (i.e., the area under the ROC graph, which takes both false negative and false positive errors into account and reflects the robustness of the classification). We can see that almost always the filtered performance is better than the conventional method. Another noticeable fact is that without clustering, TNoM would have on average the best LOOCV performance of the five scores for Alon’s colon dataset. An explanation might be that TNoM, as a nonparametric test, extracts less correlated genes andtherefore already does a good job in selecting different genes.Figure 3: Comparison of test-statistics and different prefilter methods for Alon’s data set In the top 30 t-test genes in Alon’s dataset, we have an average correlation of 0.79, whereas in the top 30 TNoM genes, we have only an average correlation of 0.56. Wilcoxon (also a nonparametric test) achieves the best result of all the tests (when comparing 30 features) and can reduce the absolute LOOCV error to 5.17 The average correlation of the top 30 Wilcoxon genes is 0.43.Doing the same comparison on Golub’s dataset yields figure 4. In Alon’s dataset it seemed that TNoM was on average the best test statistic whereas in Golub’s leukemia dataset Wilcoxon performs best. We still see that clustering can lower the error in most of the cases. A remarkable fact is that with clustering we achieve 0% error with the t-test when using more than 50 features. Notice that we had 2 errors when using all the data. Here, not only can feature selection reduce the number of genes to find, but it can also decrease the error.Although clustering for feature selection generally seems to improve the LOOCV error, the least improvements were obtained using it in conjunction with the Wilcoxon rank sum test and the best performance improvement was achieved using it together with t-tests. As illustrated above the reason for that could be that the t-test generally finds more correlated genes. The nonparametric tests do not take values into account and calculate their scores purely based on rank information what seemsto have a positive effect on selecting fewer correlated genes.Figure 4: Comparison of different prefilter methods for Golub’s data set4Conclusion and further researchIn this paper we presented three novel prefilter methods to increase classification performance with microarray data. There is no clear winner between the three proposed methods and it depends largely on the dataset and parameters used. All the proposed feature selection methods find a subset that has better LOOCV performance than the currently used approaches. One question not addressed here is how to find the correct number of clusters. It is pretty expensive to try all possible numbers for clusters to find a setting that provides us with a good LOOCV performance. One direction for future work would be to estimate the number of clusters using a BIC19 (Bayesian Information Criterion) score or switching over to model based clustering20.We addressed the problem of feature selection and outlined why feature selection has to be done and how it can be done without losing crucial information. The question not answered here is how many features/genes should be chosen in the end. One could argue to choose exactly that many genes as necessary to achieve the lowest LOOCV error. But in the end it comes down to a tradeoff between false positives and false negatives. The more genes we have in our set of interest (the feature set) the more genes might also be real candidates. The extreme would be to take all genes. Then we definitely have all candidates but also a very high percentage of false positives. The other extreme would be to take no gene at all. Then we would not mispredict anything to be a real gene but would have a very high false negative rate. The final answer on how many genes to select can only be answered by the biologists who must judge how much time they can invest in examining these genes further and which false positive/negative rate they willaccept.We feel, however, that for any fixed size the methods outlined here are likely to identify sets of genes that are stronger predictors than sets found by standard methods, which should be of significant value for diagnostic purposes as well as for guiding further exploration of the underlying biology.AcknowledgmentThanks to Bill Noble for his helpful suggestions. JJ and RS were supported in part by NIH grant NHGRI/K01-02350. WLR and JJ were supported in part by NSF DBI 62-2677.References1 A. Ben-Dor, L. Bruhn, N. Friedman, I. Nachman, M. Schummer, and Z. Yakhini. Tissueclassification with gene expression profiles. RECOMB, (2000).2 J.L. Devore, Probability and Statistics for Engineering and the Sciences, 4th edition, Duxbury Press,(1995).3 Own unpublished results4 P.J. Park, M. Pagano, M. Bonetti: A nonparametric scoring algorithm for identifying informativegenes from microarray data. PSB:52-63, (2001).5 C.M. Bishop: Neural Networks for Pattern Recognition, Oxford University Press, (1995)6 T.R. Golub, D.K. Slonim, et al. Molecular classification of cancer: Class discovery and classprediction by gene expression monitoring. Science, 286:531-537, (1999).7 I. Lonnstedt and T. P. Speed. Replicated Microarray Data. Statistical Sinica, (2002).8 J. Weston, S. Mukherjee, O. Chapelle, M. Pontil, T. Poggio, and V. Vapnik. Feature selection forSVMs, Advances in Neural Information Processing Systems13. MIT Press, (2001).9 U. Alon, N. Barkai, D.A. Notterman, K. Gish, S. Ybarra, D. Mack and A.J. Levine: Broad patternsof gene expression revealed by clustering analysis of tumor and normal colon tissues probed by oligonucleotide arrays PNAS96:6745-6750, (1999).10 Y.H. Yang, M.J. Buckley, S. Dudoit, T.P. and Speed: Comparison of methods for image analysison cDNA microarray data. Technical report (2000)11 Y. H. Yang, S. Dudoit, P. Luu and T. P. Speed. Normalization for cDNA Microarray Data. SPIEBiOS, (2001).12 C. Cortes and V. N. Vapnik. Support vector networks. Machine Learning, 20:273-297, (1995).13 /gist/14 J.C. Dunn, "A fuzzy relative of the ISODATA process and its use in detecting compact well-separated clusters", Journal of Cybernetics, 3:32--57, (1973).15 A.K. Jain, R.C. Dubes. Algorithms for Clustering Data. Prentice Hall, (1988).16 D.A. Notterman, U. Alon, A.J. Sierk, A.J. Levine: Transcriptional Gene Expression Profiles ofColorectal Adenoma, Adenocarcinoma and Normal Tissue Examined by Oligonucleotide Arrays, Cancer Research61:3124-3130, (2001).17 /homes/jj/psb18 C.E. Metz, Basic principles of ROC analysis, Seminars in Nuclear Medicine, Vol 8, No. 4, 283-298, (1978).19 G. Schwarz: Estimating the dimension of a model. Annals of Statistics, 6:461-464 (1978).20 K. Y. Yeung, C. Fraley, A. Murua, A. E. Raftery and W. L. Ruzzo, Model-based clustering anddata transformation for gene expression data, Bioinformatics17:977-987 (2001).。