Dual systems of sequents and tableaux for many-valued logics
系统生物学简介systemsbiology
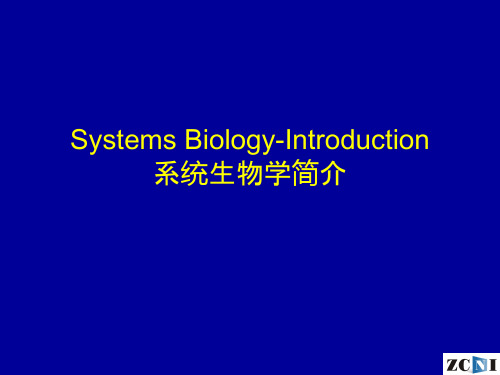
Database, schema standards
Modelling; ODEs, Constraint-based optimisation, Solving inverse problems, Novel strategies
Iteration between theory and experiment
一个自稳态系统是一个开放系统,通过由相互依赖的调控 机制严格控制的多重动态平衡来维持其结构和功能
History
• Term coined at 1960s, however theoretical people and experimental biologists diverged • Renaissance at 1990s
Genome-wide protein-metabolite binding constants Regulatory interactions Genome-wide protein-protein binding constants
Experimentation Analysis
Genome-wide high-throughput enzyme kinetics
Model organism/ system of choice
Transcriptome Proteome Metabolome
New theory
New methodology
Genome-wide protein-inhibitor binding constants (Chemical genetics)
E. Schrödinger (1944) What is life? Cambridge University Press
【高中生物】单细胞测序,你为何如此令人痴迷
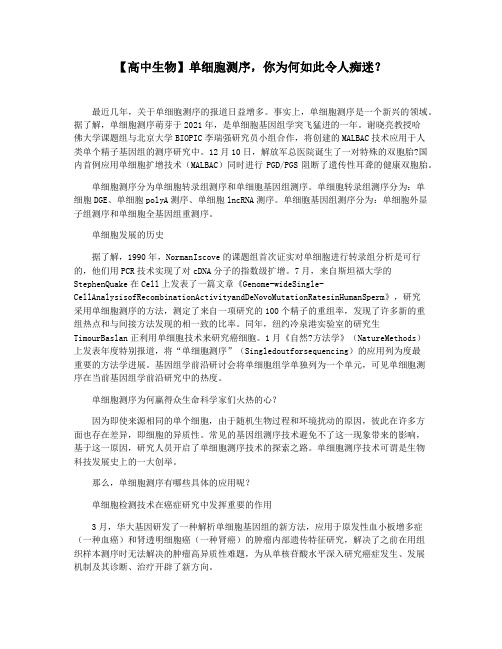
【高中生物】单细胞测序,你为何如此令人痴迷?最近几年,关于单细胞测序的报道日益增多。
事实上,单细胞测序是一个新兴的领域。
据了解,单细胞测序萌芽于2021年,是单细胞基因组学突飞猛进的一年。
谢晓亮教授哈佛大学课题组与北京大学BIOPIC李瑞强研究员小组合作,将创建的MALBAC技术应用于人类单个精子基因组的测序研究中。
12月10日,解放军总医院诞生了一对特殊的双胞胎?国内首例应用单细胞扩增技术(MALBAC)同时进行PGD/PGS阻断了遗传性耳聋的健康双胞胎。
单细胞测序分为单细胞转录组测序和单细胞基因组测序。
单细胞转录组测序分为:单细胞DGE、单细胞polyA测序、单细胞lncRNA测序。
单细胞基因组测序分为:单细胞外显子组测序和单细胞全基因组重测序。
单细胞发展的历史据了解,1990年,NormanIscove的课题组首次证实对单细胞进行转录组分析是可行的,他们用PCR技术实现了对cDNA分子的指数级扩增。
7月,来自斯坦福大学的StephenQuake在Cell上发表了一篇文章《Genome-wideSingle-CellAnalysisofRecombinationActivityandDeNovoMutationRatesinHumanSperm》,研究采用单细胞测序的方法,测定了来自一项研究的100个精子的重组率,发现了许多新的重组热点和与间接方法发现的相一致的比率。
同年,纽约冷泉港实验室的研究生TimourBaslan正利用单细胞技术来研究癌细胞。
1月《自然?方法学》(NatureMethods)上发表年度特别报道,将“单细胞测序”(Singledoutforsequencing)的应用列为度最重要的方法学进展。
基因组学前沿研讨会将单细胞组学单独列为一个单元,可见单细胞测序在当前基因组学前沿研究中的热度。
单细胞测序为何赢得众生命科学家们火热的心?因为即使来源相同的单个细胞,由于随机生物过程和环境扰动的原因,彼此在许多方面也存在差异,即细胞的异质性。
全能型酵母双杂交系统和蛋白表达系统
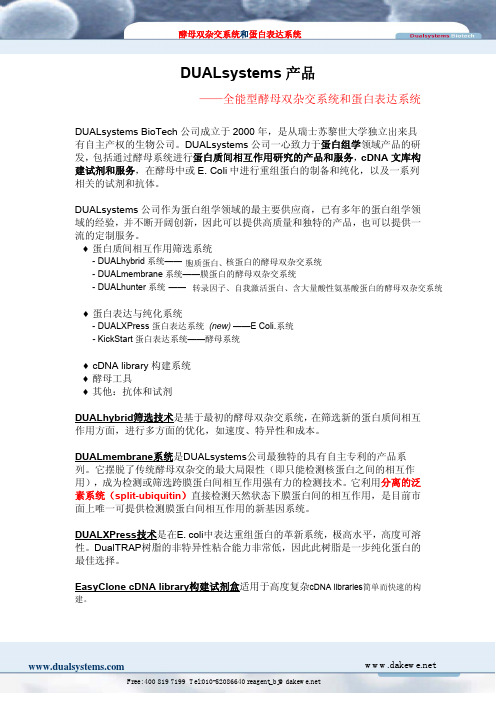
Free: 400 819 7199 Tel:010-52086640 reagent_bj@
2
酵母双杂交系统和蛋白表达系统
两个待测蛋白存在相互作用,那么融合蛋白上的 DBD 和 AD 就能重新形成有活 性的转录激活因子,从而激活靶基因的表达(图 E)。人为地将靶基因构建为表达 蛋白容易鉴定的基因,称为报告基因(report gene),从而通过检测报告基因 的表达,来筛选这两个待测蛋白是否存在相互作用。 那么这两个融合蛋白是如何构建的?又是如何进入同一个酵母细胞的呢?将 bait 和 prey 两个融合蛋白的基因分别克隆到两个质粒载体中,构建两个重组质 粒,然后将两个重组质粒共同导入酵母细胞中。从而就可以实现在同一个酵母细 胞中同时表达两个融合蛋白。
向是最常用的方向。
优势:- 两个方向的载体:避免自我激活问题的出现;增加发现新的相互作用的机会;
- LexA 系统:降低了假阳性。
Free: 400 819 7199 Tel:010-52086640 reagent_bj@
3
(lexAop)8-ADE2 GAL4
NMY51 酵母采用改良后的,三个独立的报告基因:HIS3, ADE2 和 lacZ,第 一步通过营养缺陷型报告基因(HIS3, ADE2)进行选择性生长筛选,进一步通 过 LacZ 报告基因进行 β-半乳糖分析显色的定量或半定量筛选。
优势:含有三个独立的报告基因,受不同启动子的调控,降低假阳性几率。
4
酵母双杂交系统和蛋白表达系统
- 成功机率高; - 是新研究者的最佳选择
DUALsystems 公司已为全球诸多知名企业、研究所、医院提供了定制服务,如
Roche AG(Switzerland), Cancer Research UK(group of Prof. Steve Jackson), Mount Sinai Hospital(New York), Prionics AG(Switzerland)……
课程名称中英文对照参考表

外国文学作选读Selected Reading of Foreign Literature现代企业管理概论Introduction to Modern Enterprise Managerment电力电子技术课设计Power Electronics Technology Design计算机动画设计3D Animation Design中国革命史China’s Revolutionary History中国社会主义建设China Socialist Construction集散控制DCS Distributed Control计算机控制实现技术Computer Control Realization Technology计算机网络与通讯Computer Network and CommunicationERP/WEB应用开发Application & Development of ERP/WEB数据仓库与挖掘Data Warehouse and Data Mining物流及供应链管理Substance and Supply Chain Management成功心理与潜能开发Success Psychology & Potential Development信息安全技术Technology of Information Security图像通信Image Communication金属材料及热加工Engineering Materials & Thermo-processing机械原理课程设计Course Design for Principles of Machine机械设计课程设计Course Design for Mechanical Design机电系统课程设计Course Design for Mechanical and Electrical System 创新成果Creative Achievements课外教育Extracurricular education。
雌性生殖干细胞全基因组范围选择性多聚腺苷酸化位点分析

雌性生殖干细胞全基因组范围选择性多聚腺苷酸化位点分析沈挺挺;张晓丽;张盼;康亚妮;田静;赵小东【摘要】目的描绘小鼠雌性生殖干细胞(FGSCs)和胚胎干细胞(ESCs)全基因组范围内选择性多聚腺苷酸化(APA)位点,通过细胞间的比较,分析生殖干细胞特异性的APA位点对其生物学特性的影响.方法利用本实验室之前建立的基于高通量测序的转录本3'末端poly(A)位点捕获方法3T-seq确定并比较两个细胞系中所有APA 位点,通过DAVID对具有APA位点差异的基因进行Gene Ontology分析.结果在两种细胞系中共确定16 973个基因的50 243个APA位点,发现FGSCs中相较于ESCs3'UTR发生长度变化的基因1148个,其中795个(66%)3' UTR变短,353个(34%)基因3'UTR变长,与生殖发育密切相关的基因3'UTR存在显著的缩短现象.结论 FGSCs存在与ESCs不同的APA谱,APA介导的3'UTR变化参与生殖发育相关的生物学过程,揭示了生殖干细胞的基因转录后调控机制.【期刊名称】《南方医科大学学报》【年(卷),期】2016(036)002【总页数】6页(P157-162)【关键词】雌性生殖干细胞;小鼠胚胎干细胞;全基因组APA谱【作者】沈挺挺;张晓丽;张盼;康亚妮;田静;赵小东【作者单位】上海交通大学系统生物医学教育部重点实验室,上海 200240;上海交通大学生物医学工程学院Bio-ID研究中心,上海 200240;西北大学生命科学学院,陕西西安 710069;上海交通大学生物医学工程学院Bio-ID研究中心,上海 200240;西北大学生命科学学院,陕西西安 710069;上海交通大学生物医学工程学院Bio-ID 研究中心,上海 200240【正文语种】中文选择性多聚腺苷化(APA)是一类很重要的基因转录后表达调控方式,通过调节转录本3’非翻译区(3’untranslated region, UTR)的长度来影响包括mRNA 的稳定性、亚细胞定位和翻译效率等在内的多个生物学过程[1-3]。
遗传算法在生物信息学中的应用
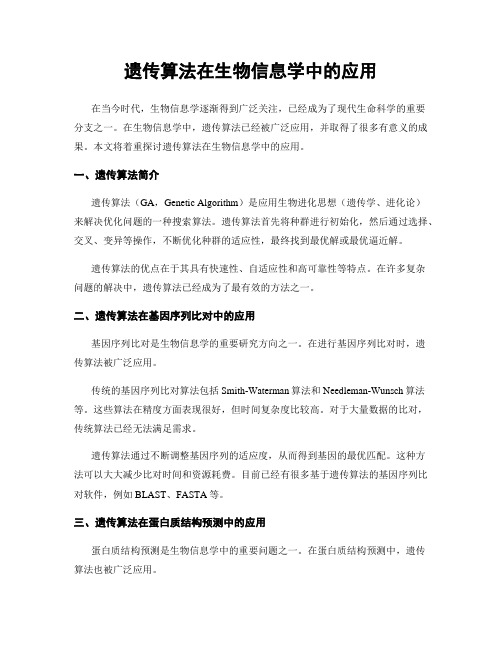
遗传算法在生物信息学中的应用在当今时代,生物信息学逐渐得到广泛关注,已经成为了现代生命科学的重要分支之一。
在生物信息学中,遗传算法已经被广泛应用,并取得了很多有意义的成果。
本文将着重探讨遗传算法在生物信息学中的应用。
一、遗传算法简介遗传算法(GA,Genetic Algorithm)是应用生物进化思想(遗传学、进化论)来解决优化问题的一种搜索算法。
遗传算法首先将种群进行初始化,然后通过选择、交叉、变异等操作,不断优化种群的适应性,最终找到最优解或最优逼近解。
遗传算法的优点在于其具有快速性、自适应性和高可靠性等特点。
在许多复杂问题的解决中,遗传算法已经成为了最有效的方法之一。
二、遗传算法在基因序列比对中的应用基因序列比对是生物信息学的重要研究方向之一。
在进行基因序列比对时,遗传算法被广泛应用。
传统的基因序列比对算法包括Smith-Waterman算法和Needleman-Wunsch算法等。
这些算法在精度方面表现很好,但时间复杂度比较高。
对于大量数据的比对,传统算法已经无法满足需求。
遗传算法通过不断调整基因序列的适应度,从而得到基因的最优匹配。
这种方法可以大大减少比对时间和资源耗费。
目前已经有很多基于遗传算法的基因序列比对软件,例如BLAST、FASTA等。
三、遗传算法在蛋白质结构预测中的应用蛋白质结构预测是生物信息学中的重要问题之一。
在蛋白质结构预测中,遗传算法也被广泛应用。
蛋白质结构预测的难点在于蛋白质的复杂性和多样性。
传统的蛋白质结构预测算法需要大量的时间和资源,而且精度也难以保证。
遗传算法通过不断优化蛋白质的结构,从而得到最优的蛋白质结构,具有极高的准确性和快速性。
目前已经有很多基于遗传算法的蛋白质结构预测软件,例如Rosetta、SwissModel等。
这些软件已经被广泛应用于生命科学研究和临床治疗中。
四、遗传算法在基因表达数据分析中的应用基因表达数据分析是生物信息学中的一个热门领域。
在基因表达数据分析中,遗传算法也得到了广泛应用。
全能型酵母双杂交系统和蛋白表达系统
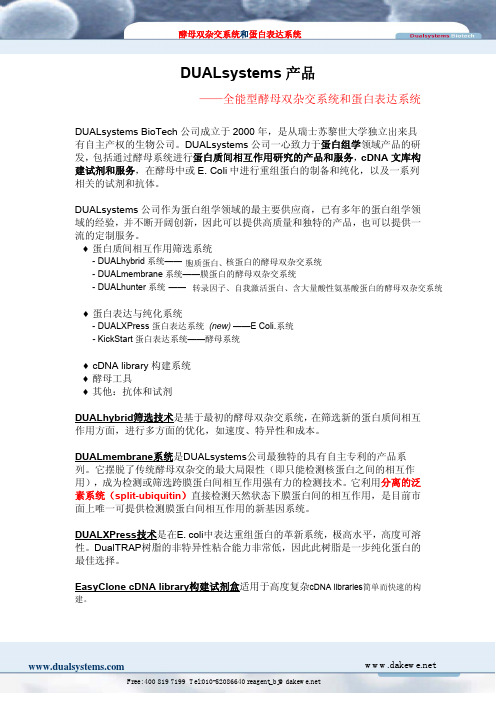
1.4 DUALhybrid 定制筛选服务
DUALsystems 公司除了提供 DUALhybrid 试剂盒外,还提供定制服务。此定制
服务分为两个独立的部分,研究者可以根据自己的需要,可选择其中的一部分或
选择完整的酵母双杂交服务。
Part I:
bai vector
提供用于酵母三杂交的载体。 优势:适用于酵母三杂交检测系统。
4)control plasmids
• pLexA-laminC, pLexA-p53 and pGAD-largeT
优势:降低假阳性,提高筛选的严谨性
5)Yeast
1.5 文献
综述: A novel genetic system to detect protein-protein interactions. Fields and Song Nature. 340, 245-6 (1989)
DUALmembrane系统是DUALsystems公司最独特的具有自主专利的产品系 列。它摆脱了传统酵母双杂交的最大局限性(即只能检测核蛋白之间的相互作 用),成为检测或筛选跨膜蛋白间相互作用强有力的检测技术。它利用分离的泛 素系统(split-ubiquitin)直接检测天然状态下膜蛋白间的相互作用,是目前市 面上唯一可提供检测膜蛋白间相互作用的新基因系统。
优势: -
研究者无需耗时建立酵母双杂交系统; 省略实验摸索的步骤,减少试剂的浪费,和大量的时间消耗; DUALsystems 公司已提供 超过10年的定制服务,经验丰富;
Free: 400 819 7199 Tel:010-52086640 reagent_bj@
酵母双杂交系统和蛋白表达系统
2)1 prey vector
Collaborative networks and product innovation performance--Toward a contingency perspective
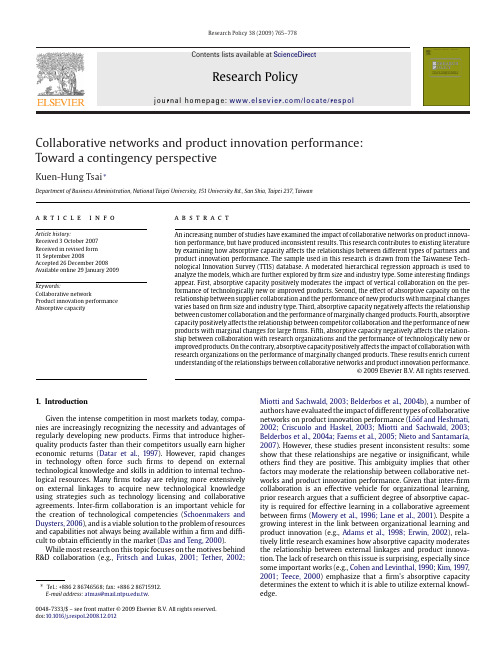
Research Policy 38(2009)765–778Contents lists available at ScienceDirectResearchPolicyj o u r n a l h o m e p a g e :w w w.e l s e v i e r.c o m /l o c a t e /r e s p olCollaborative networks and product innovation performance:Toward a contingency perspectiveKuen-Hung Tsai ∗Department of Business Administration,National Taipei University,151University Rd.,San Shia,Taipei 237,Taiwana r t i c l e i n f o Article history:Received 3October 2007Received in revised form 11September 2008Accepted 26December 2008Available online 29January 2009Keywords:Collaborative networkProduct innovation performance Absorptive capacitya b s t r a c tAn increasing number of studies have examined the impact of collaborative networks on product innova-tion performance,but have produced inconsistent results.This research contributes to existing literature by examining how absorptive capacity affects the relationships between different types of partners and product innovation performance.The sample used in this research is drawn from the Taiwanese Tech-nological Innovation Survey (TTIS)database.A moderated hierarchical regression approach is used to analyze the models,which are further explored by firm size and industry type.Some interesting findings appear.First,absorptive capacity positively moderates the impact of vertical collaboration on the per-formance of technologically new or improved products.Second,the effect of absorptive capacity on the relationship between supplier collaboration and the performance of new products with marginal changes varies based on firm size and industry type.Third,absorptive capacity negatively affects the relationship between customer collaboration and the performance of marginally changed products.Fourth,absorptive capacity positively affects the relationship between competitor collaboration and the performance of new products with marginal changes for large firms.Fifth,absorptive capacity negatively affects the relation-ship between collaboration with research organizations and the performance of technologically new or improved products.On the contrary,absorptive capacity positively affects the impact of collaboration with research organizations on the performance of marginally changed products.These results enrich current understanding of the relationships between collaborative networks and product innovation performance.©2009Elsevier B.V.All rights reserved.1.IntroductionGiven the intense competition in most markets today,compa-nies are increasingly recognizing the necessity and advantages of regularly developing new products.Firms that introduce higher-quality products faster than their competitors usually earn higher economic returns (Datar et al.,1997).However,rapid changes in technology often force such firms to depend on external technological knowledge and skills in addition to internal techno-logical resources.Many firms today are relying more extensively on external linkages to acquire new technological knowledge using strategies such as technology licensing and collaborative agreements.Inter-firm collaboration is an important vehicle for the creation of technological competencies (Schoenmakers and Duysters,2006),and is a viable solution to the problem of resources and capabilities not always being available within a firm and diffi-cult to obtain efficiently in the market (Das and Teng,2000).While most research on this topic focuses on the motives behind R&D collaboration (e.g.,Fritsch and Lukas,2001;Tether,2002;∗Tel.:+886286746568;fax:+886286715912.E-mail address:atmas@.tw .Miotti and Sachwald,2003;Belderbos et al.,2004b ),a number of authors have evaluated the impact of different types of collaborative networks on product innovation performance (Lööf and Heshmati,2002;Criscuolo and Haskel,2003;Miotti and Sachwald,2003;Belderbos et al.,2004a;Faems et al.,2005;Nieto and Santamaría,2007).However,these studies present inconsistent results:some show that these relationships are negative or insignificant,while others find they are positive.This ambiguity implies that other factors may moderate the relationship between collaborative net-works and product innovation performance.Given that inter-firm collaboration is an effective vehicle for organizational learning,prior research argues that a sufficient degree of absorptive capac-ity is required for effective learning in a collaborative agreement between firms (Mowery et al.,1996;Lane et al.,2001).Despite a growing interest in the link between organizational learning and product innovation (e.g.,Adams et al.,1998;Erwin,2002),rela-tively little research examines how absorptive capacity moderates the relationship between external linkages and product innova-tion.The lack of research on this issue is surprising,especially since some important works (e.g.,Cohen and Levinthal,1990;Kim,1997,2001;Teece,2000)emphasize that a firm’s absorptive capacity determines the extent to which it is able to utilize external knowl-edge.0048-7333/$–see front matter ©2009Elsevier B.V.All rights reserved.doi:10.1016/j.respol.2008.12.012766K.-H.Tsai/Research Policy38(2009)765–778The present study therefore addresses the question:Dofirms with a high level of absorptive capacity realize higher product innovation from close collaboration thanfirms with a low level of absorptive capacity?Answering this question can make a signif-icant contribution to the literature on this topic.While previous studies focus on the effects of collaborative networks on prod-uct innovation performance,this paper proposes a contingency framework to address the value of absorptive capacity in explain-ing the relationship between collaborative networks and product innovation performance.Additionally,this study advances research on absorptive capacity by empirically examining its effect on the use of external knowledge for product innovation.Answers to the question of absorptive capacity are also important because they are relevant tofirms that depend to a large extent on technol-ogy acquired from collaborating with different partners.In their efforts to reduce the costs and risk of technology development and to introduce higher-quality products faster than competitors,firms may count heavily on the effectiveness with which they can gain access to external sources of technological knowledge and skills. While some previous studies suggest that collaborating with dif-ferent partners is an effective way to improve product innovation (e.g.,Belderbos et al.,2004a;Nieto and Santamaría,2007),this research sheds light on the importance of absorptive capability in the effectiveness of collaborative networks.The remainder of this paper is organized as follows.Follow-ing this introduction,Section2reviews the literature and provides theoretical expectations.Section3introduces the research meth-ods,including the model,variable definitions and measurements, and the data source utilized in this study.Section4presents the results and discussions.Section5summarizes the results,discusses their implications for theory and managerial practice,and suggests possible directions for future research.2.Literature review and research hypotheses2.1.The impact of different types of partnersPrior research suggests that afirm can advance its product innovation by interacting with different collaborators,primarily including suppliers,customers,competitors,and research orga-nizations.Suppliers usually have greater expertise and more comprehensive knowledge regarding the parts and components which may be critical to afirm’s new product development.Thus, supplier collaboration can allowfirms to incorporate the exper-tise and different perspective of a supplier to improve its solutions or create new methods for product development(Bonaccorsi and Lipparine,1994;Eisenhardt and Tabrizi,1995).Supplier involve-ment also helpsfirms identify potential technical problems,thereby speeding up new product development and responses to market demands(Kessler and Chakrabatri,1996).Miotti and Sachwald (2003)used the French CIS-2survey to reveal the positive effect of collaboration with suppliers on the share of innovative prod-uct turnover.Faems et al.(2005)analyzed Belgian manufacturing firms and found a positive association between suppliers and the proportion of turnover attributed to improved products.In a sur-vey of Spanish manufacturingfirms,Nieto and Santamaría(2007) regressed product innovation on collaborative networks and found a positive link between collaboration with suppliers and the degree of product innovativeness.However,Sánchez and Pérez(2003) analyzed Spanish manufacturingfirms and concluded that collabo-rating with suppliers does not improve new product performance. Freel(2003)analyzed UK small and medium-sized manufacturing firms and found that supplier collaboration does not have a signif-icant impact on new product performance.Ledwith and Coughlan (2005)used a sample of electronicsfirms in Ireland and the UK and found an insignificant correlation between collaboration with sup-pliers and product innovation performance.In addition,Belderbos et al.(2004a)studied Dutch manufacturingfirms and found a negative but insignificant relationship between collaboration with suppliers and product innovation performance.Collaborating with customers is another important way for a firm to improve its product innovation performance(Gupta et al.,2000;Fritsch and Lukas,2001;Brockhoff,2003).Working with customers not only provides benefits in identifying market opportunities for technology development,but also reduces the likelihood of poor design in the early stages of development.In addition,understanding the needs of influential customers may helpfirms gain new ideas about solutions(von Hippel et al.,1999) and identify market trends early on,thereby increasing the chances of new product development and success.Thus,customer involve-ment may lead to product innovation advantages(Souder et al., 1997;Li and Calantone,1998).Miotti and Sachwald(2003),Freel (2003),and Faems et al.(2005)all found that collaboration with customers has a positive impact on product innovation perfor-mance.In contrast,Lööf and Heshmati(2002)analyzed Swedish manufacturingfirms and found a negative relationship between customer collaboration and product innovation performance.Nieto and Santamaría(2007)found that customer collaboration has a positive impact on product innovation with marginal changes,but does not affect significant innovation with new functions.In addi-tion,Belderbos et al.(2004a)revealed an insignificant association between collaboration with customers and changes in new product sales.Monjon and Waelbroeck(2003)analyzed French manufactur-ingfirms and found that customer collaboration has an insignificant impact on product innovation.The least frequent type of collaborative network thatfirms adopt to achieve product innovation seems to be collaboration with com-petitors(Bayona et al.,2001;Nieto and Santamaría,2007),but this type of collaboration still provides some advantages.Firms involved in a cooperative agreement may share technological knowledge and skills with each other,producing a synergistic effect on solv-ing common problems outside the competitor’s area of influence (Tether,2002).The case study of Inkpen and Pien(2006)suggests thatfirms collaborating with competitors may perform better in innovation than they would otherwise.At the same time,firms can accelerate their capability development by R&D cooperation,which allows them to reduce the time and risk involved in technologi-cal innovation(Belderbos et al.,2004a).Furthermore,collaborating with competitors enablesfirms to ascertain their competitors’tech-nological level;firms that are more knowledgeable about their competitors’technology strategies are better able to differenti-ate themselves(Linn,1994).Lööf and Heshmati(2002)found that collaborating with competitors is positively related to new prod-uct sales.However,Monjon and Waelbroeck(2003),Miotti and Sachwald(2003),and Belderbos et al.(2004a)found that com-petitor collaboration has a negative but insignificant impact on product innovation performance.Nieto and Santamaría(2007)also found that collaboration with competitors does not impact prod-uct innovation with marginal modifications,but it negatively affects product innovations with new functions.Due to governments’encouragement,more and morefirms are pursuing product innovations by collaborating with universities and research institutions.Universities and research institutes are important centers for the creation and dissemination of scientific knowledge(Hemmert,2004).Firms can interact formally and informally with universities and research institutes to acquire new scientific knowledge to benefit their product or process innovations(Caloghirou et al.,2004).In contrast,afirm choosing not to acquire technological knowledge from universities and research institutions may fall behind,reducing the likelihood that it will make a technological breakthrough leading to a commercial product(Spencer,2003).Several studies suggest that technologicalK.-H.Tsai/Research Policy38(2009)765–778767innovation relies heavily on knowledge from universities and research institutions(Bozeman,2000;McMillan et al.,2000;Vuola and Hameri,2006).Belderbos et al.(2004a),Faems et al.(2005), and Nieto and Santamaría(2007)found that collaboration with research institutes and universities positively affects product inno-vation performance.However,Monjon and Waelbroeck(2003), Caloghirou et al.(2004),and Ledwith and Coughlan(2005)found that collaboration with universities and research institutes has a negative effect on product innovation performance.Furthermore, Lööf and Heshmati(2002)revealed an insignificant relationship between collaboration with research organizations and product innovation performance.In summary,the empirical studies reviewed above show that while there is some support for collaborating with different part-ners in product innovations,there is an absence of consensus on the benefits of this type of networking.Surprisingly,though most of the studies mention the importance of absorptive capacity,they do not investigate its moderating role.Therefore,the way in which absorptive capacity affects the relationship between collaboration and product innovation performance is worthy of further study.2.2.The role of absorptive capacityAbsorptive capacity refers to afirm’s ability to use its own prior related knowledge to recognize,assimilate,and use exter-nal knowledge for its own commercial ends(Cohen and Levinthal, 1990).Zahra and George(2002)and Todorova and Durisin(2007) further characterized absorptive capacity as a bundle offive capa-bilities:recognition,acquisition,assimilation,transformation,and exploitation.Obviously,afirm with a high level of absorptive capac-ity is better able to create and exploit linkages with otherfirms (Caloghirou et al.,2004).The absorptive capacity of afirm is greatly dependent on its current level of technological knowledge(Cohen and Levinthal,1990;Kim,1997,2001),which in turn is derived from previous and current efforts in internal R&D(e.g.,Veugelers,1997; Stock et al.,2001;Schoenmakers and Duysters,2006).Numerous studies argue that a certain degree of absorptive capacity is required for effective learning in inter-organizational collaborations(e.g., Mowery et al.,1996;Kim,1998;Lane and Lubatkin,1998;Lane et al.,2001).Organizations with a greater absorptive capacity usually have a sufficiently developed technology base that enables them to have rich and detailed communications with their suppliers dur-ing the knowledge-sharing process.This communication process, in turn,may generate new ideas or solutions for product designs. Further,suchfirms are more likely to recognize the value of new ideas and effectively integrate them into their product develop-ment efforts.As certain ideas are completely de novo,it is necessary to develop new parts and components to produce significant inno-vations.Unrealistic designs or incompatible parts and components may seriously delay the development cycle(Culley et al.,1999). Thus,close collaboration with suppliers is important during the engineering process to shorten the development time and ensure the quality of new products.In contrast,an organization with a lower absorptive capacity mayfind it difficult to recognize the value of new ideas which emerge from interactions with their suppliers.Afirm may also lack sufficient ability to integrate ideas into new products.In this case, close collaboration with suppliers may waste time and money and, as a result,inhibit new product performance.Based on these argu-ments,this study expects that the greater the absorptive capacity, the stronger the relationship between collaboration with suppliers and product innovation performance.Absorptive capacity can also enhance the use of knowledge from customer collaboration in product innovation.In working closely with customers,and particularly with influential customers,afirm may uncover latent customer needs(Atuahene-Gima et al.,2005). These unarticulated needs alert thefirm to new market opportu-nities,technology developments,and ideas that challenge existing cause-effect relationships,thereby resulting in new products with significant benefianizations with a greater absorptive capac-ity are more likely to identify,convert,and exploit these needs using new technological knowledge.Absorptive capacity,then,improves afirm’s chances of capturing new market opportunities by mak-ing innovative products.Hence,afirm’s performance in product innovation may be improved by close customer collaboration.Con-versely,an organization lacking sufficient absorptive capacity will be unable to integrate the latent customer needs into new product developments.Thus,even if afirm collaborates closely with cus-tomers,these activities may not increase thefirm’s performance in product innovation,and may even be detrimental to such per-formance.Based on the arguments above,this study proposes that the greater the absorptive capacity,the stronger the relation-ship between collaboration with customers and product innovation performance.The impact of any external knowledge absorbed from com-petitor collaboration on product innovation may also depend on absorptive capacity.By establishing collaborative arrangements, afirm can access the specialized knowledge of its competitors, which is usually tacit and cannot be easily copied by simple obser-vation.Inkpen and Pien’s(2006)case study suggests thatfirms with a sufficiently developed technology base are able to iden-tify and understand the knowledge that underpins similarities and differences in their collaborators’skills.As a result,they may be more likely to incorporate competitor knowledge and expertise in their own technological innovations.Further,the research of Kim and Song(2007)suggests that absorptive capacity may facilitate the creation of new technology through collaboration with other companies.Afirm with a greater absorptive capacity has a better technology base that enables it to understand and exploit competi-tors’skills and knowledge(Cohen and Levinthal,1990),thereby resulting in significantly innovative products.For example,given that Hewlett–Packard(HP)had developed its own laser printing capabilities,it chose to work with canon to develop the desk-top laser printer and achievefirst-mover advantages in personal laser printers(Helleloid and Simonin,1994).Therefore,this study hypothesizes that the greater the absorptive capacity,the stronger the impact of collaboration with competitors on product innovation performance.Research organizations are an important source of new scien-tific knowledge.Collaborating with research organizations enables afirm to access scientific knowledge previously unexplored.This knowledge may provide thefirm with different modes of reason-ing,problem formulation,and solutions(Amabile,1988).Exposure to these different approaches adds to the repertoire that afirm can bring to bear on new product development problems.The process of combining new technological knowledge into existing knowl-edge can foster insights that can then lead to additional insights and profundity,thereby offering significantly higher potential for breakthrough innovations(Ahuja and Lampert,2001).However, case studies and empirical evidence show that absorptive capacity is important for afirm,especially for SMEs,to achieve success-ful collaboration with research institutions(Koschatzky,2002; Hadjimanolis,2006).While collaborating with research organiza-tions,firms with a high level of absorptive capacity are better able to learn new perspectives that may provide better,more effective solutions in new product development.In contrast,an organization that lacks sufficient absorptive capacity may be unable to digest advanced technologies when closely collaborating with research organizations.Thus,this study hypothesizes that the greater the absorptive capacity,the stronger the impact of collaboration with research organizations on product innovation performance.768K.-H.Tsai /Research Policy 38(2009)765–778In summary,with an adequate degree of absorptive capacity,firms will be better at internalizing their partners’knowledge,and thereby improve their chances for product innovations.Whether or not the benefits of collaborating with different types of partners can be realized may be affected by the parent organization’s absorptive capacity as derived from its existing technological knowledge.Con-versely,an inability to identify and understand the technological knowledge that underpins partners’competencies limits a firms’collaborative learning potential.3.Research method 3.1.Conceptual frameworkFig.1shows the conceptual framework investigated in this study.This framework indicates that the product innovation performance of a firm is affected by its collaborative networks in terms of differ-ent types of partners.It further proposes that these relationships are influenced by the absorptive capacity of a firm.In addition,sev-eral important controls are included in the model to eliminate or reduce the bias arising from the confounding effects.This frame-work guides the definitions and measures of the major variables used in this study.3.2.Variable definitions3.2.1.Dependent and independent variablesThe dependent variable in this study is product innovation per-formance,which is measured by innovative sales productivity.This study operationalizes this measure as the sales generated by new products per employee (i.e.,the ratio of sales attributed to new products divided by the total number of employees).These sales include (1)technologically new or technologically improved prod-ucts introduced to the market within the past 3years,and (2)marginally changed products within the same time period.Note that this study does not measure product innovation performance by the volume of new product sales because this measure signif-icantly correlates with firm size.A technologically new product is a product whose technological characteristics or intended uses differ significantly from those of existing products (OECD,1997).A technologically improved product refers to an existing product whose performance has been significantly improved or upgraded (OECD,1997).A marginally changed product is an innovative prod-uct that cannot be categorized into either of the first two groups.The independent variables in this study are the four types of col-laboration with different partners,including suppliers,customers,Fig.1.Conceptual framework.competitors,or research institutes and universities.These variables measure the level of collaboration with different types of partners.This study constructs each of these variables by the product of two variables in the TTIS database.One is a dummy variable which takes the value of 1if the firm is engaged in collaboration with a specific type of partner,else 0;the other is the relative importance (high,medium,and low)of collaboration with this partner,indicating how close the collaboration is.3.2.2.Moderator and controlsThe moderating variable in this research is absorptive capacity.The absorptive capacity of a firm depends greatly on its existing technological knowledge base (Cohen and Levinthal,1990;Kim,1997,2001).If a firm lacks a sufficiently developed technologi-cal knowledge base,it may have difficult absorbing any externally acquired technological knowledge (Schoenmakers and Duysters,2006).Firms can only be expected to learn from their collaboration partners if they have some level of prior technological knowledge which they can us to incorporate their partners’knowledge and use it for their own purposes.Prior research views in-house R&D invest-ment as the key determinant of a firm’s absorptive capacity (e.g.,Cohen and Levinthal,1990;Mowery et al.,1996;Stock et al.,2001;Carayannis and Alexander,2002;Todorova and Durisin,2007).The absorptive capacity variable is measured by dividing the firm’s total expenditures on in-house R&D activities and training programs for technological activities in the past 3years by its total number of employees in a current year.Note that the numerator of the vari-able is a stock measure,as in previous studies (e.g.,Helfat,1997;Ahuja and Katila,2001),usually used as a proxy for the firm’s tech-nology base acquired from previous and current R&D or training activities.However,this study does not use in-house R&D or train-ing stock to measure a firm’s internal efforts in innovation activities because this measure is always highly correlated with firm size.This type of measure represents a firm’s absorptive capacity more accurately than the R&D intensity measure (R&D expenditure/sales)widely used in prior research (e.g.,Jones et al.,2001;Belderbos et al.,2004a;Faems et al.,2005;Schoenmakers and Duysters,2006;Nieto and Santamaría,2007).More importantly,this measure reflects existing knowledge accumulated from past learning and intensity of effort,which are both important elements of absorptive capacity (Cohen and Levinthal,1990;Kim,1997,2001).The research model in this study also contains several impor-tant controls.The first control is the use of industry dummies for fixed industry effects.As stressed in prior research,these dummies capture various environmental dimensions such as tech-nological opportunity and competition intensity (e.g.,Veugelers,1997;McGahan and Porter,1997).This analysis uses seven indus-try dummies representing eight traditional manufacturing sectors.The second control is the size of the firm as measured by its total number of employees,which is a proxy for size in previous studies related to innovation performance (e.g.,Caloghirou et al.,2004;Schoenmakers and Duysters,2006).Next,the average ratio of employees with a university degree or higher by total number of employees serves as a proxy for the quality of the firm’s human resources,which is an important determinant of innovation output in the literature (e.g.,Rothwell and Dodgson,1991;Jones,2001).In addition,this study controls for the effects of inward technology licensing since previous studies emphasize the role of this control on innovation (e.g.,Zahra et al.,2005;Tsai and Wang,2007).Inward technology licensing in this study comprises the firm’s expendi-tures on external technology acquisition through inward licensing.The last control is a dichotomous variable that takes the value of 1if the firm is mostly foreign owned;else zero.Previous studies suggest that the subsidiary of a foreign parent company may per-form better in bringing new product products to the market than a host company (e.g.,Deeds and Hill,1996;Belderbos et al.,2004a ).K.-H.Tsai/Research Policy38(2009)765–778769Table1Means,standard deviations,and correlations(N=753).Variable PIP CL S CL C CL P CL R ACAP FS HQ ITL SBPIP 1.000CL S0.084b 1.000CL C0.116a0.435a 1.000CL P−0.0030.219a0.237a 1.000CL R0.0500.116a0.062c0.083b 1.000ACAP0.361a0.074b0.129a−0.003−0.001 1.000FS0.159a0.153a0.180a0.0030.270a0.058 1.000LQ0.123a0.094a0.0340.011−0.086b0.080b−0.039 1.000ITL0.131a0.081b0.142a0.0510.103a0.210a0.309a0.050 1.000SB0.044−0.070c−0.026−0.058−0.0110.0120.060c−0.0430.021 1.000Mean50.60.2590.4290.0900.2370.253444.410.226 5.8010.072 S.D.75.60.739 1.0020.4310.6090.6181215.000.22633.870.258Notes:(1)PIP:product innovation performance;CL S:collaboration with suppliers;CL C:collaboration with customer;CL P:collaboration with competitors;CL R:collaboration with research organizations;ACAP:absorptive capacity;FS:firm size;LQ:labor quality;ITL:inward technology licensing;SB:subsidiary.(2)Unit of analysis for PIP is thousand NT dollars;and ITL is million NT dollars;FS,person.a p<0.01.b p<0.05.c p<0.10.Therefore,this study uses this variable to control for the effects of thefirm’s managerial style on innovation output.3.3.The dataThis study analyzes data at thefirm level.Both the sample and the variables used in this analysis come from the Taiwanese Techno-logical Innovation Survey(TTIS),jointly conducted by the National Science Council and the Ministry of Economic Affairs in2002.The sample is representative of the population of traditional Taiwanese manufacturingfirms because the sampling frame was generated by a stratified random sampling process based onfirm size and industry.The database consists of1346firms in various manufac-turing industries.Among this total,firms indicating that they had not engaged in technological innovation activities during the pre-vious3years were excluded from this study since their profiles do not contain any data for external technology sourcing variables. The sample includes a total of753firms for the preliminary anal-ysis and the model estimation.Thesefirms are categorized into eight sectors:food,beverages&tobacco(40firms,5.31%);textile, wearing apparel&leather(56firms,7.44%);paper&printing(29firms,3.85%);chemical,rubber&plastic(130firms,17.26%);non-metallic mineral(28firms,3.72%);basic metal(22firms,2.92%); fabricated metal(108firms,14.34%);and machinery,electronics &transportation equipments(340firms,45.15%).Within the sam-ple,the percentage of reported collaboration with suppliers,clients, competitors,and research organizations is about20%,18%,8%,and 23%,respectively.Thesefigures reveal that competitor collaboration does not seem to be the best way to improve innovation.This may be caused by problems of technological knowledge leakage and the increased risk of hold up in competitor collaboration(Bayona et al., 2001).Thisfinding is consistent with that of Nieto and Santamaría (2007).Moreover,collaboration with research organizations seems to be the most frequent type of partnership.This phenomenon is unlike that of a large number of European countries documented in Drejer and Jørgensen(2005)and Nieto and Santamaría(2007), where collaboration with suppliers was the most important.Table1 reports the basic statistics for the variables(except for the industry dummies)used in the analysis.Table1shows some interestingfindings.First,all of the correlation coefficients for the collaboration variables(except collaboration with research organizations)achieve a statistical sig-nificance at the5%significance level.This indicates that a certain proportion of thefirms within the sample collaborate with more than one type of partner for technological innovations.In particu-lar,firms collaborating with suppliers tend to also collaborate with their customers(r=0.44,p<0.01).Second,competitor collabora-tion has the lowest value in its mean and standard deviations among the collaboration variables.This result shows that collaboration with competitors is the least common type of partnership for prod-uct innovation within the sample.Third,a significant correlation exists betweenfirm size(FS)and product innovation performance (PIP).This preliminary analysis suggests that largefirms have an innovation advantage over smallerfirms in terms of output,sup-porting the Schumpeterian hypothesis.4.Analyses4.1.ResultsThe models in this study are estimated by OLS-based hier-archical regression.Model1contains several control variables, including industry dummies(IND1–IND7),firm size(FS),labor quality(LQ),inward technology licensing(ITL),and subsidiary (multi-nationality)dummy(SB).Then,absorptive capacity(ACAP) and the collaboration variables(CL S,CL C,CL P,and CL R)are entered in Model2.The terms of the interaction between the collabora-tion variables and the ACAP variable are added in Model3.Because the interaction terms are usually highly correlated with ACAP or the collaboration variables,this study follows the procedure sug-gested by Friedrich(1982)to reduce or eliminate any bias resulting from multi-collinearity.This approachfirst standardizes the vari-ables except for the dummies and then forms the cross-product terms.Table2presents the moderated regression analysis results for the models.Table2indicates that adding the collaboration and ACAP vari-ables(Model2)to the model with only controls(Model1)increases the R2by about38.2%.The F-value(106.48)for the incremental R2 values achieves a statistical significance at the1%level.An inspec-tion of the coefficient estimates of the collaboration variables shows that these variables do not explain the change in product inno-vation performance.This result implies that collaboration with different types of partners does not increase product innovation performance when the analyses does not account for the effect of absorptive capacity.1Adding the interaction terms(Model3)to Model2further increases the R2by about1.7%.The F-value(4.63) 1This result confirms to that documented in Brouwer and Kleinknecht(1996), Love and Rpoer(2001),and Freel(2003).。
多维宏组学方法-概念解析以及定义

多维宏组学方法-概述说明以及解释1.引言【1.1 概述】多维宏组学方法是一种基于多种生物信息学技术和统计学分析方法,综合多个层面的生物学数据,以更全面深入地理解生物系统的复杂性和多样性。
随着高通量测序技术和多组学研究的快速发展,我们已经积累了大量各类生物学数据,包括基因组学、转录组学、蛋白质组学和代谢组学等多维数据。
传统的单一组学分析方法往往只能提供局部的生物信息,而多维宏组学方法则能够结合多种组学数据,通过综合分析不同层面的信息,揭示生物体内复杂的生物学网络和组织结构。
通过对多维宏组学方法的研究和应用,我们可以更全面地了解生物体内基因的表达规律、蛋白质相互作用网络、代谢通路的变化等重要生物学过程。
多维宏组学方法的关键在于整合和分析不同维度的生物学数据,从而揭示它们之间的相互关系和潜在规律。
在研究中,我们首先需要收集多个组学层面的数据,例如基因表达数据、蛋白质丰度数据和代谢产物浓度数据等。
然后,我们利用合适的统计学方法和机器学习算法,对这些数据进行整合和分析,以发现它们之间的相关性和关联性。
多维宏组学方法已经广泛应用于生物医学研究领域,为疾病的诊断、治疗和预防提供了新的思路和方法。
通过对不同组学层面的数据进行综合分析,我们可以更准确地识别出与疾病相关的生物标记物,为个体化医疗和精准药物治疗奠定基础。
此外,多维宏组学方法还可以用于研究复杂疾病的发生机制和进展过程,为疾病的早期预警和干预提供理论支持。
总而言之,多维宏组学方法作为一种综合多种组学数据的分析策略,已经在生物医学研究中展示出巨大的潜力。
通过揭示多个层面生物学数据之间的关系和规律,我们可以更好地理解生物系统的复杂性,为疾病研究和个体化医疗开辟新的研究方向。
随着生物学数据的不断积累和研究方法的不断改进,相信多维宏组学方法将逐渐成为生物医学研究的重要工具和方法。
1.2 文章结构文章结构部分的内容:本文将按照以下结构进行描述和分析多维宏组学方法。
系统论、现象学与交叉学科研究——与剑桥大学史蒂文·沃特森的对话
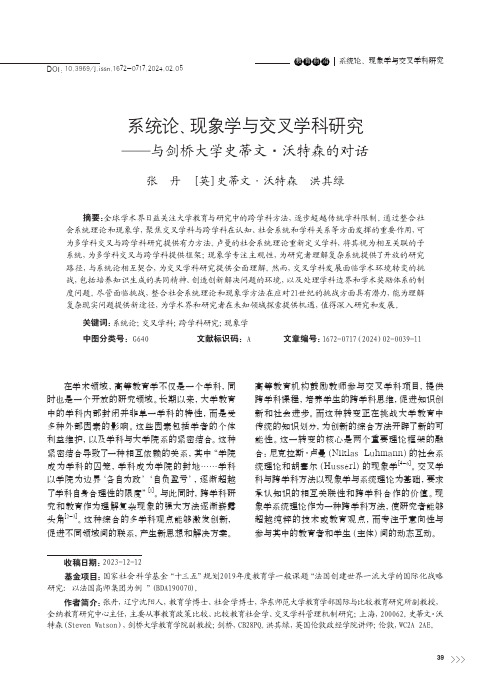
——与剑桥大学史蒂文·沃特森的对话张 丹 [英]史蒂文·沃特森 洪其绿在学术领域,高等教育学不仅是一个学科,同时也是一个开放的研究领域。
长期以来,大学教育中的学科内部封闭并非单一学科的特性,而是受多种外部因素的影响。
这些因素包括学者的个体利益维护,以及学科与大学院系的紧密结合。
这种紧密结合导致了一种相互依赖的关系,其中“学院成为学科的囚笼,学科成为学院的封地……学科以学院为边界‘各自为政’‘自负盈亏’,逐渐超越了学科自身合理性的限度”[1]。
与此同时,跨学科研究和教育作为理解复杂现象的强大方法逐渐崭露头角[2-3]。
这种综合的多学科观点能够激发创新,促进不同领域间的联系,产生新思想和解决方案。
高等教育机构鼓励教师参与交叉学科项目,提供跨学科课程,培养学生的跨学科思维,促进知识创新和社会进步。
而这种转变正在挑战大学教育中传统的知识划分,为创新的综合方法开辟了新的可能性。
这一转变的核心是两个重要理论框架的融合:尼克拉斯·卢曼(Niklas Luhmann)的社会系统理论和胡塞尔(Husserl)的现象学[4-6]。
交叉学科与跨学科方法以现象学与系统理论为基础,要求承认知识的相互关联性和跨学科合作的价值。
现象学系统理论作为一种跨学科方法,使研究者能够超越纯粹的技术或教育观点,而专注于意向性与参与其中的教育者和学生(主体)间的动态互动。
摘要:全球学术界日益关注大学教育与研究中的跨学科方法,逐步超越传统学科限制。
通过整合社会系统理论和现象学,聚焦交叉学科与跨学科在认知、社会系统和学科关系等方面发挥的重要作用,可为多学科交叉与跨学科研究提供有力方法。
卢曼的社会系统理论重新定义学科,将其视为相互关联的子系统,为多学科交叉与跨学科提供框架;现象学专注主观性,为研究者理解复杂系统提供了开放的研究路径,与系统论相互契合,为交叉学科研究提供全面理解。
然而,交叉学科发展面临学术环境转变的挑战,包括培养知识生成的共同精神、创造创新解决问题的环境,以及处理学科边界和学术奖励体系的制度问题。
免疫调节英文 PPT课件

Immunoregulation,Immunodysregulation and Autoimmune Disorders
2019/5/14
Contents
Part I Concept of Immunohomeostasis Part II Immunological Tolerance Part III Immunoregulation and Immunodysregulation Part IV Mechanisms of Autoimmune Diseases
with unique Ag specificity • Has to be able to distinguish
self from non-self
A properly coordinated (regulated) immune system will:
1 Defend against infectious microorganisms 2 Stay in harmony with self-antigens and tissues of the host 3 Maintain appropriate magnitude when responding to
2019/5/14
T cell-deficient mouse
Autoimmune diseases thyroid stomach salivary gland Langerhans islets adrenal glands ovaries testes
2019/5/14
CD4
Regulators
2019/5/14
T 细胞外周免疫耐受
非专职APC诱导初始 T 细胞进入免疫失能状态
风险偏好实验研究综述

1经典理论:预期效用模型风险偏好问题的相关论述,最早可以追溯到著名的预期效用模型(Expected Utility M odel )。
该模型是由V on Neumann 和M orgenstern 在对圣彼得堡悖论解答的基础上,进行相关的公理化阐述而形成。
预期效用模型建立了VN-M 预期效用函数,该函数的内涵是:在不确定性条件下,经济人的效用水平是通过对预期效用函数可能出现的损益结果期望加权得到的[1]。
预期效用理论的优越性在于把效用函数引入到偏好模型中,证明了效用函数在最终损益空间上的存在性和期望值的大小与排序的一致性。
此后,Savage 在预期效用理论的基础上提出了主观预期效用理论,用主观概率代替预期效用函数中的客观概率,该模型更能体现出决策者的个体差异。
预期效用模型是现代决策理论的基础,在风险偏好领域影响巨大,几乎扩展到经济理论的每个分支。
然而,由于该模型的假设过于严格且脱离实际,在此后实验经济学的兴起中,预期效用模型及其内含的个体偏好思想受到越来越多的挑战,具体可分为对公理文章编号:1008-7133(2009)05-0034-04风险偏好实验研究综述李延喜,付洁,李鹏峰,冯宝军(大连理工大学管理学院,辽宁大连116024)摘要:随着实验经济学的兴起,实验方法在风险偏好领域成果丰硕,并给传统理论带来强烈冲击,因此梳理风险偏好实验研究对把握该领域的现状和发展方向有重要意义。
风险偏好实验方法从经典理论的公理性假设与偏好存在假设两个角度出发,分别对经典理论提出了质疑、理论替代与理论修正,实验方法在该领域有着广阔的研究空间。
关键词:风险偏好;实验经济学;公理性假设;偏好存在假设中图分类号:F069.9文献标志码:ALiterature survey on risk preference experimentLI Yan -xi ,FU Jie ,LI Peng -feng ,F ENG Bao -jun(School of Management ,Dalian University of Technology ,Dalian 116024,China )Abstract :Experimental economics ,which yielded substantial achievement ,has brought severe challenge to the traditional theory in the field of risk preference study ,so it ’s necessary to arrange the experimental results to grasp the orientation.The experimental method ,sorting from the axiomatic hypothesis and preference -existed hypothesis ,conducts a literature survey on the experimental application on risk preference ,and it has an expansive research space in this field.Key words :risk preference;experimental economics;axiomatic hypothesis;preference -existed hypothesis收稿日期:2009-05-31基金项目:国家自然科学基金资助项目(70772087);教育部博士点基金资助项目(20040141031);大连理工大学人文社科基金(DUTHS2007302)作者简介:李延喜(1970—),男,教授,博士生导师;付洁(1985—),女,硕士研究生;李鹏峰(1983—),男,硕士研究生;冯宝军(1968—),男,高级审计师.第11卷第5期科技与管理Vol.11No.52009年9月Science-Technology and ManagementSep.,2009性假设和对偏好存在假设的质疑。
--医学PPT课件大全非对称基因组学用新的思维解读生命进化之谜

同一物种的同源染色体间的比较
Chromosome 1
AL gene P/A gene
Nip. 9311
Chromosome x
Nip. 9311
非对称基因间的遗传差异
Proportion of Asymmetric Located Genes
Correlation between Asymmetry and Diversity
Gene C
- More similar in A & C or B & C
- Stable gene copies
Results
A&B A &C C&B
Average diversity:
4.31%
Share polymorphic sites: 6
Fixed mutations:
12
Recombination events: 0
基因1基因2基因3基因4基因5基因6孟德尔定律等位基因的分离与重组摩尔根的连锁遗传系列等位基因的重组非非非非非非同一物种基因组中不对称的基因数量种类位置等nip9311nip9311同一物种的同源染色体间的比较chromosome1chromosomexalgenepagene非对称的基因数量236个499启示哪种基因家族更对称变异大的或小的哪种基因家族对生物的多样性贡献更大品种1日本晴的抗病基因品种29311的抗病基因483个完全不同的105个222不等位且差异大的131个277464个多一些和少一些基因对人类疾病有什么影响例1水稻基因组45000个基因基因组的非对称性约510即1960pa4170al个基因完全不同例2玉米基因组基因组比水稻的更大且比水稻的更不对称自交系间4000个杂种间8000个例3arabidopsis拟南芥30000个基因在表达的基因中
metaboanalyst的多组学选择deg思路

metaboanalyst的多组学选择deg思路下载温馨提示:该文档是我店铺精心编制而成,希望大家下载以后,能够帮助大家解决实际的问题。
文档下载后可定制随意修改,请根据实际需要进行相应的调整和使用,谢谢!并且,本店铺为大家提供各种各样类型的实用资料,如教育随笔、日记赏析、句子摘抄、古诗大全、经典美文、话题作文、工作总结、词语解析、文案摘录、其他资料等等,如想了解不同资料格式和写法,敬请关注!Download tips: This document is carefully compiled by the editor. I hope that after you download them, they can help you solve practical problems. The document can be customized and modified after downloading, please adjust and use it according to actual needs, thank you!In addition, our shop provides you with various types of practical materials, suchas educational essays, diary appreciation, sentence excerpts, ancient poems, classic articles, topic composition, work summary, word parsing, copy excerpts, other materials and so on, want to know different data formats and writing methods, please pay attention!代谢组学是一种研究生物体内代谢物的组成和变化的技术,可以帮助揭示生物系统的生理和病理状态。
TCGA多组学联合分析数据库

TCGA多组学联合分析数据库之前我们在介绍GEPIA的时候,说这个数据库只能用于TCGA表达数据的一些分析。
但是对于TCGA数据而言,里面包括相同样本的表达、突变、拷贝数、甲基化以及临床信息等数据,所以我们其实可以利用TCGA数据库来进行多组学之间的交叉分析。
今天我们就介绍一个TCGA多组学分析的经典数据库: cbioPortal(/)数据库主界面介绍1.数据集选择在这个数据库的主界面上,我们可以看到包括的相关数据集。
数据库已经把按照组织类型分好了,例如我们选择食管/胃。
从图中可以看到,这个数据库包括的数据不限于TCGA的数据。
同时还包括一些其他发表的数据,例如在胃癌里面,就有一个日本的发表在Nat Genet上的数据集,,我们可以在数据集的后面看到具体的样本量。
我们可以点击数据集当中的饼图()就可以看到具体的数据集信息。
例如这个日本的数据集,我们就可以看到,这个数据集是一个全外显子测序的数据,主要是用来检测胃癌和正常配对组织突变的情况。
下面的一些图是基于不同的临床信息,突变频率的变化。
由于TCGA的数据比较全,所以我们就选择其中一个组织分析的TCGA的结果。
2. 样本选择选择完之后,我们点击就可以进行下一步了。
接下来,我们就可以就要选择分析的样本了和输入想要分析的基因了。
其中第一个看到的让我们选择基因组图谱,这个默认的是突变和拷贝数。
这个选项只是在后面结果绘制OncoPrint图的时候有影响,其他的对于别的分析影响不大的,所以至于下面的mRNA表达以及protein表达可以不选的。
进一步的我们要选择分析的样本了,虽然TCGA的数据做了不同组学的数据。
但是也不是说作用组学做的是完全相同的样本,中间总有一两个样本做了一个组学的检测的。
但是这个结果的选择对于后续的分析影响也不大,所以我们就选择所有样本。
最后就是输入基因,基因输入的话,我们可以输入多个基因同时观察这些基因的在数据集当中的变化,同时也可以输入一个基因。
- 1、下载文档前请自行甄别文档内容的完整性,平台不提供额外的编辑、内容补充、找答案等附加服务。
- 2、"仅部分预览"的文档,不可在线预览部分如存在完整性等问题,可反馈申请退款(可完整预览的文档不适用该条件!)。
- 3、如文档侵犯您的权益,请联系客服反馈,我们会尽快为您处理(人工客服工作时间:9:00-18:30)。
Dual Systems of Sequents and Tableauxfor Many-Valued LogicsMatthias Baaz Christian G.Ferm¨u ller Richard ZachNovember25,1992Technical Report TUW–E185.2–BFZ.2–921993Workshop on Theorem Proving withAnalytic TableauxTechnische Universit¨a t WienInstitut f¨u r Computersprachen E185.2Abteilung f¨u r Anwendungen der formalen LogikResselgasse3/1,A–1040Wien,Austria/EuropePhone:(+431)58801x4088Fax:(+431)5041589Dual Systems of Sequents and Tableauxfor Many-Valued LogicsMatthias BaazChristian G.Ferm¨u llerRichard ZachThe aim of this paper is to emphasize the fact that for allfinitely-many-valued logics there is a completely systematic relation between sequent calculi and tableau systems.More importantly,we show that for both of these systems there are al-ways two dual proof sytems(not just only two ways to interpret the calculi).This phenomenon may easily escape one’s attention since in the classical(two-valued) case the two systems coincide.(In two-valued logic the assignment of a truth value and the exclusion of the opposite truth value describe the same situation.) We employ the usual definitons offirst order languages,many-valued interpre-tations(M)and induced valuation functions(val M)(see e.g.Carnielli[1987]). In the following V={v1,...,v m}always denotes the set of truth values of a logic.To stress the dualty of the two types of calculi we shall define them simul-tanously:1.Definition An(m-valued)sequent is an m-tuple offinite setsΓi of formulas, denoted asΓ1|Γ2|...|Γm.(As usual we abbreviateΓ∪∆byΓ,∆andΓ∪{A} byΓ,A.)2.Definition An interpretation M is said to p(n)-satisfy a sequentΓ1|...|Γm, if there is an i(1≤i≤m)and a formula F∈Γi,s.t.val M(F)=(=)v i.A sequent is called p(n)-valid,if it is p(n)-satisfied by every interpretation.The concept of p-satisfiability was used by Rousseau[1967](compare also Schr¨o ter[1955])in his formulation of many-valued sequents,whereas n-satisfiablity essentially already appears in Carnielli[1991].3.Definition An introduction rule for a connective2at place i in the logic L isa schema of the form:Γj1,∆j1|...|Γj m,∆j mj∈I2:iΓ1|...|Γi,2(A1,...,A n)|...|Γmwhere the arity of2is n,I is afinite set,Γl= j∈IΓj l,∆j l⊆{A1,...,A n} It is called p(n)-admissible,if for every interpretation M the following are equiv-alent:(1)2(A1,...,A n)takes(does not take)the truth value v i.(2)For all j∈I,M p(n)-satisfies the sequents∆j1|...|∆j m.4.Example We state rules for the implication of the three-valued G¨o del logic G 3with V ={f,u,t }.Let the expression A v (A =v )denote the statement “A takes (does not take)the truth value v ”.Since (A ⊃B )t iff(A f ∨A u ∨B t )∧(A f ∨B u ∨B t )we get the following p-admissible introduction rule for position t :Γ,A |∆,A |Π,B Γ ,A |∆ ,B |Π ,B Γ,Γ|∆,∆|Π,Π,A ⊃B⊃:t Because of (A ⊃B )t iffA f ∨(A u ∧B u )∨B t we get by negating both sides of the equivalence the following n-admissible introduction rule for the implication at position t :Γ,A |∆|ΠΓ |∆ ,A,B |Π Γ |∆ |Π ,BΓ,Γ ,Γ |∆,∆ ,∆ |Π,Π ,Π A ⊃B ⊃:tIt should be stressed that admissible introduction rules for a connective at a given place are far from being unique:Every p(n)-admissible introduction rule for 2(A 1,...,A n )at place i corresponds to a conjunction of disjunctions of some A v l (A =v l )which is true iff2(A 1,...,A n )takes (does not take)the truth value v i .Any such conjunctive normal form for 2(A 1,...,A n )v i will do.In particular,the truthtable 2for a connective 2immediately yields a complete conjunctive normal form.For p-sequents the corresponding rule is as in Definition 3,with:I ⊆V n is theset of all n -tuples j =(w 1,...,w n )of truth values such that 2(w 1,...,w n )=v i ;and ∆j l ={A k |1≤k ≤n,v l =w k }.For n-sequents we get:I ⊆V n consistsof all n -tuples j =(w 1,...,w n )of truth values such that 2(w 1,...,w n )=v i ;and ∆j l ={A k |1≤k ≤n,v l =w k }.5.Definition An introduction rule for a quantifier Q at place i in the logic L is a schema of the form:Γj 1,∆j 1|...|Γj m ,∆j m j ∈IΓ1|...|Γi ,(Q x )A (x )|...|ΓmQ :i where I is a finite set,Γl = j ∈I Γj l ,∆jl ⊆{A (a 1),...,A (a p )}∪{A (t 1),...,A (t q )}.The a l are metavariables for free variables (the eigenvariables of the rule)satisfying the condition that they do not occur in the lower sequent;the t k are metavariables for arbitrary terms.Q :i is called p(n)-admissible ,if for every interpretation M the following are equivalent:(1)(Q x )A (x )takes (does not take)the truth value v i under M .(2)For all d 1,...,d p ∈D ,there are e 1,...,e q ∈D s.t.for all j ∈I ,M p(n)-satisfies ∆ j 1|...|∆ j m where ∆ j l is obtained from ∆jl by instantiating theeigenvariable a k (term variable t k )with d k (e k ).The truth function Qfor a (distribution)quantifier Q immediately yields admis-sible introduction rules for place i in a way similar to the method described above for connectives:For p-sequents let I ={j ⊆V | Q(j )=v i }.Then the rule is given as in Definition 5,with ∆jl ={A (a j w )|w ∈j,w =v l }∪{A (t j )|v l ∈V \j }.In contrast,for n-sequents we take I ={ j,i |j ⊆V ∧i ∈j ∧ Q(j )=v i }and ∆ j,i l ={A (a jl )|l ∈j }∪{A (t j )|i =l }.Again,it should be stressed that in general these are not the only possible rules.6.Definition A p-sequent calculus for a logic L is given by:(1)Axioms of the form:A|A|...|A,where A is any formula,(2)For every connective2and every truth value v i a p-admissible introductionrule2:i,(3)For every quantifier Q and every truth value v i a p-admissible introductionrule Q:i,(4)Weakening rules for every place i:Γ1|...|Γi|...|Γmw:iΓ1|...|Γi,A|...|Γm(5)Cut rules for every pair of truth values(v i,v j)s.t.v i=v j:Γ1|...|Γi,A|...|Γm∆1|...|∆j,A|...|∆mcut:ijΓ1,∆1|...|Γm,∆mA n-sequent calculus for a logic L is given by:(1)Axioms of the form:∆1|...|∆m,where∆i=∆j={A}for some i,j s.t.i=j and∆k=∅otherwise(A is any formula),(2)For every connective2and every truth value v i an n-admissible introductionrule2:i,(3)For every quantifier Q and every truth value v i an n-admissible introductionrule Q:i,(4)Weakening rules(identical to the ones tor p-sequent calculi)(5)The cut rule: Γi1|...|Γi i,A|...|Γi m mi=1cut:Γ1|...|ΓmwhereΓl= 1≤j≤mΓj l.7.Theorem(Soundness and cut-free Completeness)For every p(n)-sequent cal-culus the following holds:A sequent is p(n)-provable without cut rule(s)iffit is p(n)-valid.Analytic tableaux for many-valued logics have been investigated by Surma[1977]and Carnielli[1987].H¨a hnle[1991],based on the aforementioned work,studied the applicability of these systems for automated theorem proving. H¨a hnle introduced the notation of sets-of-signs which allows a more efficient repre-sentation of tableaux and presented streamlined calculi for certain classes of logics. Here,we want to stress the striking similarity between tableaux systems and sequent calculi:In fact,there is an immediate correspondence between cut-free sequent cal-culus proofs and closed tableaux.Again,there are two dual systems for any logic.8.Definition A signed formula is an expression of the form{w}A,where w∈V.9.Definition A tableau is a downward tree of sets of signed formulas where every set is obtained from a set preceding it in the tree by application of one of the rules of the tableau system:Let R:i be a p(n)-admissible introduction rule for a connective or a quantifier as given in Definitions3and5,where at least one of the∆j is nonempty.Moreover, let F be the formula being introduced(i.e.,F≡2(A1,...,A n)or F≡(Q x)A(x)).The p(n)-tableau rule corresponding to R:i is:Γ,{v i}FΓ, m k=1∆k j∈Iwhere∆k is obtained from∆k by replacing every formula A∈∆k by{v k}A.A p(n)-analytic tableau is called closed,if every leaf contains formulas{v k}A for all k∈{1,...,m}(for k∈{i,j},i=j).10.Theorem Every closed p(n)-tableau with the root Γk corresponds to a cut-free p(n)-sequent calculus proof ofΓ1|...|Γm.Wefinally remark that also resolution calculi can be derived from sequent cal-culi:The introduction rules for sequents convert into reduction rules that translate finite sets of assignments of truth values to formulas into clause forms.(Clauses arefinite sets of assignments of truth values to atomic formulas;cf.Baaz and Ferm¨u ller[1992])ReferencesBaaz,M.and C.G.Ferm¨u ller.[1992]Resolution for many-valued logics.In Proc.Logic Programming and Automated Reasoning LPAR’92,A.Voronkov,editor,LNAI624,pp.107–118,Springer,Berlin.Carnielli,W.A.[1987]Systematization offinite many-valued logics through the method of tableaux.J.Symbolic Logic,52(2),473–493.[1991]On sequents and tableaux for many-valued logics.J.Non-Classical Logic, 8(1),59–76.H¨a hnle,R.[1991]Uniform notation of tableaux rules for multiple-valued logics.In Proc.IEEE International Symposium on Multiple-valued Logic,pp.238–245.Rousseau,G.[1967]Sequents in many valued logic I.Fund.Math.,60,23–33.Schr¨o ter,K.[1955]Methoden zur Axiomatisierung beliebiger Aussagen-und Pr¨a dikatenkalk¨u le.Z.Math.Logik Grundlag.Math.,1,241–251.Surma,S.J.[1977]An algorithm for axiomatizing everyfinite logic.In Computer Science and Multiple-valued Logic:Theory and Applications,D.C.Rine,editor,pp.137–143.North-Holland,Amsterdam.。