U.R.A. 369 C.N.R.S.-- Universite des Sciences et Technologies de Lille
大学德语考试题库及答案

大学德语考试题库及答案一、选择题(每题2分,共40分)1. 德语中的“图书馆”怎么说?A. BibliothekB. BüchereiC. LesehalleD. Bücherladen答案:A2. 下列哪个词是“学生”的复数形式?A. StudentB. StudentenC. SchülerD. Schülerinnen答案:B3. 用德语表达“我喜欢吃苹果”应该怎么说?A. Ich mag Äpfel essen.B. Ich mag Äpfel.C. Ich esse gerne Äpfel.D. Ich mag gerne Äpfel.答案:D4. “明天”在德语中怎么说?A. MorgenB. HeuteC. GesternD. Übermorgen5. “我的名字是”用德语怎么说?A. Mein Name istB. Mein Name sindC. Ich heißeD. Ich bin答案:A6. 德语中“医生”怎么说?A. ArztB. KrankenpflegerC. ApothekerD. Zahnarzt答案:A7. 用德语表达“你好吗?”应该怎么说?A. Wie geht es Ihnen?B. Wie geht es dir?C. Wie geht's?D. Wie fühlst du dich?答案:C8. “她”在德语中的复数形式是什么?A. SieB. SiesC. SieD. Sieen答案:C9. 德语中“书”怎么说?B. BücherC. BüchernD. Büchers答案:A10. “在图书馆”用德语怎么说?A. im BibliothekB. in der BibliothekC. im BüchereiD. in der Bücherei答案:B11. “你来自哪里?”用德语怎么说?A. Woher kommst du?B. Wohin gehst du?C. Wo bist du?D. Wo wohnst du?答案:A12. 德语中“老师”怎么说?A. LehrerB. SchülerC. StudentD. Arzt答案:A13. “我需要一杯咖啡”用德语怎么说?A. Ich brauche einen Kaffee.B. Ich brauche einen Tasse Kaffee.C. Ich möchte einen Kaffee.D. Ich will einen Tasse Kaffee.答案:A14. “晚上好”在德语中怎么说?A. Guten MorgenB. Guten TagC. Guten AbendD. Guten Nacht答案:C15. “你叫什么名字?”用德语怎么说?A. Wie heißt du?B. Was ist dein Name?C. Wie heißt dein Name?D. Was ist deine Name?答案:A16. 德语中“电影院”怎么说?A. KinoB. FilmtheaterC. FilmhausD. Theater答案:A17. “我不懂德语”用德语怎么说?A. Ich verstehe kein Deutsch.B. Ich verstehe nicht Deutsch.C. Ich verstehe kein Deutsch.D. Ich verstehe nicht kein Deutsch. 答案:B18. “你多大了?”用德语怎么说?A. Wie alt sind Sie?B. Wie alt bist du?C. Wie alt sind du?D. Wie alt bin ich?答案:B19. 德语中“银行”怎么说?A. BankB. GeldinstitutC. FinanzinstitutD. Sparinstitut答案:A20. “我很高兴见到你”用德语怎么说?A. Ich freue mich, dich zu sehen.B. Ich freue mich, Sie zu sehen.C. Es freut mich, dich zu sehen.D. Es freut mich, Sie zu sehen.答案:A二、填空题(每题2分,共20分)1. 德语中的“谢谢”是 _____。
研究生英语综合教程(下)-全部答案及解析
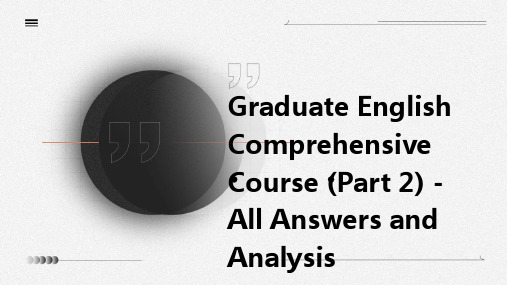
The correct answer is A. The interviewer asks about the best way to learn a new language, and the guest recommendations introduction
Listening Analysis
VS
Answer to Question 2
The correct answer is C. The author suggestions that improve their writing skills, students should read a variety of materials, write regularly, and seek feedback from peers and teachers
Analysis of tutorial characteristics
The tutorial is designed to be highly interactive and student-centered, encouraging active participation and discussion
Question 2
The correct answer is C. The speaker advice that to improve memory, one should exercise regularly, eat a balanced die, and practice relaxation techniques
Analysis 3
The interview is conducted in a case and conversational style, with the interviewer asking insightful questions and the guest offering practical tips on language learning The language used is accessible and engaging
Hazardous Products (Pacifiers) Regulations C.R.C.,_c._930

Current to June 28, 2010À jour au 28 juin 2010Published by the Minister of Justice at the following address:http://laws-lois.justice.gc.ca Publié par le ministre de la Justice à l’adresse suivante :http://laws-lois.justice.gc.caCANADACONSOLIDATION Hazardous Products (Pacifiers) RegulationsCODIFICATIONRèglement sur les produits dangereux(sucettes)C.R.C., c. 930C.R.C., ch. 930OFFICIAL STATUS OF CONSOLIDATIONS CARACTÈRE OFFICIEL DES CODIFICATIONSSubsections 31(1) and (3) of the Legislation Revision and Consolidation Act, in force on June 1, 2009, provide as follows:Les paragraphes 31(1) et (3) de la Loi sur la révision et la codification des textes législatifs, en vigueur le 1er juin 2009, prévoient ce qui suit :Published consolidation is evidence31. (1) Every copy of a consolidated statute orconsolidated regulation published by the Ministerunder this Act in either print or electronic form is ev-idence of that statute or regulation and of its contentsand every copy purporting to be published by theMinister is deemed to be so published, unless thecontrary is shown.31. (1) Tout exemplaire d'une loi codifiée oud'un règlement codifié, publié par le ministre en ver-tu de la présente loi sur support papier ou sur supportélectronique, fait foi de cette loi ou de ce règlementet de son contenu. Tout exemplaire donné commepublié par le ministre est réputé avoir été ainsi pu-blié, sauf preuve contraire.Codificationscomme élémentde preuve ...[...]Inconsistencies in regulations(3) In the event of an inconsistency between aconsolidated regulation published by the Ministerunder this Act and the original regulation or a subse-quent amendment as registered by the Clerk of thePrivy Council under the Statutory Instruments Act,the original regulation or amendment prevails to theextent of the inconsistency.(3) Les dispositions du règlement d'origine avecses modifications subséquentes enregistrées par legreffier du Conseil privé en vertu de la Loi sur lestextes réglementaires l'emportent sur les dispositionsincompatibles du règlement codifié publié par le mi-nistre en vertu de la présente loi.Incompatibilité— règlementsCHAPTER 930CHAPITRE 930HAZARDOUS PRODUCTS ACT LOI SUR LES PRODUITS DANGEREUX Hazardous Products (Pacifiers) Regulations Règlement sur les produits dangereux (sucettes)REGULATIONS RESPECTING THE ADVERTISING, SALE AND IMPORTATION OF HAZARDOUS PRODUCTS (PACIFIERS)RÈGLEMENT CONCERNANT LA VENTE, L’IMPORTATION ET LA PUBLICITÉ DES SUCETTESSHORT TITLE TITRE ABRÉGÉ1. These Regulations may be cited as the Hazardous Products (Pacifiers) Regulations.1. Le présent règlement peut être cité sous le titre : Règlement sur les produits dangereux (sucettes).INTERPRETATION DÉFINITIONS2. In these Regulations,“Act” means the Hazardous Products Act; (Loi)“product” means a pacifier or similar product included in item 27 of Part II of Schedule I to the Act. (produit)SOR/91-265, s. 2.2. Les définitions qui suivent s’appliquent au présent règlement.« Loi » La Loi sur les produits dangereux. (Act)« produit » Sucette ou produit semblable visé à l’ar-ticle 27 de la partie II de l’annexe I de la Loi. (product) DORS/91-265, art. 2.GENERAL DISPOSITIONS GÉNÉRALES3. A person may advertise, sell or import into Canadaa product only if it meets the requirements of these Reg-ulations.SOR/91-265, s. 3(F).3. La vente, l’importation et la publicité d’un produit sont autorisées à la condition que celui-ci soit conforme aux exigences du présent règlement.DORS/91-265, art. 3(F).ADVERTISING AND LABELLING PUBLICITÉ ET ÉTIQUETAGE4. (1) No reference, direct or indirect, to the Act or to these Regulations shall be made in any written material applied to or accompanying a product or in any adver-tisement thereof.4. (1) Il est interdit de faire tout renvoi direct ou indi-rect à la Loi ou au présent règlement dans les renseigne-ments écrits apposés sur un produit ou l’accompagnant, ainsi que dans la publicité de ce produit.(2) No representation in respect of the use of or modi-fication to a product shall be made in any written materi-al applied to or accompanying the product or in any ad-vertisement thereof, which use or modification would result in the failure of the product to meet a requirement of these Regulations.SOR/91-265, s. 4(F).(2) Il est interdit de donner, dans les renseignements écrits apposés sur un produit ou l’accompagnant, ou dans la publicité du produit, des indications sur un mode d’utilisation ou de modification du produit qui rendrait celui-ci non conforme aux exigences du présent règle-ment.DORS/91-265, art. 4(F).TOXICITY [SOR/92-586, s. 2]TOXICITÉ[DORS/92-586, art. 2]5. (1) [Revoked, SOR/92-586, s. 2] 5. (1) [Abrogé, DORS/92-586, art. 2](2) Every product, including all its parts and compo-nents shall meet the requirements of section 10 of the Hazardous Products (Toys) Regulations.(2) Tout produit, y compris tous ses éléments, doit ré-pondre aux prescriptions de l’article 10 du Règlement sur les produits dangereux (jouets).(3) No product or any part or component of the prod-uct shall contain more than 10 micrograms per kilogram total volatile N-nitrosamines, as determined by dichloromethane extraction.SOR/84-272, s. 1; SOR/85-478, s. 1; SOR/92-586, s. 2.(3) Aucun produit, y compris chaque élément, ne doit contenir plus de 10 microgrammes de N-nitrosamines volatiles totales par kilogramme, tel que déterminé par extraction au dichlorométhane.DORS/84-272, art. 1; DORS/85-478, art. 1; DORS/92-586, art. 2.DESIGN AND CONSTRUCTION CONCEPTION ET CONSTRUCTION6. Every product shall(a) be designed and constructed in such a manner as to protect the user, under reasonably foreseeable con-ditions of use, from(i) obstruction of the pharyngeal orifice,(ii) strangulation,(iii) ingestion or aspiration of the product or any part or component thereof, and(iv) wounding;(b) be designed and constructed so that,(i) the nipple is attached to a guard or shield of such dimensions that it cannot pass through the opening in the template illustrated in Schedule I when the nipple is centered on the opening and a load of 2.2 pounds is applied axially to the nipple in such a way as to induce the guard or shield to pull through the opening in the template,(ii) any loop of cord or other material attached to the product is not more than 14 inches in circumfer-ence,(iii) when tested in accordance with the procedure described in Schedule II(A) the nipple remains attached to the guard orshield described in subparagraph (i), and(B) no part or component is separated or brokenfree from the product that will fit, in a non-com-pressed state, into the small parts cylinder illus-trated in Schedule III, and 6. Tout produit doit êtrea) conçu et construit de façon à protéger l’utilisateur, dans les conditions d’utilisation raisonnablement pré-visibles, contre les dangers suivants :(i) obstruction de l’orifice pharyngien,(ii) strangulation,(iii) ingestion ou aspiration du produit ou d’un élé-ment du produit, et(iv) lésion;b) conçu et construit de façon(i) que la tétine soit fixée à une garde assez grande pour que celle-ci ne puisse passer par l’ouverture du gabarit indiqué à l’annexe I, lorsque la tétine est centrée sur l’ouverture et qu’une charge de 2,2 livres est appliquée à la tétine suivant l’axe de celle-ci de façon à entraîner la garde à travers l’ou-verture du gabarit,(ii) que toute boucle de corde ou d’autre matière at-tachée au produit ne mesure pas plus de 14 pouces de circonférence,(iii) que lorsque le produit est soumis à un essai conformément à la méthode exposée à l’annexe II,(A) la tétine reste fixée à la garde mentionnée ausous-alinéa (i), et(B) aucun élément qui s’insère, à l’état non com-primé, dans le cylindre pour petites pièces illustréà l’annexe III ne se détache ni ne se dégage; et(iv) any ring or handle is hinged, collapsible or flexible.SOR/2004-65, s. 1.(iv) que tout anneau ou poignée soit articulé, souple ou flexible.DORS/2004-65, art. 1.SCHEDULE I(s. 6)ANNEXE I (art. 6)GUARD TEMPLATE GABARIT DE LA GARDESCHEDULE II(s. 6)ANNEXE II (art. 6)TESTING PROCEDURE MÉTHODE D’ESSAI1. Hold the nipple of the pacifier in a fixed position. Apply a load 10 ± 0.25 pounds in the plane of the axis of the nipple to the handle of the pacifier at a rate of 1 ± 0.25 pounds per second and maintain the final load for 10 ± 0.5 seconds.1. Tenir la tétine de la sucette en position fixe. Appliquer à la poi-gnée une charge de 10 ± 0,25 livres sur le plan de l’axe de la tétine au rythme de 1 ± 0,25 livre par seconde et maintenir la tension définitive durant 10 ± 0,5 secondes.2. Hold the guard or shield of the pacifier in a fixed position. Ap-ply a load of 10 ± 0.25 pounds in the plane normal to the axis of the nipple to the handle of the pacifier at a rate of 1 ± 0.25 pounds per second and maintain the final load for 10 ± 0.5 seconds.2. Tenir la garde de la sucette en position fixe. Appliquer à la poi-gnée une charge de 10 ± 0,25 livres sur un plan normal par rapport àl’axe de la tétine au rythme de 1 ± 0,25 livre par seconde et maintenir la tension définitive durant 10 ± 0,5 secondes.3. Repeat the procedure described in section 2 with the load ap-plied to the nipple of the pacifier.3. Répéter l’opération de l’article 2, la charge étant appliquée à la tétine de la sucette.4. Immerse the pacifier in boiling water for 10 ± 0.5 minutes. Re-move the pacifier from the boiling water and allow to cool in air at 70 ± 5 degrees Fahrenheit for 15 ± 0.5 minutes. Repeat the tests de-scribed in sections 1, 2 and 3.4. Plonger la sucette dans de l’eau bouillante pour une période de 10 ± 0,5 minutes. Retirer la sucette de l’eau bouillante et laisser re-froidir à l’air à 70 ± 5 degrés Fahrenheit durant 15 ± 0,5 minutes. Ré-péter les essais des articles 1, 2 et 3.5. Repeat the entire procedure described in section 4 nine times. 5. Répéter neuf fois toute l’opération de l’article 4.SCHEDULE III (Clause 6(b)(iii)(B))ANNEXE III (division 6b)(iii)(B))SMALL PARTS CYLINDERCYLINDRE POUR PETITES PIÈCESNotes:– Not to scale– All dimensions in mmSOR/2004-65, s. 2.Remarques :– Pas à l’échelle– Dimensions en mmDORS/2004-65, art. 2.。
survey--on sentiment detection of reviews
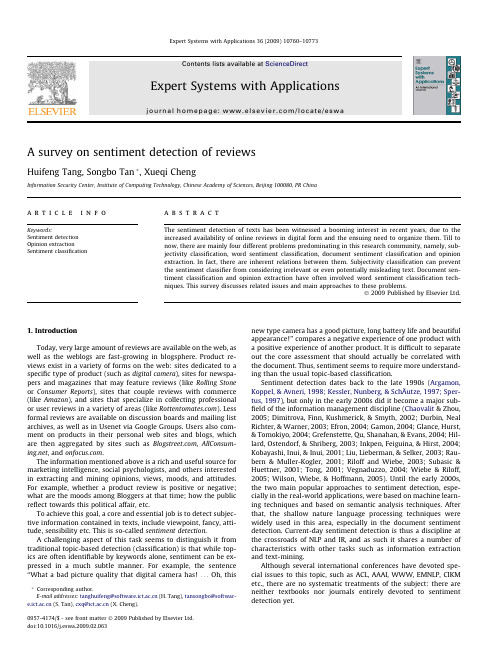
A survey on sentiment detection of reviewsHuifeng Tang,Songbo Tan *,Xueqi ChengInformation Security Center,Institute of Computing Technology,Chinese Academy of Sciences,Beijing 100080,PR Chinaa r t i c l e i n f o Keywords:Sentiment detection Opinion extractionSentiment classificationa b s t r a c tThe sentiment detection of texts has been witnessed a booming interest in recent years,due to the increased availability of online reviews in digital form and the ensuing need to organize them.Till to now,there are mainly four different problems predominating in this research community,namely,sub-jectivity classification,word sentiment classification,document sentiment classification and opinion extraction.In fact,there are inherent relations between them.Subjectivity classification can prevent the sentiment classifier from considering irrelevant or even potentially misleading text.Document sen-timent classification and opinion extraction have often involved word sentiment classification tech-niques.This survey discusses related issues and main approaches to these problems.Ó2009Published by Elsevier Ltd.1.IntroductionToday,very large amount of reviews are available on the web,as well as the weblogs are fast-growing in blogsphere.Product re-views exist in a variety of forms on the web:sites dedicated to a specific type of product (such as digital camera ),sites for newspa-pers and magazines that may feature reviews (like Rolling Stone or Consumer Reports ),sites that couple reviews with commerce (like Amazon ),and sites that specialize in collecting professional or user reviews in a variety of areas (like ).Less formal reviews are available on discussion boards and mailing list archives,as well as in Usenet via Google ers also com-ment on products in their personal web sites and blogs,which are then aggregated by sites such as , ,and .The information mentioned above is a rich and useful source for marketing intelligence,social psychologists,and others interested in extracting and mining opinions,views,moods,and attitudes.For example,whether a product review is positive or negative;what are the moods among Bloggers at that time;how the public reflect towards this political affair,etc.To achieve this goal,a core and essential job is to detect subjec-tive information contained in texts,include viewpoint,fancy,atti-tude,sensibility etc.This is so-called sentiment detection .A challenging aspect of this task seems to distinguish it from traditional topic-based detection (classification)is that while top-ics are often identifiable by keywords alone,sentiment can be ex-pressed in a much subtle manner.For example,the sentence ‘‘What a bad picture quality that digital camera has!...Oh,thisnew type camera has a good picture,long battery life and beautiful appearance!”compares a negative experience of one product with a positive experience of another product.It is difficult to separate out the core assessment that should actually be correlated with the document.Thus,sentiment seems to require more understand-ing than the usual topic-based classification.Sentiment detection dates back to the late 1990s (Argamon,Koppel,&Avneri,1998;Kessler,Nunberg,&SchÄutze,1997;Sper-tus,1997),but only in the early 2000s did it become a major sub-field of the information management discipline (Chaovalit &Zhou,2005;Dimitrova,Finn,Kushmerick,&Smyth,2002;Durbin,Neal Richter,&Warner,2003;Efron,2004;Gamon,2004;Glance,Hurst,&Tomokiyo,2004;Grefenstette,Qu,Shanahan,&Evans,2004;Hil-lard,Ostendorf,&Shriberg,2003;Inkpen,Feiguina,&Hirst,2004;Kobayashi,Inui,&Inui,2001;Liu,Lieberman,&Selker,2003;Rau-bern &Muller-Kogler,2001;Riloff and Wiebe,2003;Subasic &Huettner,2001;Tong,2001;Vegnaduzzo,2004;Wiebe &Riloff,2005;Wilson,Wiebe,&Hoffmann,2005).Until the early 2000s,the two main popular approaches to sentiment detection,espe-cially in the real-world applications,were based on machine learn-ing techniques and based on semantic analysis techniques.After that,the shallow nature language processing techniques were widely used in this area,especially in the document sentiment detection.Current-day sentiment detection is thus a discipline at the crossroads of NLP and IR,and as such it shares a number of characteristics with other tasks such as information extraction and text-mining.Although several international conferences have devoted spe-cial issues to this topic,such as ACL,AAAI,WWW,EMNLP,CIKM etc.,there are no systematic treatments of the subject:there are neither textbooks nor journals entirely devoted to sentiment detection yet.0957-4174/$-see front matter Ó2009Published by Elsevier Ltd.doi:10.1016/j.eswa.2009.02.063*Corresponding author.E-mail addresses:tanghuifeng@ (H.Tang),tansongbo@ (S.Tan),cxq@ (X.Cheng).Expert Systems with Applications 36(2009)10760–10773Contents lists available at ScienceDirectExpert Systems with Applicationsjournal homepage:/locate/eswaThis paperfirst introduces the definitions of several problems that pertain to sentiment detection.Then we present some appli-cations of sentiment detection.Section4discusses the subjectivity classification problem.Section5introduces semantic orientation method.The sixth section examines the effectiveness of applying machine learning techniques to document sentiment classification. The seventh section discusses opinion extraction problem.The eighth part talks about evaluation of sentiment st sec-tion concludes with challenges and discussion of future work.2.Sentiment detection2.1.Subjectivity classificationSubjectivity in natural language refers to aspects of language used to express opinions and evaluations(Wiebe,1994).Subjectiv-ity classification is stated as follows:Let S={s1,...,s n}be a set of sentences in document D.The problem of subjectivity classification is to distinguish sentences used to present opinions and other forms of subjectivity(subjective sentences set S s)from sentences used to objectively present factual information(objective sen-tences set S o),where S s[S o=S.This task is especially relevant for news reporting and Internet forums,in which opinions of various agents are expressed.2.2.Sentiment classificationSentiment classification includes two kinds of classification forms,i.e.,binary sentiment classification and multi-class senti-ment classification.Given a document set D={d1,...,d n},and a pre-defined categories set C={positive,negative},binary senti-ment classification is to classify each d i in D,with a label expressed in C.If we set C*={strong positive,positive,neutral,negative,strong negative}and classify each d i in D with a label in C*,the problem changes to multi-class sentiment classification.Most prior work on learning to identify sentiment has focused on the binary distinction of positive vs.negative.But it is often helpful to have more information than this binary distinction pro-vides,especially if one is ranking items by recommendation or comparing several reviewers’opinions.Koppel and Schler(2005a, 2005b)show that it is crucial to use neutral examples in learning polarity for a variety of reasons.Learning from negative and posi-tive examples alone will not permit accurate classification of neu-tral examples.Moreover,the use of neutral training examples in learning facilitates better distinction between positive and nega-tive examples.3.Applications of sentiment detectionIn this section,we will expound some rising applications of sen-timent detection.3.1.Products comparisonIt is a common practice for online merchants to ask their cus-tomers to review the products that they have purchased.With more and more people using the Web to express opinions,the number of reviews that a product receives grows rapidly.Most of the researches about these reviews were focused on automatically classifying the products into‘‘recommended”or‘‘not recom-mended”(Pang,Lee,&Vaithyanathan,2002;Ranjan Das&Chen, 2001;Terveen,Hill,Amento,McDonald,&Creter,1997).But every product has several features,in which maybe only part of them people are interested.Moreover,a product has shortcomings in one aspect,probably has merits in another place(Morinaga,Yamanishi,Tateishi,&Fukushima,2002;Taboada,Gillies,&McFe-tridge,2006).To analysis the online reviews and bring forward a visual man-ner to compare consumers’opinions of different products,i.e., merely with a single glance the user can clearly see the advantages and weaknesses of each product in the minds of consumers.For a potential customer,he/she can see a visual side-by-side and fea-ture-by-feature comparison of consumer opinions on these prod-ucts,which helps him/her to decide which product to buy.For a product manufacturer,the comparison enables it to easily gather marketing intelligence and product benchmarking information.Liu,Hu,and Cheng(2005)proposed a novel framework for ana-lyzing and comparing consumer opinions of competing products.A prototype system called Opinion Observer is implemented.To en-able the visualization,two tasks were performed:(1)Identifying product features that customers have expressed their opinions on,based on language pattern mining techniques.Such features form the basis for the comparison.(2)For each feature,identifying whether the opinion from each reviewer is positive or negative,if any.Different users can visualize and compare opinions of different products using a user interface.The user simply chooses the prod-ucts that he/she wishes to compare and the system then retrieves the analyzed results of these products and displays them in the interface.3.2.Opinion summarizationThe number of online reviews that a product receives grows rapidly,especially for some popular products.Furthermore,many reviews are long and have only a few sentences containing opin-ions on the product.This makes it hard for a potential customer to read them to make an informed decision on whether to purchase the product.The large number of reviews also makes it hard for product manufacturers to keep track of customer opinions of their products because many merchant sites may sell their products,and the manufacturer may produce many kinds of products.Opinion summarization(Ku,Lee,Wu,&Chen,2005;Philip et al., 2004)summarizes opinions of articles by telling sentiment polari-ties,degree and the correlated events.With opinion summariza-tion,a customer can easily see how the existing customers feel about a product,and the product manufacturer can get the reason why different stands people like it or what they complain about.Hu and Liu(2004a,2004b)conduct a work like that:Given a set of customer reviews of a particular product,the task involves three subtasks:(1)identifying features of the product that customers have expressed their opinions on(called product features);(2) for each feature,identifying review sentences that give positive or negative opinions;and(3)producing a summary using the dis-covered information.Ku,Liang,and Chen(2006)investigated both news and web blog articles.In their research,TREC,NTCIR and articles collected from web blogs serve as the information sources for opinion extraction.Documents related to the issue of animal cloning are selected as the experimental materials.Algorithms for opinion extraction at word,sentence and document level are proposed. The issue of relevant sentence selection is discussed,and then top-ical and opinionated information are summarized.Opinion sum-marizations are visualized by representative sentences.Finally, an opinionated curve showing supportive and non-supportive de-gree along the timeline is illustrated by an opinion tracking system.3.3.Opinion reason miningIn opinion analysis area,finding the polarity of opinions or aggregating and quantifying degree assessment of opinionsH.Tang et al./Expert Systems with Applications36(2009)10760–1077310761scattered throughout web pages is not enough.We can do more critical part of in-depth opinion assessment,such asfinding rea-sons in opinion-bearing texts.For example,infilm reviews,infor-mation such as‘‘found200positive reviews and150negative reviews”may not fully satisfy the information needs of different people.More useful information would be‘‘Thisfilm is great for its novel originality”or‘‘Poor acting,which makes thefilm awful”.Opinion reason mining tries to identify one of the critical ele-ments of online reviews to answer the question,‘‘What are the rea-sons that the author of this review likes or dislikes the product?”To answer this question,we should extract not only sentences that contain opinion-bearing expressions,but also sentences with rea-sons why an author of a review writes the review(Cardie,Wiebe, Wilson,&Litman,2003;Clarke&Terra,2003;Li&Yamanishi, 2001;Stoyanov,Cardie,Litman,&Wiebe,2004).Kim and Hovy(2005)proposed a method for detecting opinion-bearing expressions.In their subsequent work(Kim&Hovy,2006), they collected a large set of h review text,pros,cons i triplets from ,which explicitly state pros and cons phrases in their respective categories by each review’s author along with the re-view text.Their automatic labeling systemfirst collects phrases in pro and confields and then searches the main review text in or-der to collect sentences corresponding to those phrases.Then the system annotates this sentence with the appropriate‘‘pro”or‘‘con”label.All remaining sentences with neither label are marked as ‘‘neither”.After labeling all the data,they use it to train their pro and con sentence recognition system.3.4.Other applicationsThomas,Pang,and Lee(2006)try to determine from the tran-scripts of US Congressionalfloor debates whether the speeches rep-resent support of or opposition to proposed legislation.Mullen and Malouf(2006)describe a statistical sentiment analysis method on political discussion group postings to judge whether there is oppos-ing political viewpoint to the original post.Moreover,there are some potential applications of sentiment detection,such as online message sentimentfiltering,E-mail sentiment classification,web-blog author’s attitude analysis,sentiment web search engine,etc.4.Subjectivity classificationSubjectivity classification is a task to investigate whether a par-agraph presents the opinion of its author or reports facts.In fact, most of the research showed there was very tight relation between subjectivity classification and document sentiment classification (Pang&Lee,2004;Wiebe,2000;Wiebe,Bruce,&O’Hara,1999; Wiebe,Wilson,Bruce,Bell,&Martin,2002;Yu&Hatzivassiloglou, 2003).Subjectivity classification can prevent the polarity classifier from considering irrelevant or even potentially misleading text. Pang and Lee(2004)find subjectivity detection can compress re-views into much shorter extracts that still retain polarity informa-tion at a level comparable to that of the full review.Much of the research in automated opinion detection has been performed and proposed for discriminating between subjective and objective text at the document and sentence levels(Bruce& Wiebe,1999;Finn,Kushmerick,&Smyth,2002;Hatzivassiloglou &Wiebe,2000;Wiebe,2000;Wiebe et al.,1999;Wiebe et al., 2002;Yu&Hatzivassiloglou,2003).In this section,we will discuss some approaches used to automatically assign one document as objective or subjective.4.1.Similarity approachSimilarity approach to classifying sentences as opinions or facts explores the hypothesis that,within a given topic,opinion sen-tences will be more similar to other opinion sentences than to fac-tual sentences(Yu&Hatzivassiloglou,2003).Similarity approach measures sentence similarity based on shared words,phrases, and WordNet synsets(Dagan,Shaul,&Markovitch,1993;Dagan, Pereira,&Lee,1994;Leacock&Chodorow,1998;Miller&Charles, 1991;Resnik,1995;Zhang,Xu,&Callan,2002).To measure the overall similarity of a sentence to the opinion or fact documents,we need to go through three steps.First,use IR method to acquire the documents that are on the same topic as the sentence in question.Second,calculate its similarity scores with each sentence in those documents and make an average va-lue.Third,assign the sentence to the category(opinion or fact) for which the average value is the highest.Alternatively,for the frequency variant,we can use the similarity scores or count how many of them for each category,and then compare it with a prede-termined threshold.4.2.Naive Bayes classifierNaive Bayes classifier is a commonly used supervised machine learning algorithm.This approach presupposes all sentences in opinion or factual articles as opinion or fact sentences.Naive Bayes uses the sentences in opinion and fact documents as the examples of the two categories.The features include words, bigrams,and trigrams,as well as the part of speech in each sen-tence.In addition,the presence of semantically oriented(positive and negative)words in a sentence is an indicator that the sentence is subjective.Therefore,it can include the counts of positive and negative words in the sentence,as well as counts of the polarities of sequences of semantically oriented words(e.g.,‘‘++”for two con-secutive positively oriented words).It also include the counts of parts of speech combined with polarity information(e.g.,‘‘JJ+”for positive adjectives),as well as features encoding the polarity(if any)of the head verb,the main subject,and their immediate modifiers.Generally speaking,Naive Bayes assigns a document d j(repre-sented by a vector dÃj)to the class c i that maximizes Pðc i j dÃjÞby applying Bayes’rule as follow,Pðc i j dÃjÞ¼Pðc iÞPðdÃjj c iÞPðdÃjÞð1Þwhere PðdÃjÞis the probability that a randomly picked document dhas vector dÃjas its representation,and P(c)is the probability that a randomly picked document belongs to class c.To estimate the term PðdÃjj cÞ,Naive Bayes decomposes it byassuming all the features in dÃj(represented by f i,i=1to m)are con-ditionally independent,i.e.,Pðc i j dÃjÞ¼Pðc iÞQ mi¼1Pðf i j c iÞÀÁPðdÃjÞð2Þ4.3.Multiple Naive Bayes classifierThe hypothesis of all sentences in opinion or factual articles as opinion or fact sentences is an approximation.To address this, multiple Naive Bayes classifier approach applies an algorithm using multiple classifiers,each relying on a different subset of fea-tures.The goal is to reduce the training set to the sentences that are most likely to be correctly labeled,thus boosting classification accuracy.Given separate sets of features F1,F2,...,F m,it train separate Na-ive Bayes classifiers C1,C2,...,C m corresponding to each feature set. Assuming as ground truth the information provided by the docu-ment labels and that all sentences inherit the status of their docu-ment as opinions or facts,itfirst train C1on the entire training set,10762H.Tang et al./Expert Systems with Applications36(2009)10760–10773then use the resulting classifier to predict labels for the training set.The sentences that receive a label different from the assumed truth are then removed,and train C2on the remaining sentences. This process is repeated iteratively until no more sentences can be removed.Yu and Hatzivassiloglou(2003)report results using five feature sets,starting from words alone and adding in bigrams, trigrams,part-of-speech,and polarity.4.4.Cut-based classifierCut-based classifier approach put forward a hypothesis that, text spans(items)occurring near each other(within discourse boundaries)may share the same subjectivity status(Pang&Lee, 2004).Based on this hypothesis,Pang supplied his algorithm with pair-wise interaction information,e.g.,to specify that two particu-lar sentences should ideally receive the same subjectivity label. This algorithm uses an efficient and intuitive graph-based formula-tion relying onfinding minimum cuts.Suppose there are n items x1,x2,...,x n to divide into two classes C1and C2,here access to two types of information:ind j(x i):Individual scores.It is the non-negative estimates of each x i’s preference for being in C j based on just the features of x i alone;assoc(x i,x k):Association scores.It is the non-negative estimates of how important it is that x i and x k be in the same class.Then,this problem changes to calculate the maximization of each item’s score for one class:its individual score for the class it is assigned to,minus its individual score for the other class,then minus associated items into different classes for penalization. Thus,after some algebra,it arrives at the following optimization problem:assign the x i to C1and C2so as to minimize the partition cost:X x2C1ind2ðxÞþXx2C2ind1ðxÞþXx i2C1;x k2C2assocðx i;x kÞð3ÞThis situation can be represented in the following manner.Build an undirected graph G with vertices{v1,...,v n,s,t};the last two are, respectively,the source and sink.Add n edges(s,v i),each with weight ind1(x i),and n edges(v i,t),each with weight ind2(x i).Finally, addðC2nÞedges(v i,v k),each with weight assoc(x i,x k).A cut(S,T)of G is a partition of its nodes into sets S={s}US0and T={t}UT0,where s R S0,t R T0.Its cost cost(S,T)is the sum of the weights of all edges crossing from S to T.A minimum cut of G is one of minimum cost. Then,finding solution of this problem is changed into looking for a minimum cut of G.5.Word sentiment classificationThe task on document sentiment classification has usually in-volved the manual or semi-manual construction of semantic orien-tation word lexicons(Hatzivassiloglou&McKeown,1997; Hatzivassiloglou&Wiebe,2000;Lin,1998;Pereira,Tishby,&Lee, 1993;Riloff,Wiebe,&Wilson,2003;Turney&Littman,2002; Wiebe,2000),which built by word sentiment classification tech-niques.For instance,Das and Chen(2001)used a classifier on investor bulletin boards to see if apparently positive postings were correlated with stock price,in which several scoring methods were employed in conjunction with a manually crafted lexicon.Classify-ing the semantic orientation of individual words or phrases,such as whether it is positive or negative or has different intensities, generally using a pre-selected set of seed words,sometimes using linguistic heuristics(For example,Lin(1998)&Pereira et al.(1993) used linguistic co-locations to group words with similar uses or meanings).Some studies showed that restricting features to those adjec-tives for word sentiment classification would improve perfor-mance(Andreevskaia&Bergler,2006;Turney&Littman,2002; Wiebe,2000).However,more researches showed most of the adjectives and adverb,a small group of nouns and verbs possess semantic orientation(Andreevskaia&Bergler,2006;Esuli&Sebas-tiani,2005;Gamon&Aue,2005;Takamura,Inui,&Okumura, 2005;Turney&Littman,2003).Automatic methods of sentiment annotation at the word level can be grouped into two major categories:(1)corpus-based ap-proaches and(2)dictionary-based approaches.Thefirst group in-cludes methods that rely on syntactic or co-occurrence patterns of words in large texts to determine their sentiment(e.g.,Hatzi-vassiloglou&McKeown,1997;Turney&Littman,2002;Yu&Hat-zivassiloglou,2003and others).The second group uses WordNet (/)information,especially,synsets and hierarchies,to acquire sentiment-marked words(Hu&Liu, 2004a;Kim&Hovy,2004)or to measure the similarity between candidate words and sentiment-bearing words such as good and bad(Kamps,Marx,Mokken,&de Rijke,2004).5.1.Analysis by conjunctions between adjectivesThis method attempts to predict the orientation of subjective adjectives by analyzing pairs of adjectives(conjoined by and,or, but,either-or,or neither-nor)which are extracted from a large unlabelled document set.The underlying intuition is that the act of conjoining adjectives is subject to linguistic constraints on the orientation of the adjectives involved(e.g.and usually conjoins two adjectives of the same-orientation,while but conjoins two adjectives of opposite orientation).This is shown in the following three sentences(where thefirst two are perceived as correct and the third is perceived as incorrect)taken from Hatzivassiloglou and McKeown(1997):‘‘The tax proposal was simple and well received by the public”.‘‘The tax proposal was simplistic but well received by the public”.‘‘The tax proposal was simplistic and well received by the public”.To infer the orientation of adjectives from analysis of conjunc-tions,a supervised learning algorithm can be performed as follow-ing steps:1.All conjunctions of adjectives are extracted from a set ofdocuments.2.Train a log-linear regression classifier and then classify pairs ofadjectives either as having the same or as having different ori-entation.The hypothesized same-orientation or different-orien-tation links between all pairs form a graph.3.A clustering algorithm partitions the graph produced in step2into two clusters.By using the intuition that positive adjectives tend to be used more frequently than negative ones,the cluster containing the terms of higher average frequency in the docu-ment set is deemed to contain the positive terms.The log-linear model offers an estimate of how good each pre-diction is,since it produces a value y between0and1,in which 1corresponds to same-orientation,and one minus the produced value y corresponds to dissimilarity.Same-and different-orienta-tion links between adjectives form a graph.To partition the graph nodes into subsets of the same-orientation,the clustering algo-rithm calculates an objective function U scoring each possible par-tition P of the adjectives into two subgroups C1and C2as,UðPÞ¼X2i¼11j C i jXx;y2C i;x–ydðx;yÞ!ð4Þwhere j C i j is the cardinality of cluster i,and d(x,y)is the dissimilarity between adjectives x and y.H.Tang et al./Expert Systems with Applications36(2009)10760–1077310763In general,because the model was unsupervised,it required an immense word corpus to function.5.2.Analysis by lexical relationsThis method presents a strategy for inferring semantic orienta-tion from semantic association between words and phrases.It fol-lows a hypothesis that two words tend to be the same semantic orientation if they have strong semantic association.Therefore,it focused on the use of lexical relations defined in WordNet to calcu-late the distance between adjectives.Generally speaking,we can defined a graph on the adjectives contained in the intersection between a term set(For example, TL term set(Turney&Littman,2003))and WordNet,adding a link between two adjectives whenever WordNet indicates the presence of a synonymy relation between them,and defining a distance measure using elementary notions from graph theory.In more de-tail,this approach can be realized as following steps:1.Construct relations at the level of words.The simplest approachhere is just to collect all words in WordNet,and relate words that can be synonymous(i.e.,they occurring in the same synset).2.Define a distance measure d(t1,t2)between terms t1and t2onthis graph,which amounts to the length of the shortest path that connects t1and t2(with d(t1,t2)=+1if t1and t2are not connected).3.Calculate the orientation of a term by its relative distance(Kamps et al.,2004)from the two seed terms good and bad,i.e.,SOðtÞ¼dðt;badÞÀdðt;goodÞdðgood;badÞð5Þ4.Get the result followed by this rules:The adjective t is deemedto belong to positive if SO(t)>0,and the absolute value of SO(t) determines,as usual,the strength of this orientation(the con-stant denominator d(good,bad)is a normalization factor that constrains all values of SO to belong to the[À1,1]range).5.3.Analysis by glossesThe characteristic of this method lies in the fact that it exploits the glosses(i.e.textual definitions)that one term has in an online ‘‘glossary”,or dictionary.Its basic assumption is that if a word is semantically oriented in one direction,then the words in its gloss tend to be oriented in the same direction(Esuli&Sebastiani,2005; Esuli&Sebastiani,2006a,2006b).For instance,the glosses of good and excellent will both contain appreciative expressions;while the glosses of bad and awful will both contain derogative expressions.Generally,this method can determine the orientation of a term based on the classification of its glosses.The process is composed of the following steps:1.A seed set(S p,S n),representative of the two categories positiveand negative,is provided as input.2.Search new terms to enrich S p and S e lexical relations(e.g.synonymy)with the terms contained in S p and S n from a thesau-rus,or online dictionary,tofind these new terms,and then append them to S p or S n.3.For each term t i in S0p [S0nor in the test set(i.e.the set of termsto be classified),a textual representation of t i is generated by collating all the glosses of t i as found in a machine-readable dic-tionary.Each such representation is converted into a vector by standard text indexing techniques.4.A binary text classifier is trained on the terms in S0p [S0nandthen applied to the terms in the test set.5.4.Analysis by both lexical relations and glossesThis method determines sentiment of words and phrases both relies on lexical relations(synonymy,antonymy and hyponymy) and glosses provided in WordNet.Andreevskaia and Bergler(2006)proposed an algorithm named ‘‘STEP”(Semantic Tag Extraction Program).This algorithm starts with a small set of seed words of known sentiment value(positive or negative)and implements the following steps:1.Extend the small set of seed words by adding synonyms,ant-onyms and hyponyms of the seed words supplied in WordNet.This step brings on average a5-fold increase in the size of the original list with the accuracy of the resulting list comparable to manual annotations.2.Go through all WordNet glosses,identifies the entries that con-tain in their definitions the sentiment-bearing words from the extended seed list,and adds these head words to the corre-sponding category–positive,negative or neutral.3.Disambiguate the glosses with part-of-speech tagger,and elim-inate errors of some words acquired in step1and from the seed list.At this step,it alsofilters out all those words that have been assigned contradicting.In this algorithm,for each word we need compute a Net Overlap Score by subtracting the total number of runs assigning this word a negative sentiment from the total of the runs that consider it posi-tive.In order to make the Net Overlap Score measure usable in sen-timent tagging of texts and phrases,the absolute values of this score should be normalized and mapped onto a standard[0,1] interval.STEP accomplishes this normalization by using the value of the Net Overlap Score as a parameter in the standard fuzzy mem-bership S-function(Zadeh,1987).This function maps the absolute values of the Net Overlap Score onto the interval from0to1,where 0corresponds to the absence of membership in the category of sentiment(in this case,these will be the neutral words)and1re-flects the highest degree of membership in this category.The func-tion can be defined as follows,Sðu;a;b;cÞ¼0if u6a2uÀac a2if a6u6b1À2uÀacÀa2if b6u6c1if u P c8>>>>>><>>>>>>:ð6Þwhere u is the Net Overlap Score for the word and a,b,c are the three adjustable parameters:a is set to1,c is set to15and b,which represents a crossover point,is defined as b=(a+c)/2=8.Defined this way,the S-function assigns highest degree of membership (=1)to words that have the Net Overlap Score u P15.Net Overlap Score can be used as a measure of the words degree of membership in the fuzzy category of sentiment:the core adjec-tives,which had the highest Net Overlap Score,were identified most accurately both by STEP and by human annotators,while the words on the periphery of the category had the lowest scores and were associated with low rates of inter-annotator agreement.5.5.Analysis by pointwise mutual informationThe general strategy of this method is to infer semantic orienta-tion from semantic association.The underlying assumption is that a phrase has a positive semantic orientation when it has good asso-ciations(e.g.,‘‘romantic ambience”)and a negative semantic orien-tation when it has bad associations(e.g.,‘‘horrific events”)(Turney, 2002).10764H.Tang et al./Expert Systems with Applications36(2009)10760–10773。
Example-based metonymy recognition for proper nouns
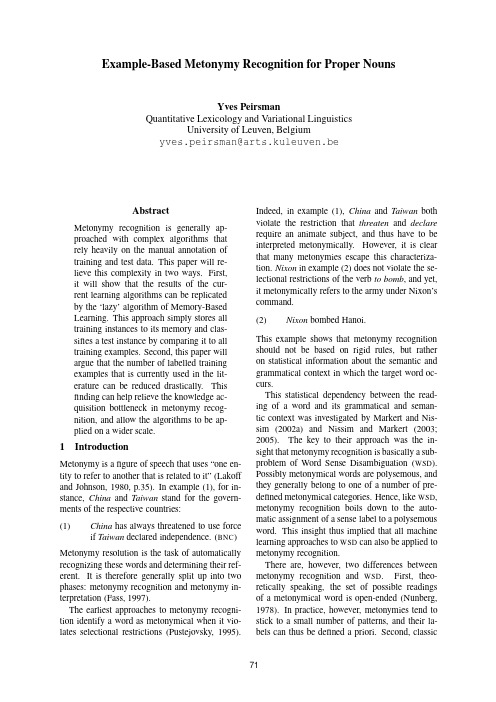
Example-Based Metonymy Recognition for Proper NounsYves PeirsmanQuantitative Lexicology and Variational LinguisticsUniversity of Leuven,Belgiumyves.peirsman@arts.kuleuven.beAbstractMetonymy recognition is generally ap-proached with complex algorithms thatrely heavily on the manual annotation oftraining and test data.This paper will re-lieve this complexity in two ways.First,it will show that the results of the cur-rent learning algorithms can be replicatedby the‘lazy’algorithm of Memory-BasedLearning.This approach simply stores alltraining instances to its memory and clas-sifies a test instance by comparing it to alltraining examples.Second,this paper willargue that the number of labelled trainingexamples that is currently used in the lit-erature can be reduced drastically.Thisfinding can help relieve the knowledge ac-quisition bottleneck in metonymy recog-nition,and allow the algorithms to be ap-plied on a wider scale.1IntroductionMetonymy is afigure of speech that uses“one en-tity to refer to another that is related to it”(Lakoff and Johnson,1980,p.35).In example(1),for in-stance,China and Taiwan stand for the govern-ments of the respective countries:(1)China has always threatened to use forceif Taiwan declared independence.(BNC) Metonymy resolution is the task of automatically recognizing these words and determining their ref-erent.It is therefore generally split up into two phases:metonymy recognition and metonymy in-terpretation(Fass,1997).The earliest approaches to metonymy recogni-tion identify a word as metonymical when it vio-lates selectional restrictions(Pustejovsky,1995).Indeed,in example(1),China and Taiwan both violate the restriction that threaten and declare require an animate subject,and thus have to be interpreted metonymically.However,it is clear that many metonymies escape this characteriza-tion.Nixon in example(2)does not violate the se-lectional restrictions of the verb to bomb,and yet, it metonymically refers to the army under Nixon’s command.(2)Nixon bombed Hanoi.This example shows that metonymy recognition should not be based on rigid rules,but rather on statistical information about the semantic and grammatical context in which the target word oc-curs.This statistical dependency between the read-ing of a word and its grammatical and seman-tic context was investigated by Markert and Nis-sim(2002a)and Nissim and Markert(2003; 2005).The key to their approach was the in-sight that metonymy recognition is basically a sub-problem of Word Sense Disambiguation(WSD). Possibly metonymical words are polysemous,and they generally belong to one of a number of pre-defined metonymical categories.Hence,like WSD, metonymy recognition boils down to the auto-matic assignment of a sense label to a polysemous word.This insight thus implied that all machine learning approaches to WSD can also be applied to metonymy recognition.There are,however,two differences between metonymy recognition and WSD.First,theo-retically speaking,the set of possible readings of a metonymical word is open-ended(Nunberg, 1978).In practice,however,metonymies tend to stick to a small number of patterns,and their la-bels can thus be defined a priori.Second,classic 71WSD algorithms take training instances of one par-ticular word as their input and then disambiguate test instances of the same word.By contrast,since all words of the same semantic class may undergo the same metonymical shifts,metonymy recogni-tion systems can be built for an entire semantic class instead of one particular word(Markert and Nissim,2002a).To this goal,Markert and Nissim extracted from the BNC a corpus of possibly metonymical words from two categories:country names (Markert and Nissim,2002b)and organization names(Nissim and Markert,2005).All these words were annotated with a semantic label —either literal or the metonymical cate-gory they belonged to.For the country names, Markert and Nissim distinguished between place-for-people,place-for-event and place-for-product.For the organi-zation names,the most frequent metonymies are organization-for-members and organization-for-product.In addition, Markert and Nissim used a label mixed for examples that had two readings,and othermet for examples that did not belong to any of the pre-defined metonymical patterns.For both categories,the results were promis-ing.The best algorithms returned an accuracy of 87%for the countries and of76%for the orga-nizations.Grammatical features,which gave the function of a possibly metonymical word and its head,proved indispensable for the accurate recog-nition of metonymies,but led to extremely low recall values,due to data sparseness.Therefore Nissim and Markert(2003)developed an algo-rithm that also relied on semantic information,and tested it on the mixed country data.This algo-rithm used Dekang Lin’s(1998)thesaurus of se-mantically similar words in order to search the training data for instances whose head was sim-ilar,and not just identical,to the test instances. Nissim and Markert(2003)showed that a combi-nation of semantic and grammatical information gave the most promising results(87%). However,Nissim and Markert’s(2003)ap-proach has two major disadvantages.Thefirst of these is its complexity:the best-performing al-gorithm requires smoothing,backing-off to gram-matical roles,iterative searches through clusters of semantically similar words,etc.In section2,I will therefore investigate if a metonymy recognition al-gorithm needs to be that computationally demand-ing.In particular,I will try and replicate Nissim and Markert’s results with the‘lazy’algorithm of Memory-Based Learning.The second disadvantage of Nissim and Mark-ert’s(2003)algorithms is their supervised nature. Because they rely so heavily on the manual an-notation of training and test data,an extension of the classifiers to more metonymical patterns is ex-tremely problematic.Yet,such an extension is es-sential for many tasks throughout thefield of Nat-ural Language Processing,particularly Machine Translation.This knowledge acquisition bottle-neck is a well-known problem in NLP,and many approaches have been developed to address it.One of these is active learning,or sample selection,a strategy that makes it possible to selectively an-notate those examples that are most helpful to the classifier.It has previously been applied to NLP tasks such as parsing(Hwa,2002;Osborne and Baldridge,2004)and Word Sense Disambiguation (Fujii et al.,1998).In section3,I will introduce active learning into thefield of metonymy recog-nition.2Example-based metonymy recognition As I have argued,Nissim and Markert’s(2003) approach to metonymy recognition is quite com-plex.I therefore wanted to see if this complexity can be dispensed with,and if it can be replaced with the much more simple algorithm of Memory-Based Learning.The advantages of Memory-Based Learning(MBL),which is implemented in the T i MBL classifier(Daelemans et al.,2004)1,are twofold.First,it is based on a plausible psycho-logical hypothesis of human learning.It holds that people interpret new examples of a phenom-enon by comparing them to“stored representa-tions of earlier experiences”(Daelemans et al., 2004,p.19).This contrasts to many other classi-fication algorithms,such as Naive Bayes,whose psychological validity is an object of heavy de-bate.Second,as a result of this learning hypothe-sis,an MBL classifier such as T i MBL eschews the formulation of complex rules or the computation of probabilities during its training phase.Instead it stores all training vectors to its memory,together with their labels.In the test phase,it computes the distance between the test vector and all these train-ing vectors,and simply returns the most frequentlabel of the most similar training examples.One of the most important challenges inMemory-Based Learning is adapting the algorithmto one’s data.This includesfinding a represen-tative seed set as well as determining the rightdistance measures.For my purposes,however, T i MBL’s default settings proved more than satis-factory.T i MBL implements the IB1and IB2algo-rithms that were presented in Aha et al.(1991),butadds a broad choice of distance measures.Its de-fault implementation of the IB1algorithm,whichis called IB1-IG in full(Daelemans and Van denBosch,1992),proved most successful in my ex-periments.It computes the distance between twovectors X and Y by adding up the weighted dis-tancesδbetween their corresponding feature val-ues x i and y i:∆(X,Y)=ni=1w iδ(x i,y i)(3)The most important element in this equation is theweight that is given to each feature.In IB1-IG,features are weighted by their Gain Ratio(equa-tion4),the division of the feature’s InformationGain by its split rmation Gain,the nu-merator in equation(4),“measures how much in-formation it[feature i]contributes to our knowl-edge of the correct class label[...]by comput-ing the difference in uncertainty(i.e.entropy)be-tween the situations without and with knowledgeof the value of that feature”(Daelemans et al.,2004,p.20).In order not“to overestimate the rel-evance of features with large numbers of values”(Daelemans et al.,2004,p.21),this InformationGain is then divided by the split info,the entropyof the feature values(equation5).In the followingequations,C is the set of class labels,H(C)is theentropy of that set,and V i is the set of values forfeature i.w i=H(C)− v∈V i P(v)×H(C|v)2This data is publicly available and can be downloadedfrom /mnissim/mascara.73P F86.6%49.5%N&M81.4%62.7%Table1:Results for the mixed country data.T i MBL:my T i MBL resultsN&M:Nissim and Markert’s(2003)results simple learning phase,T i MBL is able to replicate the results from Nissim and Markert(2003;2005). As table1shows,accuracy for the mixed coun-try data is almost identical to Nissim and Mark-ert’sfigure,and precision,recall and F-score for the metonymical class lie only slightly lower.3 T i MBL’s results for the Hungary data were simi-lar,and equally comparable to Markert and Nis-sim’s(Katja Markert,personal communication). Note,moreover,that these results were reached with grammatical information only,whereas Nis-sim and Markert’s(2003)algorithm relied on se-mantics as well.Next,table2indicates that T i MBL’s accuracy for the mixed organization data lies about1.5%be-low Nissim and Markert’s(2005)figure.This re-sult should be treated with caution,however.First, Nissim and Markert’s available organization data had not yet been annotated for grammatical fea-tures,and my annotation may slightly differ from theirs.Second,Nissim and Markert used several feature vectors for instances with more than one grammatical role andfiltered all mixed instances from the training set.A test instance was treated as mixed only when its several feature vectors were classified differently.My experiments,in contrast, were similar to those for the location data,in that each instance corresponded to one vector.Hence, the slightly lower performance of T i MBL is prob-ably due to differences between the two experi-ments.Thesefirst experiments thus demonstrate that Memory-Based Learning can give state-of-the-art performance in metonymy recognition.In this re-spect,it is important to stress that the results for the country data were reached without any se-mantic information,whereas Nissim and Mark-ert’s(2003)algorithm used Dekang Lin’s(1998) clusters of semantically similar words in order to deal with data sparseness.This fact,togetherAcc RT i MBL78.65%65.10%76.0%—Figure1:Accuracy learning curves for the mixed country data with and without semantic informa-tion.in more detail.4Asfigure1indicates,with re-spect to overall accuracy,semantic features have a negative influence:the learning curve with both features climbs much more slowly than that with only grammatical features.Hence,contrary to my expectations,grammatical features seem to allow a better generalization from a limited number of training instances.With respect to the F-score on the metonymical category infigure2,the differ-ences are much less outspoken.Both features give similar learning curves,but semantic features lead to a higherfinal F-score.In particular,the use of semantic features results in a lower precisionfig-ure,but a higher recall score.Semantic features thus cause the classifier to slightly overgeneralize from the metonymic training examples.There are two possible reasons for this inabil-ity of semantic information to improve the clas-sifier’s performance.First,WordNet’s synsets do not always map well to one of our semantic la-bels:many are rather broad and allow for several readings of the target word,while others are too specific to make generalization possible.Second, there is the predominance of prepositional phrases in our data.With their closed set of heads,the number of examples that benefits from semantic information about its head is actually rather small. Nevertheless,myfirst round of experiments has indicated that Memory-Based Learning is a sim-ple but robust approach to metonymy recogni-tion.It is able to replace current approaches that need smoothing or iterative searches through a the-saurus,with a simple,distance-based algorithm.Figure3:Accuracy learning curves for the coun-try data with random and maximum-distance se-lection of training examples.over all possible labels.The algorithm then picks those instances with the lowest confidence,since these will contain valuable information about the training set(and hopefully also the test set)that is still unknown to the system.One problem with Memory-Based Learning al-gorithms is that they do not directly output prob-abilities.Since they are example-based,they can only give the distances between the unlabelled in-stance and all labelled training instances.Never-theless,these distances can be used as a measure of certainty,too:we can assume that the system is most certain about the classification of test in-stances that lie very close to one or more of its training instances,and less certain about those that are further away.Therefore the selection function that minimizes the probability of the most likely label can intuitively be replaced by one that max-imizes the distance from the labelled training in-stances.However,figure3shows that for the mixed country instances,this function is not an option. Both learning curves give the results of an algo-rithm that starts withfifty random instances,and then iteratively adds ten new training instances to this initial seed set.The algorithm behind the solid curve chooses these instances randomly,whereas the one behind the dotted line selects those that are most distant from the labelled training exam-ples.In thefirst half of the learning process,both functions are equally successful;in the second the distance-based function performs better,but only slightly so.There are two reasons for this bad initial per-formance of the active learning function.First,it is not able to distinguish between informativeandFigure4:Accuracy learning curves for the coun-try data with random and maximum/minimum-distance selection of training examples. unusual training instances.This is because a large distance from the seed set simply means that the particular instance’s feature values are relatively unknown.This does not necessarily imply that the instance is informative to the classifier,how-ever.After all,it may be so unusual and so badly representative of the training(and test)set that the algorithm had better exclude it—something that is impossible on the basis of distances only.This bias towards outliers is a well-known disadvantage of many simple active learning algorithms.A sec-ond type of bias is due to the fact that the data has been annotated with a few features only.More par-ticularly,the present algorithm will keep adding instances whose head is not yet represented in the training set.This entails that it will put off adding instances whose function is pp,simply because other functions(subj,gen,...)have a wider variety in heads.Again,the result is a labelled set that is not very representative of the entire training set.There are,however,a few easy ways to increase the number of prototypical examples in the train-ing set.In a second run of experiments,I used an active learning function that added not only those instances that were most distant from the labelled training set,but also those that were closest to it. After a few test runs,I decided to add six distant and four close instances on each iteration.Figure4 shows that such a function is indeed fairly success-ful.Because it builds a labelled training set that is more representative of the test set,this algorithm clearly reduces the number of annotated instances that is needed to reach a given performance.Despite its success,this function is obviously not yet a sophisticated way of selecting good train-76Figure5:Accuracy learning curves for the organi-zation data with random and distance-based(AL) selection of training examples with a random seed set.ing examples.The selection of the initial seed set in particular can be improved upon:ideally,this seed set should take into account the overall dis-tribution of the training examples.Currently,the seeds are chosen randomly.Thisflaw in the al-gorithm becomes clear if it is applied to another data set:figure5shows that it does not outper-form random selection on the organization data, for instance.As I suggested,the selection of prototypical or representative instances as seeds can be used to make the present algorithm more robust.Again,it is possible to use distance measures to do this:be-fore the selection of seed instances,the algorithm can calculate for each unlabelled instance its dis-tance from each of the other unlabelled instances. In this way,it can build a prototypical seed set by selecting those instances with the smallest dis-tance on average.Figure6indicates that such an algorithm indeed outperforms random sample se-lection on the mixed organization data.For the calculation of the initial distances,each feature re-ceived the same weight.The algorithm then se-lected50random samples from the‘most proto-typical’half of the training set.5The other settings were the same as above.With the present small number of features,how-ever,such a prototypical seed set is not yet always as advantageous as it could be.A few experiments indicated that it did not lead to better performance on the mixed country data,for instance.However, as soon as a wider variety of features is taken into account(as with the organization data),the advan-pling can help choose those instances that are most helpful to the classifier.A few distance-based al-gorithms were able to drastically reduce the num-ber of training instances that is needed for a given accuracy,both for the country and the organization names.If current metonymy recognition algorithms are to be used in a system that can recognize all pos-sible metonymical patterns across a broad variety of semantic classes,it is crucial that the required number of labelled training examples be reduced. This paper has taken thefirst steps along this path and has set out some interesting questions for fu-ture research.This research should include the investigation of new features that can make clas-sifiers more robust and allow us to measure their confidence more reliably.This confidence mea-surement can then also be used in semi-supervised learning algorithms,for instance,where the clas-sifier itself labels the majority of training exam-ples.Only with techniques such as selective sam-pling and semi-supervised learning can the knowl-edge acquisition bottleneck in metonymy recogni-tion be addressed.AcknowledgementsI would like to thank Mirella Lapata,Dirk Geer-aerts and Dirk Speelman for their feedback on this project.I am also very grateful to Katja Markert and Malvina Nissim for their helpful information about their research.ReferencesD.W.Aha, D.Kibler,and M.K.Albert.1991.Instance-based learning algorithms.Machine Learning,6:37–66.W.Daelemans and A.Van den Bosch.1992.Generali-sation performance of backpropagation learning on a syllabification task.In M.F.J.Drossaers and A.Ni-jholt,editors,Proceedings of TWLT3:Connection-ism and Natural Language Processing,pages27–37, Enschede,The Netherlands.W.Daelemans,J.Zavrel,K.Van der Sloot,andA.Van den Bosch.2004.TiMBL:Tilburg Memory-Based Learner.Technical report,Induction of Linguistic Knowledge,Computational Linguistics, Tilburg University.D.Fass.1997.Processing Metaphor and Metonymy.Stanford,CA:Ablex.A.Fujii,K.Inui,T.Tokunaga,and H.Tanaka.1998.Selective sampling for example-based wordsense putational Linguistics, 24(4):573–597.R.Hwa.2002.Sample selection for statistical parsing.Computational Linguistics,30(3):253–276.koff and M.Johnson.1980.Metaphors We LiveBy.London:The University of Chicago Press.D.Lin.1998.An information-theoretic definition ofsimilarity.In Proceedings of the International Con-ference on Machine Learning,Madison,USA.K.Markert and M.Nissim.2002a.Metonymy res-olution as a classification task.In Proceedings of the Conference on Empirical Methods in Natural Language Processing(EMNLP2002),Philadelphia, USA.K.Markert and M.Nissim.2002b.Towards a cor-pus annotated for metonymies:the case of location names.In Proceedings of the Third International Conference on Language Resources and Evaluation (LREC2002),Las Palmas,Spain.M.Nissim and K.Markert.2003.Syntactic features and word similarity for supervised metonymy res-olution.In Proceedings of the41st Annual Meet-ing of the Association for Computational Linguistics (ACL-03),Sapporo,Japan.M.Nissim and K.Markert.2005.Learning to buy a Renault and talk to BMW:A supervised approach to conventional metonymy.In H.Bunt,editor,Pro-ceedings of the6th International Workshop on Com-putational Semantics,Tilburg,The Netherlands. G.Nunberg.1978.The Pragmatics of Reference.Ph.D.thesis,City University of New York.M.Osborne and J.Baldridge.2004.Ensemble-based active learning for parse selection.In Proceedings of the Human Language Technology Conference of the North American Chapter of the Association for Computational Linguistics(HLT-NAACL).Boston, USA.J.Pustejovsky.1995.The Generative Lexicon.Cam-bridge,MA:MIT Press.78。
当代大学德语3答案

当代大学德语3答案Studienweg Deutsch Kursbuch 3 H?rtexteLektion 1ü8Helfen, lernen und Abenteuer erlebenStwD: Ist es für Studenten nicht schwierig, arme Familien zu unterstützen? Als Student hat man doch selbst nicht viel Geld.Ge Wenju: Nein,so schwierig ist das nicht. Ich verdiene mit ein paar Nebenjobs ein bisschenGeld. 92 Yuan pro Semester schicke ich einem M?dchen ineinem Dorf in Hebei.Das reicht für die Schulausbildung. Einmal habenwir das Kind nach Beijing eingeladen.StwD: Wie haben Sie denn dieses M?dchen gefunden?Ge Wenju: Immer wieder reisen Leute aus unserem Club in arme Gegenden. V on denen habe ich die Adresse. Die Familie ist wirklich sehr arm. Bei derHeirat haben sie zu vielGeld ausgegeben und bald danach wurde derVater krank und konnte nicht mehr arbeiten. J etzt sammeln sie Früchteund Kr?uter im Wald, die sie dann verkaufen. Im Winter haben sie fastnichts.StwD: Frau Wen, Sie waren also Englischlehrerin.Haben denn Ihre Schüler etwas gelernt?Wen Shizhe: Na ja, das Englischlernenwar sicher nicht das Wichtigste. Viele sagten ganz offen, dass sie mehr zum Zeitvertreib zu uns kamen als um wirklichzu lernen. Gelernt haben vor allemwir Lehrer etwas: über das Lebennach dem Beruf und über die Einsamkeit im Alter.StwD: Herr Ming sprach von der Abenteuerlust. Bei Ihren Radtourenerleben Sie sicher auch Abenteuer, Herr Mi.Mi Tao: Ja, sicher, und auch b?seüberraschungen. Einmal sind wir nach Shidu gefahren, das ist eine wundersch?ne Berglandschaft im Südwesten vonBeijing. 110 Kilometer ging alles gut. Aberdann waren wir in denBergen und es wurde dunkel. Es schien kein Mond undunsere Handysfunktionierten nicht. V on 8 Uhr abends bis ein Uhr nachts irrtenwirdurch die wilde Landschaft, dann erst haben wir ein Dorf gefunden.DasAbenteuer war mir etwas peinlich, denn ich war damals derGruppenleiter.StwD:Sind der Fahrradclub, der Club der guten Herzen und die Bergadler eigentlichgro?e Clubs?Wen Shizhe: Ja, die gr??ten an der Beida. Aber Zhao Yajing und ichsind auch noch in anderen Gruppen aktiv. Sie ist im Hochschulchor, da habensie zumBeispiel das …Halleluja“ von H?ndel gesungen. Ich schreibe für unsereUni-Zeitung. In der Redaktion arbeiten 40 Studentinnen und Studentenmit. Vielleicht will ich sp?ter mal Journalistin werden.上一页下一页Lektion 2ü7KurzdialogeA ◆Entschuldigung, ich h?tte da eine Frage.(M)◇Ja, bitte?(F)◆K?nnten Sie mir bitte erkl?ren, wie diese Kamera funktioniert?◇D a müsste ich auch erst die Gebrauchsanleitung durchlesen.Einen Augenblick, bitte.B ◆Würden Sie mich bitte vorlassen, ich m?chte nur dieses Heft hier kaufen undich muss gleich zum Unterricht.(M)◇Tut mir Leid,ich bin auch sehr in Eile. K?nnten Sie es nicht an der Kasse dort drüben versuchen?(F)C ◆Du, die CD finde ich wirklich toll. K?nntest du sie mir malleihen?(M)◇Gern, aber du müsstest sie mir bald wieder zurückgeben. (M)D◆Dürfte ich Sie bitten, sich hinten anzustellen. Wir waren alle vorIhnenda.(F)◇Ja, aber, ... mein Zug nach Düsseldorf f?hrt in zehn Minuten. (M)◆Ich nehme denselben Zug.◇K?nnten Sie vielleicht auch für mich eine Kartekaufen?◆Gut,das kann ich machen. Sie müssten mir aber gleich das Geld dafür geben.◇Selbstverst?ndlich. - Und herzlichen Dank.上一页下一页Lekiton 3ü8RundfunkdiskussionA Die Stadt ist für die Menschen da, die darin leben, und nicht für Architekten, diemit tollen Sachen berühmt werden wollen. Und da frage ich: Sind 150 Meter hohe Türme menschlich? Wergeht denn gern zwischen Betonk?sten spazieren? Der Mensch braucht einenatürliche Umwelt, viel Grün, B?ume, Wasser. Es muss endlich wieder niedrigerund lockerer gebaut werden. Am Alex sollte ein Anfang gemacht werden.B Nichtsgegen Natur - drau?en auf dem Land, in den Bergen, am Meer. AberSt?dte sindnun einmal nicht natürlich. Da leben viele Menschen auf kleinem Raum zusammen, entwickeln ihre eigene Kultur, arbeiten, kaufen, konsumieren, sind aktiv. Dasmacht das Stadtleben so lebendig. Die Idee mit den Türmen finde ich gro?artig: sachlich, klar, keine überflüssige Romantik. Das entspricht doch genau demCharakter des modernen Menschen.C Ohne Hochh?user geht es nicht, da gebe ichIhnen Recht. Und ehrlich gesagt, ichliebe sch?ne Wolkenkratzer. Aber dieseTürme, das ist doch St?dtebau aus dem frühen 20. Jahrhundert! Die sind ebennicht modern. Nein, das lebendige Stadtleben muss sich auch in lebendigenFormen zeigen, in verschiedenen Formen: spitz, gerade, rund - unterschiedlicheben. Man kann das in Asien sehen, in Hongkong, in Shanghai, in Dubai zumBeispiel. Da wird modern gebaut. Aber natürlich geh?ren Stra?encafés auf denAlex und Restaurants, wo man im Freien essen kann. Leute angucken macht immerSpa?. Und warum nicht auch ein paar B?nke auf den Platz und ein bisschen Grün drum herum?上一页下一页Lektion 4ü9 InterpretationJia Hanfei: Nach meiner Meinung istHans ein froher und glücklicher Mann. Er ist vielleicht nicht sehr intellige nt, aber er tauscht die Sorge um Geld gegen ein leichtes Herz. Das ist doch ganzklug.Yang Xue: Flei?und Ehrlichkeit haben Hans reich gemacht und er h?tte eingutes Leben führen k?nnen. Aber seine Dummheit macht ihn arm. Er ist einschlechter Gesch?ftsmann. Das wichtigste für ihn ist seine Freiheit. Damitillustriert die Geschichte das Sprichwort: Wer mit wenigem zufrieden ist, derist reich.Wei Xing: Hans ist ohne Zweifel dumm und verh?lt sich falsch. Was erhat, findet er nicht so gut wie etwas anderes. Und hat er das, ist er wiederunzufrieden. Immer nach Neuem jagen und das Alte liegen lassen, dabei nichtnachdenken –eine solche Haltung kann sich nicht bezahlt machen. Aber er merktseine Dummheit gar nicht und ist immer froh und glücklich. Es ist eineir onische Geschichte über das Glück.Liu Shanshan: Das Glück von Hans dauert immer nur kurze Zeit. Wenn er mit nichts nach Hause kommt, ist er bestimmtauch nicht lange zufrieden. Aber in Wirklichkeit gibt es so einen Typen garnicht, er passt nicht in die heutige Gesellschaft.Yu Kai: Hans ist einoptimistischer junger Mann, er hat eine positive Einstellung zum Leben und istvoller Hoffnung für die Zukunft. Er will Freiheit und handelt nach Lust undLaune. Vielleicht kann man sein Handeln dumm nennen. Man kann aber auch sagen,dass es ganz vernünftig ist. Gold ist schwer und macht ihn langsam, ein Pferdmacht ihn schneller. Dem Hans kommt es auf den augenblicklichen Nutzen für ihn an. Er kümmert sich nicht um den allgemeinen Wert der Dinge.上一页下一页Lektion 5ü6W?rterbücher für fast jeden ZweckTeil 1…Schlag doch nach!“ ist sicher lich ein guter Rat bei sprachlichen Fragen. Aber wonachschlagen? Reicht der Duden? Und in welchem Duden soll man am besten nachschlagen? Das wichtigste Nachschlagewerk zur deutschen Sprache bestehtn?mlich aus 12 B?nden, wozu auch eine Grammatik, ein Aussprachew?rterbuch,eine Sammlung von Redewendungen und Sprichw?rtern und eine Sammlung mitZitaten und Aussprüchen geh?ren. Au?erdem gibt es ein sehr nützlichesW?rterbuch, das besonderen Schwierigkeiten der deutschen Sprache erkl?rt.Dieser Dudenband hei?t Richtiges und gutes Deutsch. W?rterbuch dersprachlichen Zweifelsf?lle.Teil 2Auf keinem Schreibtisch sollte ein aktuellesFremdw?rterbuch fehlen. Auf Fremdw?rter st??t man in vielen Texten und geradein diesem Bereich ver?ndert sich die Sprache schnell und vieles findet mannicht in den allgemeinen W?rterbüchern. Wichtig ist ein Fremdw?rterbuchbesonders für das Verstehen fachsprachlicher Texte. Wenn man einen deutschenText schreibt, versucht man W?rter nicht zu wiederholen; man sucht andereW?rter mit einer ?hnlichen Bedeutung, sogenannte Synonyme. Die findet man ineinem Synonymw?rterbuch. Durchschnittlich 15 Synonyme für 20 000 Stichw?rtergibt der Synonym-Duden an, der sich …W?rterbuch sinnverwandter W?rter“ nennt. Denn Synonyme sind nie ganz gleich in ihrer Bedeutung, sondern nur verwandt,bedeutungs-, sinn- und sachverwandt. Um genau den passenden Ausdruck, dastreffende Wort, zu finden, schauen auch Schriftsteller in Synonymw?rterbüch ern nach.Aber was tun, wenn man überhaupt kein Wort hat, nicht wei?, wie zumBeispiel ein bestimmtes Ding an einer Maschine hei?t? Da helfen nur Bilder,die das Ding zeigen, und neben den Bildern muss der Begriff stehen.Bildw?rterbücher hei?en diese Nachs chlagewerke, in denen man vor allem W?rterfür konkrete Gegenst?nde findet.上一页下一页Lektion 6ü4Mensch, ?rgere dich nicht!A Was soll denn das nunwieder? Fehlermeldung? Kann nicht sein. Also noch malvon vorn. – CD einlegen. So. Und jetzt Setup. O.k. Weiter anklicken. Noch mal Weiter. –Was? Schon wieder Error? So ein Mist. Wahrscheinlich ist die CD kaputt. Da muss ich mirdas Programm morgen noch mal überspielen.B Was braucht denn der heute so lang?Meint wohl ich kann ewig warten. Ich willjetzt ins Internet! L os, wird’s bald? (Klopfen mit der Maus.) Bl?de Kiste.Anschluss her, aber schnell. (st?rkeresKlopfen) Immer noch ni cht? Jetzt reicht’s mir aber. (Faustschl?ge) Wie? Was? Nein, das kann doch nicht wahr sein!Abgestürzt? Also, ... (starke Faustschl?ge)Das mach ich nicht mehr mit!Glaubt wohl ... Was? Bild auch weg. Ohne mich,mein Lieber! (Krach)C Na, was ist denn heute los mit dir? Komm doch, lade mirendlich die Datei. Magstdu nicht? Na ja, hast wahrscheinlich zu viel auf derPlatte. Also, erst mal was l?schen. – So, und jetzt sei bitte lieb. – Immer noch nicht? Was? Abgestürzt? Na gut, kann jedem mal passieren. Fahren wir dicherst mal runter上一页下一页Lektion 7ü2 Wetterbericht1. Bei Tiefsttemperaturen von 13 Gradim Nordosten wird es insgesamt w?rmer. Inder n?rdlichen Landesh?lfte liegendie Temperaturen bei etwa 16 Grad, im Süden und Westen bei 20 bis 21 Grad. Im Norden und Osten ist es bew?lkt, nur manchmal scheint die Sonne, im Westen undim Alpengebiet ist es überwiegend heiter, im Südwesten klar. Es bleibt trocken, nur in den ?stlichen Mittelgebirgen kann es ?rtlich zu Regen kommen.2. Der Tagwird im gesamten V orhersagegebiet freundlich und frühlingshaftwarm.überwiegend scheint die Sonne, nur vereinzelt treten Wolken auf. DieTemperaturen liegen bei über 19 Grad im Westen bis 23 Grad im Osten.3. Imganzen Landesgebiet herrscht trübes Wetter. Im Norden ist der Himmelbedecktund es regnet, sonst ist es bew?lkt, es kann zu Regen und Schauern kommen. Die Temperaturen liegen bei 16 bis 18 Grad, in den Bergen bei 9 bis 10 Grad. Nuran der Südseite der Berge werden Temperaturen von über 20 Grad erreicht.ü10 Wie ist das Wetter?1. A: So kannst du doch nicht ins Büro gehen. Du bist vielzu leicht angezogen.B: Schau doch zum Fenster raus: Blauer Himmel undstrahlende Sonne.A: Nach dem Wetterbericht gibt’s Frost, auf zehn Grad unterNull sollen die Temperaturen heute fallen.B: Hast recht. Da muss manvorsichtig sein.2. A: H?rst du, es donnert?B: Ja, aber ich glaube nicht, dassdas Gewitter bis zu uns kommt. Der Donner ist noch ganz weit im Osten und derWind weht aus Westen.A: Na ja, aber mit etwas Regen muss man schon rechnen.3.A: Riedel.B: Hallo, Inge, hier Monika.A: Ach, grü?dich, Monika. Ich wolltedich auch gerade anrufen. Du kannst heute wohl nicht kommen? Bei dem Wetter.B:Nee. Bei mir im Garten steht das Wasser schon fünf Zentimeter hoch.A: Oje!Eine richtige überschwemmung. Hoffentlich geht nichts kaputt. Was hat denn derWetterbericht gesagt?B: Der Regen würde in der Nacht aufh?ren und heute sei Sonnenschein.上一页下一页A: Na, hoffentlich stimmt’s wenigstens für den Nachmittag. Mach’s gut. Und Tschüss.B: Tschüss.4. Und nun die Wettervorhersage für morgen, Freitag, den 13. Mai. Weiterhinstürmische Winde aus West, Nordwest. Keine Niederschl?ge. Für die Jahreszeit zu ka lt.5. Achtung, Achtung! Eine Durchsagedes Umweltamtes. Smogalarm im RaumK?ln-Bonn. Die Bürger werden gebeten, in den Wohnungen zu bleiben und die Fenster zu schlie?en. Privatautos dürfen nurin ?u?erst dringenden F?llen benutzt werden. Da auch im Laufe des Tages keinAufkommen von Wind zu erwarten ist, kann heute mit keiner Verbesserung derLuftverh?ltnisse gerechnet werden. –Achtung, Achtung! Eine Durchsage ...6. A:Wieder 35 Grad heute! Kaum auszuhalten!B: Findest du? Mir kann es gar nichtwarm genug sein. Jeden Tag gehe ich baden.A: Hast du denn Urlaub?B: Ja, ichmache Urlaub hier zu Hause bei sch?nstem Wetter.C: Na ja, sch?n ist relativ.Ich muss n?mlich arbeiten bei dieser Hitze.上一页下一页Lektion 8ü1V orhersagenText 1Tageshoroskop: Jungfrau untergutem SternBerufIm Job stehen Jungfrauen vor wichtigen Entscheidungen. Z?gernSie nicht lange und Sie werden Erfolg haben. Helfen Sie weniger erfolgreichenKollegen und Mitarbeitern. Man wird sich daran erinnern, wenn es mal für Sie nicht ganz so gut l?uft.Liebe und FreundschaftImmer noch allein? Das kannheute anders werden. Nur Mut! Nehmen Sie Kontakt zu den Menschen auf, für dieSie sich schon lange interessieren. Vielleicht wird es eine Partnerschaft fürs Leben werden. Heute ist alles m?glich.Gesundheit und FitnessSie sind fit undbei bester Gesundheit. Joggen Sie, machen Sie Spazierg?nge. Ihr gutesK?rpergefühl l?sst Sie attraktiv und selbstbewusst wirken.Text 2Aus demPolizeibericht: Teurer GlaubeEinen besonders glaubwürdigen Eindruck hat eine Wahrsagerin auf einen Ladenbesitzer in Soest gemacht. Nachdem der Gewinnseiner Boutique in den vergangenen Wochen sehr gesunken war, lie? er sich vonder Frau überzeugen, dass das Gesch?ft unter ungünstigen Einflüssen h?herer M?chte stehe. Diese verl?ren ihre Kraft, wenn sie dreizehnmal mit 1313 Euro inder Hand um das Haus laufe. Der leichtgl?ubige Gesch?ftsmann gab derWahrsagerin das Geld und sah es ebensowenig wieder wie die Frau. Die Polizeibittet um Hinweise auf ?hnliche Hilfsangebote von einer ca. 45-j?hrigen Fraumit blondem Haar. Die Betrügerin sagt, sie k?nne aus der Hand lesen, in einerKugel in die Zukunft sehen und das Schicksal beeinflussen.Text 3 Zwei in einem:Büro und Musik in der HosentascheEine neue Generation von Mobiltelefonen wirdnach Ansicht von Microsoft-Chef Steve Ballmer auch die Funktion vonMusikplayern übernehmen. …In der Zukunft der tragbaren Ger?te werden wirviele Ver?nderungen sehen“, sagte Ballmer auf einem Management-Forum in Berlin. Das Handy sei ja schon l?ngst nicht nur Telefon, der Trend gehe ineine Richtung, bei dem der Handy-Besitzer die wichtigsten Funktionen einesBürocomputers nutzen kann. Und das Ganze bei Musik.上一页下一页Lektion 9ü8Eine Afanti-Geschichte: Der Beamte und das BootEinmal kam der Efendi an einen Fluss. Dort wartete schon ein hoher Beamterund zusammen nahmen sie ein Boot, um über den Fluss zu fahren. Es war aber daserste Mal, dass der Beamte in einem Boot sa? und in der Mitte des Flusseswurde er ganz grün im Gesicht. Er klammerte sich an den Efendi undjammerte: …Nasredin, mein lieber Nasredin, ich fürchte mich so. Wei?t du denn kein Mittel gegen meine Angst?“…Ein Mittel gegen deine Angst?“, sagte der Efendi, …Nun das wüsste ich schon. Aber ich glaube nicht, dass es Ihnengefallen würde.“…Doch, doch, ich bin sch on e inverstanden, schnell tuendlich et was für mich!“…Wie Sie wünschen“, sagte der Efendi, packte den Beamten und warf ihn ins Wasser. Der konnte natürlich nicht schwimmen. DerEfendi wartete eine Weile, bis er ihn wieder ins Boot holte.…Na?“, fragte er,…Wie fühlen Sie sich nun?“…Besser, es geht mir schon viel besser“, sagte der Beamte.…Sehen Sie“, sagte Nasredin, …so ist das nun mal: Wer niezu Fu? gehen musste, kennt nicht den Wert eines Pferdes. Wer nie ins Wassergefallen ist, kennt nicht die V orteile eines Boots. Und ein Beamter, derjeden Tag das beste Essen bekommt, lernt nie, wie sch?n es ist, wenn manüberhaupt etwas zu essen hat.“上一页下一页Lektion 10ü6Keine Standardgespr?che1. In der Wohngemeinschaft: Student A, Studentin BA: Wo ist denn mein Tennisschl?ger?B: Keine Ahnung.A: Duleihst ihn dir doch immer aus – ohne zu fragen.B: Schon eine Ewigkeit nichtmehr.A: Und gesehen haste ihn auch nicht?B: O.k. ich guck mal nach. – Oh, da ist er ja. Entschuldige.A: Du und dein Chaos.2. Auf der Stra?e: Autofahrerin A,Passant BA: Verzeihen Sie bitte, dürfte ich Sie etwas fragen?B: Aber bittegern, wenn ich Ihnen behilflich sein kann.A: K?nnten Sie mir vielleicht sagen,wie ich zum Parkhotel komme?B: Nun, da w?re es wohl am besten, Sie n?hmen dien?chste Stra?e recht s und bei der dritten Ampel müssten Sie ... Oh, das wirdein bisschen kompliziert. Ach wissen Sie, sie k?nnten hinter mir her fahren.A:Ich m?chte Ihnen aber keine Ungelegenheiten bereiten.B: Aber nein, keineswegs,ich muss auch in diese Richtung. Da vorn steht mein Wagen, der dunkelblaueMercedes. Sie brauchen mir nur zu folgen.A: Das ist aber sehr liebenswürdig von Ihnen.B: Keine Ursache. Nur einen Augenblick Geduld noch.A: Vielenherzlichen Dank.3. Auf einer Party Junger Mann A, junge Frau BA: Tanzen wir?B:Null Bock auf Tanzen.A: Ach, sei doch nicht so langweilig.B: Bin nichtlangweilig, hab blo? keine Lust.A: Biste sauer?B: Wieso soll ich sauer sein?Aber warum quatschste nicht deine Freundin Anna an?Die ist bestimmt ganz happy,wenn du mit ihr tanzt.A: O.k., dann eben nicht.上一页下一页Lektion 11ü6Tagesablauf im KindergartenIm Kindergarten liefder Tag nach festen Regeln ab, fast wie bei den Soldaten. Einfach tun, wozuman gerade Lust hatte, das gab es nicht. Einen freien Willen auchnicht.Morgens um 7 sind wir aufgestanden. Dann hatten wir zwanzig Minuten Zeitzum Waschen, K?mmen, Z?hneputzen, anschlie?end machten wir Morgensport. Umacht gab es Frühstück. Um neun mussten wir uns alle an der Hand nehmen, dann wurden wir spazieren geführt, vielleicht eine hal be oder eine ganze Stunde. Wenn wir zurückkamen, spielten wir oder wir malten. Um halb zw?lf gab esMittagessen, das dauerte ungef?hr bis halb eins. Danach mussten wir schlafen.Erst um drei durften wir wieder aufstehen, wir haben uns gewaschen und um halbvier gab es Milch und etwas Sü?es. Dann durften wir manchmal für eine Stunde drau?en spielen. Um sechs gab es Abendessen, danach, etwa um sieben, haben wir gesungen und dazu in die H?nde geklatscht. Um acht mussten wir uns noch einmalwaschen und sollten sofort wieder schlafen, auch wenn noch kein Kind müde war. Wir mussten viel zu viel schlafen.ü12Fernglas statt RollschuheIII.Rollschuhehabe ich mir gewünscht und war mir sehr, sehr sicher, dass ich sie bekommenwürde. Warum auch nicht? Ein ganz normaler W unsch zum zw?lften Geburtstag, schlie?lich habe ich ja nicht um ein Pferd gebeten. Dann kam eine Torte mitKerzen und der gro?e Schreck: Ein Buch mit dem Titel …Die V?gel unserer Wiesen und W?lder“, daneben Kassetten aus der Reihe …Die Stimmen der V?gel unserer Wiesen und W?lder“ und ein riesiges Fernglas. Keine Rollschuhe. Ichh?tte heulen k?nnen. Schuld an allem war mein Vater. Seit seiner Jugend warenV?gel sein Hobby und ich sollte seine Leidenschaft teilen. Und dann, dieAusflüge in den Wald mit Leber wurstbroten, das Warten auf die V?gel: Nichtskonnte langweiliger sein. Und doch war es gut, dass ich meinen Vater und seine Leberwurstbrote in dieser Zeit für mich allein hatte.Frauke Finsterwalder上一页下一页Lektion 12ü7Was war da los?1.A: Guten Morgen.B: Mor gen, Herr Kramer, wie geht’s?A: Ganz gut, danke. – Ach, es tut mir sehr leid, aber dakamen Herren von einer Firma und die haben Ihren Parkplatz besetzt. Sie h?tteneinen Termin bei Ihnen, sagten sie. Ich wollte nichts sagen. Ich wusste janicht, wie wichtig die Leute sind.B: Aha, einen Termin bei mir?Firmenvertreter? Na ja. mal sehen. – Aber macht nichts, ich habe meinen Wagen dort hinten geparkt. Würden Sie ihn bitte auf meinen Parkplatz stellen, wenndie Besucher weg sind? Hier der Schlüssel.A: Mach ich. Und nochmals Entschuldigung.B: Kein Problem, Herr Kramer, da konnten Sie wohl nichts machen.Einen sch?nen Tag noch.2.A: Moritz.B: Guten Tag, Weber. Ich rufe wegen derWohnung im Tagesspiegel an.A: Ach, ja. Guten Tag.B: Ist die Wohnung noch zuhaben?A: Ja. Es haben schon einige Interessenten angerufen, aber ich habe sienoch nicht vermietet. B: K?nnte ich sie mir mal anschauen?A: Gern. Aber,Frau ...B: Weber.A: Ja, Frau Weber, damit Sie nicht umsonst kommen. Ich m?chtekeine Katzen und Hunde im Haus haben. Also, wenn Sie Haustiere haben ...B:Haben wir nicht.A: Sie, - Sie sind nicht alleinstehend?B: Nein, verheiratet.–Und da habe ich auch gleich eine Frage: Sie haben doch nichts gegen Kinder?A:Kinder? Wie viele denn?B: Noch keins. Aber in fünf, sechs Mon aten ist es soweit.A: Nein, gegen ein Kind kann ich natürlich nichts haben. Hat Ihr Mann eigentlich eine feste Stelle?B: Wir sind beide berufst?tig.A: Gut, - ja, gut,schauen Sie sich die Wohnung doch mal an.B: K?nnten wir heute Abendvorbeikommen, so gegen halb sechs?A: Ja, ich bin den ganzen Abend da. Ichwohne im Haus.B: Es ist doch im Wedding?A: Ja, Freienwalderstra?e 7. KlingelnSie bitte im ersten Stock bei Moritz.B: Sch?n, Frau Moritz, erst mal vielenDank.A: Bis heute Abend, Frau Weber. Auf Wiederh?ren.B: Auf Wiederh?ren.上一页下一页ü11 Rundfunkreportage: …Schüler k?nnen nicht mehrDeutsch“Nicht nur ausl?ndische Jugendliche haben Schwierigkeiten mit derdeutschen Sprache –sondern immer ?fter auch die deutschen. H?ren Sie zun?chstein Beispiel. Ich sprach mit der sechsj?hrigen Kerstin.Reporterin: Na, Kerstin,wie alt bist du denn?Kerstin: Sechs.Reporterin: Und gehst du schon zurSchule?Kerstin: Nee.Reporterin: Ja, bist du dann noch im Kindergarten?Kerstin:Nee, in V or... – bin ein V or... – V orkind.Reporterin: So, du bist ein V orschulkind, du gehst in die V orschule.Kerstin: Genau.Reporterin: Und waslernst du in der V orschule?Kerstin: Wei? nicht.Reporterin: Ja, was macht ihrdenn da?Kerstin: Gestern fahrten wir Stra?enbahn.Reporterin: Aha. Wohindenn?Ke rstin: In ′n Zoo, zu die Elefanten und Giraffen.Kerstins Bruder Marioist schon zw?lf, geht aber noch in die fünfte Klasse. Sitzengeblieben, auchwegen einer Fünf in Deutsch. Diktat und Aufsatz, liegen ihm nicht, sagt er. Ober schon mal ein Buch gelesen h at? Ja schon, aber nur Bücher mit Comic-Bildern und Sprechblasen. Seine Eltern, sagt er, lesen auch nicht.Kerstin und Mariosind nur zwei von Millionen Schülern mit Sprachproblemen. ...上一页下一页。
大学德语考试试题及答案
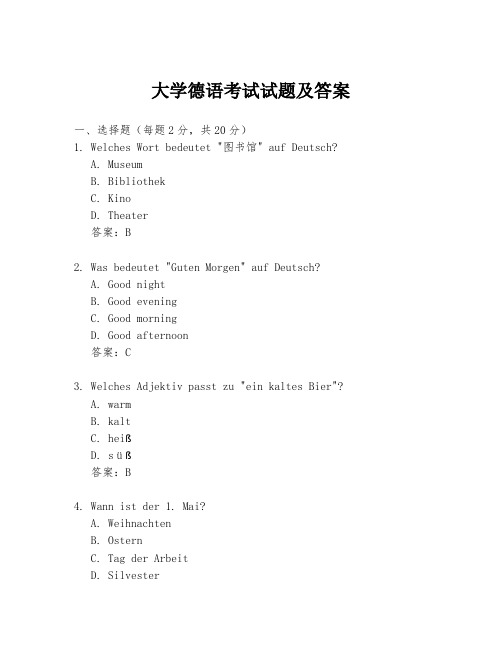
大学德语考试试题及答案一、选择题(每题2分,共20分)1. Welches Wort bedeutet "图书馆" auf Deutsch?A. MuseumB. BibliothekC. KinoD. Theater答案:B2. Was bedeutet "Guten Morgen" auf Deutsch?A. Good nightB. Good eveningC. Good morningD. Good afternoon答案:C3. Welches Adjektiv passt zu "ein kaltes Bier"?A. warmB. kaltC. heißD. süß答案:B4. Wann ist der 1. Mai?A. WeihnachtenB. OsternC. Tag der ArbeitD. Silvester答案:C5. Welches Präfix bedeutet "un-" auf Deutsch?A. un-B. ent-C. er-D. ver-答案:A6. Welche Stadt ist die Hauptstadt von Deutschland?A. MünchenB. FrankfurtC. BerlinD. Hamburg答案:C7. Was bedeutet "Ich habe Hunger"?A. I am hungryB. I am thirstyC. I am tiredD. I am happy答案:A8. Welches Verb bedeutet "to read" auf Deutsch?A. schreibenB. sprechenC. lesenD. hören答案:C9. Welches Substantiv passt zu "das Brot"?A. der TischB. der StuhlC. das BrotD. der Tee答案:C10. Welches Adjektiv passt zu "ein rotes Kleid"?A. rotB. blauC. grünD. gelb答案:A二、填空题(每空1分,共20分)1. Das Wort "Buch" bedeutet ________ auf Deutsch.答案:book2. Der 24. Dezember ist ein wichtiger Tag, weil es ________ ist.答案:Heiliger Abend3. Die deutsche Flagge hat drei Farben: Schwarz, Rot und________.答案:Gold4. "Ich lerne Deutsch" bedeutet "I am learning ________".答案:German5. Der deutsche Begriff für "computer" ist ________.答案:Computer6. "Guten Tag" ist eine Höflichkeitsform, die man am________ Tag sagt.答案:Tag7. Die deutsche Hauptstadt ist ________.答案:Berlin8. Der deutsche Begriff für "teacher" ist ________.答案:Lehrer9. "Ich mag Schokolade" bedeutet "I like ________".答案:chocolate10. Die deutsche Flagge hat die Farben Schwarz, Rot und________.答案:Gold三、阅读理解(每题4分,共20分)阅读以下短文,并回答问题。
The Cross-Section of Volatility and Expected Returns
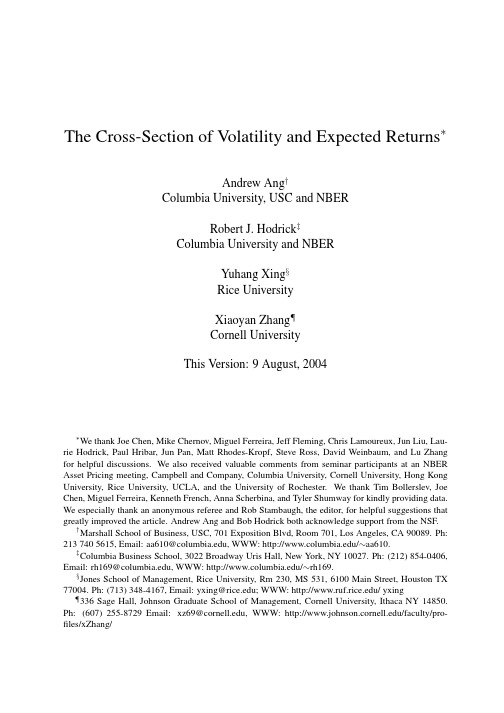
The Cross-Section of V olatility and Expected Returns∗Andrew Ang†Columbia University,USC and NBERRobert J.Hodrick‡Columbia University and NBERYuhang Xing§Rice UniversityXiaoyan Zhang¶Cornell UniversityThis Version:9August,2004∗We thank Joe Chen,Mike Chernov,Miguel Ferreira,Jeff Fleming,Chris Lamoureux,Jun Liu,Lau-rie Hodrick,Paul Hribar,Jun Pan,Matt Rhodes-Kropf,Steve Ross,David Weinbaum,and Lu Zhang for helpful discussions.We also received valuable comments from seminar participants at an NBER Asset Pricing meeting,Campbell and Company,Columbia University,Cornell University,Hong Kong University,Rice University,UCLA,and the University of Rochester.We thank Tim Bollerslev,Joe Chen,Miguel Ferreira,Kenneth French,Anna Scherbina,and Tyler Shumway for kindly providing data. We especially thank an anonymous referee and Rob Stambaugh,the editor,for helpful suggestions that greatly improved the article.Andrew Ang and Bob Hodrick both acknowledge support from the NSF.†Marshall School of Business,USC,701Exposition Blvd,Room701,Los Angeles,CA90089.Ph: 2137405615,Email:aa610@,WWW:/∼aa610.‡Columbia Business School,3022Broadway Uris Hall,New York,NY10027.Ph:(212)854-0406, Email:rh169@,WWW:/∼rh169.§Jones School of Management,Rice University,Rm230,MS531,6100Main Street,Houston TX 77004.Ph:(713)348-4167,Email:yxing@;WWW:/yxing ¶336Sage Hall,Johnson Graduate School of Management,Cornell University,Ithaca NY14850. Ph:(607)255-8729Email:xz69@,WWW:/faculty/pro-files/xZhang/AbstractWe examine the pricing of aggregate volatility risk in the cross-section of stock returns. Consistent with theory,wefind that stocks with high sensitivities to innovations in aggregate volatility have low average returns.In addition,wefind that stocks with high idiosyncratic volatility relative to the Fama and French(1993)model have abysmally low average returns. This phenomenon cannot be explained by exposure to aggregate volatility risk.Size,book-to-market,momentum,and liquidity effects cannot account for either the low average returns earned by stocks with high exposure to systematic volatility risk or for the low average returns of stocks with high idiosyncratic volatility.1IntroductionIt is well known that the volatility of stock returns varies over time.While considerable research has examined the time-series relation between the volatility of the market and the expected re-turn on the market(see,among others,Campbell and Hentschel(1992),and Glosten,Jagan-nathan and Runkle(1993)),the question of how aggregate volatility affects the cross-section of expected stock returns has received less attention.Time-varying market volatility induces changes in the investment opportunity set by changing the expectation of future market returns, or by changing the risk-return trade-off.If the volatility of the market return is a systematic risk factor,an APT or factor model predicts that aggregate volatility should also be priced in the cross-section of stocks.Hence,stocks with different sensitivities to innovations in aggregate volatility should have different expected returns.Thefirst goal of this paper is to provide a systematic investigation of how the stochastic volatility of the market is priced in the cross-section of expected stock returns.We want to de-termine if the volatility of the market is a priced risk factor and estimate the price of aggregate volatility risk.Many option studies have estimated a negative price of risk for market volatil-ity using options on an aggregate market index or options on individual stocks.1Using the cross-section of stock returns,rather than options on the market,allows us to create portfolios of stocks that have different sensitivities to innovations in market volatility.If the price of ag-gregate volatility risk is negative,stocks with large,positive sensitivities to volatility risk should have low average ing the cross-section of stock returns also allows us to easily con-trol for a battery of cross-sectional effects,like the size and value factors of Fama and French (1993),the momentum effect of Jegadeesh and Titman(1993),and the effect of liquidity risk documented by P´a stor and Stambaugh(2003).Option pricing studies do not control for these cross-sectional risk factors.Wefind that innovations in aggregate volatility carry a statistically significant negative price of risk of approximately-1%per annum.Economic theory provides several reasons why the price of risk of innovations in market volatility should be negative.For example,Campbell (1993and1996)and Chen(2002)show that investors want to hedge against changes in mar-ket volatility,because increasing volatility represents a deterioration in investment opportuni-ties.Risk averse agents demand stocks that hedge against this risk.Periods of high volatility also tend to coincide with downward market movements(see French,Schwert and Stambaugh (1987),and Campbell and Hentschel(1992)).As Bakshi and Kapadia(2003)comment,assets 1See,among others,Jackwerth and Rubinstein(1996),Bakshi,Cao and Chen(2000),Chernov and Ghysels (2000),Burashi and Jackwerth(2001),Coval and Shumway(2001),Benzoni(2002),Jones(2003),Pan(2002), Bakshi and Kapadia(2003),Eraker,Johannes and Polson(2003),and Carr and Wu(2003).with high sensitivities to market volatility risk provide hedges against market downside risk. The higher demand for assets with high systematic volatility loadings increases their price and lowers their average return.Finally,stocks that do badly when volatility increases tend to have negatively skewed returns over intermediate horizons,while stocks that do well when volatil-ity rises tend to have positively skewed returns.If investors have preferences over coskewness (see Harvey and Siddique(2000)),stocks that have high sensitivities to innovations in market volatility are attractive and have low returns.2The second goal of the paper is to examine the cross-sectional relationship between id-iosyncratic volatility and expected returns,where idiosyncratic volatility is defined relative to the standard Fama and French(1993)model.3If the Fama-French model is correct,forming portfolios by sorting on idiosyncratic volatility will obviously provide no difference in average returns.Nevertheless,if the Fama-French model is false,sorting in this way potentially provides a set of assets that may have different exposures to aggregate volatility and hence different aver-age returns.Our logic is the following.If aggregate volatility is a risk factor that is orthogonal to existing risk factors,the sensitivity of stocks to aggregate volatility times the movement in aggregate volatility will show up in the residuals of the Fama-French model.Firms with greater sensitivities to aggregate volatility should therefore have larger idiosyncratic volatilities relative to the Fama-French model,everything else being equal.Differences in the volatilities offirms’true idiosyncratic errors,which are not priced,will make this relation noisy.We should be able to average out this noise by constructing portfolios of stocks to reveal that larger idiosyncratic volatilities relative to the Fama-French model correspond to greater sensitivities to movements in aggregate volatility and thus different average returns,if aggregate volatility risk is priced.While high exposure to aggregate volatility risk tends to produce low expected returns,some economic theories suggest that idiosyncratic volatility should be positively related to expected returns.If investors demand compensation for not being able to diversify risk(see Malkiel and Xu(2002),and Jones and Rhodes-Kropf(2003)),then agents will demand a premium for holding stocks with high idiosyncratic volatility.Merton(1987)suggests that in an information-segmented market,firms with largerfirm-specific variances require higher average returns to compensate investors for holding imperfectly diversified portfolios.Some behavioral models, 2Bates(2001)and Vayanos(2004)provide recent structural models whose reduced form factor structures have a negative risk premium for volatility risk.3Recent studies examining total or idiosyncratic volatility focus on the average level offirm-level volatility. For example,Campbell,Lettau,Malkiel and Xu(2001),and Xu and Malkiel(2003)document that idiosyncratic volatility has increased over time.Brown and Ferreira(2003)and Goyal and Santa-Clara(2003)argue that id-iosyncratic volatility has positive predictive power for excess market returns,but this is disputed by Bali,Cakici, Yan and Zhang(2004).like Barberis and Huang(2001),also predict that higher idiosyncratic volatility stocks should earn higher expected returns.Our results are directly opposite to these theories.Wefind that stocks with high idiosyncratic volatility have low average returns.There is a strongly significant difference of-1.06%per month between the average returns of the quintile portfolio with the highest idiosyncratic volatility stocks and the quintile portfolio with the lowest idiosyncratic volatility stocks.In contrast to our results,earlier researchers either found a significantly positive relation between idiosyncratic volatility and average returns,or they failed tofind any statistically sig-nificant relation between idiosyncratic volatility and average returns.For example,Lintner (1965)shows that idiosyncratic volatility carries a positive coefficient in cross-sectional regres-sions.Lehmann(1990)alsofinds a statistically significant,positive coefficient on idiosyncratic volatility over his full sample period.Similarly,Tinic and West(1986)and Malkiel and Xu (2002)unambiguouslyfind that portfolios with higher idiosyncratic volatility have higher av-erage returns,but they do not report any significance levels for their idiosyncratic volatility premiums.On the other hand,Longstaff(1989)finds that a cross-sectional regression coeffi-cient on total variance for size-sorted portfolios carries an insignificant negative sign.The difference between our results and the results of past studies is that the past literature either does not examine idiosyncratic volatility at thefirm level or does not directly sort stocks into portfolios ranked on this measure of interest.For example,Tinic and West(1986)work only with20portfolios sorted on market beta,while Malkiel and Xu(2002)work only with 100portfolios sorted on market beta and size.Malkiel and Xu(2002)only use the idiosyncratic volatility of one of the100beta/size portfolios to which a stock belongs to proxy for that stock’s idiosyncratic risk and,thus,do not examinefirm-level idiosyncratic volatility.Hence,by not di-rectly computing differences in average returns between stocks with low and high idiosyncratic volatilities,previous studies miss the strong negative relation between idiosyncratic volatility and average returns that wefind.The low average returns to stocks with high idiosyncratic volatilities could arise because stocks with high idiosyncratic volatilities may have high exposure to aggregate volatility risk, which lowers their average returns.We investigate this issue andfind that this is not a complete explanation.Our idiosyncratic volatility results are also robust to controlling for value,size, liquidity,volume,dispersion of analysts’forecasts,and momentum effects.Wefind the effect robust to different formation periods for computing idiosyncratic volatility and for different holding periods.The effect also persists in both bull and bear markets,recessions and expan-sions,and volatile and stable periods.Hence,our results on idiosyncratic volatility represent a substantive puzzle.The rest of this paper is organized as follows.In Section2,we examine how aggregate volatility is priced in the cross-section of stock returns.Section3documents thatfirms with high idiosyncratic volatility have very low average returns.Finally,Section4concludes.2Pricing Systematic Volatility in the Cross-Section2.1Theoretical MotivationWhen investment opportunities vary over time,the multi-factor models of Merton(1973)and Ross(1976)show that risk premia are associated with the conditional covariances between as-set returns and innovations in state variables that describe the time-variation of the investment opportunities.Campbell’s(1993and1996)version of the Intertemporal CAPM(I-CAPM) shows that investors care about risks from the market return and from changes in forecasts of future market returns.When the representative agent is more risk averse than log utility,assets that covary positively with good news about future expected returns on the market have higher average returns.These assets command a risk premium because they reduce a consumer’s abil-ity to hedge against a deterioration in investment opportunities.The intuition from Campbell’s model is that risk-averse investors want to hedge against changes in aggregate volatility because volatility positively affects future expected market returns,as in Merton(1973).However,in Campbell’s set-up,there is no direct role forfluctuations in market volatility to affect the expected returns of assets because Campbell’s model is premised on homoskedastic-ity.Chen(2002)extends Campbell’s model to a heteroskedastic environment which allows for both time-varying covariances and stochastic market volatility.Chen shows that risk-averse in-vestors also want to directly hedge against changes in future market volatility.In Chen’s model, an asset’s expected return depends on risk from the market return,changes in forecasts of future market returns,and changes in forecasts of future market volatilities.For an investor more risk averse than log utility,Chen shows that an asset that has a positive covariance between its return and a variable that positively forecasts future market volatilities causes that asset to have a lower expected return.This effect arises because risk-averse investors reduce current consumption to increase precautionary savings in the presence of increased uncertainty about market returns.Motivated by these multi-factor models,we study how exposure to market volatility risk is priced in the cross-section of stock returns.A true conditional multi-factor representation of expected returns in the cross-section would take the following form:r i t+1=a it+βim,t(r mt+1−γm,t)+βiv,t(v t+1−γv,t)+Kk=1βik,t(f k,t+1−γk,t),(1)where r it+1is the excess return on stock i,βim,tis the loading on the excess market return,βiv,tis the asset’s sensitivity to volatility risk,and theβik,tcoefficients for k=1...K representloadings on other risk factors.In the full conditional setting in equation(1),factor loadings, conditional means of factors,and factor premiums potentially vary over time.The model inequation(1)is written in terms of factor innovations,so r mt+1−γm,t represents the innovation in the market return,v t+1−γv,t represents the innovation in the factor reflecting aggregate volatility risk,and innovations to the other factors are represented by f k,t+1−γk,t.The conditional mean of the market and aggregate volatility are denoted byγm,t andγv,t,respectively,while the conditional mean of the other factors are denoted byγk,t.In equilibrium,the conditional mean of stock i is given by:a i t =E t(r it+1)=βim,tλm,t+βiv,tλv,t+Kk=1βik,tλk,t,(2)whereλm,t is the price of risk of the market factor,λv,t is the price of aggregate volatility risk, and theλk,t are prices of risk of the other factors.Note that only if a factor is traded is the conditional mean of a factor equal to its conditional price of risk.The main prediction from the factor model setting of equation(1)that we examine is that stocks with different loadings on aggregate volatility risk have different average returns.4How-ever,the true model in equation(1)is infeasible to examine because the true set of factors is unknown and the true conditional factor loadings are unobservable.Hence,we do not attempt to directly use equation(1)in our empirical work.Instead,we simplify the full model in equation (1),which we now detail.2.2The Empirical FrameworkTo investigate how aggregate volatility risk is priced in the cross-section of equity returns we make the following simplifying assumptions to the full specification in equation(1).First,we use observable proxies for the market factor and the factor representing aggregate volatility risk. We use the CRSP value-weighted market index to proxy for the market factor.To proxy innova-tions in aggregate volatility,(v t+1−γv,t),we use changes in the V IX index from the Chicago 4While an I-CAPM implies joint time-series as well as cross-sectional predictability,we do not examine time-series predictability of asset returns by systematic volatility.Time-varying volatility risk generates intertemporal hedging demands in partial equilibrium asset allocation problems.In a partial equilibrium setting,Liu(2001)and Chacko and Viceira(2003)examine how volatility risk affects the portfolio allocation of stocks and risk-free assets, while Liu and Pan(2003)show how investors can optimally exploit the variation in volatility with options.Guo and Whitelaw(2003)examine the intertemporal components of time-varying systematic volatility in a Campbell (1993and1996)equilibrium I-CAPM.Board Options Exchange(CBOE).5Second,we reduce the number of factors in equation(1) to just the market factor and the proxy for aggregate volatility risk.Finally,to capture the con-ditional nature of the true model,we use short intervals,one month of daily data,to take into account possible time-variation of the factor loadings.We discuss each of these simplifications in turn.Innovations in the V IX IndexThe V IX index is constructed so that it represents the implied volatility of a synthetic at-the-money option contract on the S&P100index that has a maturity of one month.It is constructed from eight S&P100index puts and calls and takes into account the American features of the option contracts,discrete cash dividends and microstructure frictions such as bid-ask spreads (see Whaley(2000)for further details).6Figure1plots the V IX index from January1986to December2000.The mean level of the daily V IX series is20.5%,and its standard deviation is7.85%.Because the V IX index is highly serially correlated with afirst-order autocorrelation of 0.94,we measure daily innovations in aggregate volatility by using daily changes in V IX, which we denote as∆V IX.Dailyfirst differences in V IX have an effective mean of zero(less than0.0001),a standard deviation of2.65%,and also have negligible serial correlation(the first-order autocorrelation of∆V IX is-0.0001).As part of our robustness checks in Section 2.3,we also measure innovations in V IX by specifying a stationary time-series model for the conditional mean of V IX andfind our results to be similar to using simplefirst differences. While∆V IX seems an ideal proxy for innovations in volatility risk because the V IX index is representative of traded option securities whose prices directly reflect volatility risk,there are two main caveats with using V IX to represent observable market volatility.Thefirst concern is that the V IX index is the implied volatility from the Black-Scholes 5In previous versions of this paper,we also considered sample volatility,following Schwert and Stambaugh (1987);a range-based estimate,following Alizadeh,Brandt and Diebold(2002);and a high-frequency estima-tor of volatility from Andersen,Bollerslev and Diebold(2003).Using these measures to proxy for innovations in aggregate volatility produces little spread in cross-sectional average returns.These tables are available upon request.6On September22,2003,the CBOE implemented a new formula and methodology to construct its volatility index.The new index is based on the S&P500(rather than the S&P100)and takes into account a broader range of strike prices rather than using only at-the-money option contracts.The CBOE now uses V IX to refer to this new index.We use the old index(denoted by the ticker V XO).We do not use the new index because it has been constructed by back-filling only to1990,whereas the V XO is available in real-time from1986.The CBOE continues to make both volatility indices available.The correlation between the new and the old CBOE volatility series is98%from1990-2000,but the series that we use has a slightly broader range.(1973)model,and we know that the Black-Scholes model is an approximation.If the true stochastic environment is characterized by stochastic volatility and jumps,∆V IX will reflect total quadratic variation in both diffusion and jump components(see,for example,Pan(2002)). Although Bates(2000)argues that implied volatilities computed taking into account jump risk are very close to original Black-Scholes implied volatilities,jump risk may be priced differ-ently from volatility risk.Our analysis does not separate jump risk from diffusion risk,so our aggregate volatility risk may include jump risk components.A more serious reservation about the V IX index is that V IX combines both stochastic volatility and the stochastic volatility risk premium.Only if the risk premium is zero or constant would∆V IX be a pure proxy for the innovation in aggregate volatility.Decomposing∆V IX into the true innovation in volatility and the volatility risk premium can only be done by writing down a formal model.The form of the risk premium depends on the parameterization of the price of volatility risk,the number of factors and the evolution of those factors.Each different model specification implies a different risk premium.For example,many stochastic volatility option pricing models assume that the volatility risk premium can be parameterized as a linear function of volatility(see,for example,Chernov and Ghysels(2000),Benzoni(2002),and Jones(2003)).This may or may not be a good approximation to the true price of risk.Rather than imposing a structural form,we use an unadulterated∆V IX series.An advantage of this approach is that our analysis is simple to replicate.The Pre-Formation RegressionOur goal is to test if stocks with different sensitivities to aggregate volatility innovations(prox-ied by∆V IX)have different average returns.To measure the sensitivity to aggregate volatility innovations,we reduce the number of factors in the full specification in equation(1)to two,the market factor and∆V IX.A two-factor pricing kernel with the market return and stochastic volatility as factors is also the standard set-up commonly assumed by many stochastic option pricing studies(see,for example,Heston,1993).Hence,the empirical model that we examine is:r i t =β0+βiMKT·MKT t+βi∆V IX·∆V IX t+εit,(3)where MKT is the market excess return,∆V IX is the instrument we use for innovations inthe aggregate volatility factor,andβiMKT andβi∆V IXare loadings on market risk and aggregatevolatility risk,respectively.Previous empirical studies suggest that there are other cross-sectional factors that have ex-planatory power for the cross-section of returns,such as the size and value factors of the Fama and French(1993)three-factor model(hereafter FF-3).We do not directly model these effectsin equation(3),because controlling for other factors in constructing portfolios based on equa-tion(3)may add a lot of noise.Although we keep the number of regressors in our pre-formation portfolio regressions to a minimum,we are careful to ensure that we control for the FF-3factors and other cross-sectional factors in assessing how volatility risk is priced using post-formation regression tests.We construct a set of assets that are sufficiently disperse in exposure to aggregate volatility innovations by sortingfirms on∆V IX loadings over the past month using the regression(3) with daily data.We run the regression for all stocks on AMEX,NASDAQ and the NYSE,with more than17daily observations.In a setting where coefficients potentially vary over time,a 1-month window with daily data is a natural compromise between estimating coefficients with a reasonable degree of precision and pinning down conditional coefficients in an environment with time-varying factor loadings.P´a stor and Stambaugh(2003),among others,also use daily data with a1-month window in similar settings.At the end of each month,we sort stocks into quintiles,based on the value of the realizedβ∆V IX coefficients over the past month.Firms in quintile1have the lowest coefficients,whilefirms in quintile5have the highestβ∆V IX loadings. Within each quintile portfolio,we value-weight the stocks.We link the returns across time to form one series of post-ranking returns for each quintile portfolio.Table1reports various summary statistics for quintile portfolios sorted by pastβ∆V IX over the previous month using equation(3).Thefirst two columns report the mean and standard deviation of monthly total,not excess,simple returns.In thefirst column under the heading ‘Factor Loadings,’we report the pre-formationβ∆V IX coefficients,which are computed at the beginning of each month for each portfolio and are value-weighted.The column reports the time-series average of the pre-formationβ∆V IX loadings across the whole sample.By con-struction,since the portfolios are formed by ranking on pastβ∆V IX,the pre-formationβ∆V IX loadings monotonically increase from-2.09for portfolio1to2.18for portfolio5.The columns labelled‘CAPM Alpha’and‘FF-3Alpha’report the time-series alphas of these portfolios relative to the CAPM and to the FF-3model,respectfully.Consistent with the negative price of systematic volatility risk found by the option pricing studies,we see lower average raw returns,CAPM alphas,and FF-3alphas with higher past loadings ofβ∆V IX.All the differences between quintile portfolios5and1are significant at the1%level,and a joint test for the alphas equal to zero rejects at the5%level for both the CAPM and the FF-3model.In particular,the5-1spread in average returns between the quintile portfolios with the highest and lowestβ∆V IX coefficients is-1.04%per month.Controlling for the MKT factor exacerbates the5-1spread to-1.15%per month,while controlling for the FF-3model decreases the5-1 spread to-0.83%per month.Requirements for a Factor Risk ExplanationWhile the differences in average returns and alphas corresponding to differentβ∆V IX loadings are very impressive,we cannot yet claim that these differences are due to systematic volatility risk.We will examine the premium for aggregate volatility within the framework of an uncon-ditional factor model.There are two requirements that must hold in order to make a case for a factor risk-based explanation.First,a factor model implies that there should be contemporane-ous patterns between factor loadings and average returns.For example,in a standard CAPM, stocks that covary strongly with the market factor should,on average,earn high returns over the same period.To test a factor model,Black,Jensen and Scholes(1972),Fama and French(1992 and1993),Jagannathan and Wang(1996),and P´a stor and Stambaugh(2003),among others,all form portfolios using various pre-formation criteria,but examine post-ranking factor loadings that are computed over the full sample period.While theβ∆V IX loadings show very strong patterns of future returns,they represent past covariation with innovations in market volatility. We must show that the portfolios in Table1also exhibit high loadings with volatility risk over the same period used to compute the alphas.To construct our portfolios,we took∆V IX to proxy for the innovation in aggregate volatil-ity at a daily frequency.However,at the standard monthly frequency,which is the frequency of the ex-post returns for the alphas reported in Table1,using the change in V IX is a poor approximation for innovations in aggregate volatility.This is because at lower frequencies,the effect of the conditional mean of V IX plays an important role in determining the unanticipated change in V IX.In contrast,the high persistence of the V IX series at a daily frequency means that thefirst difference of V IX is a suitable proxy for the innovation in aggregate volatility. Hence,we should not measure ex-post exposure to aggregate volatility risk by looking at how the portfolios in Table1correlate ex-post with monthly changes in V IX.To measure ex-post exposure to aggregate volatility risk at a monthly frequency,we follow Breeden,Gibbons and Litzenberger(1989)and construct an ex-post factor that mimics aggre-gate volatility risk.We term this mimicking factor F V IX.We construct the tracking portfolio so that it is the portfolio of asset returns maximally correlated with realized innovations in volatility using a set of basis assets.This allows us to examine the contemporaneous relation-ship between factor loadings and average returns.The major advantage of using F V IX to measure aggregate volatility risk is that we can construct a good approximation for innovations in market volatility at any frequency.In particular,the factor mimicking aggregate volatility innovations allows us to proxy aggregate volatility risk at the monthly frequency by simply cumulating daily returns over the month on the underlying base assets used to construct the mimicking factor.This is a much simpler method for measuring aggregate volatility innova-。
韩国大学考试题目及答案
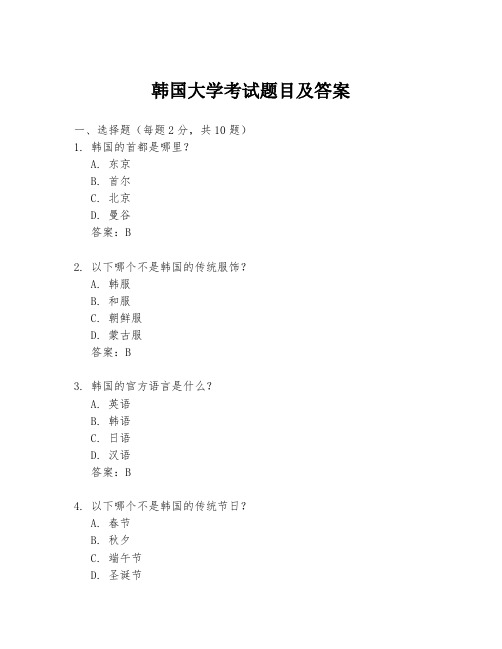
韩国大学考试题目及答案一、选择题(每题2分,共10题)1. 韩国的首都是哪里?A. 东京B. 首尔C. 北京D. 曼谷答案:B2. 以下哪个不是韩国的传统服饰?A. 韩服B. 和服C. 朝鲜服D. 蒙古服答案:B3. 韩国的官方语言是什么?A. 英语B. 韩语C. 日语D. 汉语答案:B4. 以下哪个不是韩国的传统节日?A. 春节B. 秋夕C. 端午节D. 圣诞节答案:D5. 韩国货币的单位是什么?A. 韩元B. 日元C. 美元D. 欧元答案:A6. 韩国最大的岛屿是什么?A. 济州岛B. 台湾岛C. 海南岛D. 本州岛答案:A7. 以下哪个是韩国的著名大学?A. 首尔大学B. 东京大学C. 北京大学D. 牛津大学答案:A8. 韩国的国花是什么?A. 玫瑰B. 木槿花C. 樱花D. 牡丹答案:B9. 以下哪个不是韩国的流行文化元素?A. K-POPB. K-DramaC. K-FoodD. K-Sport答案:D10. 韩国的人口大约有多少?A. 5000万B. 1亿C. 3000万D. 7000万答案:A二、填空题(每题2分,共5题)11. 韩国位于朝鲜半岛的________部分。
答案:南部12. 韩国的三大移动通信公司是SK Telecom、KT和________。
答案:LG Uplus13. 韩国的国宝级文化遗产之一是________。
答案:昌德宫14. 韩国的代表性传统乐器是________。
答案:伽倻琴15. 韩国的著名传统美食之一是________。
答案:泡菜三、简答题(每题10分,共2题)16. 简述韩国的教育体系。
答案:韩国的教育体系分为基础教育和高等教育两个阶段。
基础教育包括六年制的小学和三年制的初中。
高等教育则包括三年制的高中或职业学校以及大学本科教育。
韩国的教育体系强调学术成就和考试,其中大学入学考试(大学修学能力考试)是学生教育生涯中非常重要的一部分。
17. 描述韩国的经济发展模式。
Generalized WDVV equations for B_r and C_r pure N=2 Super-Yang-Mills theory

a r X i v :h e p -t h /0102190v 1 27 F eb 2001Generalized WDVV equations for B r and C r pure N=2Super-Yang-Mills theoryL.K.Hoevenaars,R.MartiniAbstractA proof that the prepotential for pure N=2Super-Yang-Mills theory associated with Lie algebrasB r andC r satisfies the generalized WDVV (Witten-Dijkgraaf-Verlinde-Verlinde)system was given by Marshakov,Mironov and Morozov.Among other things,they use an associative algebra of holomorphic diffter Ito and Yang used a different approach to try to accomplish the same result,but they encountered objects of which it is unclear whether they form structure constants of an associative algebra.We show by explicit calculation that these objects are none other than the structure constants of the algebra of holomorphic differentials.1IntroductionIn 1994,Seiberg and Witten [1]solved the low energy behaviour of pure N=2Super-Yang-Mills theory by giving the solution of the prepotential F .The essential ingredients in their construction are a family of Riemann surfaces Σ,a meromorphic differential λSW on it and the definition of the prepotential in terms of period integrals of λSWa i =A iλSW ∂F∂a i ∂a j ∂a k .Moreover,it was shown that the full prepotential for simple Lie algebras of type A,B,C,D [8]andtype E [9]and F [10]satisfies this generalized WDVV system 1.The approach used by Ito and Yang in [9]differs from the other two,due to the type of associative algebra that is being used:they use the Landau-Ginzburg chiral ring while the others use an algebra of holomorphic differentials.For the A,D,E cases this difference in approach is negligible since the two different types of algebras are isomorphic.For the Lie algebras of B,C type this is not the case and this leads to some problems.The present article deals with these problems and shows that the proper algebra to use is the onesuggested in[8].A survey of these matters,as well as the results of the present paper can be found in the internal publication[11].This paper is outlined as follows:in thefirst section we will review Ito and Yang’s method for the A,D,E Lie algebras.In the second section their approach to B,C Lie algebras is discussed. Finally in section three we show that Ito and Yang’s construction naturally leads to the algebra of holomorphic differentials used in[8].2A review of the simply laced caseIn this section,we will describe the proof in[9]that the prepotential of4-dimensional pure N=2 SYM theory with Lie algebra of simply laced(ADE)type satisfies the generalized WDVV system. The Seiberg-Witten data[1],[12],[13]consists of:•a family of Riemann surfacesΣof genus g given byz+µz(2.2)and has the property that∂λSW∂a i is symmetric.This implies that F j can be thought of as agradient,which leads to the followingDefinition1The prepotential is a function F(a1,...,a r)such thatF j=∂FDefinition2Let f:C r→C,then the generalized WDVV system[4],[5]for f isf i K−1f j=f j K−1f i∀i,j∈{1,...,r}(2.5) where the f i are matrices with entries∂3f(a1,...,a r)(f i)jk=The rest of the proof deals with a discussion of the conditions1-3.It is well-known[14]that the right hand side of(2.1)equals the Landau-Ginzburg superpotential associated with the cor-∂W responding Lie ing this connection,we can define the primaryfieldsφi(u):=−∂x (2.10)Instead of using the u i as coordinates on the part of the moduli space we’re interested in,we want to use the a i .For the chiral ring this implies that in the new coordinates(−∂W∂a j)=∂u x∂a jC z xy (u )∂a k∂a k )mod(∂W∂x)(2.11)which again is an associative algebra,but with different structure constants C k ij (a )=C k ij(u ).This is the algebra we will use in the rest of the proof.For the relation(2.7)weturn to another aspect of Landau-Ginzburg theory:the Picard-Fuchs equations (see e.g [15]and references therein).These form a coupled set of first order partial differential equations which express how the integrals of holomorphic differentials over homology cycles of a Riemann surface in a family depend on the moduli.Definition 6Flat coordinates of the Landau-Ginzburg theory are a set of coordinates {t i }on mod-uli space such that∂2W∂x(2.12)where Q ij is given byφi (t )φj (t )=C kij (t )φk (t )+Q ij∂W∂t iΓ∂λsw∂t kΓ∂λsw∂a iΓ∂λsw∂a lΓ∂λsw∂t r(2.15)Taking Γ=B k we getF ijk =C lij (a )K kl(2.16)which is the intended relation (2.7).The only thing that is left to do,is to prove that K kl =∂a mIn conclusion,the most important ingredients in the proof are the chiral ring and the Picard-Fuchs equations.In the following sections we will show that in the case of B r ,C r Lie algebras,the Picard-Fuchs equations can still play an important role,but the chiral ring should be replaced by the algebra of holomorphic differentials considered by the authors of [8].These algebras are isomorphic to the chiral rings in the ADE cases,but not for Lie algebras B r ,C r .3Ito&Yang’s approach to B r and C rIn this section,we discuss the attempt made in[9]to generalizethe contentsof the previoussection to the Lie algebras B r,C r.We will discuss only B r since the situation for C r is completely analogous.The Riemann surfaces are given byz+µx(3.1)where W BC is the Landau-Ginzburg superpotential associated with the theory of type BC.From the superpotential we again construct the chiral ring inflat coordinates whereφi(t):=−∂W BC∂x (3.2)However,the fact that the right-hand side of(3.1)does not equal the superpotential is reflected by the Picard-Fuchs equations,which no longer relate the third order derivatives of F with the structure constants C k ij(a).Instead,they readF ijk=˜C l ij(a)K kl(3.3) where K kl=∂a m2r−1˜C knl(t).(3.4)The D l ij are defined byQ ij=xD l ijφl(3.5)and we switched from˜C k ij(a)to˜C k ij(t)in order to compare these with the structure constants C k ij(t). At this point,it is unknown2whether the˜C k ij(t)(and therefore the˜C k ij(a))are structure constants of an associative algebra.This issue will be resolved in the next section.4The identification of the structure constantsThe method of proof that is being used in[8]for the B r,C r case also involves an associative algebra. However,theirs is an algebra of holomorphic differentials which is isomorphic toφi(t)φj(t)=γk ij(t)φk(t)mod(x∂W BC2Except for rank3and4,for which explicit calculations of˜C kij(t)were made in[9]we will rewrite it in such a way that it becomes of the formφi(t)φj(t)=rk=1 C k ij(t)φk(t)+P ij[x∂x W BC−W BC](4.3)As afirst step,we use(3.4):φiφj= Ci·−→φ+D i·−→φx∂x W BC j= C i−D i·r n=12nt n2r−1 C n·−→φ+D i·−→φx∂x W BCj(4.4)The notation −→φstands for the vector with componentsφk and we used a matrix notation for thestructure constants.The proof becomes somewhat technical,so let usfirst give a general outline of it.The strategy will be to get rid of the second term of(4.4)by cancelling it with part of the third term,since we want an algebra in which thefirst term gives the structure constants.For this cancelling we’ll use equation(3.4)in combination with the following relation which expresses the fact that W BC is a graded functionx ∂W BC∂t n=2rW BC(4.5)Cancelling is possible at the expense of introducing yet another term which then has to be canceled etcetera.This recursive process does come to an end however,and by performing it we automatically calculate modulo x∂x W BC−W BC instead of x∂x W BC.We rewrite(4.4)by splitting up the third term and rewriting one part of it using(4.5):D i·−→φx∂x W BC j= −12r−1 D i·−→φx∂x W BC j= −D i2r−1·−→φx∂x W BC j(4.6) Now we use(4.2)to work out the productφkφn and the result is:φiφj= C i·−→φ−D i2r−1·r n=12nt n D n·−→φx∂x W BC j +2rD i2r−1·rn=12nt n −D n·r m=12mt m2r−1[x∂x W BC−W BC]j(4.8)Note that by cancelling the one term,we automatically calculate modulo x∂x W BC −W BC .The expression between brackets in the first line seems to spoil our achievement but it doesn’t:until now we rewrote−D i ·r n =12nt n 2r −1C m ·−→φ+D n ·−→φx∂x W BCj(4.10)This is a recursive process.If it stops at some point,then we get a multiplication structureφi φj =r k =1C k ij φk +P ij (x∂x W BC −W BC )(4.11)for some polynomial P ij and the theorem is proven.To see that the process indeed stops,we referto the lemma below.xby φk ,we have shown that D i is nilpotent sinceit is strictly upper triangular.Sincedeg (φk )=2r −2k(4.13)we find that indeed for j ≥k the degree of φk is bigger than the degree ofQ ij5Conclusions and outlookIn this letter we have shown that the unknown quantities ˜C k ijof[9]are none other than the structure constants of the algebra of holomorphic differentials introduced in [8].Therefore this is the algebra that should be used,and not the Landau-Ginzburg chiral ring.However,the connection with Landau-Ginzburg can still be very useful since the Picard-Fuchs equations may serve as an alternative to the residue formulas considered in [8].References[1]N.Seiberg and E.Witten,Nucl.Phys.B426,19(1994),hep-th/9407087.[2]E.Witten,Two-dimensional gravity and intersection theory on moduli space,in Surveysin differential geometry(Cambridge,MA,1990),pp.243–310,Lehigh Univ.,Bethlehem,PA, 1991.[3]R.Dijkgraaf,H.Verlinde,and E.Verlinde,Nucl.Phys.B352,59(1991).[4]G.Bonelli and M.Matone,Phys.Rev.Lett.77,4712(1996),hep-th/9605090.[5]A.Marshakov,A.Mironov,and A.Morozov,Phys.Lett.B389,43(1996),hep-th/9607109.[6]R.Martini and P.K.H.Gragert,J.Nonlinear Math.Phys.6,1(1999).[7]A.P.Veselov,Phys.Lett.A261,297(1999),hep-th/9902142.[8]A.Marshakov,A.Mironov,and A.Morozov,Int.J.Mod.Phys.A15,1157(2000),hep-th/9701123.[9]K.Ito and S.-K.Yang,Phys.Lett.B433,56(1998),hep-th/9803126.[10]L.K.Hoevenaars,P.H.M.Kersten,and R.Martini,(2000),hep-th/0012133.[11]L.K.Hoevenaars and R.Martini,(2000),int.publ.1529,www.math.utwente.nl/publications.[12]A.Gorsky,I.Krichever,A.Marshakov,A.Mironov,and A.Morozov,Phys.Lett.B355,466(1995),hep-th/9505035.[13]E.Martinec and N.Warner,Nucl.Phys.B459,97(1996),hep-th/9509161.[14]A.Klemm,W.Lerche,S.Yankielowicz,and S.Theisen,Phys.Lett.B344,169(1995),hep-th/9411048.[15]W.Lerche,D.J.Smit,and N.P.Warner,Nucl.Phys.B372,87(1992),hep-th/9108013.[16]K.Ito and S.-K.Yang,Phys.Lett.B415,45(1997),hep-th/9708017.。
(完整版)研究生英语综合教程-下课后习题答案
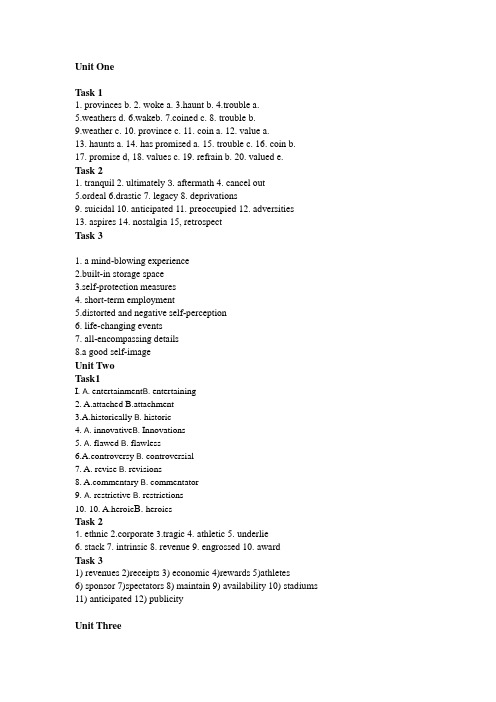
Task 11. provinces b.2. woke a.3.haunt b.4.trouble a.5.weathers d.6.wakeb.7.coined c.8. trouble b.9.weather c. 10. province c. 11. coin a. 12. value a.13. haunts a. 14. has promised a. 15. trouble c. 16. coin b. 17. promise d, 18. values c. 19. refrain b. 20. valued e.Task 21. tranquil2. ultimately3. aftermath4. cancel out5.ordeal6.drastic7. legacy8. deprivations9. suicidal 10. anticipated 11. preoccupied 12. adversities 13. aspires 14. nostalgia 15, retrospectTask 31. a mind-blowing experience2.built-in storage space3.self-protection measures4. short-term employment5.distorted and negative self-perception6. life-changing events7. all-encompassing details8.a good self-imageUnit TwoTask1I. A. entertainment B. entertaining2. A.attached B.attachment3.A.historically B. historic4. A. innovative B. Innovations5. A. flawed B. flawless6.A.controversy B. controversial7. A. revise B. revisions8. mentary B. commentator9.A. restrictive B. restrictions10.10. A.heroic B. heroicsTask 21. ethnic2.corporate3.tragic4. athletic5. underlie6. stack7. intrinsic8. revenue9. engrossed 10. awardTask 31) revenues 2)receipts 3) economic 4)rewards 5)athletes6) sponsor 7)spectators 8) maintain 9) availability 10) stadiums 11) anticipated 12) publicityUnit Three1.B 2, D 1 A 4, C 5, A 6.B 7,C 8. A 9.B 10. CTask2LA. discrete B. discreet C. discretion2.A. auditors B. auditorium C. audit D. auditory E. audited1 A. conception B.contrivance C. contrive D. conceive4.A. giggling B. gasped C. gargling D. gossip5.A. affectionate B. passion C. affection D. passionate6.A.reluctant B. relentless C. relevant7.A. reverence B. reverent C. revere8.A. peeping/peep B.peered C. perceive D.poringTask31) gain 2) similarities 3) diverse 4)enrich 5) perspective6)discover 7)challenging 8) specific 9)adventure 10)enlightens11) opportunities 12) memories 13) joyful 14) outweighs 15) span)Unit FourTask 11) uncomfortable 2)reading 3)immerse 4)deep 5) access 6)concentration 7)stopped 8)altered 9)change 10) different 11)decoders12) disengaged 13) variations 14) words 15) tighterTask 21.D2.A3. B4.B5.D6. A7. C8. CTask 1Step 1l)i 2)f 3)a 4)b 5)h 6)j 7)c 8)e 9)d 10)gStep 21)fidgety2)crushing3)pithy4) foraging5) definitive ,6)propelled7) applauded8) ubiquity9) duly10) curtailTask 21. above2.on3. to4.on5.on/about6. to 7 .with 8. at 9. on/about10. in Task 31. may have a subtle effect on2.provide free access toe-books3. isinthe midst ofa sea change4. has been onthe faculty ofHarvard University5.a voracious book reader6. you'll stay focused onit7. the conduit for information8.your check came asanabsolute godsend9. lost the thread ofthe story10. stroll through elegant proseTask 11.A2.C3.D4.B5.C6.B7.C8.D9.A10.C11.B12D.13.D14.A15.BTask21.sheer2.slip3desert4. revenge5.sheered6. level7.deserted8.skirted9.protested10. duplicates11. level12. revenge13.skirt14. protests15. slip16.duplicate Unit SixTask 1I.C 2.A 3.C 4.A 5.D 6.C 7.B 8.D 9.A 10.C lI.B 12.ATask21. Water isnot an effective shield2.engulfed inflames3.the rights ofsovereign nations4. outpaced its rivals inthe market5. There's no need tobelabor the point6. She invoked several eminent scholars7. from two embattled villages8. According tothe witness's testimony9. Inspite ofour best endeavors10. After many trials and tribulationsTask21) remain2) childish3)reaffirm4)precious5)equal6)measure7)greatness8) journey9)leisure10) fame11) obscure12) prosperityUnit SevenTask1I.C 2.B 3.B 4.D 5.B 6.C 7.C 8.A 9.B 10.BTask21. patrons b.2.designated b.3. reference d.4. inclination c5. host d.6. diffusing b.7. host c8.inclination a.9. references c.10. patrons a.11. reference a.12. host a.13. diffuses a..14. designate a.15. designate c.Task31) alive2)awakened3) trip4)stone5)remains6)beyond7)records8)social 9)across10) surrounding11) mental12) miracle13) having14) failure15) participateUnit EightTask 11.B2.D3. A4.B5.A6. D7. D8.A9. A 10. CTask21. A. outburst B.bursting C. outbreak2.A. adverse B.adversity C. advised3.A. distinguishes B.distinct C. distinguished4.A. sight/vision B. view C. outlook D. visions5. A. implicit B.implicit/implied C. underlying6.A.washed B. awash C. washing7.A. jumped/sprang B. springs C.leap D.jumped8. A. trail B. trail/track C. traceD. trackE.trace9.A. sensed B.sensible C. senseD. sensitiveE.sensational10.A. prosperous B.prosperity C. prospects D. prophecyTask31)echoes2) pays heed to3)hidden4) objectively5) decipher6)presence7)conviction 8)shot9)however10) slaughter11) bare12) trim13) are connected to14) strive15) yield Unit NineTask 11.A2.B3.D4.A5.B6.B7.C8.A9.C 10.DTask2I. explain, plain, complained, plain2. tolerate, tolerant, tolerance3. consequence,sequence,consequent4. commerce, commercial, commercial, commercialism, commercially5. arouse, arising, arise, arousal6. irritant, irritation, irritable, irritate7. democratic, dynamic, automated, dramatic8. dominate, dominant, predominant, predominate9. celebrate, celebrity, celebrated, celebration10. temporal, contemporary, temporaryTask3I) encompassing2)standard3)constraints4)presented5)resolution6) constitute7) entertainment8) interchangeably9) distinction10) fuzzy11) technically12) devoted to13) ranging14) competing15) biasesUnit TenTask 11) beware of2)unpalatable3)delineate4) Ingrained5) amplify6) supplanted7) pin down8)discretionary9) stranded10)swept throughTask21. that happy-to-be-alive attitude2.anl-told-you-so air3. the-end-justifies-the-means philosophy4.Aheart-in-the-mouth moment5.a now-or-never chance6. a touch-and-go situation7.a wait-and-see attitude8.too-eager-not-to-lose9.a cards-on-the-table approach10. anine-to-five lifestyle11.a look-who's-talking tone12. around-the-clock service13. a carrot-and-stick approach14. a rags-to-riches man15. a rain-or-shine picnicTask3I) exquisite2)soothe3)equivalent4)literally5)effective6)havoc7)posted8)notify9) clumsy10) autonomously。
On the effectiveness of address-space randomization
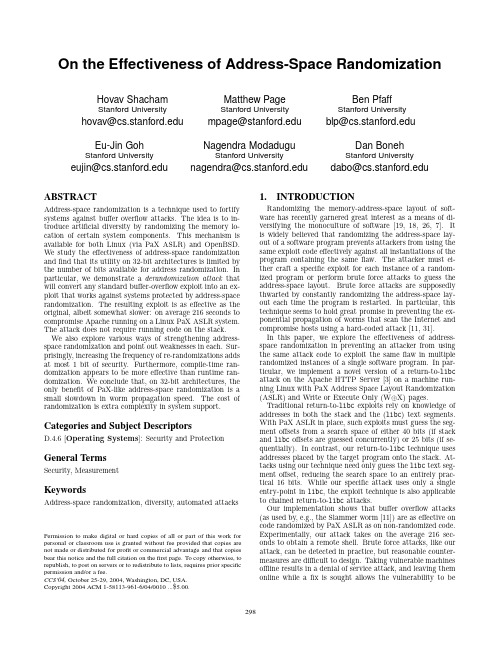
On the Effectiveness of Address-Space RandomizationHovav ShachamStanford University hovav@Matthew PageStanford Universitympage@Ben PfaffStanford Universityblp@Eu-Jin GohStanford University eujin@Nagendra ModaduguStanford Universitynagendra@Dan BonehStanford Universitydabo@ABSTRACTAddress-space randomization is a technique used to fortify systems against buffer overflow attacks.The idea is to in-troduce artificial diversity by randomizing the memory lo-cation of certain system components.This mechanism is available for both Linux(via PaX ASLR)and OpenBSD. We study the effectiveness of address-space randomization andfind that its utility on32-bit architectures is limited by the number of bits available for address randomization.In particular,we demonstrate a derandomization attack that will convert any standard buffer-overflow exploit into an ex-ploit that works against systems protected by address-space randomization.The resulting exploit is as effective as the original,albeit somewhat slower:on average216seconds to compromise Apache running on a Linux PaX ASLR system. The attack does not require running code on the stack.We also explore various ways of strengthening address-space randomization and point out weaknesses in each.Sur-prisingly,increasing the frequency of re-randomizations adds at most1bit of security.Furthermore,compile-time ran-domization appears to be more effective than runtime ran-domization.We conclude that,on32-bit architectures,the only benefit of PaX-like address-space randomization is a small slowdown in worm propagation speed.The cost of randomization is extra complexity in system support.Categories and Subject DescriptorsD.4.6[Operating Systems]:Security and ProtectionGeneral TermsSecurity,MeasurementKeywordsAddress-space randomization,diversity,automated attacks Permission to make digital or hard copies of all or part of this work for personal or classroom use is granted without fee provided that copies are not made or distributed for profit or commercial advantage and that copies bear this notice and the full citation on thefirst page.To copy otherwise,to republish,to post on servers or to redistribute to lists,requires prior specific permission and/or a fee.CCS’04,October25-29,2004,Washington,DC,USA.Copyright2004ACM1-58113-961-6/04/0010...$5.00.1.INTRODUCTIONRandomizing the memory-address-space layout of soft-ware has recently garnered great interest as a means of di-versifying the monoculture of software[19,18,26,7].It is widely believed that randomizing the address-space lay-out of a software program prevents attackers from using the same exploit code effectively against all instantiations of the program containing the sameflaw.The attacker must ei-ther craft a specific exploit for each instance of a random-ized program or perform brute force attacks to guess the address-space layout.Brute force attacks are supposedly thwarted by constantly randomizing the address-space lay-out each time the program is restarted.In particular,this technique seems to hold great promise in preventing the ex-ponential propagation of worms that scan the Internet and compromise hosts using a hard-coded attack[11,31].In this paper,we explore the effectiveness of address-space randomization in preventing an attacker from using the same attack code to exploit the sameflaw in multiple randomized instances of a single software program.In par-ticular,we implement a novel version of a return-to-libc attack on the Apache HTTP Server[3]on a machine run-ning Linux with PaX Address Space Layout Randomization (ASLR)and Write or Execute Only(W⊕X)pages. Traditional return-to-libc exploits rely on knowledge of addresses in both the stack and the(libc)text segments. With PaX ASLR in place,such exploits must guess the seg-ment offsets from a search space of either40bits(if stack and libc offsets are guessed concurrently)or25bits(if se-quentially).In contrast,our return-to-libc technique uses addresses placed by the target program onto the stack.At-tacks using our technique need only guess the libc text seg-ment offset,reducing the search space to an entirely prac-tical16bits.While our specific attack uses only a single entry-point in libc,the exploit technique is also applicable to chained return-to-libc attacks.Our implementation shows that buffer overflow attacks (as used by,e.g.,the Slammer worm[11])are as effective on code randomized by PaX ASLR as on non-randomized code. Experimentally,our attack takes on the average216sec-onds to obtain a remote shell.Brute force attacks,like our attack,can be detected in practice,but reasonable counter-measures are difficult to design.Taking vulnerable machines offline results in a denial of service attack,and leaving them online while afix is sought allows the vulnerability to beexploited.The problem of detecting and managing a brute force attack is especially exacerbated by the speed of our attack.While PaX ASLR appears to provide a slowdown in attack propagation,work done by Staniford et al.[31]sug-gests that this slowdown may be inadequate for inhibiting worm propagation.Although our discussion is specific to PaX ASLR,the attack is generic and applies to other address-space ran-domization systems such as that in OpenBSD.The attack also applies to any software program accessible locally or through a network connection.Our attack demonstrates what we call a derandomization attack;derandomization converts any standard buffer-overflow exploit into an ex-ploit that works against systems protected by address-space randomization.The resulting exploit is as effective as the original,but slower.On the other hand,the slowdown is not sufficient to prevent its being used in worms or in a targeted attack.In the second part of the paper,we explore and analyze the effectiveness of more powerful randomization techniques such as increasing the frequency of re-randomization and alsofiner grained randomizations.We show that subse-quent re-randomizations(regardless of frequency)after the initial address-space randomization improve security against a brute force attack by at most a factor of2.This result suggests that it would be far more beneficial to focus on increasing the entropy in the address-space layout.Further-more,this result shows that our brute force attacks are still feasible against network servers that are restarted with dif-ferent randomization upon crashing(unlike Apache).We also analyze the effectiveness of crash detectors in mitigat-ing such attacks.Our analysis suggests that runtime address-space random-ization is far less effective on32-bit architectures than com-monly pile-time address-space randomization can be more effective than runtime randomization because the address space can be randomized at a muchfiner gran-ularity at compile-time than runtime(e.g.,by reordering functions within libraries).We note that buffer overflow mitigation techniques can prevent some attacks,including the one we present in this paper.However,overflow mitiga-tion by itself without any address-space randomization also defeats many of these attacks.Thus,the security provided by overflow mitigation is largely orthogonal to address-space randomization.We speculate that the most promising solution appears to be upgrading to a64-bit architecture.Randomization comes at a cost:in both32and64bit architectures,randomized executables are more difficult to debug and support.1.1Related WorkExploits.Buffer overflow exploits started with simple stack smashing techniques where the return address of the current stack frame is overwritten to point to injected code[1].After the easy stack smashing vulnerabilities were discovered and exploited,aflurry of new attacks emerged that exploited overflows in the heap[20],format string errors[28],integer overflows[35],and double-free()errors[2]. Countermeasures.Several techniques were developed to counter stack smashing—StackGuard by Cowan et al.[14] detects stack smashing attacks by placing canary values next to the return address.StackShield by Vendicator[32]makes a second copy of the return address to check against before using it.These techniques are effective for reducing the number of exploitable buffer overflows but does not com-pletely remove the threat.For example,Bulba and Kil3r[8] show how to bypass these buffer overflow defenses. ProPolice by Etoh[16]extends the ideas behind Stack-Guard by reordering local variables and function arguments, and placing canaries in the stack.ProPolice also copies function pointers to an area preceding local variable buffers. ProPolice is packaged with the latest versions of OpenBSD. PointGuard by Cowan et al.[13]prevents pointer corruption by encrypting them while in memory and only decrypting values before dereferencing.W⊕X Pages and Return-to-libc.The techniques described so far aim to stop attackers from seizing control of program execution.A orthogonal technique called W⊕X nullifies at-tacks that inject and execute code in a process’s address space.W⊕X is based on the observation that most of the exploits so far inject malicious code into a process’s address space and then circumvent program control to execute the injected code.Under W⊕X,pages in the heap,stack,and other memory segments are marked either writable(W)or executable(X),but not both.StackPatch by Solar De-signer[29]is a Linux kernel patch that makes the stack non-executable.The latest versions of Linux(through the PaX project[26])and of OpenBSD contain implementations of W⊕X.Our sample attack works on a system running PaX with W⊕X.With W⊕X memory pages,attackers cannot inject and execute code of their own choosing.Instead,they must use existing executable code—either the program’s own code or code in libraries loaded by the program.For example,an attacker can overwrite the stack above the return address of the current frame and then change the return address to point to a function he wishes to call.When the function in the current frame returns,program controlflow is redi-rected to the attacker’s chosen function and the overwritten portions of the stack are treated as arguments. Traditionally,attackers have chosen to call functions in the standard C-language library,libc,which is an attrac-tive target because it is loaded into every Unix program and encapsulates the system-call API by which programs access such kernel services as forking child processes and commu-nicating over network sockets.This class of attacks,orig-inally suggested by Solar Designer[30],is therefore known as“return-to-libc.”Implementations of W⊕X on CPUs whose memory-man-agement units lack a per-page execute bit—for example, current x86chips—incur a significant performance penalty. Another defense against malicious code injection is ran-domized instruction sets[6,21].On the other hand,ran-domized instruction sets are ineffective against return-to-libc attacks for the same reasons as those given above for W⊕X pages.Address-Space Randomization.Observe that a“return-to-libc”attack needs to know the virtual addresses of the libc functions to be written into a function pointer or return address.If the base address of the memory segment con-taining libc is randomized,then the success rate of such an attack significantly decreases.This idea is implemented inPaX as ASLR[27].PaX ASLR randomizes the base address of the stack,heap,code,and mmap()ed segments of ELF ex-ecutables and dynamic libraries at load and link time.We implemented our attack against a PaX hardened system and will give a more detailed description of PaX in Sect.2.1. Previous projects have employed address randomization as a security mechanism.Yarvin et al.[34]develop a low-overhead RPC mechanism by placing buffers and executable-but-unreadable stubs at random locations in the address space,treating the addresses of these buffers and stubs as ca-pabilities.Their analysis shows that a32-bit address space is insufficient to keep processes from guessing such capabil-ity addresses,but that a64-bit address space is,assuming a time penalty is assessed on bad guesses.Bhatkar et al.[7]define and discuss address obfuscation. Their implementation randomizes the base address of the stack,heap,and code segments and adds random padding to stack frame and malloc()function calls.They imple-mented a binary tool that rewrites executables and object files to randomize addresses.Randomizing addresses at link and compilation timefixes the randomizations when the sys-tem is built.This approach has the shortcoming of giv-ing an attacker afixed address-space layout that she can probe repeatedly to garner information.Their solution to this problem is periodically to“re-obfuscate”executables and libraries—that is,periodically relink and recompile ex-ecutables and libraries.As pointed out in their paper,this solution interferes with host based intrusion detection sys-tems based onfiles’integrity checksums.Our brute force attack works just as well on the published version of this system because their published implementation only ran-domizes the base address of libraries`a la PaX.Xu et al.[33]designed a runtime randomization system that does not require kernel changes,but is otherwise sim-ilar to PaX.The primary difference between their system and PaX is that their system randomizes the location of the Global Offset Table(GOT)and patches the Procedu-ral Linkage Table(PLT)accordingly.Our attack also works against their system because:(1)their system uses13bits of randomness(3bits less than PaX),and(2)our attack does not need to determine the location of the GOT.2.BREAKING PAX ASLRWe briefly review the design of PaX and Apache before describing our attack and experimental results.2.1PaX ASLR DesignPaX applies ASLR to ELF binaries and dynamic libraries. For the purposes of ASLR,a process’s user address space consists of three areas,called the executable,mapped,and stack areas.The executable area contains the program’s executable code,initialized data,and uninitialized data;the mapped area contains the heap,dynamic libraries,thread stacks,and shared memory;and the stack area is the main user stack.ASLR randomizes these three areas separately,adding to the base address of each one an offset variable randomly chosen when the process is created.For the Intel x86ar-chitecture,PaX ASLR provides16,16,and24bits of ran-domness,respectively,in these memory areas.In particu-lar,the mapped data offset,called delta mmap,is limited to 16bits of randomness because(1)altering bits28through 31would limit the mmap()system call’s ability to handle large memory mappings,and(2)altering bits0through11 would cause memory mapped pages not to be aligned on page boundaries.Our attack takes advantage of two characteristics of the PaX ASLR system.First,because PaX ASLR randomizes only the base addresses of the three memory areas,once any of the three delta variables is leaked,an attacker can fix the addresses of any memory location within the area controlled by the variable.In particular,we are interested in the delta mmap variable that determines the randomized offset of segments allocated by mmap().As noted above, delta mmap only contains16bits of randomness.Because our return-to-libc technique does not need to guess any stack addresses(unlike traditional return-to-libc attacks), our attack only needs to brute force the small amount of entropy in delta mmap.Our attack only requires a linear search of the randomized address space.That is,our exploit requires216=65,536probes at worst and32,768probes on the average,which is a relatively small number.Second,in PaX each offset variable isfixed throughout a process’s lifetime,including any processes that fork()from a parent process.Many network daemons,specifically the Apache web server,fork child processes to handle incoming connections,so that determining the layout of any one of these related processes reveals that layout for all of them. Although this behavior on fork()is not a prerequisite for our attack,we show in Sect.3.2that it halves the expected time to success.2.2Return-to-libc AttackWe give a high level overview of the attack before describ-ing its implementation in greater detail and giving experi-mental data.We emphasize that although our discussion is specific to PaX ASLR,the attack applies to other address-space randomization systems such as that in OpenBSD. 2.2.1OverviewWe implemented our attack on the Apache web server running on Linux with PaX ASLR and W⊕X pages.The current version of the Apache server(1.3.29)has no known overflows,so we replicated a buffer overflow similar to one discovered in the Oracle9PL/SQL Apache module[10,22]. This Oracle hole can be exploited using a classic buffer over-flow attack—an attacker injects her own code by supply-ing an arbitrarily long request to the web server that over-flows an internal buffer.Nevertheless,this attack fails in an Apache server protected by PaX W⊕X.Instead,we ex-ploit this hole using the return-to-libc technique discussed in Sect.1.1.Our return-to-libc technique is non-standard.Chained return-to-libc attacks generally rely on prior knowledge of stack addresses.PaX randomizes24bits of stack base ad-dresses(on x86),making these attacks infeasible.However, PaX does not randomize the stack layout,which allows us to locate a pointer to attacker supplied data on the stack. Moreover,a randomized layout would provide no protection against access to data in the top stack frame,and little pro-tection against access to data in adjacent frames.Our attack against Apache occurs in two steps.Wefirst determine the value of delta mmap using a brute force at-tack that pinpoints an address in libc.Once the delta mmap value is obtained,we mount a return-to-libc attack to ob-tain a shell.ap getline()argumentssaved EIPsaved EBP64byte buffer...bottom of stack(lower addresses)Figure1:Apache child process stack before probeFirst,the attack repeatedly overflows the stack buffer ex-posed by the Oracle hole with guesses for the address of the libc function usleep()in an attempt to return into the usleep()function.An unsuccessful guess causes the Apache child process to crash,and the parent process to fork a new child in its place,with the same randomization deltas.A successful exploit causes the connection to hang for16seconds and gives enough information for us to de-duce the value of delta mmap.Upon obtaining delta mmap, we now know the location of all functions in libc,including the system()function.1With this information,we can now mount a return-to-libc attack on the same buffer exposed by the Oracle hole to invoke the system()function.Our attack searches for usleep()first only for conve-nience;it could instead search directly for system()and check periodically whether it has obtained a shell.Our at-tack can therefore be mounted even if libc entry points are independently randomized,a possibility we consider in Sect.3.3.2.2.2.2ImplementationWefirst describe the memory hole in the Oracle9PL/SQL Apache module.Oracle Buffer Overflow.We create a buffer overflow in Apache similar to one found in Oracle9[10,22].Specifically, we add the following lines to the function ap getline()in http protocol.c:char buf[64];...strcpy(buf,s);/*Overflow buffer*/ Although the buffer overflow in the Oracle exploit is1000 bytes long,we use a shorter buffer for the sake of brevity. In fact,a longer buffer works to the attacker’s advantage because it gives more room to supply shell commands. Precomputing libc Addresses.In order to build the ex-ploit,we mustfirst determine the offsets of the functions system(),usleep(),and a ret instruction in the libc li-brary.The offsets are easily obtained using the system objdump tool.With these offsets,once the exploit deter-mines the address of usleep(),we can deduce the value of delta mmap followed by the correct virtual addresses of system()and ret,with the simple sumaddress=0x40000000+offset+delta mmap.1The system()function executes user-supplied commands via the standard shell(usually/bin/sh).0x010101010xDEADBEEFguessed address of usleep()0xDEADBEEF64byte buffer,nowfilled with A’s...bottom of stack(lower addresses)Figure2:Stack after one probe(Here0x40000000is the standard base address for memory obtained with mmap()under Linux.)Exploit Step1.As mentioned in the overview,thefirst step is to determine the value of delta mmap.We do this by re-peatedly overflowing the stack buffer exposed by the Oracle hole with guesses for usleep()’s address in an attempt to return into the usleep()function in libc.More specifically, the brute force attack works as follows:1.Iterate over all possible values for delta mmap startingfrom0and ending at65535.2.For each value of delta mmap,compute the guess forthe randomized virtual address of usleep()from its offset.3.Create the attack buffer(described later)and send itto the Apache web server.4.If the connection closes immediately,continue with thenext value of delta mmap.If the connection hangs for 16seconds,then the current guess for delta mmap is correct.The contents of the attack buffer sent to Apache are best described by illustrations of the Apache child process’s stack before and after overflowing the buffer with the current guess for usleep()’s address.Figure1shows the Apache child process’s stack before the attack is mounted and Fig-ure2shows the same stack after one guess for the address of usleep().The saved return address of ap getline()(saved EIP) is overwritten with the guessed address of the usleep() function in the libc library,the saved EBP pointer is over-written with usleep()’s return address0xDEADBEEF,and 0x01010101(decimal16,843,009)is the argument passed to usleep()(the sleep time in microseconds).Any shorter time interval results in null bytes being included in the attack buffer.2Note that the method for placing null bytes onto the stack by Nergal[24]is infeasible because stack addresses are strongly randomized.Finally,when ap getline()returns, control passes to the guessed address of usleep().If the value of delta mmap(and hence the address of usleep()) is guessed correctly,Apache will hang for approximately 16seconds and then terminate the connection.If the ad-dress of usleep()is guessed incorrectly,the connection ter-2Null bytes act as C string terminators,causing strcpy() (our attack vector)to terminate before overflowing the entire buffer.ap getline()argumentssaved EIPsaved EBP64byte buffer...bottom of stack(lower addresses)Figure3:Apache child process stack before overflowminates immediately.This difference in behavior tells us when we have guessed the correct value of delta mmap. Exploit Step2.Once delta mmap has been determined,we can compute the addresses of all other functions in libc with certainty.The second step of the attack uses the same Ora-cle buffer overflow hole to conduct a return-to-libc attack. The composition of the attack buffer sent to the Apache web server is the critical component of step2.Again,the con-tents of the attack buffer are best described by illustrations of the Apache child process’s stack before and after the step 2attack.Figure3shows the Apache child process’s stack before the attack and Figure4shows the stack immediately after the strcpy()call in ap getline()(the attack buffer has already been injected).Thefirst64bytes of the attack buffer isfilled with the shell command that we want system()to execute on a suc-cessful exploit.The shell command is followed by a series of pointers to ret instructions that serves as a“stack pop”sequence.Recall that the ret instruction pops4bytes from the stack into the EIP register,and program execution con-tinues from the address now in EIP.Thus,the effect of this sequence of ret s is to pop a desired number of32-bit words offthe stack.Just above the pointers to ret instructions,the attack buffer contains the address of system().The stack pop sequence“eats up”the stack until it reaches a pointer pointing into the original64byte buffer,which serves as the argument to the system()function.Wefind such a pointer in the stack frame of ap getline()’s calling function. After executing strcpy()on the exploited buffer,Apache returns into the sequence of ret instructions until it reaches system().Apache then executes the system()function with the supplied commands.In our attack,the shell command is“wget /dropshell;chmod+x dropshell;./dropshell;”where dropshell is a pro-gram that listens on a specified port and provides a remote shell with the user id of the Apache process.Note that any shell command can be executed.2.2.3ExperimentsThe brute force exploit was executed on a2.4GHz Pen-tium4machine against a PaX ASLR(for Linux kernel ver-sion2.6.1)protected Apache server(version1.3.29)running on a Athlon1.8GHz machine.The two machines were con-nected over a100Mbps network.Each probe sent by our exploit program results in a to-tal of approximately200bytes of network traffic,including Ethernet,IP,and TCP headers.Therefore,our brute force attack only sends a total of12.8MB of network data at worst,and6.4MB of network data on expectation.pointer into64byte buffer0xDEADBEEFaddress of system()address of ret instruction...address of ret instruction0xDEADBEEF64byte buffer(contains shell commands)...bottom of stack(lower addresses)Figure4:Stack after buffer overflowAfter running10trials,we obtained the following timing measurements(in seconds)for our attack against the PaX ASLR protected Apache server:Average Max Min21681029The speed of our attack is limited by the number of child processes Apache allows to run concurrently.We used the default setting of150in our experiment.2.3Information Leakage AttacksIn the presence of information leakage,attacks can be crafted that require fewer probes and are therefore more ef-fective than our brute force attack in defeating randomized layouts.For instance,Durden[15]shows how to obtain the delta_mmap variable from the stack by retrieving the return address of the main()function using a format string vulner-ability.Durden also shows how to convert a special class of buffer overflow vulnerabilities into a format string vulnera-bility.Not all overflows,however,can be exploited to create a format string bug.Furthermore,for a remote exploit,the leaked information has to be conveyed back to the attacker over the network,which may be difficult when attacking a network daemon.Note that the brute force attack de-scribed in the previous section works against any buffer over-flows and does not make any assumptions about the network server.3.IMPROVEMENTS TO ADDRESS-SPACERANDOMIZATION ARCHITECTURE Our attack on address-space randomization relied on sev-eral characteristics of the implementation of PaX ASLR.In particular,our attack exploited the low entropy(16bits)of PaX ASLR on32-bit x86processors,and the feature that address-space layouts are randomized only at program load-ing and do not change during the process lifetime.This sec-tion explores the consequences of changing either of these assumptions by moving to a64-bit architecture or making the randomization more frequent or morefine-grained. 3.164-Bit ArchitecturesIn case of Linux on32-bit x86machines,16of the32ad-dress bits are available for randomization.As our resultsshow,16bits of address randomization can be defeated by a brute force attack in a matter of minutes.Any64-bit machine,on the other hand,is unlikely to have fewer than 40address bits available for randomization given that mem-ory pages are usually between4kB and4MB in size.On-line brute force attacks that need to guess at least40bits of randomness can be ruled out as a threat,since an attack of this magnitude is unlikely to go unnoticed.Although64-bit machines are now beginning to be more widely deployed,32-bit machines are likely to remain the most widely deployed machines in the short and medium term.Furthermore,ap-plications that run in32-bit compatibility mode on a64-bit machine are no less vulnerable than when running on a32-bit machine.Some proposed64-bit systems implement a global virtual address space,that is,all applications share a single64-bit address space[12].Analyzing the effectiveness of ad-dress randomization in these operating systems is beyond the scope of this paper.3.2Randomization FrequencyPaX ASLR randomizes a process’s memory segments only at process creation.If we randomize the address space lay-out of a process more frequently,we might naively expect a significant increase in security.However,we will demon-strate that after the initial address space randomization, periodic re-randomizing adds no more than1bit of secu-rity against brute force attacks regardless of the frequency, providing little extra security.This also shows that brute force attacks are feasible even against non-forking network daemons that crash on every probe.On the other hand,fre-quent re-randomizations can mitigate the damage when the layout of afixed randomized address space is leaked through other channels.We analyze the security implications of increasing the fre-quency of address-space randomization by considering two brute force attack scenarios:1.The address-space randomization isfixed during theduration of an attack.For example,this scenario ap-plies to our brute force attack against the current im-plementation of PaX ASLR or in any situation where the randomized address space isfixed at compile-time.2.The address-space randomization changes with eachprobe.It is pointless to re-randomize the address space more than once between any two probes.Therefore, this scenario represents the best re-randomization fre-quency for a ASLR program.This scenario applies,for example,to brute force attacks attacks against non-forking servers protected by PaX ASLR that crash on every probe;these servers are restarted each time witha different randomized address-space layout.The brute force attacks in the two scenarios are different. In scenario1,a brute force attack can linear search the ad-dress space through its probes before launching the exploit (exactly our attack in Sect.2).In scenario2,a brute force attack guesses the layout of the address space randomly, tailors the exploit to the guessed layout,and launches the exploit.We now analyze the expected number of probe attempts for a brute force attack to succeed against a network server in both scenarios.In each case,let n be the number of bits of randomness that must be guessed to successfully mount the attack,implying that there are2n possibilities.Fur-thermore,only1out of these2n possibilities is correct.The brute force attack succeeds once it has determined the cor-rect state.Scenario1.In this scenario,the server has afixed address-space randomization throughout the attack.Since the ran-domization isfixed,we can compute the expected number of probes required by a brute force attack by viewing the problem as a standard sampling without replacement prob-lem.The probability that the brute force attack succeeds only after taking exactly t probes is2n−12n·2n−22n−1...2n−t−12n−t|{z}Pr[first t−1probes fail]·12n−t−1=12n,where n is the number of bits of randomness in the address space.Therefore,the expected number of probes required for scenario1is2nXt=1t·12n=12n·2nXt=1t=(2n+1)/2≈2n−1.Scenario2.In this scenario,the server’s address space is re-randomized with every probe.Therefore,the expected number of probes required by a brute force attack can be computed by viewing the problem as a sampling with re-placement problem.The probability that the brute force attack succeeds only after taking exactly t probes is given by the geometric random variable with p=1/2n.The ex-pected number of probes required is1/p=2n. Conclusions.We can easily see that a brute force attack in scenario2requires approximately2n/2n−1=2times as many probes compared to scenario1.Since scenario2repre-sents the best possible frequency that an ASLR program can do,we conclude that increasing the frequency of address-space re-randomization is at best equivalent to increasing the entropy of the address space by only1bit.The difference between a forking server and a non-forking server for the purposes of our brute force attack is that for the forking server the address-space randomization is the same for all the probes,whereas the non-forking server crashes and has a different address-space randomization on every probe.This difference is exactly that between scenar-ios1and2.Therefore,the brute force attack is also feasible against non-forking servers if the address-space entropy is low.For example,in the case of Apache protected by PaX ASLR,we expect to perform215=32,768probes beforefix-ing the value of delta mmap,whereas if Apache were a single-process event-driven server that crashes on each probe,the expected number of probes required would double to a mere 216=65,536.3.3Randomization GranularityPaX ASLR only randomizes the offset location of an en-tire shared library.Below,we discuss the feasibility of ran-domizing addresses at an evenfiner granularity.For ex-ample,in addition to randomizing segment base addresses, we could also randomize function and variable addresses。
26个英文字母拼音书写笔顺标准及练习-耐磨一号打印版.docx
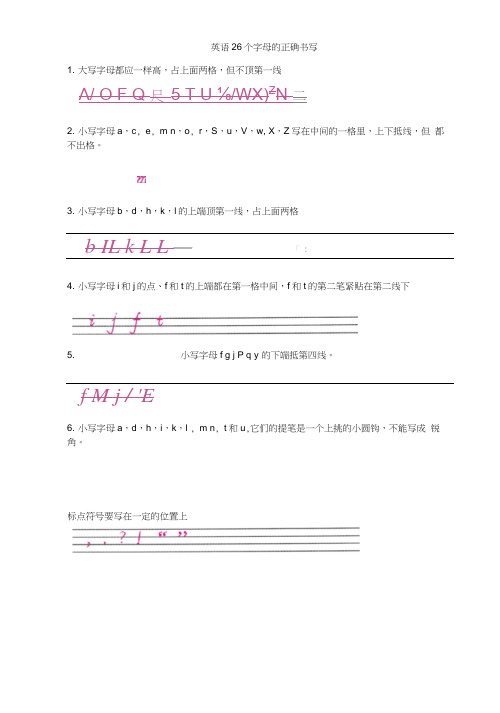
英语26个字母的正确书写
1. 大写字母都应一样高,占上面两格,但不顶第一线
Λ/ O F Q 尺5 T U ⅛/WX)Z N 二
2. 小写字母a,c, e, m n,o, r,S,u,V,w, X,Z写在中间的一格里,上下抵线,但都不出格。
3. 小写字母b,d,h,k,l的上端顶第一线,占上面两格
b IL k L L —「:
4. 小写字母i和j的点、f和t的上端都在第一格中间,f和t的第二笔紧贴在第二线下
5. 小写字母f g j P q y 的下端抵第四线。
f M j / 'E
6. 小写字母a,d,h,i,k,l , m n, t和u,它们的提笔是一个上挑的小圆钩,不能写成锐角。
标点符号要写在一定的位置上
字母的笔顺字母要按一定的笔划顺序书写,其书写笔顺如下。
(请注意书写的格式和大小写的区别。
)
汉语拼音字母表
23个声”
b Pmfd t n~~1
g k h JqX
Zh Ch Sh r Z C Sy 应二、6个草豪母表
CloeiU 口二
土母:9牛Ju⅛母.5个IuH⅛母二4个后鼻韵母
αi ei LJi αo OLl in ie ije er α∩en in UnOn αng eng ing Ong
四、16个整体认读音节
Zhi Chi Shi ri Zi Ci Si
yι WU y u ye yue.y ua n
BEl %
S ≡E S ⅞⅛.。
《Meet-Ms.Liu》Me-and-My-Class-【品质课件】
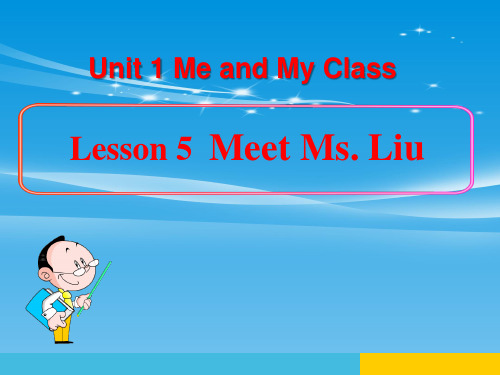
speak loudly in class.
4. ---Let’s _d_i_s_c__u_s__s_ (讨论) the problem together!
---That’s a good idea.
9. Ms. Liu has many interests. (同义句)
Ms. Liu i_s__ i_n_t_e__r_e_s__t_e_d___ _i_n__ many things.
10. She started teaching English seven years ago. 同义句)
Sheb_e__c_a__m__ean Englisht_e_a__c_h__e_rseven years ago.
8. be patient with
对___…__…__有___耐__心______
9. give sb.enough time to do sth给某__人___足__够__时___间__做__某__事
10. with a smile on one’s face 面___带__微__笑___________
5. We had a d__is__c_u__s_s__io__n_(discuss) about how to stay
healthy in class yesterday.
6. Jenny is a good girl. She is always ready _t_o__h__e__lp__
What a good , kind teacher she is !
长风破浪会有时,直挂云帆济沧海。努力,终会有所收获,功夫不负有心人。以铜为镜,可以正衣冠;以古为镜,可以知兴替;以人为镜,可以明得失。前进的路上 照自己的不足,学习更多东西,更进一步。穷则独善其身,达则兼济天下。现代社会,有很多人,钻进钱眼,不惜违法乱纪;做人,穷,也要穷的有骨气!古之立大 之才,亦必有坚忍不拔之志。想干成大事,除了勤于修炼才华和能力,更重要的是要能坚持下来。士不可以不弘毅,任重而道远。仁以为己任,不亦重乎?死而后已, 理想,脚下的路再远,也不会迷失方向。太上有立德,其次有立功,其次有立言,虽久不废,此谓不朽。任何事业,学业的基础,都要以自身品德的修炼为根基。饭 而枕之,乐亦在其中矣。不义而富且贵,于我如浮云。财富如浮云,生不带来,死不带去,真正留下的,是我们对这个世界的贡献。英雄者,胸怀大志,腹有良策, 吞吐天地之志者也英雄气概,威压八万里,体恤弱小,善德加身。老当益壮,宁移白首之心;穷且益坚,不坠青云之志老去的只是身体,心灵可以永远保持丰盛。乐 其乐;忧民之忧者,民亦忧其忧。做领导,要能体恤下属,一味打压,尽失民心。勿以恶小而为之,勿以善小而不为。越是微小的事情,越见品质。学而不知道,与 行,与不知同。知行合一,方可成就事业。以家为家,以乡为乡,以国为国,以天下为天下。若是天下人都能互相体谅,纷扰世事可以停歇。志不强者智不达,言不 越高,所需要的能力越强,相应的,逼迫自己所学的,也就越多。臣心一片磁针石,不指南方不肯休。忠心,也是很多现代人缺乏的精神。吾日三省乎吾身。为人谋 交而不信乎?传不习乎?若人人皆每日反省自身,世间又会多出多少君子。人人好公,则天下太平;人人营私,则天下大乱。给世界和身边人,多一点宽容,多一份担 为生民立命,为往圣继绝学,为万世开太平。立千古大志,乃是圣人也。丹青不知老将至,贫贱于我如浮云。淡看世间事,心情如浮云天行健,君子以自强不息。地 载物。君子,生在世间,当靠自己拼搏奋斗。博学之,审问之,慎思之,明辨之,笃行之。进学之道,一步步逼近真相,逼近更高。百学须先立志。天下大事,不立 川,有容乃大;壁立千仞,无欲则刚做人,心胸要宽广。其身正,不令而行;其身不正,虽令不从。身心端正,方可知行合一。子曰:“知者不惑,仁者不忧,勇者不惧 者,不会把时间耗费在负性情绪上。好学近乎知,力行近乎仁,知耻近乎勇。力行善事,有羞耻之心,方可成君子。操千曲尔后晓声,观千剑尔后识器做学问和学技 的练习。第一个青春是上帝给的;第二个的青春是靠自己努力当眼泪流尽的时候,留下的应该是坚强。人总是珍惜未得到的,而遗忘了所拥有的。谁伤害过你,谁击 重要的是谁让你重现笑容。幸运并非没有恐惧和烦恼;厄运并非没有安慰与希望。你不要一直不满人家,你应该一直检讨自己才对。不满人家,是苦了你自己。最深 一个人,而是心里没有了任何期望。要铭记在心;每一天都是一年中最完美的日子。只因幸福只是一个过往,沉溺在幸福中的人;一直不知道幸福却很短暂。一个人 贡献什么,而不应当看他取得什么。做个明媚的女子。不倾国,不倾城,只倾其所有过的生活。生活就是生下来,活下去。人生最美的是过程,最难的是相知,最苦 的是真爱,最后悔的是错过。两个人在一起能过就好好过!不能过就麻利点分开。当一个人真正觉悟的一刻,他放下追寻外在世界的财富,而开始追寻他内心世界的 弱就是自己最大的敌人。日出东海落西山,愁也一天,喜也一天。遇事不转牛角尖,人也舒坦,心也舒坦。乌云总会被驱散的,即使它笼罩了整个地球。心态便是黑 可以照亮整个世界。生活不是单行线,一条路走不通,你可以转弯。给我一场车祸。要么失忆。要么死。有些人说:我爱你、又不是说我只爱你一个。生命太过短暂 不一定能得到。删掉了关于你的一切,唯独删不掉关于你的回忆。任何事都是有可能的。所以别放弃,相信自己,你可以做到的。、相信自己,坚信自己的目标,去 的磨难与挫折,不断去努力、去奋斗,成功最终就会是你的!既然爱,为什么不说出口,有些东西失去了,就在也回不来了!对于人来说,问心无愧是最舒服的枕头 他人的成功,被人嫉妒,表明自己成功。在人之上,要把人当人;在人之下,要把自己当人。人不怕卑微,就怕失去希望,期待明天,期待阳光,人就会从卑微中站 想去拥抱蓝天。成功需要成本,时间也是一种成本,对时间的珍惜就是对成本的节约。人只要不失去方向,就不会失去自己。过去的习惯,决定今天的你,所以,过 今天的一败涂地。让我记起容易,但让我忘记我怕我是做不到。不要跟一个人和他议论同一个圈子里的人,不管你认为他有多可靠。想象困难做出的反应,不是逃避 面对它们,同它们打交道,以一种进取的和明智的方式同它们奋斗。他不爱你,你为他挡一百颗子弹也没用。坐在电脑前,不知道做什么,却又不想关掉它。做不了 间帮你决定。如果还是无法决定,做了再说。宁愿犯错,不留遗憾。发现者,尤其是一个初出茅庐的年轻发现者,需要勇气才能无视他人的冷漠和怀疑,才能坚持自 把研究继续下去。我的本质不是我的意志的结果,相反,我的意志是我的本质的结果,因为我先有存在,后有意志,存在可以没有意志,但是没有存在就没有意志。 的福利,可以使可憎的工作变为可贵,只有开明人士才能知道克服困难所需要的热忱。立志用功如种树然,方其根芽,犹未有干;及其有干,尚未有枝;枝而后叶, 出现不是对愿望的否定,而是把愿望合并和提升到一个更高的意识无论是美女的歌声,还是鬓狗的狂吠,无论是鳄鱼的眼泪,还是恶狼的嚎叫,都不会使我动摇。即 难,已经开始了的事情决不放弃。最可怕的敌人,就是没有坚强的信念。既然我已经踏上这条道路,那么,任何东西都不应妨碍我沿着这条路走下去。意志若是屈从 它都帮助了暴力。有了坚定的意志,就等于给双脚添了一对翅膀。意志坚强,只有刚强的人,才有神圣的意志,凡是战斗的人,才能取得胜利。卓越的人的一大优点 的遭遇里百折不挠。疼痛的强度,同自然赋于人类的意志和刚度成正比。能够岿然不动,坚持正见,度过难关的人是不多的。钢是在烈火和急剧冷却里锻炼出来的, 么也不怕。我们的一代也是这样的在斗争中和可怕的考验中锻炼出来的,学习了不在生活面前屈服。只要持续地努力,不懈地奋斗,就没有征服不了的东西。
海信电视TC2169-TF2106CH-TC2111CH-TF2111DG-TF2108D-TC2108D-TC2119CH-TF21R68-TF2166H-TF2168H-TF21S69说

12
G
10 11
14
+<')65
= >8H
?Q S Q SC< ,
15 16 17
8H C< 8H , 8H D
7=>+,SDE =>C ^GS X
M O ,-VN(
W#12 Y"
^Y@ <12 J,21385( $%6 0% G3G4 5Z*
>?1 2$678(
YZ [\ ]R4^-
YG B_ `>: Z [
A KL M !"#$% &NNJOPQYR STUV W%&XYN&#'OZ([\]^_`><="#Wa ()
."
>
JKL "#32 &X56 _ =
= G XUV >456 & . &CN"# MN& $ N "&' X () !" # $ & CN % 2 >Y &&' (5 *+&)+*(+
) a BCDE@A@ABCDE-'0 78DE@A( 3 9:;<K= =KG <.'( a>?CBC;@DAB@ DE ;F- ! "
CD( a : E-`F BCHF" H( a : E BCD AGH IJ*G KL ( BCHMN_O R-( : *BIB ,PQ R S3[T K UQ9( V G:- K3W PBI^ a@ BCDE;F-! "
绝热规定30-46-0000-01-001Rev01
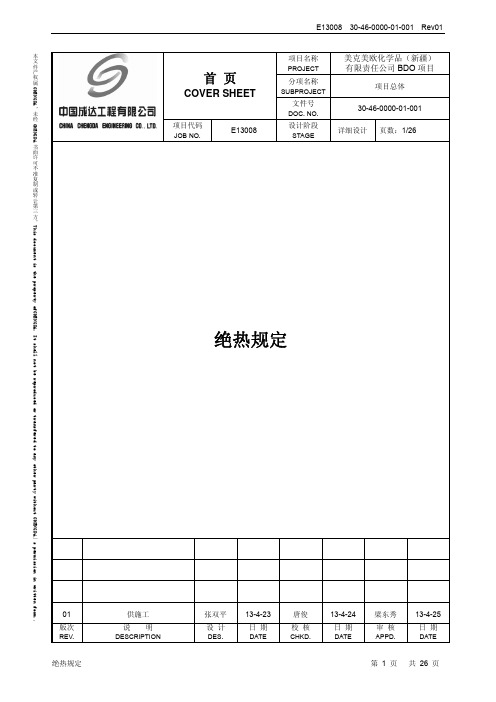
绝热规定
第 6 页
共 26 页
E13008
30-46-0000-01-001 Rev01
5) 在输送过程中,由于热损失可能析出结晶的管道; 6) 输送介质由于热损失粘度增高,系统阻力增加,输送量下降,达不到工艺最小允许量 的管道; 7) 输送介质的凝固点等于或高于环境温度的管道; 8) 输送含水酸性介质的露点等于或高于环境温度的管道。 5. 5.1 5.1.1 绝热结构 保温结构 保温结构一般由保温层和保护层组成。埋地管道和设备或敷设在地沟内的管道和设 备,保温层外表面应设置防潮层,防潮层材料可采用聚乙烯薄膜。保温结构设计应 符合保温效果好,施工方便,防火,耐久和美观等要求。 5.1.2 保温层厚度按 10mm 为单位进行分挡。除浇注型和填充型绝热材料,在无其它说明 的情况下,当采用一种绝热制品,保温层厚度大于 80mm 时,应分为两层或多层逐 层施工,各层厚度宜相近,缝隙应互相错开。 5.1.3 高低温度交替的设备和管道的保温层,其保温材料应在高温区及低温区均能安全使 用。 5.1.4 保温结构应具有一定的机械强度,不因受自重或偶然外力的作用而破坏。对于振动 的设备与管道的保温结构,应进行加固。 5.1.5 保温结构一般不考虑可拆卸性,但需经常维修的部位如法兰,阀门和人孔宜采用可 拆卸的保温结构。 5.1.6 外表面有加热盘管的容器设备,应在加热盘管和绝热材料之间设置一层与保护层材 料相同的铝合金薄板。 5.2 5.2.1 保冷结构 保冷结构一般由保冷层、防潮层和保护层组成。保冷结构设计应符合保冷效果好, 施工方便,防火,耐久和美观等要求。 5.2.2 保冷层厚度按 10mm 为单位进行分档。 硬质泡沫塑料保冷材料的最小厚度为 30mm。 除浇注型和填充型绝热材料,在无其它说明的情况下,当采用一种绝热制品,保冷 层厚度大于 80mm 时,应分为两层或多层逐层施工,各层厚度宜相近,缝隙应互
- 1、下载文档前请自行甄别文档内容的完整性,平台不提供额外的编辑、内容补充、找答案等附加服务。
- 2、"仅部分预览"的文档,不可在线预览部分如存在完整性等问题,可反馈申请退款(可完整预览的文档不适用该条件!)。
- 3、如文档侵犯您的权益,请联系客服反馈,我们会尽快为您处理(人工客服工作时间:9:00-18:30)。
Complete Classes of Strategiesfor theClassical Iterated Prisoner’s DilemmaBruno B EAUFILS,Jean-Paul D ELAHAYE,and Philippe M ATHIEULaboratoire d’Informatique Fondamentale de LilleU.R.A.369C.N.R.S.–Universit´e des Sciences et Technologies de LilleU.F.R.d’I.E.E.A.Bˆa t.M359655Villeneuve d’Ascq Cedex–FRANCEbeaufils,delahaye,mathieu@lifl.frAbstract.The Classical Iterated Prisoner’s Dilemma(CIPD)is used to studythe evolution of cooperation.We show,with a genetic approach,how basic ideascould be used in order to generate automatically a great numbers of strategies.Then we show some results of ecological evolution on those strategies,with thedescription of the experimentations we have made.Our main purpose is tofind anobjective method to evaluate strategies for the CIPD.Finally we use the formerresults to add a new argument confirming that there is,in order to be good,aninfinite gradient in the level of complexity in structure of strategies.1The Classical Iterated Prisoner’s DilemmaIntroduced by Merill M.F LOOD and Melvin D RESHER in the RAND Corporation in 1952,see[3],who tried to introduce some irrationality in the game theory of John VON N EUMANN and Oskar M ORGENSTERN[8],the Classical Iterated Prisoner’s Dilemma (CIPD)is based on this simple story quoted by Albert T UCKER for instance in[5,pages 117–118]:Two men,charged with a joint violation of law,are held separately by the police.Each is told that(1)if one confesses and the other does not,the former will be given a re-ward...and the latter will befined(2)if both confess,each will befined...At the same time,each has a good reason to believe that(3)if neither confesses,both will go clear.It seems clearly obvious that the most reasonable choice is to betray its partner.More formally the CIPD is represented,using game theory,as a two-person non-zero-sum non-cooperative and simultaneous game where each player has to choose between two moves:–COOPERATE,let us write C,and let us say to be nice–DEFECT,let us write D,and let us say to be naughtyTable1.CIPD payoff matrix.Row player score are givenfirst.CooperateCooperate,Sucker’s payoffTemptation to defect ,Temptation to defectSucker’s payoffc corresponds to the C strategy of the one shot game applied without modifica-tions in the CIPD:it always plays Callforcd plays periodically C then D,let us note(CD)softfor2Round Robin Tournament and Ecological EvolutionNow the main problem is to evaluate strategies for the CIPD,in order to compare them.Two kinds of experimentation could be used for this purpose.The basic one,is to make a pairwise round-robin tournament between some different strategies.The payoff to each one would be the total sum of each iterated game.A ranking could then be computed according to the score of each strategy.The higher a strategy is ranked,the better it is.Good strategies in round-robin tournament are well adapted to their environments,but often are not very robust to environment modifications.The second kind of experimentation is a kind of imitation of the natural selection pro-cess,and is closely related to population dynamics.Let us consider a population of players,each one adopting a particular strategy.At the beginning we consider that each strategy is equally represented in the population.Then a tournament is made,and good strategies are favoured,whereas bad ones are disadvantaged,by a proportional popula-tion redistribution.This redistribution process,also called a generation,is repeated until an eventual population stabilisation,i.e.no changes between two generations.A good strategy is then a strategy which stays alive in the population for the longest possible time,and in the biggest possible proportion.An example of evolution between all strategies described in the previous section is shown in Figure 1.The -axis represents the generation number,whereas the -axis represents the size of the population for each strategy.For simplicity we make our computation with a fixed global population size.5010015020025030035040005101520253035N u m b e r o f i n d i v i d u a l sGenerationstit_for_tat soft_majo spiteful all_c prober per_cd all_dFig.1.Example of ecological evolution.Classical results in this field,which were presented by A XELROD in [1],show that to be good a strategy must:–be nice,i.e.not be the first to defect–be reactive–forgive–not be too clever,i.e.to be simple in order to be understood by its opponentThe well-known tit tat strategy,which satisfies all those criteria,has,since[1], been considered by a lot of people using the dilemma-but not by game theorist-to be one of the best strategies not only for cooperation but also for evolution of cooperation. We think that the simplicity criterium is not so good,and have thus introduced a strategy called gradual which illustrates our point of view.Gradual cooperates on thefirst move,then after thefirst opponent’s defection defects one time,and cooperates two times,after the second opponent’s defection defects two times and cooperates two times,...,after the opponent’s defection defects times, let us call it the punishment period,and cooperates two times,let us name it the lull time.Gradual had better results than tit tat in almost all our experiments, see[2].It is easy to imagine strategies derived from gradual,for instance in modifying the function of punishment,which is the identity in the gradual case(defections punishment of length).Our main research direction to establish our ideas about a strategy’s complexity is to –try a lot of different strategies,in an automatic and objective manner–have a general and,as far as possible,objective method to evaluate strategies,for instance to compare them.3Complete Classes of StrategiesTo make our research process easier,we have tofind a descriptive method to define strategies,which is less risky than an exhaustive method,which are never objective,nor complete.One of the ways we have chosen is to use a genetic approach.We describe a structure(a genotype),which can be decoded in a particular behaviour(a phenotype). Then a way to have a strategy,is simply tofill this structure.A manner to have a lot of strategies is to consider all the ways offilling this genotype,i.e.to consider all possible individuals based on a given genotype.Let us call the set of all strategies described by a particular genotype,the complete class of strategies issued by this genotype.We have described three genotypes based on the same simple idea,in order to remain objective.This idea is to consider the observable length of the game’s history.Such idea of strategies has already been studied in[4,7].Those three genotypes are: memory:Each strategy can only see moves of its past,and moves of its opponent’s past.The strategy is then started by moves predefinedin the genotype.All other moves are coded in the genotype,according to the visible past configuration.The genotype length is then. binarymemoryforD DThis strategy is one way of coding spiteful.4Some ExperimentsWe have conducted some experiments using those complete classes.The main purpose was to evaluate other strategies in big ecological evolution,but also to try tofind some new good strategies.In all our experiments we have computed one ecological evolution between all strategies of a class,and then another with gradual added to the popula-tion.Thus we have been able to partially confirm our ideas on the strength of this strat-egy,as shown in Table2.In all the results of Table2,gradual is better evaluated than tit tat.We,however,do not show results of the evaluation of tit tat here since it is included in some of the classes explored,thus its evaluation is only partial.4.1Some Memory and BinaryTable 2.Some results of the evaluation of gradual in complete classes.Class size is the number of described strategies,whereas evaluation is the rank of the strategy at the end of an evolution of the complete class.class size tittatmemory11106421211102413binary3221113automata113210020030040050060070080005101520253035N u m b e r o f i n d i v i d u a l sGenerationsstr01_c_cd str01_d_cd str01_c_dd str01_d_dd str01_d_dc str01_c_dc str01_d_cc str01_c_cc10020030040050060070005101520253035404550N u m b e r o f i n d i v i d u a l sGenerationsgradual str01_c_cd str01_d_cc str01_c_cc str01_d_cd str01_c_dd str01_d_dd str01_d_dc str01_c_dcFig.2.Evolution of class memory (and).Strategies issued from suchcomplete classes are named strn4(defections punishment of length ))which does win.Itis not exactly the case when those gradual ’s variation are added in complete classes as shown in Figure 3.One example of evolution of a binaryautomata classDue to the explosion of memoryautomata class with and,is a strategy we called str01e 011111C C5000100001500020000020406080100120140160180N u m b e r o f i n d i v i d u a l sGenerationsstr12_cc_cdcddcdd str12_cc_cdcdccdc str12_cc_cdcdcddc str12_cc_cdcddcdc str12_cc_cdcddddc str12_cc_cccddddd str12_cc_cccddcdd str12_cc_cdcddddd str12_cc_cdcdccdd str12_cc_cddddcdd str12_cc_ccdddcdd str12_cc_cdcdcddd str12_cc_cdddccdc str12_cc_cccdcddd str12_cc_cdddcddc str12_cc_cccdccdd str12_cc_cddddcdc str12_cc_cdcdddcd str12_cc_cdddccdd str12_cc_ccddccdd20004000600080001000012000140001600018000020406080100120140160N u m b e r o f i n d i v i d u a l sGenerationsstr12_cc_cdcddcdd str12_cc_cdcdccdc str12_cc_cdcdcddc str12_cc_cdcddcdc str12_cc_cdcddddc str12_cc_cccddddd str12_cc_cdcddddd str12_cc_cccddcdd str12_cc_cdcdccddgraduel_n4str12_cc_cddddcdd str12_cc_ccdddcdd str12_cc_cdcdcddd str12_cc_cdddccdc str12_cc_cdddcddc str12_cc_cccdcddd str12_cc_cccdccdd str12_cc_cddddcdc str12_cc_cdddccdd str12_cc_cdcdddcdFig.3.Evolution of class memory (and).Strategies issued from suchcomplete classes are named strmemory (and).Strategies issued fromsuch complete classes are named str bfor5001000150020002500020406080100120140160N u m b e r o f i n d i v i d u a l sGenerationsstr01e_c_0111_cccd str01e_c_1111_cccd str01e_c_0000_ccdc str01e_c_0000_ccdd str01e_c_0000_cddc str01e_c_0000_cddd str01e_c_0001_ccdc str01e_c_0001_ccdd str01e_c_0001_cddc str01e_c_0001_cddd str01e_c_0010_ccdd str01e_c_0011_ccdd str01e_c_0100_ccdc str01e_c_0100_ccdd str01e_c_0100_cddc str01e_c_0100_cddd str01e_c_0101_ccdc str01e_c_0101_ccdd str01e_c_0101_cddc str01e_c_0101_cddd1000200030004000500020406080100120140160N u m b e r o f i n d i v i d u a l sGenerationsgradualstr01e_c_0111_cccd str01e_c_1111_cccd str01e_c_0000_ccdd str01e_c_0000_ccdc str01e_c_0101_ccdd str01e_c_0000_cddc str01e_c_0000_cddd str01e_c_1111_ccdd str01e_c_0100_cddd str01e_c_0101_ccdc str01e_c_1110_ccdd str01e_c_0100_cddc str01e_c_1000_ccdd str01e_c_1011_ccdd str01e_c_0101_cddc str01e_c_0101_cddd str01e_c_0110_ccdd str01e_c_0001_ccdc str01e_c_0001_ccddFig.5.Evolution of class memorygenotype .Fig.6.Best strategy of class memoryReferences1.R.Axelrod.The Evolution of Cooperation.Basic Books,New York,USA,1984.2. B.Beaufils,J.Delahaye,and P.Mathieu.Our meeting with gradual,a good strategy for theiterated prisoner’s dilemma.In ngton and K.Shimohara,editors,Artificial Life V: Proceedings of the Fifth International Workshop on the Synthesis and Simulation of Living Systems,pages202–209,Cambridge,MA,USA,1996.The MIT Press/Bradford Books.3.M.M.Flood.Some experimental games.Research memorandum RM-789-1-PR,RANDCorporation,Santa-Monica,CA,USA,June1952.4.K.Lindgren.Evolutionary phenomena in simple dynamics.In ngton,C.Taylor,J.D.Farmer,and S.Rasmussen,editors,Artificial Life II:Proceedings of the Second Interdisci-plinary Workshop on the Synthesis and Simulation of Living Systems,volume10of Santa Fe Institute Studies in the Sciences of Complexity,pages295–312,Reading,MA,USA,1992.Addisson–Wesley.5.W.Poundstone.Prisoner’s Dilemma:John von Neumann,Game Theory,and the Puzzle ofthe Bomb.Oxford University Press,Oxford,UK,1993.6. A.Salhi,H.Glaser,D.D.Roure,and J.Putney.The prisoner’s dilemma revisited.Tech-nical Report DSSE-TR-96-2,University of Southhampton,Department of Electronics and Computer Science,Declarative Systems and Software Engineering Group,Southampton,UK, March1996.7.T.W.Sandholm and R.H.Crites.Multiagent reinforcement learning in the iterated prisoner’sdilemma.BioSystems,37(1,2):147–166,1996.8.J.von Neumann and O.Morgenstern.Theory of Games and Economics Behavior.PrincetonUniversity Press,Princeton,NJ,USA,1944.。