美赛论文mcm模版
MCM美国大学生数学建模竞赛模板-公式

由假设得到公式1.We assume laminar flow and use Bernoulli’s equation:(由假设得到的公式)公式Where符号解释According to the assumptions, at every junction we have(由于假设)公式由原因得到公式2.Because our field is flat, we have公式, so the height of our source relative to our sprinklers does not affect the exit speed v2 (由原因得到的公式);公式Since the fluid is incompressible(由于液体是不可压缩的), we have公式Where公式用原来的公式推出公式3.Plugging v1 into the equation for v2 ,we obtain(将公式1代入公式2中得到)公式11.Putting these together(把公式放在一起), because of the law of conservation of energy, yields:[]公式12.Therefore, from (2),(3),(5), we have the ith junction(由前几个公式得)公式Putting (1)-(5) together, we can obtain pup at every junction. In fact, at the last junction, we have公式Putting these into (1) ,we get(把这些公式代入1中)公式Which means that theCommonly, h is aboutFrom these equations, (从这个公式中我们知道)we know that ………引出约束条件4.Using pressure and discharge data from Rain Bird 结果,We find the attenuation factor (得到衰减因子,常数,系数)to be公式计算结果6.To find the new pressure ,we use the ( 0 0),which states that the volume of water flowing in equals the volume of water flowing out : (为了找到新值,我们用什么方程)公式Where() is ;;7.Solving for VN we obtain (公式的解)公式Where n is the …..8.We have the following differential equations for speeds in the x- and y- directions:公式Whose solutions are (解)公式9.We use the following initial conditions ( 使用初值) to determine the drag constant:公式根据原有公式10.We apply the law of conservation of energy(根据能量守恒定律). The work done by the forces is公式The decrease in potential energy is (势能的减少)公式The increase in kinetic energy is (动能的增加)公式Drug acts directly against velocity, so the acceleration vector from drag can be found Newton’s law F=ma as : (牛顿第二定律)Where a is the acceleration vector and m is massUsing the Newton’s Second Law, we have that F/m=a and公式So that公式Setting the two expressions for t1/t2 equal and cross-multiplying gives公式22.We approximate the binomial distribution of contenders with a normal distribution:公式Where x is the cumulative distribution function of the standard normal distribution. Clearing denominators and solving the resulting quadratic in B gives公式As an analytic approximation to . for k=1, we get B=c26.Integrating, (使结合)we get PVT=constant, where公式The main composition of the air is nitrogen and oxygen, so i=5 and r=1.4, so23.According to First Law of Thermodynamics, we get公式Where ( ) . we also then have公式Where P is the pressure of the gas and V is the volume. We put them into the Ideal Gas Internal Formula:公式Where对公式变形13.Define A=nlw to be the ( )(定义); rearranging (1) produces (将公式变形得到)公式We maximize E for each layer, subject to the constraint (2). The calculations are easier if we minimize 1/E.(为了得到最大值,求他倒数的最小值)Neglecting constant factors (忽略常数), we minimize公式使服从约束条件14.Subject to the constraint (使服从约束条件)公式Where B is constant defined in (2). However, as long as we are obeying this constraint, we can write (根据约束条件我们得到)公式And thus f depends only on h , the function f is minimized at (求最小值)公式At this value of h, the constraint reduces to公式结果说明15.This implies(暗示)that the harmonic mean of l and w should be公式So , in the optimal situation. ………5.This value shows very little loss due to friction.(结果说明)The escape speed with friction is公式16.We use a similar process to find the position of the droplet, resulting in公式With t=0.0001 s, error from the approximation is virtually zero.17.We calculated its trajectory(轨道) using公式18.For that case, using the same expansion for e as above,公式19.Solving for t and equating it to the earlier expression for t, we get公式20.Recalling that in this equality only n is a function of f, we substitute for n and solve for f. the result is公式As v=…, this equation becomes singular (单数的).由语句得到公式21.The revenue generated by the flight is公式24.Then we have公式We differentiate the ideal-gas state equation公式Getting公式25.We eliminate dT from the last two equations to get (排除因素得到)公式22.We fist examine the path that the motorcycle follows. Taking the air resistance into account, we get two differential equations公式Where P is the relative pressure, we must first find the speed v1 of water at our source: (找初值)公式自己根据计算所画的图:1、为了…….(目的),我们作了…….图。
国际数学建模竞赛优秀论文英文模板
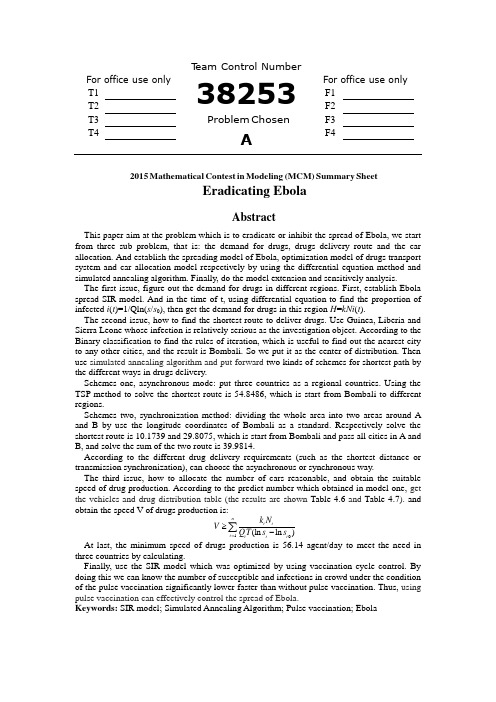
T eam Control NumberFor office use only38253For office use onlyT1F1 T2 F2 T3 Problem ChosenF3 T4 AF42015 Mathematical Contest in Modeling (MCM) Summary SheetEradicating EbolaAbstractThis paper aim at the problem which is to eradicate or inhibit the spread of Ebola, we start from three sub problem, that is: the demand for drugs, drugs delivery route and the car allocation. And establish the spreading model of Ebola, optimization model of drugs transport system and car allocation model respectively by using the differential equation method and simulated annealing algorithm. Finally, do the model extension and sensitively analysis.The first issue, figure out the demand for drugs in different regions. First, establish Ebola spread SIR model. And in the time of t, using differential equation to find the proportion of infected i (t )=1/Qln(s /s 0), then get the demand for drugs in this region H =kNi (t ).The second issue, how to find the shortest route to deliver drugs. Use Guinea, Liberia and Sierra Leone whose infection is relatively serious as the investigation object. According to the Binary classification to find the rules of iteration, which is useful to find out the nearest city to any other cities, and the result is Bombali. So we put it as the center of distribution. Then use simulated annealing algorithm and put forward two kinds of schemes for shortest path by the different ways in drugs delivery.Schemes one, asynchronous mode: put three countries as a regional countries. Using the TSP method to solve the shortest route is 54.8486, which is start from Bombali to different regions.Schemes two, synchronization method: dividing the whole area into two areas around A and B by use the longitude coordinates of Bombali as a standard. Respectively solve the shortest route is 10.1739 and 29.8075, which is start from Bombali and pass all cities in A and B, and solve the sum of the two route is 39.9814.According to the different drug delivery requirements (such as the shortest distance or transmission synchronization), can choose the asynchronous or synchronous way.The third issue, how to allocate the number of cars reasonable, and obtain the suitable speed of drug production. According to the predict number which obtained in model one, get the vehicles and drug distribution table (the results are shown Table 4.6 and Table 4.7). and obtain the speed V of drugs production is:10(ln ln )ni ii i i i k N V Q T s s =≥-∑At last, the minimum speed of drugs production is 56.14 agent/day to meet the need in three countries by calculating.Finally, use the SIR model which was optimized by using vaccination cycle control. By doing this we can know the number of susceptible and infections in crowd under the condition of the pulse vaccination significantly lower faster than without pulse vaccination. Thus, using pulse vaccination can effectively control the spread of Ebola.Keywords: SIR model; Simulated Annealing Algorithm; Pulse vaccination; EbolaEradicating EbolaContent1 Restatement of the Problem (1)1.1 Introduction (1)1.2 The Problem (1)2 General Assumptions (1)3 Variables and Abbreviations (2)4 Modeling and Solving (2)4.1 Model I (2)4.1.1 Analysis of the Problem (2)4.1.2 Model Design (2)4.2 Model II (6)4.2.1 Analysis of the Problem (6)4.2.2 Model Design (6)4.3 Model Ⅲ (8)4.3.1 Analysis of the Problem (8)4.3.2 Model Design (9)4.4 Extent our models (11)5 Sensitivity Analysis (14)5.1 Effect of Daily Contact Rate (14)5.2 Effect of inoculation rate (14)6 Model Analysis (15)6.1 The Advantages of Model (15)6.2 The Disadvantages of Model (15)7 Non-technical Explanation (16)References (18)1Restatement of the Problem1.1IntroductionEbola virus is a very rare kind of virus. It can cause humans and primates produce Ebola hemorrhagic fever virus, and has a high mortality rate. The largest and most complex Ebola outbreak appeared in the West African country in 2014. This outbreak occurred in guinea first, then through various ways to countries such as Sierra Leone, Liberia, Nigeria and Senegal. The number of cases and deaths, which occurred in this outbreak, is more than the sum of all the other epidemic. And outbreak continued to spread between countries. On August 8, 2014, the general-director of the world health organization announced the outbreak of public health emergency of international concern.In this paper, a realistic and reasonable mathematic model, which considers several aspects such as vaccine manufacturing and drug delivery, has been built.Then optimizing the model to eliminate or suppress the harm done by the Ebola virus.1.2The ProblemEstablishing a model to solve the spread of the disease, amount of drugs needed, possible feasible transportation system, transporting position, the speed of a vaccine or drug manufacturing and any other key factor. Thus, we decompose the problem into three sub-problem, modeling and finding the optimization method to face the Ebola virus.♦Building a model, which can solve the spread of the disease and the demand for drugs.♦Building a model to find the best solution.♦Using the goal programming to solve the problems of production and distribution and optimization of other factors..2General AssumptionsTo simplify the problem, we make the following basic assumptions, each of which is properly justified.♦Our assumptions is reasonable and effective.♦Vehicles only run in the path which we have simulated♦This assumption greatly simplify our model and allow us to focus on the shortest path.♦We consider the model that are enclosed.♦People who recovered, will not infected again, and exit the transmission system3Variables and AbbreviationsThe variables and abbreviations used in this paper are listed in Table 3.1.Table 3.1 Assuming variableSymbol DefinitionS the number of susceptible peopleI the number of infected personsR the number of recoveredT a vaccine or drug production cycleH the amount of drugs needed by RegionA a cycle of a vaccine or drug productionL drug reserve area to the shortest path to all affected areasV speed of vaccine or pharmaceutical productionV’vehicle speedλrate of patient contact per dayμday cure rate per dayαn rights of those infected regions weight4Modeling and Solving4.1Model I4.1.1Analysis of the ProblemAccording to the literature that different types of virus has its own different propagation process characteristics, we do not analyze the spread of viruses from a medical point of view, but from the general to analyze the propagation mechanism. So we have to analyze the spread of the Ebola virus and the requirements of drugs through the SIR[1] model.4.1.2Model DesignIn the dynamics of infectious diseases, the main follow Kermack and McKendrick SIR epidemic model which the dynamics of the established method in 1927. SIR model until now is still widely used and continue to develop. SIR model of the total population is divided into the following three categories: susceptibles, the ratio of the number denoted by s(t), at time t is not likely to be infected, but the number of infectious diseases such proportion of the total; infectives, the ratio of the number denoted by i(t), at time t become a patient has been infected and has the proportion of the total number of contagious; recovered, the ratio of the number denoted by r(t), expressed the number of those infected at time t removed from the total proportion (ie, it has quit infected systems). Assuming a total population of N(t), then there are N(t) = s(t) + i(t) + r(t).SIR model is established based on the following two assumptions:In the investigated region-wide spread of the disease is not considered during the births, deaths, population mobility and other dynamic factors. Total population N(t) remainunchanged, the population remains a constant N.The patients’ contact rate (the average number of effective contacts per patient per day) is constant λ, the cure rate (patients be cured proportion of the total number of patients a day) is a constant μ, clearly the average infectious period of 1/μ, infectious period contact number for Q = λ/μ.In the model based on the assumption that we develop a susceptible person to recover fromthe sick person in the process, such as Figure 4.1:Figure 4.1 SIR the model flowchartSIR basis differential equation model can be expressed as:disi i dt dssi dt dri dt λμλμ⎧=-⎪⎪⎪=-⎨⎪⎪=⎪⎩(5.1)But it can see that s(t), i(t) is more difficult to solve, so we use the numerical calculations to esti mate general variation. Assuming λ = 1, μ = 0.3, i(0) = 0.02, s(0) = 0.98 (at the initial time), then we borrow MATLAB software programming to get results. And according to Table 4.1 analyzed i(t), s(t) of the general variation.Figure4.2 s(t),i(t)The patient scale map Figure 4.3 i ~s Phase track diagramFrom Table 4.1 and Figure4.2, we can see that i(t) increased from the initial value to about t = 7(maximum), and then began to decrease.Based on the calculating the numerical and graphical observation, use of phase trajectories discussed i(t), s(t) in nature. Here i ~ s plane is phase plane , the domain (s, i)∈D in phase plane for:{}(,)0,0,1D s i s i s i =≥≥+≤(5.2)According to equation (5.1) and con tact number of the infectious period Q = λ / μ, we can eliminate dt, get:0011(1)(1)i s i s s sdi ds di ds Q Q =-⋅⇒=-⋅⎰⎰(5.3)Calculated using integral characteristics:0001()()ln si t s i s Q s =+-=(5.4)Curve in the domain of definition, equation(5.3) is a phase trajectory.According to equation(5.1) and equation(5.3), have to analyze the changes. If and only if the patient i(t) for some period of growth, it think that in the spread of infectious diseases , then 1/Q is a threshold. If s 0> 1/Q, infectious diseases will spread , and reduce infectious period the number of contacts with Q, namely raising the threshold 1/Q and will make s 0≤1/Q, then it will not spread diseases.And we note that Q = λ/μ in the formula, the higher the level of people's health, the smaller patients’ contact rate; the higher the level of medical, the cure rate is larger and the smaller Q. Therefore, to improve the level of hygiene and medical help to control the spread of infectious diseases. Of course, can also herd immunity and prevention, to reduce s 0.In the process, we analyzed the spread of the disease, then we are going to discuss the amount of medication needed.According to equation(5.4), you can get i(t) values, we can calculate the number of people infected with the disease who I was:()()I i t N t =⋅(5.5)And the amount of drug required, we can be expressed as: H kI =(k is a constant, w> 0)If k> 0, it indicates that the number of infections is still rising, measures to control the virus also needs to be strengthened, and the amount of drugs is a growing demand mode until fluctuation; if k≤0, it means reducing the number of people infected, the virus the measure is better, and the dose of demand is also gradually reduced.According to the data provided by the WHO, we can get the number of infections various,which areas before January 30, 2015. see Table 4.2:Table 4.2 As the number of infections January 30, 2015Region Number Proportion Region Number ProportionNzerekore 2 0.0045 Koinadugu 1 0.0022Macenta 1 0.0022 Kambia 25 0.0558Kissdougou 1 0.0022 Western Urban 105 0.2344Kankan 1 0.0022 Western Rural 64 0.1429Faranah 4 0.0089 Mali 1 0.0022Kono 28 0.0625 Boffa 4 0.0089Bo 6 0.0134 Dubreka 11 0.0246Kenema 2 0.0045 Kindia 2 0.0045Moyamba 8 0.0179 Coyah 11 0.0246Port Loko 78 0.1741 Forecariah 24 0.0536Tonkolili 18 0.0402 Conakry 20 0.0446Bombal 18 0.0402 Montserrado 13 0.029Based on the latest data Ebola virus infections in January 2015, and the regional population and the associated parameter value Ebola assumptions, the model has been solved to a time t proportion of those infected i(t) = 1/Q ln (s/s0), using MATLAB software, we have predict the number of infections each region in February, then get a weight value of those infected forecast for each region in February 2015, as can be show Table 4.3.Table 4.3 As the number of infections February 28, 2015Region Number Proportion Region Number ProportionNzerekore 1 0.00233 Koinadugu 8 0.01864Macenta 3 0.00700 Kambia 24 0.05594Kissdougou 2 0.00470 Western Urban 69 0.16083Kankan 1 0.00233 Western Rural 78 0.18182Faranah 2 0.00470 Mali 4 0.00932Kono 22 0.05130 Boffa 2 0.00470Bo 5 0.01166 Dubreka 10 0.02331 Kenema 5 0.01166 Kindia 1 0.00233Moyamba 1 0.00233 Coyah 9 0.020979Port Loko 100 0.23310 Forecariah 20 0.046620Tonkolili 12 0.02797 Conakry 18 0.041968Bombal 23 0.05361 Montserrado 9 0.020979From Table 4.2 can be known, According to the number of cases of expression,we made a rough prediction that Ebola outbreak in February. it’s provide a reference for the production of vaccines and drugs. Indeed, it have provide a theoretical basis for the relevant departments which take appropriate precautions.4.2Model II4.2.1Analysis of the ProblemBased on the model I, we obtained the equation expression of disease transmission speed and number of drugs. However, in addition to these two factors, we should also consider how to transport drugs to the demanded area quickly and effectively. Thus, it is very important to develop a good transportation system, which can greatly improve the efficiency of drug transport and reduce the cost.4.2.2Model DesignBy searching on Wikipedia, we obtain cities which have erupted Ebola, and the latitude and longitude coordinates[2]. The results are shown in Table 4.4We get the best point, which is Bombali by programming. So, we assume it as the city which produces drugs.Because these cities are breaking points, both as a place of delivery. In order to find out the optimal path, we make following assumptions:♦The demand for each city is same♦The quantity of vehicles can meet the demand of transport♦Vehicles only run in the path which we have simulated4.2.2.1SA modelSA[3] is a random algorithm which is established by imitating metal annealing principle. It can be implemented in large rough search and local fine search by controlling the changes of temperature.Basic principle of SA:♦First, generated initial solution x0 randomly, and make it as the current best solution xopt. Then calculate the value of objective function f (xopt).♦Second, make a random fluctuation on the current solution. Then calculate the value of the new objective function f (x).♦Calculating and judgingΔf = f(x) - f(xopt).IfΔf >0, accept it as the current best solution;Otherwise, accept it in the form of probability P.The calculation method of P is:10=exp[(()())]0opt i f P f x f x f ≤⎧⎨-->⎩ (5.6)In this chapter, the SA algorithm is extended by selecting Bombali as a starting point to solve the optimal path. In the extended SA algorithm.we exploits the exponential cooling strategies and controls the change of temperature, namely10k i T Apha T -=⨯(5.7)Where T i is current controlled temperature, T 0 is the initial temperature, Apha is temperature reduction coefficient, k is the iterations.Solving the initial temperature 0T by means of random iterative and setting Apha = 0.9, the results are shown in Figure 4.4Longitude coordinates of citiesP a r a l l e l v a l u e o f c i t i e sthe total distance:54.8486Figure 4.4 Path graphThe value of the shortest total distance y is 54.8486 The shortest path is presented as follow:Bombali →Tonkolili →Nzerekore →Moyamba →Kambia →Port Loko →Coyah →Mali →Bo →Kindia →Western Urban →Kono →Dubreka →Faranah →Western Rural →Kenema →Kiss-dou gou →Kankan →Forecariah →Boffa →Macenta →Conakry →Montserrado →Koinadugu → Bombali4.2.2.2 SA model refinementSA model got all the shortest path problem of the city, but transport route is single and the efficiency is not high. So we use the longitude coordinates of Bombali as the basis to divide these cities into two parts. Urban classification is shown inTable 4.5, then simulate respectively.Table 4.5 The divided city distributionClassify CitiesLeft half Conakry, Moyamba, Port Loko, Kambia, Western Urban, Western Rural, Boffa, Dubreka, Kindia, Coyah, Forecariah, Bombali.Right halfMontserrado, Nzerekore, Macenta, Kissdougou, Kankan, Faranah, Kono, Bo, Kenema, Tonkolili, Koinadugu, Mali, Bombali .Bombali appears twice, because it is the starting point.After the algorithm simulation result is shown in Figure4.5 and Figure 4.6:Longitude coordinates of citiesP a r a l l e l v a l u e o f c i t i e sLongitude coordinates of citiesP a r a l l e l v a l u e o f c i t i e sthe total distance:28.2716Figure4.5 Left half Figure 4.6 Right halfThe path of left half :Bombali →Port Loko →Boffa →Forecariah →Dubreka →Moyamba →Kindia →Coyah →West e-rnRural →Conakry →Kambia →Western Urban →Bombali The path of right half :Bombali →Kenema →Faranah →Mali →Nzerekore →Bo →Kissdougou →Kankan →Koinadu gu →Kono →Tonkolili →Montserrado →Macenta →Bombali The total distance is:L=10.1739+29.8075=39.9814.It is smaller than the answer before, the transport time is reduced and the efficiency of transportation is improved.4.3 Model Ⅲ4.3.1 Analysis of the ProblemAccording to the above analysis of the first model and the second model, we can learn something about the spreading of Ebola, then finding the shortest path to transport medicines or vaccines. On the basis of the spreading of Ebola, we can know the numbers of illness with Ebola, then, get the quantity demanded of illness. According to the city distribution of infected zone, we find the shortest path to transport medicines, as well as ensure the shortest transporting route.After comprehending the demand for vaccine in infected zones and its the shortest transporting route, the next problem we think about is how to transport the vaccines or drugs from storage zone to infected zone using the maximum efficiency. Besides, we also need to consider whether the production speed can keep up with the demand for drugs and delivery speed. That is to say, the quantity of medicine production must be greater than or equal to the demand for drugs. Only in this method can we give sufficient vaccines or drugs to infected zones by using the fastest speed to control the spread of Ebola. 4.3.2 Model DesignIn the second model, we consider the shortest path and find the shortest path to all infected zones, then get its occurrence of distance. Getting the basic solve of the first model and the second model, the drugs or vaccines transport system can allot cars for infected zones judging by the weight of the numbers of infections in different cities. hypothesis :♦ All allocation cars are the same vehicle size, moreover, have sufficient cars. That is to say, the quantity of vaccines or drugs in all cars is equal.♦ All delivery routes will not block up, and the cars will not break down. That is to say, all allocation cars can reach the infected area on time.♦ In order to avoid Ebola propagate to other place, this area should be isolated immediately once this area burst Ebola.♦ The car allocation in different regions can match up with the pharmaceutical demand in different regions. That is to say, they are positively related♦ By looking for date, we can get the number of infections in different regions :I1,I2,I3….In, then get the weight of the number of infections in different regions:11,2,3nn nnn I n Iα===∑(5.8)The pharmaceutical demand in different regions is:1,2,3n n H C n α==(5.9)C is the total quantity of car ,αn is the weight of the number of infections in different regions.According to the hypothesis, we can know that the pharmaceutical demand in each infected zone is directly related to the car allocation, so, we allot all cars in the light of weight. That is to say, the bigger weight can get more cars, the smaller weight will get less cars. Thus, we not only can save time, but also cost.According to the above analysis, we can know that the model also should meet the follow conditions:123'n A H H H H L T V ≥++++⎧⎪⎨≤⎪⎩(5.10)H n is the pharmaceutical demand in different regions, V 'is vehicle speed, T is theproduction cycle of vaccines or drugs. According to the model I solving scheme, we can get the proportion of infected is i(t)=1/Qln(s/s 0)in t time, At the same time the region's demand for drugs is H=kNi(t), Drug production speed need to meet :10(ln ln )ni ii i i i k N V QT s s =≥-∑(5.11)We seek the latest date information from WTO official website [4], and get the new casedistribution graphs of Guinea 、Sierra Leone 、Liberia .You can see on Figure 4.7Figure 4.7 Geographical distribution of new and total confirmed casesWe can get the number of infections about 24 cities in infected zones from the diagram [5], then figure out the weight of infection numbers in different regions and clear up these dates. You can see on the Table 4.1.According to the model I, it have forecast the number of infections in 2015 February, and calculate the number of infections in various regions of the weight, the allocation of all transport vehicles, and have meet the demand for drugs in February at epidemic area. so, according to the predicted values, We can get the drug distribution table show in Table 4.6 and vehicle allocation table show in Table 4.7.the future of the epidemic and how to reasonable distribution of drugs,.According to the above model analysis, after ensuring the demand for vaccines and medicines in different regions and the shortest transport route, and on the double bind of medicine production speed and medicine delivery speed. we have a discussion ,then get the car allocation in different regions to make sure the medicines or vaccines reach the infected zones by using the fastest speed. So, we can remit current epidemic situation of Ebola.4.4 Extent our modelsIn the model I, we have studied the classical SIR epidemic model, then we have an improved in the model I, the improved model is:()()()()()()()()dSN I S t dt dIS t I t I t dt dRI t R t dt λβλβλμμλ⎧=-+⎪⎪⎪=-+⎨⎪⎪=-⎪⎩(5.12)In the infectious disease model, We've added the μto the population birth rate and natural mortality, ‘β’is the coefficient of the spread of the disease, ‘N’ is the number of species number. In this model assumes that there is no population move out and the death due to illness, the number of population is constant.As mentioned above, the ‘I’ is the number of infected patients, if the ‘S’ ‘I’ ‘R’ have given the initialvalue, By solving the differential equations(5.12), can get the value of ‘I(t)’ at a certain moment. For this model, we expect the people infected can stable at a low level, this means that the spread of infectious diseases has been effectively controlled. Analyzed the infectious disease model, if we want to control effectively to ‘I’, should decrease the coefficient of the spread of the disease β, and improve disease recovery rate λ, In terms of emergency rescue, it’s should ensure that there are have adequate relief drug to patients in emergency treatment, and make the probability of recovery to increase, then , it can control effectively to the increase of ‘I’.At the beginning of the outbreak of infectious diseases, when it ’s have a pulse vaccination for the population cycle T, the spread of the corresponding SIR epidemic model [6] is shown in Figure 4.8, Propagation model expressed in equation (4.13).S λI λRλFigure 4.8 The flow chart of pulse SIR1()()()()()()()()()(1)()()()0,1,2()()()nn nn dSN I S t dt dI S t I t I t t tdt dR I t R t t t T dtS t p S t I t I t t t n R t R t pS t λβλβλμμλ+----⎧=-+⎪⎪⎪=-+≠⎪⎪⎨=-=+⎪⎪=-⎪⎪===⎪=+⎩(5.13)P is vaccination rate.Impulsive vaccination is different from traditional large-scale disposable vaccination, it can ensure to make an effective control by using the spread of lower vaccination rate. We can obtain something from the analysis of the first model that i(t) is the function which increase first and then decrease with the time. Thus, the population infected will tend to zero ultimately. If 0dIdt <, then the critical value of c S is:()(1)(1)T c TT p e pTS T p e λλλγλλβλ+--+=>-+ (5.14)Then the critical value of c p is :()(1)()(1)T c T T e p T e λλλλμβμβλμβ+--=--+- (5.15)We can know that, if the vaccination rate p>p c , system can obtain a stable disease-free periodic solution.When the infectious disease, which is described at model(5.12), burst out at one region, we should firstly know the demand for vaccine in different rescue cycle area before doing vaccinate to the infected populations. On account of epidemical diffusion law that indicated by SIR model(5.13), which possessing the pulse vaccination, we use the following form of demand forecasting that change over time.()k k D pS T -=(5.16)We can know something from the second model that we divide the whole infected zone into two regions. The two regions are assumed to be A and B. There is a stockpile around A and B. Known about the above information, we use the suggested model to do car allocation for A and B.Given the parameters in Ebola spread model(5.13) and its initial value, as shown in the Table 4.8 and Table 4.9. If the pulse vaccination cycle T=50, we use MATLAB programming to figure out the arithmetic solution of Ebola spread model (5.8) and model(5.9), as shown in the follow form:Table 4.8 Infectious disease model parametersParameter λ β μ p T Numerical0.000060.000020.0080.150Table 4.9 A and B area initial values i r Infected area A 830 370 0 Infected area B92278daysn u m b e r sthe SIR model with pulse vaccination in the demand point Adaysn u m b e r sthe SIR model without pulse vaccination in the demand point A(a) (b)daysn u m b e r sthe SIR model with pulse vaccination in the demand point Bdaysn u m b e r sthe SIR model without pulse vaccination in the demand point B(c) (d)Figure 4.9 Numerical solution of diffusion model SIR diseaseCompare Figure 4.9(a) with Figure 4.9(b), we can see that infected people and vulnerable people are going down faster under the circumstance of pulse vaccination. The same circumstance can be seen in the comparison of Figure 4.9(c) and Figure 4.9(d), it indicate that the pulse vaccination can control the spread of Ebola more effective. Because of this, we use the pulse vaccination to make our model solve the spread of Ebola preferably.5 Sensitivity Analysis5.1 Effect of Daily Contact RateIn model Ⅰ, we get the variation of function i (t ) and s (t ) by assuming variable value. So further discuss the value of λ is 2 or 3 whether impact on the result.Based on MATLAB software programming, can get the graphics when λ=2 or λ=3.daysn u m b e r sThe rate of healthy people and patientsdaysn u m b e r sThe rate of healthy people and patientsFigure 5.1 λ=2 or λ=3Conclusion:♦ Through comparing with Figure 4.2 ( λ=1 ) in model Ⅰ, it can be seen that the growth of the I (t) section is slightly reduced.♦ Observe the Figure 5.1, you can see λ=2 or λ=3 graphics haven't changed much5.2 Effect of inoculation rateIn the model Ⅲ, we have introduced the method of pulse vaccination. At the same time drew a conclusion that pulse vaccination can effectively control the spread of the virus.。
美赛论文(最终版)

For office use onlyT1________________ T2________________ T3________________ T4________________Team Control Number 46639Problem ChosenCFor office use onlyF1________________F2________________F3________________F4________________2016 MCM/ICM Summary SheetAn Optimal Investment Strategy ModelSummaryWe develop an optimal investment strategy model that appears to hold promise for providing insight into not only how to sort the schools according to investment priority, but also identify optimal investment amount of a specific school. This model considers a large number of parameters thought to be important to investment in the given College Scorecard Data Set.In order to develop the required model, two sub-models are constructed as follows: 1.For Analytic Hierarchy Process (AHP) Model, we identify the prioritizedcandidate list of schools by synthesizing the elements which have an influence on investment. First we define the specific value of any two elements’ effect on investment. And then the weight of each element’s influence on investment can be identified. Ultimately, we take the relevant parameters into the calculated weight, and then we get any school’s recommended value of investment.2.For Return On Investment M odel, it’s constructed on the basis of AHP Model.Let us suppose that all the investment is used to help the students to pay tuition fee.Then we can see optimal investment as that we help more students to the universities of higher return rate. However, because of dropout rate, there will be an optimization investment amount in each university. Therefore, we can change the problem into a nonlinear programming problem. We identify the optimal investment amount by maximizing return-on-investment.Specific attention is given to the stability and error analysis of our model. The influence of the model is discussed when several fundamental parameters vary. We attempt to use our model to prioritize the schools and identify investment amount of the candidate schools, and then an optimal investment strategy is generated. Ultimately, to demonstrate how our model works, we apply it to the given College Scorecard Data Set. For various situations, we propose an optimal solution. And we also analyze the strengths and weaknesses of our model. We believe that we can make our model more precise if more information are provided.Contents1.Introduction 21.1Restatement of the Problem (2)1.2Our Approach (2)2.Assumptions 23.Notations 34.The Optimal Investment Model 44.1Analytic Hierarchy Process Model (4)4.1.1Constructing the Hierarchy (4)4.1.2Constructing the Judgement Matrix (5)4.1.3Hierarchical Ranking (7)4.2Return On Investment Model (8)4.2.1Overview of the investment strategy (8)4.2.2Analysis of net income and investment cost (9)4.2.3Calculate Return On Investment (11)4.2.4Maximize the Total Net Income (11)5.Test the Model125.1Error Analysis (12)5.2Stability Analysis (13)6.Results136.1Results of Analytic Hierarchy Process (13)6.2Results of Return On Investment Model (14)7.Strengths and Weaknesses157.1Strengths (15)7.2Weaknesses (16)References16 Appendix A Letter to the Chief Financial Officer, Mr. Alpha Chiang.171.Introduction1.1Restatement of the ProblemIn order to help improve educational performance of undergraduates attending colleges and universities in the US, the Goodgrant Foundation intends to donate a total of $100,000,000 to an appropriate group of schools per year, for five years, starting July 2016. We are to develop a model to determine an optimal investment strategy that identifies the school, the investment amount per school, the return on that investment, and the time duration that the organization’s money should be provided to have the highest likelihood of producing a strong positive effect on student performance. Considering that they don’t want to duplicate the investments and focus of other large grant organizations, we interpret optimal investment as a strategy that maximizes the ROI on the premise that we help more students attend better colleges. So the problems to be solved are as follows:1.How to prioritize the schools by optimization level.2.How to measure ROI of a school.3.How to measure investment amount of a specific school.1.2Our ApproachWe offer a model of optimal investment which takes a great many factors in the College Scorecard Data Set into account. To begin with, we make a 1 to N optimized and prioritized candidate list of school we are recommending for investment by the AHP model. For the sake that we invest more students to better school, several factors are considered in the AHP model, such as SAT score, ACT score, etc. And then, we set investment amount of each university in the order of the list according to the standard of maximized ROI. The implement details of the model will be described in section 4.2.AssumptionsWe make the following basic assumptions in order to simplify the problem. And each of our assumptions is justified.1.Investment amount is mainly used for tuition and fees. Considering that theincome of an undergraduate is usually much higher than a high school students, we believe that it’s necessary to help more poor students have a chance to go to college.2.Bank rates will not change during the investment period. The variation ofthe bank rates have a little influence on the income we consider. So we make this assumption just to simplify the model.3.The employment rates and dropout rates will not change, and they aredifferent for different schools4.For return on investment, we only consider monetary income, regardlessof the intangible income.3.NotationsWe use a list of symbols for simplification of expression.4.The Optimal Investment ModelIn this section, we first prioritize schools by the AHP model (Section 4.1), and then calculate ROI value of the schools (Section 4.2). Ultimately, we identify investment amount of every candidate schools according to ROI (Section 4.3).4.1Analytic Hierarchy Process ModelIn order to prioritize schools, we must consider each necessary factor in the College Scorecard Data Set. For each factor, we calculate its weight value. And then, we can identify the investment necessity of each school. So, the model can be developed in 3 steps as follows:4.1.1Constructing the HierarchyWe consider 19 elements to measure priority of candidate schools, which can be seen in Fig 1. The hierarchy could be diagrammed as follows:Fig.1AHP for the investment decisionThe goal is red, the criteria are green and the alternatives are blue. All the alternatives are shown below the lowest level of each criterion. Later in the process, each alternatives will be rated with respect to the criterion directly above it.As they build their hierarchy, we should investigate the values or measurements of the different elements that make it up. If there are published fiscal policy, for example, or school policy, they should be gathered as part of the process. This information will be needed later, when the criteria and alternatives are evaluated.Note that the structure of the investment hierarchy might be different for other foundations. It would definitely be different for a foundation who doesn't care how much his score is, knows he will never dropout, and is intensely interested in math, history, and the numerous aspects of study[1].4.1.2Constructing the Judgement MatrixHierarchy reflects the relationship among elements to consider, but elements in the Criteria Layer don’t always weigh equal during aim measure. In deciders’ mind, each element accounts for a particular proportion.To incorporate their judgments about the various elements in the hierarchy, decision makers compare the elements “two by two”. The fundamental scale for pairwise comparison are shown in Fig 2.Fig 2Right now, let's see which items are compared. Our example will begin with the six criteria in the second row of the hierarchy in Fig 1, though we could begin elsewhere if we want. The criteria will be compared as to how important they are to the decisionmakers, with respect to the goal. Each pair of items in this row will be compared.Fig 3 Investment Judgement MatrixIn the next row, there is a group of 19 alternatives under the criterion. In the subgroup, each pair of alternatives will be compared regarding their importance with respect to the criterion. (As always, their importance is judged by the decision makers.) In the subgroup, there is only one pair of alternatives. They are compared as to how important they are with respect to the criterion.Things change a bit when we get to the alternatives row. Here, the factor in each group of alternatives are compared pair-by-pair with respect to the covering criterion of the group, which is the node directly above them in the hierarchy. What we are doing here is evaluating the models under consideration with respect to score, then with respect to Income, then expenditure, dropout rate, debt and graduation rate.The foundation can evaluate alternatives against their covering criteria in any order they choose. In this case, they choose the order of decreasing priority of the covering criteria.Fig 4 Score Judgement MatrixFig 5 Expenditure Judgement MatrixFig 6 Income Judgement MatrixFig 7 Dropout Judgement MatrixFig 8 Debt Judgement MatrixFig 9 Graduation Matrix4.1.3 Hierarchical RankingWhen the pairwise comparisons are as numerous as those in our example, specialized AHP software can help in making them quickly and efficiently. We will assume that the foundation has access to such software, and that it allows the opinions of various foundations to be combined into an overall opinion for the group.The AHP software uses mathematical calculations to convert these judgments to priorities for each of the six criteria. The details of the calculations are beyond the scope of this article, but are readily available elsewhere[2][3][4][5]. The software also calculates a consistency ratio that expresses the internal consistency of the judgments that have been entered. In this case the judgments showed acceptable consistency, and the software used the foundation’s inputs to assign these new priorities to the criteria:Fig 10.AHP hierarchy for the foundation investing decision.In the end, the AHP software arranges and totals the global priorities for each of the alternatives. Their grand total is 1.000, which is identical to the priority of the goal. Each alternative has a global priority corresponding to its "fit" to all the foundation's judgments about all those aspects of factor. Here is a summary of the global priorities of the alternatives:Fig 114.2 ROI Model4.2.1 Overview of the investment strategyConsider a foundation making investment on a set of N geographically dispersed colleges and university in the United States, D = {1, 2, 3……N }. Then we can select top N schools from the candidate list which has been sorted through analytic hierarchy process. The total investment amount is M per year which is donated by the Goodgrant Foundation. The investment amount is j m for each school j D ∈, satisfying the following balance constraint:j j D mM ∈=∑ (1)W e can’t invest too much or too little money to one school because we want to help more students go to college, and the student should have more choices. Then the investment amount for each school must have a lower limit lu and upper limit bu as follows:j lu m bu ≤≤ (2)The tuition and fees is j p , and the time duration is {1,2,3,4}j t ∈. To simplify ourmodel, we assume that our investment amount is only used for freshmen every year. Because a freshmen oriented investment can get more benefits compared with others. For each school j D ∈, the number of the undergraduate students who will be invested is j n , which can be calculated by the following formula :,jj j j m n j D p t =∈⨯ (3)Figure12The foundation can use the ROI model to identify j m and j t so that it canmaximize the total net income. Figure1 has shown the overview of our investment model. We will then illustrate the principle and solution of this model by a kind of nonlinear programming method.4.2.2 Analysis of net income and investment costIn our return on investment model, we first focus on analysis of net income and investment cost. Obviously, the future earnings of undergraduate students are not only due to the investment itself. There are many meaning factors such as the effort, the money from their parents, the training from their companies. In order to simplify the model, we assume that the investment cost is the most important element and we don’t consider other possible influence factors. Then we can conclude that the total cost of the investment is j m for each school j D ∈.Figure 13For a single student, the meaning of the investment benefits is the expected earnings in the future. Assuming that the student is not going to college or university after graduating from high school and is directly going to work. Then his wage base is 0b as a high school graduate. If he works as a college graduate, then his wage base is 0a . Then we can give the future proceeds of life which is represented symbolically by T and we use r to represent the bank rates which will change over time. We assume that the bank rates will not change during the investment period. Here, we use bank rates in 2016 to represent the r . The future proceeds of life of a single undergraduate student will be different due to individual differences such as age, physical condition environment, etc. If we consider these differences, the calculation process will be complicated. For simplicity’s sake, we uniform the future proceeds of life T for 20 years. Then we will give two economics formulas to calculate the total expected income in the next T years for graduates and high school graduates:40(1)Tk k a u r +==+∑(4) 40(1)T kk b h r +==+∑(5) The total expected income of a graduate is u , and the total expected income of a highschool graduate is h .Then, we continue to analyze the net income. The net income can be calculated by the following formula:os NetIncome TotalIncome C t =- (6) For each school j D ∈, the net income is j P , the total income is j Q , and the cost is j m . Then we will get the following equation through formula (6):j j j P Q m =- (7)Therefore, the key of the problem is how to calculate j Q . In order to calculate j Q, weneed to estimate the number of future employment j ne . The total number of the invested is j n , which has been calculated above. Considering the dropout rates j α and the employment rates j β for each school j , we can calculate the number of future employment j ne through the following formula:(4)(1)jt j j j j n e n βα-=⨯⨯- (8)That way, we can calculate j Q by the following formula:()j j Q ne u h =⨯- (9)Finally, we take Eq. (2) (3) (4) (7) (8) into Eq. (6), and we will obtain Eq. (9) as follows:4(4)00400(1)()(1)(1)j TT t j j j j j k kk k j jm a b P m p t r r βα+-+===⨯⨯-⨯--⨯++∑∑ (10) We next reformulate the above equation of j P for concise presentation:(4)(1)j t j jj j j jc m P m t λα-⨯⨯=⨯-- (11)where jj j p βλ= and 400400(1)(1)TT k kk k a b c r r ++===-++∑∑ .4.2.3 Calculate Return On InvestmentROI is short of return on investment which can be determined by net income andinvestment cost [7]. It conveys the meaning of the financial assessment. For each schoolj D ∈ , the net income is j P , and the investment cost equals to j m . Then the j ROIcan be calculated by the following formula:100%j j jP ROI m =⨯ (12)We substitute Eq. (10) into Eq. (11), and we will get a new formula as follows:(4)((1)1)100%j t j j j jc ROI t λα-⨯=⨯--⨯ (13)4.2.4 Maximize the Total Net IncomeGiven the net income of each school, we formulate the portfolio problem that maximize the total net income, S=Max(4)((1))j t j jj j j j Dj Djc m P m t λα-∈∈⨯⨯=⨯--∑∑ (14)S. T.jj DmM ∈=∑,{1,2,3,4}t = ,j lu m bu ≤≤ ,Considering the constraint jj DmM ∈=∑, we can further simplify the model,S is equivalent to S’=Max(4)((1))j t j jj j j Dj Djc m P t λα-∈∈⨯⨯=⨯-∑∑ (15)S. T.jj DmM ∈=∑,{1,2,3,4t = ,j l u m b u ≤≤. By solving the nonlinear programming problem S’, we can get the sameanswer as problem S.5. Testing the Model 5.1 Error AnalysisSince the advent of analytic hierarchy process, people pay more attention to it due to the specific applicability, convenience, practicability and systematization of the method. Analytic hierarchy process has not reached the ideal situation whether in theory or application level because the results depend largely on the preference and subjective judgment. In this part, we will analyze the human error problem in analytic hierarchy process.Human error is mainly caused by human factors. The human error mainly reflects on the structure of the judgment matrix. The causes of the error are the following points:1. The number of times that human judge the factors’ importance is excessive.2. The calibration method is not perfect.Then we will give some methods to reduce errors:1. Reduce times of human judgment. One person repeatedly gave the samejudgment between two factors. Or many persons gave the same judgment between two factors one time. Finally, we take the average as result.2. Break the original calibration method. If we have defined the ranking vector111121(,...)n a a a a =between the factor 1A with others. Then we can get all theother ranking vector. For example : 12122111(,1...)na a a a a =.5.2 Stability AnalysisIt is necessary to analyze the stability of ranking result [6], because the strong subjectivefactors. If the ranking result changed a little while the judgment changed a lot, we can conclude that the method is effective and the result is acceptable, and vice versa. We assume that the weight of other factors will change if the weight of one factor changed from i ξ to i η:[8](1)(,1,2...,)(1)i j j i i j n i j ηξηξ-⨯==≠- (16)And it is simple to verify the equation:11nii η==∑ (17)And the new ranking vector ω will be:A ωη=⨯ (18)By this method, the Relative importance between other factors remain the same while one of the factor has changed.6. Results6.1 Results of Analytic Hierarchy ProcessWe can ranking colleges through the analytic hierarchy process, and we can get the top N = 20 schools as follows6.2 Results of Return On Investment ModelBased on the results above, we next use ROI model to distribute investment amountj m and time duration j t for each school j D ∈ by solving the following problem:Max (4)((1))j t j jj j j Dj Djc m P t λα-∈∈⨯⨯=⨯-∑∑S. T.jj DmM ∈=∑,{1,2,3,4t = , j l u m b u≤≤ . In order to solve the problem above, we collected the data from different sources. Inthe end, we solve the model with Lingo software. The program code is as follows:model: sets:roi/1..20/:a,b,p,m,t;endsets data:a = 0.9642 0.9250 0.9484 0.9422 0.9402 0.9498 0.90490.9263 0.9769 0.9553 0.9351 0.9123 0.9410 0.98610.9790 0.9640 0.8644 0.9598 0.9659 0.9720;b = 0.8024 0.7339 0.8737 0.8308 0.8681 0.7998 0.74920.6050 0.8342 0.8217 0.8940 0.8873 0.8495 0.87520.8333 0.8604 0.8176 0.8916 0.7527 0.8659;p = 3.3484 3.7971 3.3070 3.3386 3.3371 3.4956 3.22204.0306 2.8544 3.1503 3.2986 3.3087 3.3419 2.78452.9597 2.92713.3742 2.7801 2.5667 2.8058;c = 49.5528;enddatamax=@sum(roi(I):m(I)/t(I)/p(I)*((1-b(I))^4)*c*(1-a(I)+0.05)^(4-t(I)));@for(roi:@gin(t));@for(roi(I):@bnd(1,t(I),4));@for(roi(I):@bnd(0,m(I),100));@sum(roi(I):m(I))=1000;ENDFinally, we can get the investment amount and time duration distribution as follows:7.Strengths and Weaknesses7.1Strengths1.Fixing the bank rates during the investment period may run out, but it will haveonly marginal influences.2.For return on investment, we only consider monetary income, regardless of the3.intangible income. But the quantization of these intangible income is very importantand difficult. It needs to do too much complicated technical analysis and to quantify 4.too many variables. Considering that the investment persists for a short time, thiskind of random error is acceptable.5.Due to our investment which is freshmen oriented, other students may feel unfair.It is likely to produce adverse reaction to our investment strategy.6.The cost estimation is not impeccable. We only consider the investment amount andignore other non-monetary investment.5. AHP needs higher requirements for personnel quality.7.2Weaknesses1.Our investment strategy is distinct and clear, and it is convenient to implement.2.Our model not only identifies the investment amount for each school, but alsoidentifies the time duration that the organization’s money should be provide d.3.Data processing is convenient, because the most data we use is constant, average ormedian.4.Data sources are reliable. Our investment strategy is based on some meaningful anddefendable subset of two data sets.5.AHP is more simple, effective and universal.References[1] Saaty, Thomas L. (2008). Decision Making for Leaders: The Analytic Hierarchy Process for Decisions in a Complex World. Pittsburgh, Pennsylvania: RWS Publications. ISBN 0-9620317-8-X.[2] Bhushan, Navneet, Kanwal Rai (January 2004). Strategic Decision Making: Applying the Analytic Hierarchy Process. London: Springer-Verlag. ISBN 1-8523375-6-7.[3] Saaty, Thomas L. (2001). Fundamentals of Decision Making and Priority Theory. Pittsburgh, Pennsylvania: RWS Publications. ISBN 0-9620317-6-3.[4] Trick, Michael A. (1996-11-23). "Analytic Hierarchy Process". Class Notes. Carnegie Mellon University Tepper School of Business. Retrieved 2008-03-02.[5] Meixner, Oliver; Reiner Haas (2002). Computergestützte Entscheidungs-findung: Expert Choice und AHP – innovative Werkzeuge zur Lösung komplexer Probleme (in German). Frankfurt/Wien: Redline Wirtschaft bei Ueberreuter. ISBN 3-8323-0909-8.[6] Hazelkorn, E. The Impact of League Tables and Ranking System on Higher Education Decision Making [J]. Higher Education Management and Policy, 2007, 19(2), 87-110.[7] Leslie: Trainer Assessment: A Guide to Measuring the Performance of Trainers and Facilitors, Second Edition, Gower Publishing Limited, 2002.[8] Aguaron J, Moreno-Jimenea J M. Local stability intervals in the analytic hierarchy process. European Journal of Operational Research. 2000Letter to the Chief Financial Officer, Mr. Alpha Chiang. February 1th, 2016.I am writing this letter to introduce our optimal investment strategy. Before I describe our model, I want to discuss our proposed concept of a return-on-investment (ROI). And then I will describe the optimal investment model by construct two sub-model, namely AHP model and ROI model. Finally, the major results of the model simulation will be showed up to you.Considering that the Goodgrant Foundation aims to help improve educational performance of undergraduates attending colleges and universities in the US, we interpret return-on-investment as the increased income of undergraduates. Because the income of an undergraduate is generally much higher than a high school graduate, we suggest all the investment be used to pay for the tuition and fees. In that case, if we take both the income of undergraduates’ income and dropout rate into account, we can get the return-in-investment value.Our model begins with the production of an optimized and prioritized candidate list of schools you are recommending for investment. This sorted list of school is constructed through the use of specification that you would be fully qualified to provided, such as the score of school, the income of graduate student, the dropout rate, etc. With this information, a precise investment list of schools will be produced for donation select.Furthermore, we developed the second sub-model, ROI model, which identifies the investment amount of each school per year. If we invest more money in a school, more students will have a chance to go to college. However, there is an optimal investment amount of specific school because of the existence of dropout. So, we can identify every candidate school’s in vestment amount by solve a nonlinear programming problem. Ultimately, the result of the model simulation show that Washington University, New York University and Boston College are three schools that worth investing most. And detailed simulation can be seen in our MCM Contest article.We hope that this model is sufficient in meeting your needs in any further donation and future philanthropic educational investments within the United States.。
美赛memo的格式
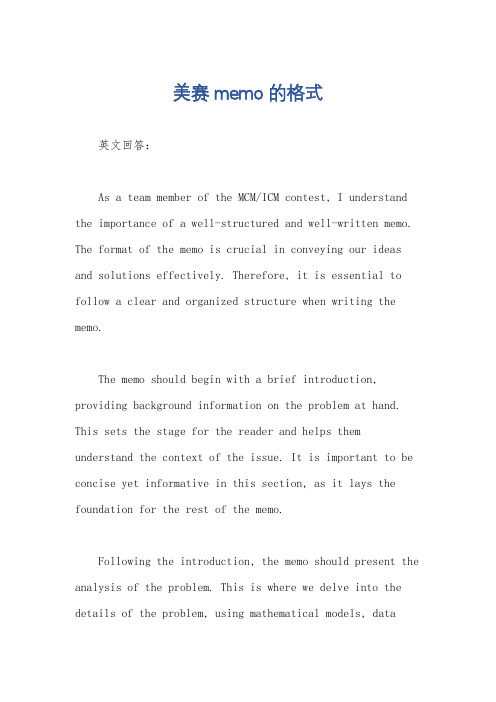
美赛memo的格式英文回答:As a team member of the MCM/ICM contest, I understand the importance of a well-structured and well-written memo. The format of the memo is crucial in conveying our ideas and solutions effectively. Therefore, it is essential to follow a clear and organized structure when writing the memo.The memo should begin with a brief introduction, providing background information on the problem at hand. This sets the stage for the reader and helps them understand the context of the issue. It is important to be concise yet informative in this section, as it lays the foundation for the rest of the memo.Following the introduction, the memo should present the analysis of the problem. This is where we delve into the details of the problem, using mathematical models, dataanalysis, and any other relevant methods to provide a thorough understanding of the issue. It is crucial to be clear and logical in our explanations, ensuring that the reader can follow our thought process.After the analysis, the memo should propose solutions to the problem. This is the crux of the memo, where we showcase our creativity and critical thinking skills. It is important to present multiple solutions, weighing the pros and cons of each, and ultimately recommending the most effective approach. Providing real-life examples or case studies can further strengthen our argument.Finally, the memo should conclude with a summary of the key points and recommendations. This section should reiterate the main findings and emphasize the proposed solutions. It is important to leave the reader with a clear understanding of our conclusions and the next steps to be taken.Overall, the memo should be well-organized, clear, and persuasive. By following a structured format and providingcompelling content, we can effectively communicate ourideas and solutions to the judges.中文回答:作为数学建模竞赛的团队成员,我深知一份结构良好、写作规范的备忘录的重要性。
MCM(美国数学建模论文)
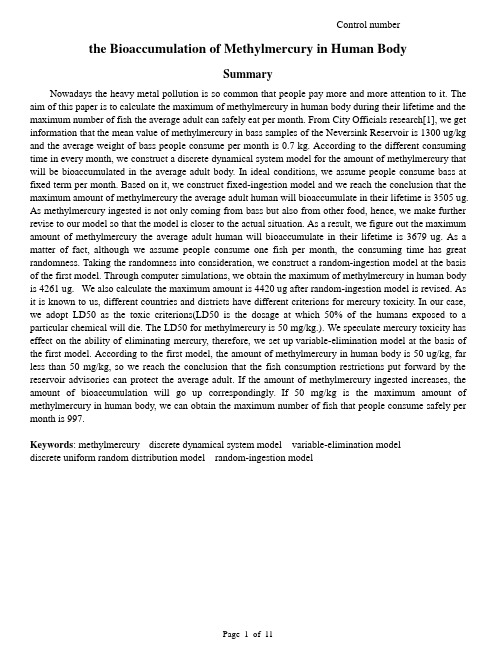
the Bioaccumulation of Methylmercury in Human BodySummaryNowadays the heavy metal pollution is so common that people pay more and more attention to it. The aim of this paper is to calculate the maximum of methylmercury in human body during their lifetime and the maximum number of fish the average adult can safely eat per month. From City Officials research[1], we get information that the mean value of methylmercury in bass samples of the Neversink Reservoir is 1300 ug/kg and the average weight of bass people consume per month is 0.7 kg. According to the different consuming time in every month, we construct a discrete dynamical system model for the amount of methylmercury that will be bioaccumulated in the average adult body. In ideal conditions, we assume people consume bass at fixed term per month. Based on it, we construct fixed-ingestion model and we reach the conclusion that the maximum amount of methylmercury the average adult human will bioaccumulate in their lifetime is 3505 ug. As methylmercury ingested is not only coming from bass but also from other food, hence, we make further revise to our model so that the model is closer to the actual situation. As a result, we figure out the maximum amount of methylmercury the average adult human will bioaccumulate in their lifetime is 3679 ug. As a matter of fact, although we assume people consume one fish per month, the consuming time has great randomness. Taking the randomness into consideration, we construct a random-ingestion model at the basis of the first model. Through computer simulations, we obtain the maximum of methylmercury in human body is 4261 ug. We also calculate the maximum amount is 4420 ug after random-ingestion model is revised. As it is known to us, different countries and districts have different criterions for mercury toxicity. In our case, we adopt LD50 as the toxic criterions(LD50 is the dosage at which 50% of the humans exposed to a particular chemical will die. The LD50 for methylmercury is 50 mg/kg.). We speculate mercury toxicity has effect on the ability of eliminating mercury, therefore, we set up variable-elimination model at the basis of the first model. According to the first model, the amount of methylmercury in human body is 50 ug/kg, far less than 50 mg/kg, so we reach the conclusion that the fish consumption restrictions put forward by the reservoir advisories can protect the average adult. If the amount of methylmercury ingested increases, the amount of bioaccumulation will go up correspondingly. If 50 mg/kg is the maximum amount of methylmercury in human body, we can obtain the maximum number of fish that people consume safely per month is 997.Keywords: methylmercury discrete dynamical system model variable-elimination modeldiscrete uniform random distribution model random-ingestion modelIntroductionWith the development of industry, the degree of environmental pollution is also increasing. Human activities are responsible for most of the mercury emitted into the environment. Mercury, a byproduct of coal, comes from acid rain from the smokestack emissions of old, coal-fired power plants in the Midwest and South. Its particles rise on the smokestack plumes and hitch a ride on prevailing winds, which often blow northeast. After colliding with the Catskill mountain range, the particles drop to the earth. Once in the ecosystem, micro-organisms in the soil and reservoir sediment break down the mercury and produce a very toxic chemical form known as methylmercury. It has great effect on human health.Public officials are worried about the elevated levels of toxic mercury pollution in reservoirs providing drinking water to the New York City. They have asked for our assistance in analyzing the severity of the problem. As a result of the bioaccumulation of methylmercury, if the reservoir is polluted, we can make sure that the amount of methylmercury in fish is also increasing. If each person adheres to the fish consumption restrictions as published in the Neversink Reservoir advisory and consumes no more than one fish per month, through analyzing, we construct a discrete dynamical system model of time for the amount of methylmercury that will bioaccumulate in the average adult person. Then we can obtain the maximum amount of methylmercury the average adult human will bioaccumulate in their lifetime. At the same time, we can also get the time that people have taken to achieve the maximum amount of methylmercury. As we know, different countries and districts have different criterions for the mercury toxicity. In our case, we adopt the criterion of Keller Army Community Hospital. If the maximum amount of methylmercury in human body is far less than the safe criterion, we can reach the conclusion that the reservoir is not polluted by mercury or the polluted degree is very low, otherwise we can say the reservoir is great polluted by mercury. Finally, the degree of pollution is determined by the amount of methylmercury in human body.Problem Onediscrete dynamical system modelThe mean value of methylmercury in bass samples of the Neversink Reservoir is 1300 ug/kg and the average weight of bass is 0.7 kg. According to the subject, people consume no more than one fish per month. For the safety of people, we must consider the bioaccumulation of methylmercury under the worst condition that people absorb the maximum amount of methylmercury. Therefore, we assume that people consume one fish per month. Assumptions● The amount of methylmercury in fish is absorbed completely and instantly by people. ● The elimination of mercury is proportional to the amount remaining. ● People absorb fixed amount of methylmercury at fixed term per month. ● We assume the half-life of methylmercury in human body is 69.3 days. SolutionsLet 1α denote the proportion of eliminating methylmercury per month, 1β denote the accumulation proportion. As we know, methylmercury decays about 50 percent every 65 to 75 days, if no further methylmercury is ingested during that time. Consequently,111,βα=-69.3/3010.5.β=Through calculating, we get10.7408.β=L et’s define the following variables :ω denotes the amount of methylmercury at initial time,n denotes the number of month,nω denotes the amount of methylmercury in human body at the moment people have just ingested the methylmercury in the month n ,1xdenotes the amount of methylmercury that people ingest per month and 113000.7910x ug ug =⨯=.Moreover, we assume0=0.ωThough,111,n n x ωωβ-=⋅+we get1011x ωωβ=⋅+ 2201111x x ωωββ=⋅+⋅+⋅⋅⋅10111111nn n x x x ωωβββ-=⋅+⋅+⋅⋅⋅+⋅+ 121111(1)n n n x ωβββ--=++⋅⋅⋅++⋅11111.1n n x βωβ--=-With the remaining amount of methylmercury increasing, the elimination of methylmercury is also going up. We know the amount of ingested methylmercury per mouth is a constant. Therefore, with time going by, there will be a balance between absorption and elimination. We can obtain the steady-state value of remaining methylmercury as n approaches infinity.1*1111111lim3505.11n n n x x ug βωββ-→∞-===--The value of n ω is shown by figure 1.Figure 1. merthylmercury completely coming from fish and ingested at fixed term per monthIf the difference of the remaining methylmercury between the month n and 1n - is less than five percent of the amount of methylmercury that people ingest per month, that is,115%.n n x ωω--<⋅Then we can get11=3380ug.ωAt the same time, we can work out the time that people have taken to achieve 3380 ug is 11 months.From our model, we reach the conclusion that the maximum amount of methylmercury the average adult human will bioaccumulate in their lifetime is 3505 ug.If people ingest methylmercury every half of a month, however, the sum of methylmercury ingested per month is constant, consequently,11910405,0.86.2x ug β===As a result, we obtain the maximun amount of methylmercury in human body is 3270ug. When the difference is within 5 %, we get the time people have taken to achieve it is 11 months.Similarly, if people ingest methylmercury per day, we get the maximum amount is 3050ug, and the time is 10 months. Revising ModelAs a matter of fact, the amount of methylmercury in human body is not completely coming from fish. According to the research of Hong Kong SAR Food and Environmental Hygiene Department [1], under normal condition, about 76 percent of methylmercury comes from fish and 24 percent comes from other seafood. In order to make our model more and more in line with the actual situation, it is necessary for us to revise it. The U.S. environmental Protection Agency (USEPA) set the safe monthly dose for methylmercury at 3 microgram per kilogram (ug/kg) of body weight. If we adopt USEPA criterion, we can calculate the amount of methylmercury that the average adult ingest from seafood is 50.4 ug per month. AssumptionsThe amount of methylmercury in the seafood is absorbed completely and instantly by people.● The elimination of methylmercury is proportional to the amount remaining. ● People ingest fixed amount of methylmercury from other seafood every day. ● We assume the half-life of methylmercury in human body is 69.3 days. SolutionsLet 0ωdenote the amount of methylmercury at initial time, t denote the number of days, t ω denote the remaining amount on the day t , and 2x denote the amount of methylmercury that people ingest per day. Moreover, we assume0=0.ωIn addition, we work out2x =50.4/30=1.68 ug.The proportion of remaining methylmercury each day is 2β, then69.320.5.β=Through calculating, we get20.99.β=Because of12221,1t t x βωβ--=-we obtain steady-state value of methylmercury1*2222211lim168.11t t t x x ug βωββ-→∞-===--If the difference of remaining methylmercury between the day t and 1t - is less than five percent of the amount of methylmercury that people ingest every day, that is,125%.t t x ωω--<⋅We have301= 160 ug.ωSo we can reach the conclusion that the maximum amount of methylmercury the average adult human will bioaccumulate from seafood is 160 ug and the time that people take to achieve the maximum is 301 days.Let 1x denote the amount of methylmercury people ingest through bass at fixed term per month, so the amount of methylmercury an average adult accumulate on the day t is1221221if t is a positive integer and not divisible by 30if t is a positive integer and divisible by 30.t t t t x x x ωωβωωβ--=⋅+⎧⎨=⋅++⎩The value of t ω is shown by figure 2.Figure 2. merthylmercury coming from fish and other seafood and ingested at fixed term per day The change oftωreflects the change of the amount of methylmercury in human body. Through revising model, we can figure out the maximum amount of methylmercury the average adult human will bioaccumulate in their lifetime is 3679 ug.Problem TwoRandom-ingestion modelAlthough people consume one fish per month, the consuming time has great randomness. We speculate the randomness has effect on the bioaccumulation of methylmercury, therefore, we construct a new model. Assumptions●The amount of methylmercury in fish is absorbed completely and instantly by people.●The elimination of methylmercury is proportional to the amount remaining.●People consume one fish per month, but the consuming time has randomness.●We assume the half-life of methylmercury in human body is 69.3 days.LetL denote the amount of methylmercury at initial time, n L denote the amount of methylmercury at the moment people have just ingested methylmercury in the month n, and x denote the amount of methylmercury that people absorb each time.We assume0=0.LWe have910.x ug=We define1βthe proportion of remaining methylmercury every day. Through69.3 10.5,β=we can get10.99.β=Let i obey discrete uniform random distribution with maximum 30 and minimum 1 and n t denote the number of days between the day1n i -of the month 1n - and the day n i of the month n , then we have-130-,n n n t i i =+(1)1.n tn n L L x β-=⋅+The value of n Lis shown by figure 3.Figure 3. merthylmercury completely coming from fish and ingested at random per monthFigure 3 shows the amount of methylmercury in human body has a great change due to the randomness of consuming time. Through the computer simulation, if we have numberless samples, n L will achieve the maximum value. That is,4261.n L ug =Revising modelIn order to make our model more accurate, we need to make further revise. We take methylmercury coming from other seafood into consideration. We know the amount of methylmercury that people ingest from other seafood every day is 1.68 ug.In that situation, we have1212.30(-1)30(-1)n n n n n n L L x if n n i L L x x if n n i ββ=⋅+≠⨯+⎧⎨=⋅++=⨯+⎩Through the computer simulation, we can get a set of data about n L shown by figure 4.Figure 4. remaining merthylmercury coming from fish consumed at random per month and other food consumed at fixedterm per dayThough the revised model, we reach the conclusion that if we have numberless samples, n L will achieve the maximum value. That is,4420.n L ug =Variable-eliminateion modelAs a matter of fact, the state of human health can affect metabolice rate so that the ability of eliminating methylmercury is not constant. We have koown the amount of methylmercury in human body will affect human health. So we can draw the conclusion that the amount of methylmercury in human body will affect the abilitity of eliminating methylmercury. Assumptions● The amount of methylmercury in fish is absorbed completely and instantly by people.● the elimination of methylmercury is not only proportional to the amount remaining, but also affected bythe change of human health which are caused by the amount of methylmercury.● People absorb fixed amount of methylmercury at fixed term per month through consuming bass. ● We assume the half-life of methylmercury in human body is 69.3 days.● In condition that no further methylmercury is ingested during a period of time, we let χ denote theeliminating proportion per month. We have known methylmercury decays about 50 percent every other day 5 to a turn 5 days, so we determine the half-life of methylmercury in human body is 69.3 days. Then we have69.3/301(1)0.5χ⋅-=. By calculating, we getχ=0.2592.We adopt LD50 as the toxic criterions, then we get the maximum amount of methylmercury in human body is 63.510⨯ ug.L et’s define the following variables :ω denotes the amount of methylmercury at initial time,n denotes the number of month,nω denotes the amount of methylmercury in human body at the moment people have just ingested the methylmercury in the month n ,n χ denotes the ability of eliminating methylmercury in the month n . γ denotes the effect on human health caused by methylmercury toxicity.1161 3.510r n n ωχχ-⎛⎫⎡⎤=⋅- ⎪⎢⎥ ⎪⨯⎣⎦⎝⎭1(1)n n n ωωχϕ-=⋅-+Hence, we have101(1)ωωχϕ=⋅-+20212(1)(1)(1)ωωχχϕχϕ=⋅-⋅-+⋅-+[]01233(1)...(1)(1)(1)...(1)(1)...(1)...(1)1n n n n n ωωχχϕχχχχχχ=⋅--+⋅-⋅--+--++-+We define the value of γ is 0.5, then we get the maximum amount of maximum in human body is 3567 ug, that is,*=3567 ug n ωNot taking the effect on the ability of eliminating maximum caused by methylmercury toxicity into account in model one,we obtain the maximum amount is 3510 ug. The difffference proves methylmercury toxicity has effect on eliminating methylmercury. We find out through calculating when r increases, the amount of methylmercury go up correspondingly. The reason for it is that methylmercury toxicity rises as a result of r increasing. Correspondingly, the effect on human health will increase, which is in accordance with fact.Problem ThreeAccording to the first model revised, we can get the maximum amount of bioaccumulation methylmercury is 3679 ug. We assume the average weight of an adult is 70 kg and the amount of methylmercury in human body is 53 ug/kg, far less than 50 mg/kg. Therefore, according to our model, the fish consumption restrictions put forward by the reservoir advisories can protect the average adult fromreaching the LD50(LD50 is the dosage at which 50% of the humans exposed to a particular chemical will die. The LD50 for methylmercury is 50 mg/kg).We assume the lethal dosage of methylmercury is not gradually increasing. If the amount of methylmercury people ingests goes up rapidly, the bioaccumulation amount will reach to a higher value. Moreover, the value probably endangers human safety. Let LD50 be the maximum amount of methylmercury in human body, that is,*n =50 m g/kg 70 kg=3500 m g.ω⨯Let 1x denote the amount of methylmercury people ingest per month. According to the first model,1*1111111lim.11n n n x x βωββ-→∞-==--We can figure out1 x =907.2 mg.We know the mean value of methylmercury in bass samples is 1.3 mg/kg, hence, we can obtain the maximum amount of fish that people consume safely per month is1m ax 698.1.3x M kg =≈The maximum number of fish is 698/0.7=997.ConclusionIn problem one, the paper calculates the final steady-state value at the same time interval per month, per half a month and per day. Through comparing the results, we get the final bioaccumulation amount of methylmercury is less, when discrete time unit is smaller. It shows when the interval of consuming fish is smaller and the sum of methylmercury ingested is constant for a period of time, the possibility of poisoning is lower.In problem two, we analyze the change of the amount of methylmercury under the condition that consuming time is random. We find out the amount of methylmercury in human body is changing constantly in fixed range, when people have just consumed fish. Moreover, the maximum is 4261 ug, which is far bigger than 3505 ug. So we can reach the conclusion that people are more endangered when the consuming time is irregular.In order to closer to the actual situation, we construct a model in which the half-life of methylmercury in human body is not constant. Through analyzing the data of computer simulation, the maximum amount of methylmercury will increase, that is, the risk of poisoning will be higher.Control numberReferences[1] Dr.D.N.Rahni, PHD. Airborne Mercury Contamination and the NeversinkReservoir./dnabirahni/rahnidocs/Envsc/Airborne%20Mercury%20Contamination%20and %20the%20Neversink%20Reservoir.doc[2] Hu Dong Bai Ke. Bass. /wiki%E9%B2%88%E9%B1%BC.[3] Centre for Food Safety Food and Environmental Hygiene Department The Government of the HongKong Special Administrative Region. Mercury in Fish and Food Safety..hk/english/Programmme/programme_rafs/Programme_rafs_fc_01_19_mercury_in_fi sh.html.Page 11 of 11。
MCMICM论文格式

Journal Citation(to be inserted by the publisher)Copyright by Trans Tech PublicationsYour Paper's Title Starts Here:Please Centeruse Arial14First Author1,Second Author2and Others3(use Arial14)1Full address of first author,including country,email(use Arial11)2Full address of second author,including country,email3List all distinct addresses in the same wayKeywords::List the keywords covered in your paper.These keywords will also be used by the Keywordspublisher to produce a keyword index.(use Arial11)For the rest of the paper,please use Times New Roman12Abstract.This document explains and demonstrates how to prepare your camera-ready manuscript for Trans Tech Publications.The best is to read these instructions and follow the outline of this text. The text area for your manuscript must be17cm wide and25cm high(6.7and9.8inches,resp.). Do not place any text outside this e good quality,white paper of approximately21x29cm or8x11inches.Your manuscript will be reduced by approximately20%by the publisher.Please keep this in mind when designing your figures and tables etc.IntroductionAll manuscripts must be in English.Please keep a second copy of your manuscript in your office (just in case anything gets lost in the mail).When receiving the manuscript,we assume that the corresponding authors grant us the copyright to use the manuscript for the book or journal in question.Should authors use tables or figures from other Publications,they must ask the corresponding publishers to grant them the right to publish this material in their paper.Use italic for emphasizing a word or phrase.Do not use boldface typing or capital letters except for section headings(cf.remarks on section headings,below).Use a laser printer,not a matrix dot printer.Organization of the TextSection Headings.The section headings are in boldface capital and lowercase letters.Second level headings are typed as part of the succeeding paragraph(like the subsection heading of this paragraph).Page Numbers.Do not print page numbers:Please number each sheet slightly in the left corner near the bottom(outside the typing area)with a light blue pencil.Footnotes.Footnotes1should be single spaced and separated from the text.Ideally,footnotes appear on the page of their reference,and are placed at the foot of the text,separated from the text by a horizontal line.Tables.Tables(refer with:Table1,Table2,...)should be presented as part of the text,but in such a way as to avoid confusion with the text.A descriptive title should be placed above each table. The caption should be self-contained and placed below or beside the table.Units in tables should be given in square brackets[meV].If square brackets are not available,use curly{meV}or standard brackets(meV).1This is a footnote2Title of Publication(to be inserted by the publisher)Figures.Figures(refer with:Fig.1,Fig.2,...)also should be presented as part of the text, leaving enough space so that the capt-ion will not be confused with the text.The caption should be self-contained and placed below or beside the figure.Generally,only original drawings or photographic reproductions are acceptable.Only very good photocopies are acceptable.Utmost care must be taken to insert the figures in correct alignment with the text.Half-tone pictures should be in the form of glossy prints.If possible,please include your figures as graphic images in the electronic version.If TTP is required to scan and insert images,please keep the following points in mind:(a)the allotted space(for inserting illustrations)must exactly match the space made available inthe camera-ready version,so that the electronic version is identical to the hard copy with regard to page and line breaks.(b)the required positioning of any high-quality separate illustration must be clearly indicated onits reverse side.The size of the illustrations must exactly match the space left in the camera-ready manuscript.Equations.Equations(refer with:Eq.1,Eq.2,...)should be indented5mm(0.2").There should be one line of space above the equation and one line of space below it before the text continues.The equations have to be numbered sequentially,and the number put in parentheses at the right-hand edge of the text.Equations should be punctuated as if they were an ordinary part of the text.Punctuation appears after the equation but before the equation number,e.g.c2=a2+b2.(1)Literature ReferencesReferences are cited in the text just by square brackets[1].(If square brackets are not available, slashes may be used instead,e.g./2/.)Two or more references at a time may be put in one set of brackets[3,4].The references are to be numbered in the order in which they are cited in the text and are to be listed at the end of the contribution under a heading References,see our example below.SummaryOn your floppy disk,please indicate the format and word processor used.Please also provide your phone number,fax number and e_mail address for rapid communication with the publisher(will not be published).Please always send your disk along with a hard copy that must match the disk's content exactly.If you follow the foregoing,your paper will conform to the requirements of the publisher and facilitate a problem-free publication process.References[1]Dj.M.Maric,P.F.Meier and S.K.Estreicher:Mater.Sci.Forum Vol.83-87(1992),p.119[2]M.A.Green:High Efficiency Silicon Solar Cells(Trans Tech Publications,Switzerland1987).This document is available on the web at /download **Please submit your paper in hardcopy and also electronically to the conference editor.。
美国大学生数学建模竞赛MCM写作模板(各个部分)
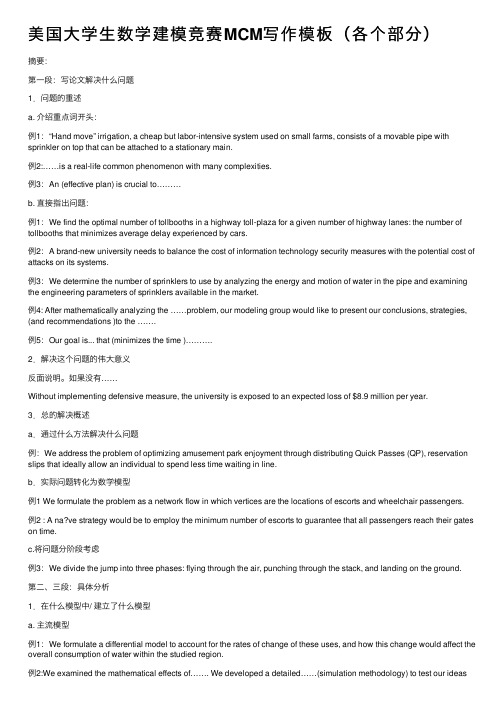
美国⼤学⽣数学建模竞赛MCM写作模板(各个部分)摘要:第⼀段:写论⽂解决什么问题1.问题的重述a. 介绍重点词开头:例1:“Hand move” irrigation, a cheap but labor-intensive system used on small farms, consists of a movable pipe with sprinkler on top that can be attached to a stationary main.例2:……is a real-life common phenomenon with many complexities.例3:An (effective plan) is crucial to………b. 直接指出问题:例1:We find the optimal number of tollbooths in a highway toll-plaza for a given number of highway lanes: the number of tollbooths that minimizes average delay experienced by cars.例2:A brand-new university needs to balance the cost of information technology security measures with the potential cost of attacks on its systems.例3:We determine the number of sprinklers to use by analyzing the energy and motion of water in the pipe and examining the engineering parameters of sprinklers available in the market.例4: After mathematically analyzing the ……problem, our modeling group would like to present our conclusions, strategies, (and recommendations )to the …….例5:Our goal is... that (minimizes the time )……….2.解决这个问题的伟⼤意义反⾯说明。
MCM美赛论文集

高教社杯全国大学生数学建模竞赛承诺书我们仔细阅读了中国大学生数学建模竞赛的竞赛规则。
我们完全明白,在竞赛开始后参赛队员不能以任何方式(包括电话、电子邮件、网上咨询等)与队外的任何人(包括指导教师)研究、讨论与赛题有关的问题。
我们知道,抄袭别人的成果是违反竞赛规则的,如果引用别人的成果或其他公开的资料(包括网上查到的资料),必须按照规定的参考文献的表述方式在正文引用处和参考文献中明确列出。
我们郑重承诺,严格遵守竞赛规则,以保证竞赛的公正、公平性。
如有违反竞赛规则的行为,我们将受到严肃处理。
我们参赛选择的题号是(从A/B/C/D中选择一项填写):A我们的参赛报名号为(如果赛区设置报名号的话):99999所属学校(请填写完整的全名):西安交通大学参赛队员(打印并签名):1.一作者2.二作者3.三作者指导教师或指导教师组负责人(打印并签名):导师日期:2011年8月1日赛区评阅编号(由赛区组委会评阅前进行编号):2011高教社杯全国大学生数学建模竞赛编号专用页赛区评阅编号(由赛区组委会评阅前进行编号):赛区评阅记录(可供赛区评阅时使用):评阅人评分备注全国统一编号(由赛区组委会送交全国前编号):全国评阅编号(由全国组委会评阅前进行编号):全国大学生数学建模竞赛L A T E X2ε模板摘要这是数学建模论文模板mcmthesis的示例文件。
特别地,这篇文档是“全国大学生数学建模竞赛(CUMCM)”模板的示例文件。
这个模板使用于参加高教社杯全国大学生数学竞赛的同学准备他们的建模论文,帮助他们更多的关注于论文内容而非论文的排版。
这个模板的设计是根据2010年修订的《全国大学生数学建模竞赛论文格式规范》[1]制作,完全符合该论文格式规范,但是该模板未得到官方认可,请使用者自己斟酌使用。
这个示例文档逐条展示其对[1]的实现效果,并对所有自定义命令进行说明。
这个示例文件还包含了一些对公示、插图、表格、交叉引用、参考文献、代码等的测试部分,以展示其效果,并作简要的使用说明。
MCM优秀论文
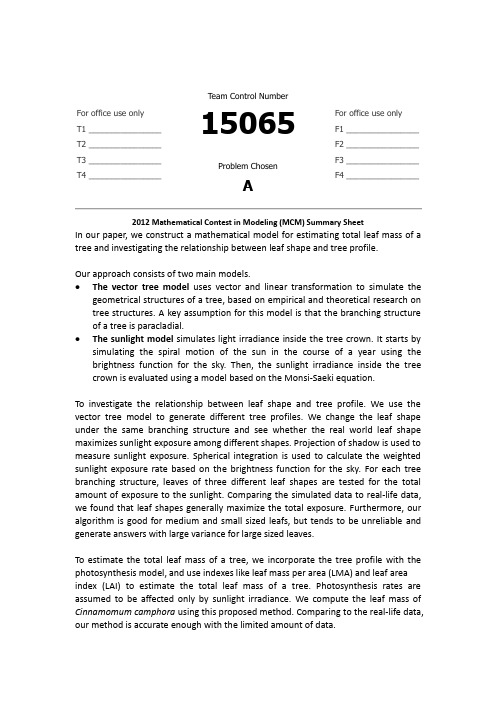
For office use onlyT1________________ T2________________ T3________________ T4________________Team Control Number15065Problem ChosenAFor office use onlyF1________________F2________________F3________________F4________________2012 Mathematical Contest in Modeling (MCM) Summary SheetIn our paper, we construct a mathematical model for estimating total leaf mass of a tree and investigating the relationship between leaf shape and tree profile.Our approach consists of two main models.●The vector tree model uses vector and linear transformation to simulate thegeometrical structures of a tree, based on empirical and theoretical research on tree structures. A key assumption for this model is that the branching structure of a tree is paracladial.●The sunlight model simulates light irradiance inside the tree crown. It starts bysimulating the spiral motion of the sun in the course of a year using the brightness function for the sky. Then, the sunlight irradiance inside the tree crown is evaluated using a model based on the Monsi-Saeki equation.To investigate the relationship between leaf shape and tree profile. We use the vector tree model to generate different tree profiles. We change the leaf shape under the same branching structure and see whether the real world leaf shape maximizes sunlight exposure among different shapes. Projection of shadow is used to measure sunlight exposure. Spherical integration is used to calculate the weighted sunlight exposure rate based on the brightness function for the sky. For each tree branching structure, leaves of three different leaf shapes are tested for the total amount of exposure to the sunlight. Comparing the simulated data to real-life data, we found that leaf shapes generally maximize the total exposure. Furthermore, our algorithm is good for medium and small sized leafs, but tends to be unreliable and generate answers with large variance for large sized leaves.To estimate the total leaf mass of a tree, we incorporate the tree profile with the photosynthesis model, and use indexes like leaf mass per area (LMA) and leaf area index (LAI) to estimate the total leaf mass of a tree. Photosynthesis rates are assumed to be affected only by sunlight irradiance. We compute the leaf mass of Cinnamomum camphora using this proposed method. Comparing to the real-life data, our method is accurate enough with the limited amount of data.Geometrical Tree Leaf mass & leaf-tree relationshipTeam # 15065Content1.Introduction (3)1.1Outline of Our Approach (3)1.2General Assumptions (4)2Vector Tree Model (4)2.1Leaf classification (4)2.2Branching Structure Model (6)3Sunlight Model (10)3.1Orbit of the sun & Brightness function for the sky (10)3.2Modeling the light irradiation at a certain position inside the tree crown (11)4Leaf shape & tree profile/branching structure (12)4.1Sunlight exposure rate (12)4.2Matching leaf shape with tree profile/branching structure (14)5Leaf Mass of a Tree (15)5.1Computation of Leaf Mass per Area Ratio (LMA) (16)5.2Total leaf Area (18)5.3Case study: a real tree—Cinnamomum camphora (19)5.4Correlation between the leaf mass and the size characteristics of the tree (21)6Improving the model (23)6.1Leaf classification (23)6.2Exposure area of leaves (23)6.3Determinants of photosynthesis rate (23)7Conclusion (23)8Letter to a scientific journal editor (24)Reference (24)Appendix: Matlab code for implementing our model (26)1.IntroductionIn this paper we present a mathematical model for investigating the relationship between tree profiles and leaf shapes and estimating the total leaf mass of a tree. Specifically, we created a tree profile using 3-dimensional coordinates and linear transformation. We simulate the sunlight irradiance in the tree crown using the brightness function of the sky and Monsi and Saeki equation (Monsi, 1953). We then incorporate the sunlight irradiance into different tree profiles to find a leaf shape that maximizes sunlight exposure. The chosen shapes match with real leaf shapes, suggesting a close relationship between leaf shape and tree profile: leaf shape maximizes the overall sunlight exposure under a given tree profile. We then incorporate the tree profile with the photosynthesis model (Tsukaya, 2006), and use indexes like leaf mass per area (LMA) and leaf area index (LAI) to estimate the total leaf mass of a tree.1.1Outline of Our ApproachWe first introduce two models which will be useful in the latter applications:●Vector Tree Model: The first part of our paper will be devoted to presenting thetheoretical framework of this model. Our objective is to create a spatial structure of the tree crown (branching structure and leaf distribution). We use vectors to simulate leaf shape, distribution of leaves on branches, and the tree profile/branching structure. Linear transformation of vectors is used to simulate the relationship between daughter branch and parent branch.●Sunlight Model: The second part of the paper will introduce the sunlight model.Our objective is to simulate solar irradiance across a year. Brightness function over the celestial sphere is used to describe solar irradiance from different directions, Monsi and Saeki equation is used to calculate the light attenuation ina tree crown due to overlapping of leaf shadows.The latter sections present two applications of the models.•Investigating the Relationship between Leaf Shape and Tree Profile We use the vector tree model to construct different tree profiles. Combining the tree profile with the sunlight model, we are able to calculate the sunlight exposure rate of the leaves. We then adopt different leaf shapes for the same tree profile and find the one that maximizes the sunlight exposure. Comparing this chosen leaf shape with the real leaf shape of the tree, if there is a match, we may conclude that the leaf shape is associated with the tree profile in that it maximizes the sunlight exposure.•Estimating Total Leaf Mass of a TreeGiven a tree, we use the vector tree model to simulate its profile, and use the sunlight model to determine the light irradiance at each leaf. Then, we derive the relationship between light irradiance and the leaf mass per area (LMA) according to a photosynthesis model. Thus, we can derive the LMA for each leaf and calculate the weighted average LMA for the entire tree. We then use the sunlight model again to find the shadow of the tree crown and calculate the total leaf area of the tree using leaf area index (LAI). Finally, the total leaf mass is calculated by multiplying LMA with the total leaf area.1.2General Assumptions●Trees are assumed to be paracladial, i.e. if any branch is cut off, it has the samestructural characteristics, apart from size, as the parent from which it is cut.●The majority of leaves will grow on the last generation of branches.●Sun light is assumed to propagate in a straight line. Diffraction and refraction oflights are ignored.●Photosynthesis rate is assumed to be affected only by the rate of light irradiance.Other factors such as CO2concentration in the air are assumed to be homogeneous at any part of the tree crown.●Photosynthesis due to light sources other than the sun is neglected. (e.g.moonlight at night and artificial light)●The environmental destructive effects are minimized and thus negligible, such asnatural disasters and herbivory.●Nutrient supplies are sufficient.2Vector Tree Model2.1Leaf classificationThe leaf is a major part of the plant-body plan. How to classify different leaves? Traditional plant taxonomy focuses on leaf functions and leaf shape. Recent research also suggests that venation is a strong indicator in leaf classification.The general leaf shape is an important factor for the plant to receive sunlight. Traditional parameters in describing leaf shape include presence/absence of leaf petiole, flatness, leaf index (a ratio of leaf length to leaf width), margin type, and overall size. (Tsukaya, 2006)Since we are going to construct a computerized simulation of a tree, is it important for us to classify leaves quantitatively such that they are easy for representation and simulation. Hence we develop a model to classify the leaves into 4 basic shape categories.To classify a given leaf to one of the four basic shape categories, we focus on 3 factors of the leaf: shape convexity, leaf index (ratio of leaf length to leaf width), and the position of the longest width on a leaf.To determine the shape convexity, we first simulate a leaf using a polygon. We first fit the leaf into a grid with each cell size 0.5cm×0.5cm. Next we select the outmost grids that have been covered more than half by the leaf. Then we plot and connect the centers of these grids. Hence we have a simulating polygon of the leaf.Two illustrations are shown as below.We are now able to determine the convexity of the polygon of leaf—convex polygons are such that all diagonals lie entirely inside the polygon; concave polygons are such that some diagonals will lie outside the polygon. Hence we can determine the convexity of the leaf.If a leaf is concave, we immediately classify it as Palmate. Convex leaves are left for further determination.To classify other leaves, the second factor we look at is leaf index, which is the ratioof leaf length to leaf width. According to empirical data (Tsukaya, 2006) (Johnson, 1990), we classify leaves with leaf ratio of 3.5 and above as Linear. Those with leaf ratio below 3.5 will be either Elliptic or Deltoid.To classify Elliptic leaf and Deltoid leaf, we look at the position of longest-width on a leaf. In order to have a more quantitative view, we are interested in the ratio of distance between leaf tip and longest-width-position (D) to the leaf length (L).If the ratio (D/L) lies within [1/4, 3/4], we classify the leaf as Elliptic. Leaves whose ratio<1/4 or >3/4 are Deltoid.To summarize the decision rule of classifying a leaf, we provide a decision flow chart.2.2 Branching Structure2.2.1 Biological Backgrounds and Existing ModelsThe existing geometrical models simulating branching structures of trees are essentially empirical based on a rule that specifies the relative angular direction and length of a daughter branch to its parent branch (Johnson, 1990). In order to obtain a more realistic model, studies considering whorls and bifurcations as rule in branching simulation have also been carried out (Fisher, 1977) (Fisher, 1979).Convex ≥3.5 Concave ∈[1/4, 3/4] Leaf polygon: convex?Palmate Leaf indexLinear Ratio of distance between leaf tipand longest-width-position (D) tothe leaf length (L) EllipticDeltoid<1/4 or >3/4 <3.52.2.2Model Description(/01/002_en.html)In this model, our objective is to represent each branching point and the position of each leaf using their position vector (x,y,z) in a 3D coordinate shown above with z-axis set to be the main stem of the tree.2.2.2.1Child Branch FormationGiven the length and location of a parent branch, we want to find the direction and length of the child branch. At this point, the Child branches generated will subject to the following:a)Number of child branches at the branching point N is given byN=[θ∗σ(k)],where●k: A species-wise constant coefficient which is related to the mean of thenumber of child branches for the species.●θ:Uncertainty compensator which incorporates the genetic uncertainties andenvironmental uncertainties.●σ: A function which maps the species of a tree to the mean number of childbranches generated at this branching point.b)At each branching point, the length and direction of the N child branches aredetermined by multiplying the direction vector of the parent branch withtransformation matrices A1,A2,…,A N respectively.A i is defined as the following:A i(θi,φi)=λi∗(cosφi sinφi0−cosθi sinφi cosθi cosφi sinθi sinθi sinφi−sinθi cosφi cosθi)where λi is the length change, φi is the rotated angle with respect to the parent branch about Oz and θi is the rotated angle with respect to the parent branch about local x axis Ox with respect to the parent branch. Note that at each branching point, the coordinate system adopted for this transformation will be the local coordinate system with respect to the local parent branch, and the parent branch will lie on the z-axis.c)Now, we are able to determine the coordinate of each branch.We first number the branches according to the generating sequence. Let r N denotes the vector representing the branch of the N th generated branch. If r t is the parent branch of r k, then the equation:r k=A j r tis to be satisfied, where A j is the transformation matrix that governs the rule between t th branch and k th branch (abbreviated as branch t and branch k in later context).Let R N denote the coordinate of N th generated branch.Then R1=r1and R k=R t+ r k.In particular, in the situation where only bifurcations are concerned (Johnson, 1990),{r2k=A1r k r2k+1=A2r kand{R2k=r2k+R k R2k+1=r2k+1+R k2.2.2.2Leaf FormationNow, we are ready to construct leaves on the branches.Define set A={r p ϵ Tree| ∀ r k ϵ Tree,brancℎ p is not tℎe parent of brancℎ k} and the growing of leaves is subject to the following:a)Basic phyllotaxis patterns:(/phyllo//About/Classification.html)●Distichous Phyllotaxis, in distichous phyllotaxis, leaves or other botanicalelements grow one by one, each at 180 degrees from the previous one.;●Whorled Phyllotaxis, In whorled phyllotaxis, two or more elements grow at thesame node on the stem.;●Spiral Phyllotaxis, In spiral phyllotaxis, botanical elements grow one by one, eachat a constant divergence angle d from the previous one.;●Multijugate Phyllotaxis, elements in a whorl (group of elements at a node) arespread evenly around the stem and each whorl is at a constant divergence angled from the previous one.b)The spacing of leaves on the branch is determined by the function d(I)which takes in the intensity of sunlight irradiance and outputs the spacing ofthe leaves.c)The probability that a leaf grows at a certain spot is determined by thefunction p(I)which takes in the intensity of sunlight irradiance and outputs the probability that a leaf is likely to grow on a certain spot of the branch.In our model, for each r p ϵ A, we grow the leaves on branch p according to the archived Basic phyllotaxis patterns for different species. The spacing between two different potential leaf-growing spots is d(I)and the probability of a leaf growing ata certain spot is p(I).2.2.3Different tree profiles generated by our modelUsing the model introduced above, we are able to generate a wide range of tree profiles by varying the coefficients in the model. Three examples are shown below.Zooming in to the picture, the leaves are represented as this:3Sunlight ModelPhotosynthesis is important for the growth of leaves, and it is driven by solar energy. Thus, in this section, we will consider the light irradiance in a tree crown. By modeling the sun radiation using brightness function, and using Monsi-Saeki equation (Monsi, 1953) to describe the light attenuation within a tree crown, we will be able to calculate light irradiance at any point within the tree crown.3.1Orbit of the sun & Brightness function for the skyOrbit of the sunSince the sun moves in a spiral during the course of a year (in the view of an observer standing on the earth), we will first model its orbit using celestial sphere. At different latitude, the sun will trace out a different trajectory in a year. Three examples are shown below.North temperate zone Equator the Arctic PoleBrightness function for the skyThe brightness function (Johnson, 1990) for the sky is defined in terms of spherical polar coordinates (r,θ,φ), although it does not involve the r coordinate. When defining the position of the sun, θis known as the zenith angle (when θ=0, the sun is directly overhead) and φis termed the azimuth angle.The brightness function of the sky is defined as B(θ,φ), with units W m-2 srad-1. The steradian (srad) is the SI unit for solid angle: a solid angle is the area of the surface of a portion of sphere divided by the square of the radius of the sphere. Thus the total solid angle of a sphere is 4πsradDeriving the brightness functionTo illustrate the method of deriving B(θ,φ), we use trees on the equator as an example. Brightness function for the sky at other latitude and longitude can be derived in a similar way with slight modification of the algorithm.A BOn the equator, the orbit of the sun will shift from circle A to circle B from summer solstice to winter solstice (strictly speaking it is not a circle but a spiral).We denote the rate of solar radiation that reaches the earth as E with unit of W m-2 s-1. E is known from previous research of solar energy.When the sun is at a certain position (θ,φ)on the celestial sphere, the rate of solar energy that is absorbed by the earth surface (E a) depends on the angle between the ground and the light beam (γ).−φ)E a≈E×sinγ, (γ=π2Thus, since we know the orbit of the sun in the course of a year, the brightness function can be derived by integration of E a over time (t). This should be done by computer simulation and discretization of the spiral trajectory is used for ease of implementation.3.2Sunlight irradiation inside the tree crownIn plant models, light attenuation in a canopy is generally described by the equation (known as Monsi and Saeki equation (Monsi, 1953)):I(l)=I0e−kswhere I0and I(l)(W (m2 ground)-1) are the irradiances above and within the tree crown respectively at path-length s, and k(m2ground(m2 leaf)-1) is known as theextinction coefficient which is assumed to be constant.For any point P within the tree crown, the path-length s varies with the angles θ and φ. Monsi-Saeki equation can be used to describe the attenuation along the path-length s that the radiation passes to reach P . And according to our definition of brightness function, I 0= B (θ,φ). Thus, the total irradiation at a point P within the plant I p is given by the integralI p =∫∫B (θ,φ)e −ks cosθsinφdθdφ2ππ/2In practice, this integral is evaluated numerically using computer programs.4 Leaf shape & tree profile/branching structureIn this section, we use the model developed in chapter 2 to investigate the relationship between leaf shape and tree profile/branching structure. We raise a hypothesis that for any tree profile, the leaf shape will maximize its exposure to sunlight.To test this hypothesis, we calculate the sunlight exposure rate for each type of leaf shape under a given branching structure. Then, we will choose the leaf shape which maximizes sunlight exposure. If the leaf shape chosen coincides with the real life shape, our hypothesis is true.4.1 Sunlight exposure rateTo define the sunlight exposure rate of leaves, we first ignore the motion of the sun in the sky and assume that the sun is always directly overhead of the tree. Then, the sunlight exposure rate (SE) is defined asSE =∑ A i TA,Where A i is the area of shadow casted by an individual leave with index i (denoted by pink dots on the graph below), and TA is the total area within thePsB (θ,φ)borderline of the shadow casted by the tree crown (denoted by the red circle on the graph below). SE measures the proportion of sunlight on the tree crown that is blocked by leaves.Now, we consider the motion of the sun in the sky. We have introduced the concept of brightness function for the sky B(θ,φ) in section 2.2 which models the motion of sun in the course of a year by assigning each point (θ,φ) on the celestial sphere a solar irradiance rate.We define the sunlight exposure rate for each direction (θ,φ) in a similar way to the overhead sunlight.SE(θ,φ)=∑ A i (θ,φ)TA(θ,φ)Then, the overall sunlight exposure rate for the tree is an integral of SE (θ,φ) over the entire celestial sphere with weight B (θ,φ) for each (θ,φ):SE total =∫∫B(θ,φ)∙SE (θ,φ)cosθsinφdθdφ2π0π/2N,where N =∫∫B(θ,φ)cosθsinφdθdφ2π0π/2is a normalization factor. The range ofSE total is [0, 1].4.2Matching leaf shape with tree profileNow, we are ready to investigate the relationship between leaf shape and branching structures.As illustrated in section 2, we classify leaf shapes into the following categories.We choose three types of tree profiles to study:Profile 1: pine tree Profile 2: poplar tree Profile 3: Janpanese bananaFor each tree profile, we calculate the SE total for the four types of leaves and chose the one with the highest SE total. We set d (the distance between adjacent leaves on the same branch) and S (the size of a leaf) based on data in ‘manual of leaf architecture’(Beth Ellis, 2009) To derive the brightness function of the sky, we assume that the tree is located at a place with latitude: 45o N. The results are shown below:tree tree Japanese bananabranches:0.53 0.62 0.820.670.490.47Shape shapeSince the results are generally expected, our hypothesis stands, i.e. there is correlation between leaf shapes and tree structures: leaf shapes tend to maximize sunlight exposure of this tree. This provides insights into why leaves have different shapes. A larger pool of tree profiles should be studied using this model before we can draw a solid conclusion on the exact relationship. However, that is beyond the mathematical context of this topic, we leave it for readers who are interested to investigate further.5 Leaf Mass of a TreeIn this section, we will use the vector tree model and sunlight model to estimate the total leaf mass of a tree. The general formula to calculate total leaf mass of a tree is Total leaf mass =weighted Leaf mass per area ratio (LMA )×total leaf area The computation of LMA and leaf area will be introduced.This figure is the detailed implementation of poplar tree with Deltoid leaves (numerical discretion is adopted). From the figure we could see that different angles of sun will influence the exposure area of the leaves on the tree. In our implementation, when θ and φ are small, the light irradiance on the tree is largest. We computed the weighted sum according to the method described in the previous sections.5.1 Leaf Mass per Area Ratio (LMA)Leaf Mass per Area (LMA) is defined as the ratio of leaf mass over its area (g m-2).In this section, we describe a method to calculate the average LMA for the tree.Since Leaves on a tree have different thicknesses due to different photosynthesis rate determined by the sunlight irradiance at the leaf (SARAH J. COOKSON1, 2005), they tend to have different LMA. Thus, we need to calculate LMA for each leaf based on their photosynthesis rate and then calculate the weighted average of all the individual LMAs.5.1.1 Photosynthesis RateWe adopted the model in (Johnson, 1990) which relates the internal gross photosynthetic rate P with sunlight irradiance I l , internal CO 2 concentration of the plant C i and the CO 2 concentration in the air C a .P =αI l C i r x αI l +C ir x(*)where α is known as the photochemical efficiency (kg CO 2 J -1) and r x as the carboxylation resistance (sm −1).Next, denoteP n as the net photosynthetic rate, and the following equation issatisfiedP n =P -R d(**)andP n =C a -C ir d(***)where R d is the constant dart respiration rate,r d is the diffusion rate.Use (*) (**) and (***) to solve for P and P n , we get0=P n 2r d -P n [a I 1(r x +r d )+C a -R d r d ]+a I 1C a -R d (a I 1r x +C a ) Therefore, we getP n =dHowever, by (Johnson, 1990) only the negative root is biologically valid, thus we can solve for P n . In our model, I l is obtained from the sunlight model in section 3, C a r x r d R d are all assumed to be constant at any position in the tree crown.5.1.2 Leaf Mass Per Area Ratio (LMA) Affected by Venation NetworksIn modern ecology, LMA is an important measure in classifying different kinds of leaves. By observing the venation network of a given leaf area, one can also find a surprisingly correlated relationship between three venation functional traits and LMA (Benjamin Blonder, 2011). We follow the modeling process of the derivation of LMA with respect to the following functional traits: ● Density σ : total path length of veins in the area of interest (ROI) divided bythe ROI area; ● Distance d : the mean diameter of the largest circular masks that fit in eachclosed loop ● Loopiness x : the mean number of closed loops in ROIIn (Benjamin Blonder, 2011) the derivation of the relationship between LMA and three functional traits shows the following relationship:d =k 0d(1)LMA =p r V 2(r V -r L )s +2r L dk 0(2)Where ● ρv is the inner radius of the veins in the ROI ● r v is mass density of the terminal veins ● ρl is the mass density of the lamina ● δ is the thickness of the leaf at ROI ● k 0 is a constant which relates d and δ5.1.3 Peak Carbon Assimilation Rate Per MassIn [BB], a detailed model is given to derive the Peak Carbon Assimilation Rate per Mass (A m )A m =[p c 0(1-h )k 0n s a s WUE ]s[2r L d +k 0p r V 2(r V -r L )s ][(p t s +sqrt (p a s ))s +2a s n s log (d k 0r V)] (3)where a s is the maximum aperture of a stomate, n s is the number density of open stomata, t s is the thickness of a stomatal pore, D is the temperature and pressure dependent diffusion constant of water in air, C 0 is the temperature and pressure dependent saturation vapor concentration of water in air, WUE is water-use efficiency and h is the relative humidity ( (Buck, 1981) (Nobel, 1999).5.1.4 Derivation of LMANow we use the results in the above sections to derive LMA. One observation we make is P n ≤A m , since A m is the peak photosynthetic rate. Therefore, we use m which accounts for environmental factor such thatm Pn =Am(4) .Therefore, by assumption, we can solve for LMA combining equations (1) – (4) even if the thickness of the leaf d is unknown. Then we can get the desired LMA. By applying the above method for each leaf, we will be able to get the average LMA for the tree.5.2Total leaf AreaWe will calculate the total leaf area of a tree by the following formula:Total leaf area=Leaf Area Index(LAI)× ground area covered by the tree crown In the following sections, we will introduce the concept of LAI and the method of getting the ground area covered by the tree crown from our vector tree model.5.2.1Leaf Area IndexLeaf Area Index (LAI) is defined as the one sided green leaf area per unit ground area in broadleaf canopies, or as the projected needle leaf area per unit ground area in needle canopies.It is an indispensable parameter in studying plant physiology, since vegetation surface is an important determinant of various plant functions such as photosynthesis and transpiration. Methods used for measuring LAI in hardwood forests include destructive sampling, allometric equations, litter fall, and light interception based techniques. (Vose et al, 1995)A data set for LAI has been compiled containing 1008 records of worldwide data on leaf area index for the time period 1945-2000. (Scurlock, 2001)5.2.2Ground area of a treeWe can derive the ground area of a tree from the vector tree model introduced in section 2.2. By computer simulation, we project the tree crown onto the ground, and plot the margin of the projection. Hence calculate the ground surface area according to the projected polygon hull in the computer. Below is an illustration of a simulated tree and its crown projection.。
美赛论文模板(超实用)

TitileSummaryDuring cell division, mitotic spindles are assembled by microtubule-based motor proteins1, 2. The bipolar organization of spindles is essential for proper segregation of chromosomes, and requires plus-end-directed homotetrameric motor proteins of the widely conserved kinesin-5 (BimC) family3. Hypotheses for bipolar spindle formation include the 'push−pull mitotic muscle' model, in which kinesin-5 and opposing motor proteins act between overlapping microtubules2, 4, 5. However, the precise roles of kinesin-5 during this process are unknown. Here we show that the vertebrate kinesin-5 Eg5 drives the sliding of microtubules depending on their relative orientation. We found in controlled in vitro assays that Eg5 has the remarkable capability of simultaneously moving at 20 nm s-1 towards the plus-ends of each of the two microtubules it crosslinks. For anti-parallel microtubules, this results in relative sliding at 40 nm s-1, comparable to spindle pole separation rates in vivo6. Furthermore, we found that Eg5 can tether microtubule plus-ends, suggesting an additional microtubule-binding mode for Eg5. Our results demonstrate how members of the kinesin-5 family are likely to function in mitosis, pushing apart interpolar microtubules as well as recruiting microtubules into bundles that are subsequently polarized by relative sliding. We anticipate our assay to be a starting point for more sophisticated in vitro models of mitotic spindles. For example, the individual and combined action of multiple mitotic motors could be tested, including minus-end-directed motors opposing Eg5 motility. Furthermore, Eg5 inhibition is a major target of anti-cancer drug development, and a well-defined and quantitative assay for motor function will be relevant for such developmentsContentTitile (1)Summary (1)1Introduction (1)1.1Restatement of the Problem (1)1.2Background (1)1.1.1Common Solving Technique (1)1.1.2Previous Works (1)1.3Example (1)2Analysis of the Problem (1)2.1Outline of the Approach (1)2.2Basic Assumptions (2)2.3Definitions and Key Terms (2)3Calculating and Simplifying the Model (2)4The Model Results (3)5Validating the Model (3)6Strengths and Weaknesses (3)6.1Strengths (3)6.2Weaknesses (3)7Food for Thought (3)8Conclusion (3)References (4)Appendices (4)Appendix A Source Code (4)Appendix B (4)1Introduction1.1Restatement of the Problem …1.2Background…1.1.1Common Solving Technique…1.1.2Previous Works…1.3Example…2Analysis of the Problem …2.1Outline of the Approach…2.2Basic Assumptions●●●●●2.3Definitions and Key Terms●●●●Table 1.…Symbol Meaning Unit3Calculating and Simplifying the Model …4The Model Results……5Validating the Model…6Strengths and Weaknesses6.1S trengths●●●●6.2W eaknesses●●●●7Food for Thought…8Conclusion….References…AppendicesAppendix A Source CodeHere are the simulation programmes we used in our model as follow. Input matlab source:……….Appendix B…….Input C++ source:…………..…………..。
美赛论文模板
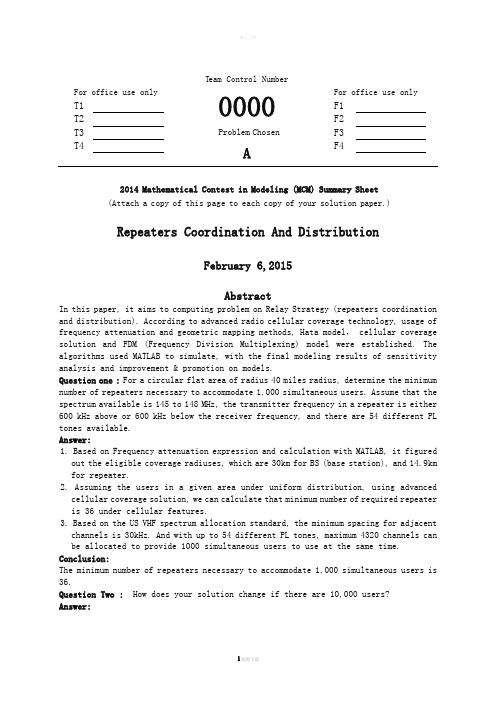
T eam Control NumberFor office use only0000For office use onlyT1 F1T2 F2T3 Problem Chosen F3T4 A F42014 Mathematical Contest in Modeling (MCM) Summary Sheet(Attach a copy of this page to each copy of your solution paper.)Repeaters Coordination And DistributionFebruary 6,2015AbstractIn this paper, it aims to computing problem on Relay Strategy (repeaters coordination and distribution). According to advanced radio cellular coverage technology, usage of frequency attenuation and geometric mapping methods, Hata model, cellular coverage solution and FDM (Frequency Division Multiplexing) model were established. The algorithms used MATLAB to simulate, with the final modeling results of sensitivity analysis and improvement & promotion on models.Question one : For a circular flat area of radius 40 miles radius, determine the minimum number of repeaters necessary to accommodate 1,000 simultaneous users. Assume that the spectrum available is 145 to 148 MHz, the transmitter frequency in a repeater is either 600 kHz above or 600 kHz below the receiver frequency, and there are 54 different PL tones available.Answer:1. Based on Frequency attenuation expression and calculation with MATLAB, it figuredout the eligible coverage radiuses, which are 30km for BS (base station), and 14.9km for repeater.2. Assuming the users in a given area under uniform distribution, using advancedcellular coverage solution, we can calculate that minimum number of required repeater is 36 under cellular features.3. Based on the US VHF spectrum allocation standard, the minimum spacing for adjacentchannels is 30kHz. And with up to 54 different PL tones, maximum 4320 channels can be allocated to provide 1000 simultaneous users to use at the same time. Conclusion:The minimum number of repeaters necessary to accommodate 1,000 simultaneous users is 36.Question Two : How does your solution change if there are 10,000 users?Answer:1. Since the given spectrum is in a fixed range, even if 54 different PL tones can not be allocated enough channels for 10,000 simultaneous users. So the number of repeaters will be increased, meanwhile, the given area will be divided into different parts.2. On the assumption that uniform distribution of the population in the given area, it will be divided into 3 sub-regions equally by analyzing the binding domain, frequency spectrum and PL tones three independent factors. And then the number of repeaters within each sub-region will be classified discussion.3. The FDM (Frequency Division Multiplexing) model is established here to improve channel efficiency to accommodate up to 10,000 simultaneous users Conclusion:The minimum number of repeaters necessary to accommodate 10,000 simultaneous users is 126.Question Three : Discuss the case where there might be defects in line-of-sight propagation caused by mountainous areas. Answer:Basically, under the same condition for question 1&2, the mountainous area will be analyzed as following:1. The function for relationship between radio attenuation x caused by obstacles and the eligible coverage radius d for repeater is 2249.354371.4110x d -=, which is to analyze the impact on the number of repeaters under full signal coverage. 2. For the mountain barrier, based on the different situation of mountains, the addition of repeaters on the suitable location will be discussed to achieve full coverage. This paper describes model established by using of cellular coverage technology and frequency attenuation expression, to achieve simple, fast, accurate algorithm. And also illustrated the effect takes the entire article. In the end, the sensitivity analysis and error calculation are applied for modeling, making the model practically.Key words: Cellular Coverage technology, frequency attenuation expression, channel allocation, MatlabRepeaters coordination and distributionContent1 Restatement of the Problem (1)1.1 Introduction (1)1.2 The Problem (1)2 Simplifying Assumption (1)3 Phrase explain (1)4 Model (2)4.1 Model I (2)4.1.1 Analysis of the Problem (2)4.1.2 Model Design (2)5 Sensitivity analysis (2)6 Model extension (2)7 Evaluating our model (2)7.1 The strengths of model (2)7.2 The weaknesses of model (2)References (3)1 Restatement of the Problem1.1 IntroductionThe VHF radio spectrum involves line-of-sight transmission and reception. This limitation can be overcome by “repeaters,” which pick up weak signals, amplify them, and retransmit them on a different frequency. Thus, using a repeater, low-power users (such as mobile stations) can communicate with one another in situations where direct user-to-user contact would not be possible. However, repeaters can interfere with one another unless they are far enough apart or transmit on sufficiently separated frequencies.1.2 The ProblemYour job is to:◆Design a scheme that determines the minimum number of repeaters necessaryto accommodate 1,000 simultaneous users in a circular flat area of radius40 miles radius.And assume that the spectrum available is 145 to 148 MHz,the transmitter frequency in a repeater is either 600 kHz above or 600 kHz below the receiver frequency, and there are 54 different PL tones available.◆Change your scheme to accommodate 1,0000 simultaneous users base on yourmodel.◆Discuss the case where there might be defects in line-of-sight propagationcaused by mountainous areas.2 Simplifying Assumption3 Phrase explain4 Model4.1 Model I4.1.1 Analysis of the Problem4.1.2 Model Design5 Sensitivity analysisSymbol◆N: the number of total repeaters in the circle area ◆Q: the number of the users in the circle area◆k: the number of the red circle in figure 2最前面最好有一个Symbol List6 Model extension7 Evaluating our model7.1 The strengths of model7.2 The weaknesses of modelReferences参考文献不要引用非常差的期刊的论文,要引用比较厉害的英文期刊,证明你有足够的阅读文献量。
美赛论文mcm模版

Number of People Entered Shanghai World Expo ParkAbstract:Key words:Contents1. Introduction (3)1.1 Why does toll way collects toll? (3)1.2 Toll modes (3)1.3 Toll collection methods (3)1.4 Annoyance in toll plazas (3)1.5 The origin of the toll way problem (3)1.6 Queuing theory (4)2. The Description of Problem (5)2.1 How do we approximate the whole course of paying toll? (5)2.2 How do we define the optimal configuration? (5)2.2.1 From the perspective of motorist (5)2.2.2 From the perspective of the toll plaza (6)2.2.3 Compromise (6)2.3 Overall optimization and local optimization (6)2.4 The differences in weights and sizes of vehicles (7)2.5 What if there is no data available? (7)3. Models (7)3.1 Basic Model (7)3.1.1 Symbols and Definitions (7)3.1.2 Assumptions (8)3.1.3 The Foundation of Model (9)3.1.4 Solution and Result (11)3.1.5 Analysis of the Result (11)3.1.6 Strength and Weakness (13)3.2 Improved Model (14)3.2.1 Extra Symbols (14)3.2.2 Additional Assumptions (14)3.2.3 The Foundation of Model (14)3.2.4 Solution and Result (15)3.2.5 Analysis of the Result (18)3.2.6 Strength and Weakness (19)4. Conclusions (19)4.1 Conclusions of the problem (19)4.2 Methods used in our models (19)4.3 Application of our models (19)5. Future Work (19)5.1 Another model (19)5.2 Another layout of toll plaza (23)5.3 The newly- adopted charging methods (23)6.References (23)7.Appendix (23)Programs and codes (24)I. IntroductionIn order to indicate the origin of the toll way problems, the following background is worth mentioning.1.11.21.31.41.51.6II. The Description of the Problem2.1 How do we approximate the whole course of paying toll?●●●●2.2 How do we define the optimal configuration?1) From the perspective of motorist:2) From the perspective of the toll plaza:3) Compromise:2.3 The local optimization and the overall optimization●●●Virtually:2.4 The differences in weights and sizes of vehicles2.5 What if there is no data available?III. Models3.1 Basic Model3.1.1 Terms, Definitions and SymbolsThe signs and definitions are mostly generated from queuing theory.●●●●●3.1.2 Assumptions●●●●●3.1.3 The Foundation of Model1) The utility function●The cost of toll plaza:●The loss of motorist:●The weight of each aspect:●Compromise:2) The integer programmingAccording to queuing theory, we can calculate the statistical properties as follows.3)The overall optimization and the local optimization●The overall optimization:●The local optimization:●The optimal number of tollbooths:3.1.4 Solution and Result1) The solution of the integer programming:2) Results:3.1.5 Analysis of the Result●Local optimization and overall optimization:●Sensitivity: The result is quite sensitive to the change of the threeparameters●Trend:●Comparison:3.1.6 Strength and Weakness●Strength: In despite of this, the model has proved that . Moreover, wehave drawn some useful conclusions about . T he model is fit for, such as●Weakness: This model just applies to . As we have stated, . That’sjust what we should do in the improved model.3.2 Improved Model3.2.1 Extra SymbolsSigns and definitions indicated above are still valid. Here are some extra signs and definitions.●●●●3.2.2 Additional Assumptions●●●Assumptions concerning the anterior process are the same as the Basic Model.3.2.3 The Foundation of Model1) How do we determine the optimal number?As we have concluded from the Basic Model,3.2.4 Solution and Result1) Simulation algorithmBased on the analysis above, we design our simulation arithmetic as follows.●Step1:●Step2:●Step3:●Step4:●Step5:●Step6:●Step7:●Step8:●Step9:2) Flow chartThe figure below is the flow chart of the simulation.3) Solution3.2.5 Analysis of the Result3.2.6 Strength and Weakness●Strength: The Improved Model aims to make up for the neglect of .The result seems to declare that this model is more reasonable than the Basic Model and much more effective than the existing design.●Weakness: . Thus the model is still an approximate on a large scale. Thishas doomed to limit the applications of it.IV. Conclusions4.1 Conclusions of the problem●●●4.2 Methods used in our models●●●4.3 Applications of our models●●●V. Future Work5.1 Another model5.1.1The limitations of queuing theory 5.1.25.1.35.1.41)●●●●2)●●●3)●●●4)5.2 Another layout of toll plaza5.3 The newly- adopted charging methodsVI. References[1][2][3][4]VII. Appendix。
mcm格式
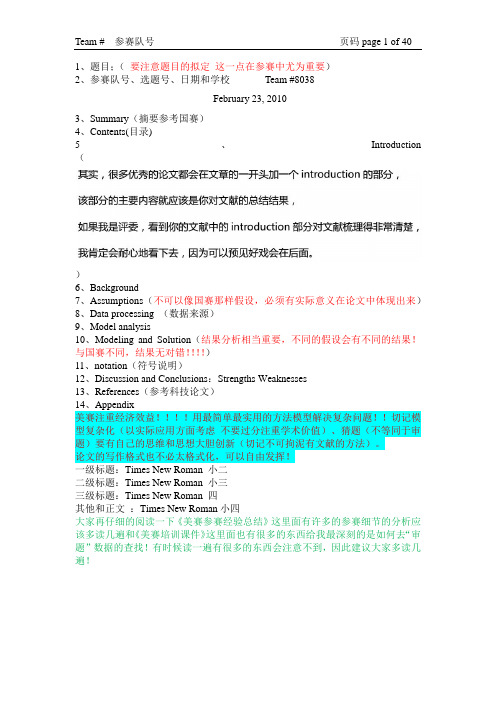
1、题目;(要注意题目的拟定这一点在参赛中尤为重要)2、参赛队号、选题号、日期和学校Team #8038February 23, 20103、Summary(摘要参考国赛)4、Contents(目录)5、Introduction ()6、Background7、Assumptions(不可以像国赛那样假设,必须有实际意义在论文中体现出来)8、Data processing (数据来源)9、Model analysis10、Modeling and Solution(结果分析相当重要,不同的假设会有不同的结果!与国赛不同,结果无对错!!!!)11、notation(符号说明)12、Discussion and Conclusions:Strengths Weaknesses13、References(参考科技论文)14、Appendix美赛注重经济效益!!!!用最简单最实用的方法模型解决复杂问题!!切记模型复杂化(以实际应用方面考虑不要过分注重学术价值)、猜题(不等同于审题)要有自己的思维和思想大胆创新(切记不可拘泥有文献的方法)。
论文的写作格式也不必太格式化,可以自由发挥!一级标题:Times New Roman 小二二级标题:Times New Roman 小三三级标题:Times New Roman 四其他和正文:Times New Roman小四大家再仔细的阅读一下《美赛参赛经验总结》这里面有许多的参赛细节的分析应该多读几遍和《美赛培训课件》这里面也有很多的东西给我最深刻的是如何去“审题”数据的查找!有时候读一遍有很多的东西会注意不到,因此建议大家多读几遍!美国赛虽未这样具体明确地指出评审原则,但从其大量评述文章中可以看出假设和合理性、建模的创造性和文字表述的清晰程度也是其重要标准,只是没有一篇评述文章强调结果的正确性,反而有评委强调:由于所作的假设和建立的模型各不相同甚至相差较大,因此结果亦可能有相当大的差别。
美赛(mcm)论文LaTeX模板
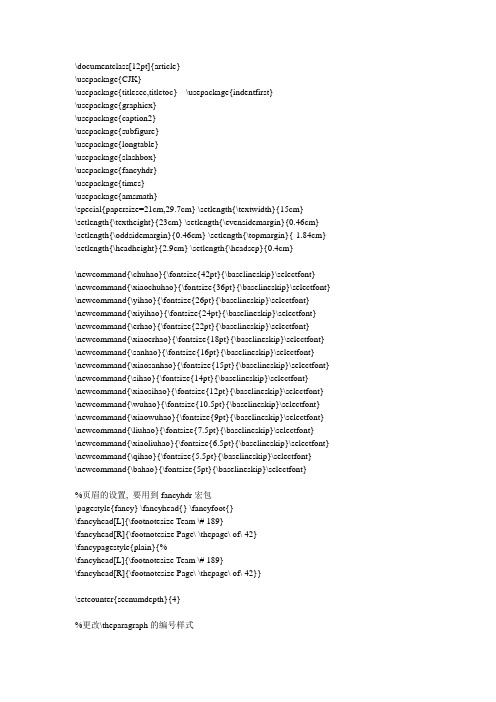
\documentclass[12pt]{article}\usepackage{CJK}\usepackage{titlesec,titletoc} \usepackage{indentfirst}\usepackage{graphicx}\usepackage{caption2}\usepackage{subfigure}\usepackage{longtable}\usepackage{slashbox}\usepackage{fancyhdr}\usepackage{times}\usepackage{amsmath}\special{papersize=21cm,29.7cm} \setlength{\textwidth}{15cm}\setlength{\textheight}{23cm} \setlength{\evensidemargin}{0.46cm}\setlength{\oddsidemargin}{0.46cm} \setlength{\topmargin}{-1.84cm} \setlength{\headheight}{2.9cm} \setlength{\headsep}{0.4cm}\newcommand{\chuhao}{\fontsize{42pt}{\baselineskip}\selectfont}\newcommand{\xiaochuhao}{\fontsize{36pt}{\baselineskip}\selectfont} \newcommand{\yihao}{\fontsize{26pt}{\baselineskip}\selectfont}\newcommand{\xiyihao}{\fontsize{24pt}{\baselineskip}\selectfont}\newcommand{\erhao}{\fontsize{22pt}{\baselineskip}\selectfont}\newcommand{\xiaoerhao}{\fontsize{18pt}{\baselineskip}\selectfont} \newcommand{\sanhao}{\fontsize{16pt}{\baselineskip}\selectfont}\newcommand{\xiaosanhao}{\fontsize{15pt}{\baselineskip}\selectfont} \newcommand{\sihao}{\fontsize{14pt}{\baselineskip}\selectfont}\newcommand{\xiaosihao}{\fontsize{12pt}{\baselineskip}\selectfont} \newcommand{\wuhao}{\fontsize{10.5pt}{\baselineskip}\selectfont} \newcommand{\xiaowuhao}{\fontsize{9pt}{\baselineskip}\selectfont} \newcommand{\liuhao}{\fontsize{7.5pt}{\baselineskip}\selectfont}\newcommand{\xiaoliuhao}{\fontsize{6.5pt}{\baselineskip}\selectfont} \newcommand{\qihao}{\fontsize{5.5pt}{\baselineskip}\selectfont}\newcommand{\bahao}{\fontsize{5pt}{\baselineskip}\selectfont}%页眉的设置, 要用到fancyhdr宏包\pagestyle{fancy} \fancyhead{} \fancyfoot{}\fancyhead[L]{\footnotesize Team \# 189}\fancyhead[R]{\footnotesize Page\ \thepage\ of\ 42}\fancypagestyle{plain}{%\fancyhead[L]{\footnotesize Team \# 189}\fancyhead[R]{\footnotesize Page\ \thepage\ of\ 42}}\setcounter{secnumdepth}{4}%更改\theparagraph的编号样式\makeatletter\renewcommand{\theparagraph}{\@arabic\c@paragraph}\makeatother%章节格式的设置\titleformat{\section}{\erhao\bf}{}{0em}{}[]\titleformat{\subsection}{\xiaoerhao\bf}{}{0em}{}[]\titleformat{\subsubsection}{\sanhao\bf}{}{0em}{}[]\titleformat{\paragraph}[hang]{\vspace*{0.5ex}\sihao\bf}{\hspace*{1em}\theparagraph)}{0.5em }{}[\vspace*{-0.5ex}]%更改插图的标题\renewcommand{\figurename}{\wuhao\bf\sf Figure}\renewcommand{\captionlabeldelim}{\ }%更改表格的标题\renewcommand{\tablename}{\wuhao\bf\sf Table}%更改图形或表格与其标题的间距\setlength{\abovecaptionskip}{10pt}\setlength{\belowcaptionskip}{10pt}%定义产生不浮动图形和表格的标题的命令\figcaption和\tabcaption\makeatletter\newcommand\figcaption{\def\@captype{figure}\caption}\newcommand\tabcaption{\def\@captype{table}\caption}\makeatother%自定义的可以调整粗细的水平线命令, 用于绘制表格, 调用格式\myhline{0.5mm}.\makeatletter\def\myhline#1{%\noalign{\ifnum0=`}\fi\hrule \@height #1 \futurelet\reserved@a\@xhline}\makeatother%第一层列表序号为带圈的阿拉伯数字\renewcommand{\labelenumi}{\textcircled{\arabic{enumi}}}%更改脚注设置\renewcommand{\thefootnote}{\fnsymbol{footnote}}\begin{document}\begin{CJK*}{GBK}{song}\CJKtilde\title{\bf\yihao Aviation Baggage Screening\\{\&} Flight Schedule}\author{}\date{}\maketitle\section{Introduction}Following the terrorist attacks on September 11, 2001, there isintense interest in improving the security screening process forairline passengers and their baggage. Airlines and airports areconsidered high-threat targets for terrorism, so aviation securityis crucial to the safety of the air-travelling public. Bombs andexplosives have been known to be introduced to aircraft by holdbaggage and cargo, carried on by passengers, and hidden withinaircraft supplies.At present To Screen or Not to Screen, that is a Hobson's choice.US Current laws mandate 100{\%} screening of all checked bags at the 429passenger airports throughout the nation by explosive detection systems(EDS) by the end of the Dec 31 2003. However, because the manufacturers arenot able to produce the expected number of EDS required to meet the federalmandate, so it is significant to determine the correct number of devicesdeploy at each airport, and to take advantage of them effectively.The Transportation Security Administration (TSA) needs a complicatedanalysis on how to allocate limited device and how to best use them.Our paper contains the mathematical models to determine the number of EDSsand flight schedules for all airports in Midwest Region. We also discuss theETD devices as the additional security measures and the future developmentof the security systems.\section{Assumption and Hypothesis}\begin{itemize}\item The passengers who will get on the same airplane will arrive uniformly, namely the distribution is flat.\item The detection systems, both EDS and ETD, operate all the time during peak hour, except downtime.\item The airline checks the passengers randomly, according to its claim.\item The passengers, who are just landing and leave out, do not have to be checked through EDS or ETD.\item According to the literature, the aircraft loads approximately equal among the sets of departing flight during the peak hour.\item The landing flight did not affect the departure of the plane.\item Once a passenger arrives, he can go to EDS to be checked, except he has to wait in line.\item Once passengers finish screening, they can broad on the plane in no time.\item During peak hours, a set of flights departs at the same time every the same minutes.\item All the runways are used as much as possible during peak hours.\item The maximum number of the baggage is two, which a passenger can carry on plane. []\item The detection machine examines the bags at the same speed.\item EDS cannot make mistakes that it detect a normal object as an explosive.\end{itemize}\section{Variable and Definition}\begin{longtable}{p{100pt}p{280pt}}\caption{Variables}\\ %第一页表头的标题\endfirsthead %第一页的标题结束\caption{(continued)}\\ %第二页的标题\endhead %第二页的标题结束\hline\hline\textbf{Symbol}&\textbf{Description}\\\hline$n_{ij}$&The airplane number of the $i^{\mathrm{th}}$ type in the $j^{\mathrm{th}}$ flight set\\\hline${NP}_i\:(i=1,2,\ldots)$&The number of passengers on each airplanes of the same type.\\\hline$\xi_{ij}\:(i,j = 1,2,\cdots)$&The number of baggage on each airplane of the $j^{\mathrm{th}}$ flights\\\hline$a$&The maximal number of airplanes type\\\hline$B_j^{set}$&The total baggage number of each set of flight\\\hline${NF}_i$&Number of airplanes of each type\\\hline$\bar{\rho}$&The mean value of passengers' baggage coming per minute in every flight set\\ \hline$N_{set}$&The number of flight sets\\\hline$B_{total}$&The total number of checked baggage during the peak hour\\\hline$H_{peak}$&The length of the peak hour\\\hline$T_{set}$&The time length during which each flight set's passengers wait to be checked\\\hline$\Delta t$&The time interval between two consecutive flight set\\\hline$N_{EDS}$&The number of all the EDSs\\\hline$N_{shadow}$&The number of flight sets whose passengers will be mixed up before being checked\\\hline$v_{EDS}$&The number of baggage checking by one EDS per minute\\\hline$\rho_j$&The number of passengers' baggage coming per minute in one flight set\\\hline$N_{runway}$&The number of an airport's runway\\\hline\\*[-2.2ex]${\bar{B}}^{set}$&The mean value of checked baggage number of every flight set\\\hline$M$&The security cost\\\hline\hline\label{tab1}\end{longtable}\subsubsection{Definition:}\begin{description}\item[Flight set] A group of flights take off at the same time\item[The length of peak hour] The time between the first set of flight and the last set\end{description}\section{Basic Model}During a peak hour, many planes and many passengers would departfrom airports. Therefore, It is difficult to arrange for thepassengers to enter airports. If there are not enough EDSs forpassengers' baggage to check, it will take too long time for themto enter. That would result in the delay of airplanes. On thecontrary, if there are too many EDSs, it will be a waste. It isour task to find a suitable number of EDSs for airport. In orderto reach this objective, we use the linear programming method tosolve it.\subsection{Base analysis}The airplanes are occupied at least partly. The passengers'baggage would be checked by EDSs before they get on the airplanes.We have assumed that every passenger carry two baggages. Thisassumption would simplify the problem. According to the data fromthe problem sheet, we can obtain the useful information thatairlines claim 20{\%} of the passengers do not check any luggage,20{\%} check one bag, and the remaining passengers check two bags.Therefore, we can gain the total number of passengers' baggagethat should be carried on one plane: $\xi_{ij}$. Moreover, we canget the equation that calculate $\xi_{ij}$:\[\xi_{ij}={NP}_i\times 20\%+{NP}_i\times 60\%\times 2\]We define the matrix below as airplane baggage number matrix:\[\overset{\rightharpoonup}{\xi}_j=\left[\xi_{1j}\quad\xi_{2j}\quad\cdots\quad\xi_{ij}\quad\cdots\ right]\]We define the matrix below as flight schedule matrix:\[\left[\begin{array}{llcl}n_{11}&n_{12}&\cdots&n_{1N_{set}}\\n_{21}&n_{22}&\cdots&n_{2N_{set}}\\\multicolumn{4}{c}\dotfill\\n_{a1}&n_{a2}&\cdots&n_{aN_{set}}\end{array}\right]\]In this matrix, $n_{ij}$ is the airplane number of the$i^{\mathrm{th}}$ type in the $j^{\mathrm{th}}$ flight set whichwill take off. Apparently, this value is an integer.We define the matrix below as flight set baggage number matrix:\[\left[B_1^{set}\quad B_2^{set}\quad\cdots\quad B_j^{set}\quad\cdots\quad B_a^{set}\right] \]It is clear that they meet the relation below:\begin{equation}\begin{array}{cl}&\left[\xi_{1j}\quad\xi_{2j}\quad\cdots\quad\xi_{ij}\quad\cdots\right]\cdot\left[\begin{array}{llcl}n_{11}&n_{12}&\cdots&n_{1N_{set}}\\n_{21}&n_{22}&\cdots&n_{2N_{set}}\\\multicolumn{4}{c}\dotfill\\n_{a1}&n_{a2}&\cdots&n_{aN_{set}}\end{array}\right]\\=&\left[B_1^{set}\quad B_2^{set}\quad\cdots\quad B_j^{set}\quad\cdots\quadB_a^{set}\right]\end{array}\label{Flight:baggage}\end{equation}Then, we know:\[B_j^{set}=\sum\limits_{i=1}^a\xi_{ij}\times n_{ij}\]There are some constraints to the equation (\ref{Flight:baggage}).First, for each set of flight, the total number of airplanesshould be less than the number of runways. Second, the totalairplane number of the same type listed in the equation(\ref{Flight:baggage}) from every set of flight should be equal tothe actual airplane number of the same type during the peak hour.We can express them like these:\[\sum\limits_{i=1}^a n_{ij}\le N_{runway}\quad\quad\sum\limits_{j=1}^b n_{ij}={NF}_i \]We should resolve the number of flight sets. According to our assumptions,during the peak hour, the airlines should make the best use of the runways.Then get the number of flight sets approximately based on the number of allthe airplanes during the peak hour and that of the runways. We use anequation below to express this relation:\begin{equation}N_{set}=\left\lceil\frac{\sum\limits_{j=1}^{N_{set}}\sum\limits_{i=1}^an_{ij}}{N_{runway}}\right\rceil\label{sets:number}\end{equation}The checked baggage numbers of each flight set are equal to eachother according to our assumption. We make it based on literature.It can also simplify our model. We define $\bar{B}^{set}$ as themean value of checked baggage number of every flight set.Moreover, We define $\bar{\rho}$ as the mean value of checkedbaggage number of every flight set per minute:\[\bar{B}^{set}=\frac{B_{total}}{N_{set}}\]\[\bar{\rho}=\frac{\bar{B}^{set}}{T_{set}}=\frac{B_{total}}{T_{set}N_{set}}=\frac{B_{total}\Delta t}{T_{set}H_{peak}}\]The course of passengers' arrival and entering airport isimportant for us to decide the number of EDSs and to make the flights schedule. Therefore, we should analyze this process carefully. Passengers will arrive between forty-five minutes andtwo hours prior to the departure time, and the passengers who will get on the same airplane will arrive uniformly. Then we can getthe flow density of all checked baggage at any time during passengers' entering. This value is the sum of numbers of passengers' checked baggage coming per minute. To calculate this value, firstly, we should obtain flow density of each flight set's checked baggage. We define $\rho_j $, namely the number of checked baggage per minute of one flight set:\[\rho_j=\frac{B_j^{set}}{T_{set}}\]Secondly, we draw graphic to help us to understand. We use rectangle to express the time length for all the passengers of one flight set to come and check bags. In the graphic, the black partis the period for them to come. During the white part, no passengers for this flight set come. According to the problem sheet, the former is 75 minute, and the latter is 45 minute. The length of rectangle is 120 minute. $T_{set}$ is the period during which all passengers of one flight set wait to be checked. Sincewe have assumed that each time interval between two consecutive flight set is same value, we define $\Delta t$ as it. Observe the section that value we want to solve is $\sum\limits_j\rho_j$. Moreover, we can get another important equation from the graphic below:\begin{equation}N_{set}=\frac{H_{peak}}{\Delta t}\label{PeakHour}\end{equation}\begin{figure}[hbtp]\centering\includegraphics[width=298.2pt,totalheight=141.6pt]{fig01.eps} \caption{}\label{fig1}\end{figure}Each EDS has certain capacity. If the number of EDSs is $N_{EDS}$and one EDS can check certain number of baggage per minute (Thatis checking velocity, marked by $v_{EDS}$), the total checkingcapacity is $N_{EDS}\cdot\frac{v_{EDS}}{60}$. $v_{EDS}$ is between160 and 210.Now we can easily decide in what condition the passengers can be checkedwithout delay:\[\sum\limits_j\rho_j\le v_{EDS}\]The passengers have to queue before being checked:$\sum\limits_j\rho_j>v_{EDS}$Well then, how can we decide how many $\rho_j$? It depends on howmany flight sets whose passengers will be mixed up before beingchecked. We note it as $N_{shadow} $. Return to the Figure\ref{fig1}, we can know:\[N_{shadow}=\left\lfloor\frac{T_{set}}{\Delta t}\right\rfloor\]\begin{figure}%[htbp]\centering\includegraphics[width=240pt,totalheight=131.4pt]{fig02.eps}\caption{}\label{fig2}\end{figure}From Figure \ref{fig1} and Figure \ref{fig2}, we can get theresult as follows:\begin{enumerate}\item If $N_{shadow}\le N_{set}$, namely $H_{peak}>T_{set}$, then $\sum\limits_{j=1}^{N_{shadow}}\rho _j\le N_{EDS}\frac{v_{EDS}}{60}$\renewcommand{\theequation}{\arabic{equation}a}That is:\begin{equation}N_{EDS}\ge\frac{60}{v_{EDS}}\sum\limits_{j=1}^{N_{shadow}}\rho_j\approx\frac{60}{v_{ EDS}}N_{shadow}\bar{\rho}=\frac{60B_{total}\Deltat}{v_{EDS}T_{set}H_{peak}}N_{shadow}\label{EDS:number:a}\end{equation}\item If $N_{shadow}>N_{set}$, namely $H_{peak}\le T_{set}$, then $\sum\limits_{j=1}^{N_{set}}\rho_j\le N_{EDS}\frac{v_{EDS}}{60}$\setcounter{equation}{3}\renewcommand{\theequation}{\arabic{equation}b}That is:\begin{equation}N_{EDS}\ge\frac{60}{v_{EDS}}\sum\limits_{j=1}^{N_{set}}\rho_j\approx\frac{60}{v_{EDS} }N_{set}\bar{\rho}=\frac{60B_{total}\Delta t}{v_{EDS}T_{set}H_{peak}}N_{set}\label{EDS:number:b}\end{equation}\end{enumerate}\subsection{The number of EDSs}Then we begin to resolve the number of EDSs assisted by the linearprogramming method.EDS is operational about 92{\%} of the time. That is to say, whenever it isduring a peak hour, there are some EDSs stopping working. Then the workingefficiency of all the EDSs is less than the level we have expected.Therefore, the airline has to add more EDSs to do the work, which can bedone with less EDSs without downtime.We use binomial distribution to solve this problem. $N$ is the number ofactual EDSs with downtime and $k$ is the number of EDSs without downtime. Ifprobability is $P$, we can get the equation below:\[\left(\begin{array}{c}N\\k\end{array}\right)\cdot98\%^k\cdot(1-98\%)^{N-k}=P\]We can obtain $N$ when we give $P$ a certain value. In this paper,$P$ is 95{\%}. The $N_{EDS}$ is the actual number we obtainthrough the equation above.Now we have assumed that passengers can be checked unless be delayed by thepeople before him once he arrives at airport. Apparently, if the time lengthbetween two sets of flight is short, the density of passengers will begreat. It will bring great stress to security check and may even make somepassengers miss their flight. To resolve this question, the airline has toinstall more EDSs to meet the demand. However, this measure will cost muchmore money. Consequently, we have to set a suitable time interval betweentwo set of flight.Based on the base analysis above. We can use the equation(\ref{sets:number}) to decide the number of flight sets $N_{set}$assuming we know the number of runways of a certain airport. Thenbased on the equation (\ref{PeakHour}), we can decide the peakhour length $H_{peak}$ when we assume a time interval between two consecutive flight sets. Then we use \textcircled{1} and\textcircled{2} to decide which to choose between equation(\ref{EDS:number:a}) and equation (\ref{EDS:number:b}). In consequence, we can obtain the minimum of EDSs number.If we choose different numbers of runways and the time intervalsbetween two flight sets, we can get different EDSs numbers. Inthis paper that followed, we gain a table of some value of$N_{runway}$ and $\Delta t$ with the corresponding EDSs numbers. Moreover, we draw some figure to reflect their relation.For a certain airport, its number of runway is known. Givencertain time interval ($\Delta t$), we can get the length of thepeak hour ($H_{peak}$). When the $N_{runway}$ is few enough,perhaps $H_{peak}$ is too long to be adopted. However, for acertain airline, they can decide the time interval of their ownpeak hour. In this given time interval, they could find theminimum of $N_{runway}$ through the Figure \ref{fig3}. We draw a sketch map to describe our steps.\begin{figure}[hbtp]\centering\includegraphics[width=352.8pt,totalheight=214.2pt]{fig03.eps}\caption{}\label{fig3}\end{figure}\subsection{The Flight Schedule }According to the base analysis, we can know that the flightschedule matrix and $\Delta t$ is one form of flight timetable. In``The number of EDSs'', we can get suitable $\Delta t$. Then weshould resolve the flight schedule matrix.Because we have assumed that the checked baggage numbers of each flight set are equal to each other. It can be described as follows:\[\left\{\begin{array}{l}\rho_j\approx\bar{\rho}\\B_j^{set}\approx\bar{B}^{set}\end{array}\right.\begin{array}{*{20}c}\hfill&{j=1,2,\cdots,N_{set}}\hfill\end{array}\]The flight schedule matrix subject to this group:\[\left\{\begin{array}{ll}\sum\limits_{j=1}^{N_{set}}n_{ij}={NF}_i&i=1,2,\cdots\\\sum\limits_{i=1}^a n_{ij}\le N_{runway}&j=1,2,\cdots,N_{set}\\n_{ij}\ge0,&\mathrm{and}\:n_{ij}\:\mathrm{is}\:\mathrm{a}\:\mathrm{Integer}\end{array}\right.\]In order to make the best use of runway, we should make$\sum\limits_{i=1}^a n_{ij}$ as great as we can unless it exceed$N_{runway}$.Then we can see that how to resolve the flight schedule matrix is a problemof divide among a group of integers. This group is all the numbers of eachflight passengers' baggage in one flight set. We program for this problemusing MA TLAB and we get at least one solution in the end. However, thematrix elements we have obtained are not integer, we have to adjust them tobe integers manually.\subsection{Results and Interpretation for Airport A and B}The number of passengers in a certain flight (${NP}_i$), the timelength of security checking ($T_{set}$), the checking velocity ofEDS ($v_{EDS}$), and the number of baggage carried by onepassenger are random.\subsubsection{Data Assumption:}\begin{itemize}\item $T_{set}$ is 110 minutes, which is reasonable for airline.\item To simplify the problem, we assume that every passenger carry 2 baggage. If some of thepassengers carry one baggage, the solution based on 2 baggages per passenger meets therequirement.\item The number of runways in airport A and airport B is 5.\end{itemize}\subsubsection{Airport A:}Once the number of runway and the number of the flights aredecided, the flight schedule matrix is decided, too. We producethis matrix using MATLAB. This matrix companied by $\Delta t$ isthe flight schedule for airport A. $\Delta t$ will be calculatedin (\ref{Flight:baggage}), (\ref{sets:number}) and(\ref{PeakHour}).We calculate $N_{EDS}$ and make the flight timetable in threeconditions. The three conditions and the solution are listed asfollowed:\paragraph{Every flight are fully occupied}The checking speed of EDS is 160 bags/hour.\begin{table}[htbp]\centering\caption{}\begin{tabular}{*{11}c}\myhline{0.4mm}$\mathbf{\Deltat(\min)}$&\textbf{2}&\textbf{4}&\textbf{6}&\textbf{8}&\textbf{10}&\textbf{12}&\textbf{14} &\textbf{16}&\textbf{18}&\textbf{20}\\\myhline{0.4mm}$N_{EDS}(\ge)$&31&31&31&31&31&29&24&22&20&17\\\hline$H_{peak}(\min)$&20&40&60&80&100&120&140&160&180&200\\\myhline{0.4mm}\end{tabular}\label{tab2}\end{table}We assume that the suitable value of $H_{peak}$ is 120 minutes.Then the suitable value of $\Delta t$ is about 12 minutes, and$N_{EDS}$ is 29 judged from Figure \ref{fig4}. Certainly, we canwork $\Delta t$ and $N_{EDS}$ out through equation.\begin{figure}[htbp]\centering\includegraphics[width=294.6pt,totalheight=253.2pt]{fig04.eps}\caption{}\label{fig4}\end{figure}\paragraph{Every flight is occupied by the minimal number of passengers onstatistics in the long run.}The checking speed of EDS is 210 bags/hour.\begin{table}[htbp]\centering\caption{}\begin{tabular}{*{11}c}\myhline{0.4mm}$\mathbf{\Deltat(\min)}$&\textbf{2}&\textbf{4}&\textbf{6}&\textbf{8}&\textbf{10}&\textbf{12}&\textbf{14} &\textbf{16}&\textbf{18}&\textbf{20}\\\myhline{0.4mm}$N_{EDS}(\ge)$&15&15&15&15&15&14&13&12&10&7\\\hline$H_{peak}(\min)$&20&40&60&80&100&120&140&160&180&200\\\myhline{0.4mm}\end{tabular}\label{tab3}\end{table}We assume that the suitable value of $H_{peak}$ is 120 minutes.Then the suitable value of $\Delta t$ is about 12 minutes, and$N_{EDS}$ is 14 judging from Figure \ref{fig5}. Certainly, we canwork $\Delta t$ and $N_{EDS}$ out through equation.\begin{figure}[htbp]\centering\includegraphics[width=294.6pt,totalheight=253.2pt]{fig05.eps}\caption{}\label{fig5}\end{figure}\paragraph{${NP}_i$ and $v_{EDS}$ are random value produced by MATLAB.}\begin{table}[htbp]\centering\caption{}\begin{tabular}{*{11}c}\myhline{0.4mm}$\mathbf{\Deltat(\min)}$&\textbf{2}&\textbf{4}&\textbf{6}&\textbf{8}&\textbf{10}&\textbf{12}&\textbf{14} &\textbf{16}&\textbf{18}&\textbf{20}\\\myhline{0.4mm}$N_{EDS}(\ge)$&15&22&21&21&15&17&21&16&13&14\\\hline$H_{peak}(\min)$&20&40&60&80&100&120&140&160&180&200\\\myhline{0.4mm}\end{tabular}\label{tab4}\end{table}We assume that the suitable value of $H_{peak}$ is 120 minutes.Then the suitable value of $\Delta t$ is about 12 minutes, and$N_{EDS}$ is 17 judging from Figure \ref{fig6}. Certainly, we canwork $\Delta t$ and $N_{EDS}$ out through equation.\begin{figure}[htbp]\centering\includegraphics[width=294.6pt,totalheight=249.6pt]{fig06.eps}\caption{}\label{fig6}\end{figure}\subsubsection{Interpretation:}By analyzing the results above, we can conclude that when$N_{EDS}$ is 29, and $\Delta t$ is 12, the flight schedule willmeet requirement at any time. The flight schedule is:\\[\intextsep]\begin{minipage}{\textwidth}\centering\tabcaption{}\begin{tabular}{c|*{8}c|c|c}\myhline{0.4mm}\backslashbox{\textbf{Set}}{\textbf{Type}}&\textbf{1}&\textbf{2}&\textbf{3}&\textbf{4}&\te xtbf{5}&\textbf{6}&\textbf{7}&\textbf{8}&\textbf{Numbers of Bags}&\textbf{Numbers of Flights}\\\myhline{0.4mm}1&2&0&0&0&2&1&0&0&766&5\\\hline2&2&0&2&0&2&0&0&0&732&4\\\hline3&0&1&1&1&2&0&0&0&762&4\\\hline4&0&1&0&0&2&1&0&0&735&4\\\hline5&0&1&0&0&2&1&0&0&735&5\\\hline6&2&0&0&0&1&0&0&1&785&5\\\hline7&2&0&0&0&2&0&1&0&795&5\\\hline8&0&1&0&0&2&1&0&0&735&4\\\hline9&2&0&0&0&2&1&0&0&766&5\\\hline10&0&0&0&2&2&0&0&0&758&5\\\hlineTotal&10&4&3&3&19&5&1&1&7569&46\\\myhline{0.4mm}\end{tabular}\label{tab5}\end{minipage}\\[\intextsep]We have produced random value for ${NP}_i$ and $v_{EDS}$. On thiscondition, the number of EDSs is 17, which is less than 29 that wedecide for the airport A. That is to say our solution can meet thereal requirement.\subsubsection{Airport B:}\paragraph{The passenger load is 100{\%}}The checking speed of EDS is 160 bags/hour.\begin{table}[htbp]\centering\caption{}\begin{tabular}{*{11}c}\myhline{0.4mm}$\mathbf{\Deltat(\min)}$&\textbf{2}&\textbf{4}&\textbf{6}&\textbf{8}&\textbf{10}&\textbf{12}&\textbf{14} &\textbf{16}&\textbf{18}&\textbf{20}\\\myhline{0.4mm}$N_{EDS}(\ge)$&33&33&33&33&33&30&27&23&21&19\\\hline$H_{peak}(\min)$&20&40&60&80&100&120&140&160&180&200\\\myhline{0.4mm}\end{tabular}\label{tab6}\end{table}We assume that the suitable value of $H_{peak}$ is 120 minutes.Then the suitable value of $\Delta t$ is about 12 minutes, and。
美赛写作模板及参赛经验分享
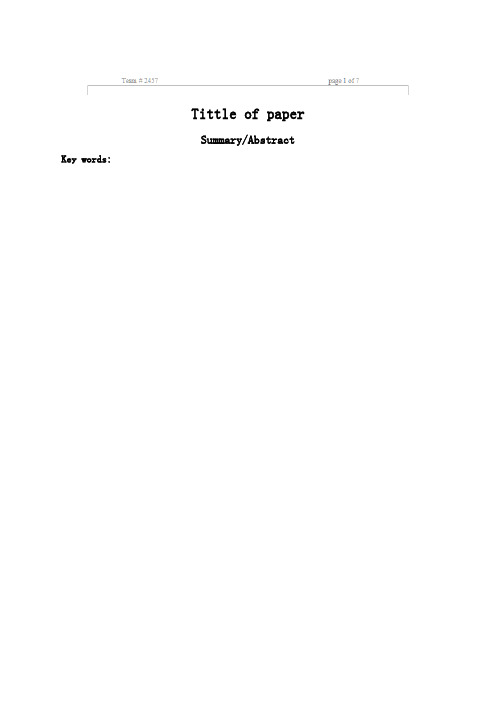
Tittle of paperSummary/Abstract Key words:I.Introduction(引言)Organ transplantation is a preferable treatment for the most serious forms of end-stage diseases. In recent years, advances in medical science and technology have made solid organ transplantation an increasingly successful and common medical procedure, a literal ''second chance at life". Not only does it offer the best hope for complete rehabilitation, but it has also proved to be the most cost-effective of all treatment options, including dialysis. Consequently, more and more people are benefiting from organ transplants and their survival rates are steadily improving. The surgical techniques involved have been mastered for half a century and are now considered as routine. The two main sources of kidneys for transplantation are deceased-donor kidneys and live-donations from family and friends. However, unfortunately, there is a considerable shortage of donor organs, compared to demands. As a matter of fact, efficient matching and allocation of organs donated has become an exigent problem.The United Network for Organ Sharing (UNOS), as the operator of the Organ Procurement and Transplantation Network (OPTN), is responsible for transplant organ distribution in the United States. UNOS oversees the allocation of many different types of transplants,including liver, kidney, pancreas, heart, lung, and cornea.Focusing on kidney transplantation, based on UNOS Kidney Allocation Model, we develop a mathematical model for US transplant networks. First, incoming organs are matched with waiting candidates by medical institutions considering the factors as ABO blood compatibility, the degree of recipient major HLA mismatch in order to obtain a matching degree applied on the allocation part. After that, from the patients’perspective, on the basis of linear regression, priority weight is defined by pondering age, disease severity, time on waiting, PRA level, and region. Applying this mechanism of ranking, we realize MWBM (Maximum Weight Bipartite-graph Matching) and SMGS (Stable Matching based on Gale-Shapley algorithm). MWBM focuses on the optimal assignment of donors following the algorithm of bipartite-graph maximum weight matching; SMGS emphasizes the process of exchanges in order to obtain the stable exchanges between donors and candidates on the waiting list.II.T he Description of Problem(问题重述)III.Basic Assumptions●The level of mismatch is only relative to the number of antigens.●The data and information are accurately registered according tothe medical measures●The data and information are refreshed in time according to thestatus of the patients●No differences in the quality of the donor kidneys●The quality of the donor kidney is constantIV.D efinitions and Notations●Kidney transplantation: A kidney transplant is a surgical procedure to implant a healthykidney into a patient with kidney failure.●Prioritization●MD: Matching Degree●PW: Prioritization weight●MWSM: Maximum Weight Bipartite Matching●SMGS: Stable Matching based on Gale-Shapley algorithm或V.ModelsThrough the investigation of US transplantation network, we draw a general picture of the mechanism. With reference to some resources available on the website of UNOS, a flow chart (Figure 1) is developed showing the procedure of the network.Currently, the initial waiting list is composed of patients whoare waiting for a kidney or combined kidney-pancreas transplant. For the first time, the patients are requested to show the correct and scientific information to the US kidney transplant network which is needed for donor-recipient matching, the ranking of patients on the waiting list, and determining the outcome of those transplanted. The patients’waiting lists are composed of initial patients, historical patients and unsuccessful recipient after transplantation. Historical patients refer to registered patients whose status have changed and have an influence on the procedure.A patient is taken off the waiting list when a graft is offered and accepted by that patient or the patient is dead while waiting for a transplant. Unsuccessful recipients refer to the patients who have a bad result of transplantation calling for transplantation again, as it is so-called relistFigure 1. A schematic depicting the steps occurring in thetransplantation networks......Table 1.Survival rate involving HLA mismatchVI.C onclusions.Our model for the optimal allocation of the donor organs is established by three modules, procurement of MD and PW, optimal assignment by MWBM model and Stable Matching of Gale-Shapley algorithm. The model has offered a convincing procedure of the allocation with the ……VII.Strengths and weaknesses(模型优缺点)Strengths……WeaknessesVIII.References注意文献的积累,不要等到文章写完再去重新寻找文献。
美赛论文模板(中文版)
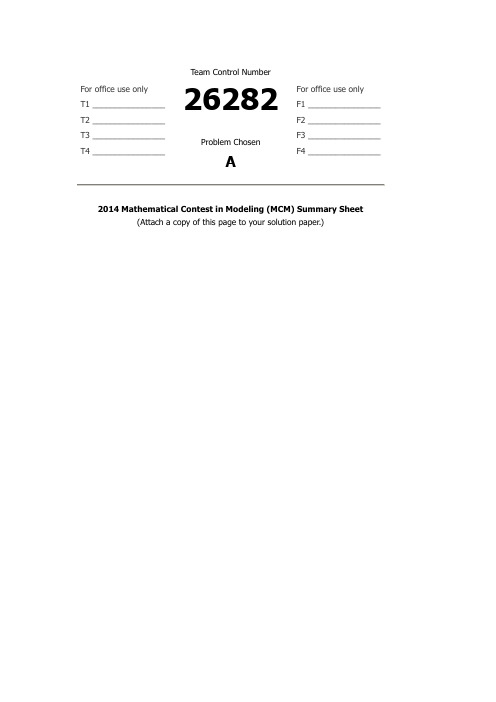
For office use onlyT1________________ T2________________ T3________________ T4________________Team Control Number 26282Problem ChosenAFor office use onlyF1________________F2________________F3________________F4________________2014 Mathematical Contest in Modeling (MCM) Summary Sheet (Attach a copy of this page to your solution paper.)1.Introduction近年来,世界上的交通拥堵问题越来越严重,严重的交通拥堵问题引发了人们的对现行交通规则的思考。
在汽车驾驶规则是右侧的国家多车道高速公路经常遵循除非超车否则靠右行驶的交通规则,那么这个交通规则是否能够对交通拥堵起着什么作用呢?在汽车驾驶规则是右侧的国家多车道高速公路经常遵循以下原则:司机必须在最右侧驾驶,除非他们正在超车,超车时必须先移到左侧车道在超车后再返回。
根据这个规则,在美国单向的3车道高速公路上,最左侧的车道是超车道,这条车道的目的就是超车。
现在我们提出了4个问题:1、什么是低负荷和高负荷,如何界定他们?2、这条规则在提升车流量的方面是否有效?3、这条规则在安全问题上所起的作用?4、这条规则对速度的限制?1.1 Survey of Previous Research1.2 Restatement of the problem本题需要我们建立一个数学模型对这个规则进行评价。
我们需要解决的问题如下:●什么是低负荷和高负荷,如何界定他们?●这条规则在提升车流量的方面是否有效?●这条规则在安全问题上所起的作用?●这条规则对速度的限制?●对于靠左行的规则,该模型能否可以使用??(待定)●如果交通运输完全在智能系统的控制下,会怎样影响建立的模型?针对以上问题,我们的解题思路和方法如下所示:◆我们根据交通密度对低负荷和高负荷进行界定,交通密度是指:在某时刻,每单位道路长度内一条道路的车辆数。
建模美赛获奖范文
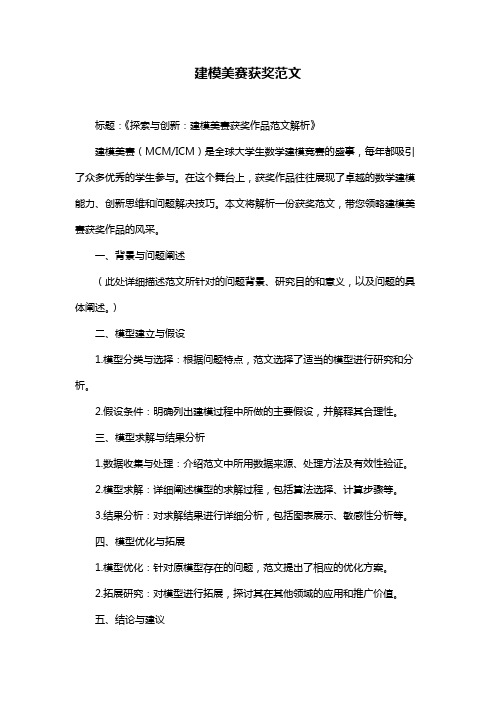
建模美赛获奖范文标题:《探索与创新:建模美赛获奖作品范文解析》建模美赛(MCM/ICM)是全球大学生数学建模竞赛的盛事,每年都吸引了众多优秀的学生参与。
在这个舞台上,获奖作品往往展现了卓越的数学建模能力、创新思维和问题解决技巧。
本文将解析一份获奖范文,带您领略建模美赛获奖作品的风采。
一、背景与问题阐述(此处详细描述范文所针对的问题背景、研究目的和意义,以及问题的具体阐述。
)二、模型建立与假设1.模型分类与选择:根据问题特点,范文选择了适当的模型进行研究和分析。
2.假设条件:明确列出建模过程中所做的主要假设,并解释其合理性。
三、模型求解与结果分析1.数据收集与处理:介绍范文中所用数据来源、处理方法及有效性验证。
2.模型求解:详细阐述模型的求解过程,包括算法选择、计算步骤等。
3.结果分析:对求解结果进行详细分析,包括图表展示、敏感性分析等。
四、模型优化与拓展1.模型优化:针对原模型存在的问题,范文提出了相应的优化方案。
2.拓展研究:对模型进行拓展,探讨其在其他领域的应用和推广价值。
五、结论与建议1.结论总结:概括范文的研究成果,强调其创新点和贡献。
2.实践意义:分析建模结果在实际问题中的应用价值和意义。
3.建议:针对问题解决,提出具体的建议和措施。
六、获奖亮点与启示1.创新思维:范文在模型选择、求解方法等方面展现出创新性。
2.严谨论证:文章结构清晰,逻辑严密,数据充分,论证有力。
3.团队合作:建模美赛强调团队协作,范文体现了成员间的紧密配合和分工合作。
总结:通过分析这份建模美赛获奖范文,我们可以学到如何从问题背景出发,建立合理的模型,进行严谨的求解和分析,以及如何优化和拓展模型。
同时,也要注重创新思维和团队合作,才能在建模美赛中脱颖而出。
MCM北美数学建模论文模版
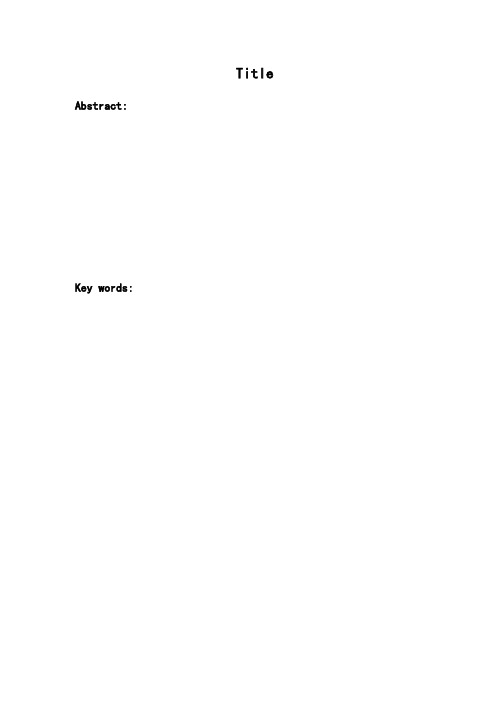
Title Abstract:Key words:Contents1. Introduction............................................................................ .. (3)1.1 Why does toll way collects toll? (3)1.2 Toll modes (3)1.3 Toll collection methods (3)1.4 Annoyance in toll plazas (3)1.5 The origin of the toll way problem (3)1.6 Queuing theory (4)2. The Description of Problem................................................................................... .. (5)2.1 How do we approximate the whole course of payingtoll? (5)2.2 How do we define the optimalconfiguration? (5)2.2.1 From the perspective of motorist (5)2.2.2 From the perspective of the toll plaza (6)2.2.3 Compromise (6)2.3 Overall optimization and local optimization (6)2.4 The differences in weights and sizes of vehicles (7)2.5 What if there is no dataavailable? ............................................................................. .. (7)3. Models......................................................................................... .. (7)3.1 Basic Model.................................................................................. .. (7)3.1.1 Symbols andDefinitions (7)3.1.2 Assumptions (8)3.1.3 The Foundation of Model (9)3.1.4 Solution and Result (11)3.1.5 Analysis of the Result (11)3.1.6 Strength and Weakness (13)3.2 Improved Model.............................................................. .. (14)3.2.1 Extra Symbols (14)3.2.2 Additional Assumptions (14)3.2.3 The Foundation of Model (14)3.2.4 Solution and Result (15)3.2.5 Analysis of the Result (18)3.2.6 Strength and Weakness (19)4. Conclusions............................................................................. (19)4.1 Conclusions of the problem (19)4.2 Methods used in our models (19)4.3 Application of our models (19)5. Future Work...................................................................................... .. (19)5.1 Another model………………………………………………………………………………………195.2 Another layout of toll plaza (23)5.3 The newly- adopted charging methods (23)6. References.............................................................................. (23)7. Appendix................................................................................. (23)Programs and codes………………………………………………………………..……………………24I. IntroductionIn order to indicate the origin of the toll way problems, the following background is worth mentioning.1.11.21.31.41.51.6II. The Description of the Problem2.1 How do we approximate the whole course of paying toll?●●●●2.2 How do we define the optimal configuration?1) From the perspective of motorist:2) From the perspective of the toll plaza:3) Compromise:2.3 The local optimization and the overall optimization●●●Virtually:2.4 The differences in weights and sizes of vehicles2.5 What if there is no data available?III. Models3.1 Basic Model3.1.1 Terms, Definitions and SymbolsThe signs and definitions are mostly generated from queuing theory.●●●●●3.1.2 Assumptions●●●●●3.1.3 The Foundation of Model1) The utility function●The cost of toll plaza:●The loss of motorist:●The weight of each aspect:●Compromise:2) The integer programmingAccording to queuing theory, we can calculate the statistical properties as follows.3)The overall optimization and the local optimization●The overall optimization:●The local optimization:●The optimal number of tollbooths:3.1.4 Solution and Result1) The solution of the integer programming:2) Results:3.1.5 Analysis of the Result●Local optimization and overall optimization:●Sensitivity: The result is quite sensitive to the change of thethree parameters●Trend:●Comparison:3.1.6 Strength and Weakness●Strength: In despite of this, the model has proved that .Moreover, we have drawn some useful conclusions about . T hemodel is fit for, such as●Weakness: This model just applies to . As we have stated, .That’s just what we should do in the improved model.3.2 Improved Model3.2.1 Extra SymbolsSigns and definitions indicated above are still valid. Here are some extra signs and definitions.●●●●3.2.2 Additional Assumptions●●●Assumptions concerning the anterior process are the same as the Basic Model.3.2.3 The Foundation of Model1) How do we determine the optimal number?As we have concluded from the Basic Model,3.2.4 Solution and Result1) Simulation algorithmBased on the analysis above, we design our simulation arithmetic as follows.●Step1:●Step2:●Step3:●Step4:●Step5:●Step6:●Step7:●Step8:●Step9:2) Flow chartThe figure below is the flow chart of the simulation.3) Solution3.2.5 Analysis of the Result3.2.6 Strength and Weakness●Strength: The Improved Model aims to make up for the neglectof . The result seems to declare that this model is more reasonable than the Basic Model and much more effective than the existing design.●Weakness: . Thus the model is still an approximate on a largescale. This has doomed to limit the applications of it.IV. Conclusions4.1 Conclusions of the problem●●●4.2 Methods used in our models●●●4.3 Applications of our models●●●V. Future Work 5.1 Another model5.1.1The limitations of queuing theory 5.1.25.1.35.1.41)●●●●2)●●●3)●●●4)标准实用5.2 Another layout of toll plaza5.3 The newly- adopted charging methodsVI. References[1][2][3][4][5]VII. Appendix文案大全。