数学建模及其论文写作原版-2016
2016年全国数学建模A题论文设计(最终版)
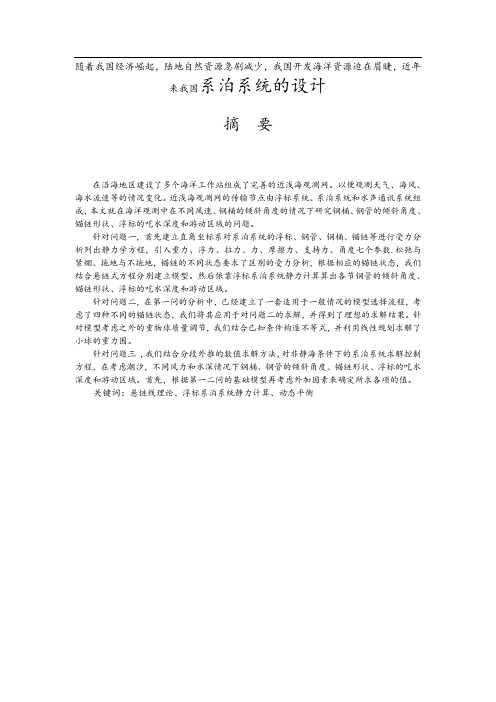
随着我国经济崛起,陆地自然资源急剧减少,我国开发海洋资源迫在眉睫,近年来我国系泊系统的设计摘要在沿海地区建设了多个海洋工作站组成了完善的近浅海观测网。
以便观测天气、海风、海水流速等的情况变化。
近浅海观测网的传输节点由浮标系统、系泊系统和水声通讯系统组成,本文就在海洋观测中在不同风速、钢桶的倾斜角度的情况下研究钢桶、钢管的倾斜角度、锚链形状、浮标的吃水深度和游动区域的问题。
针对问题一,首先建立直角坐标系对系泊系统的浮标、钢管、钢桶、锚链等进行受力分析列出静力学方程,引入重力、浮力、拉力、力、摩擦力、支持力、角度七个参数.松弛与紧绷、拖地与不拖地,锚链的不同状态要求了区别的受力分析,根据相应的锚链状态,我们结合悬链式方程分别建立模型。
然后依靠浮标系泊系统静力计算算出各节钢管的倾斜角度、锚链形状、浮标的吃水深度和游动区域。
针对问题二,在第一问的分析中,已经建立了一套适用于一般情况的模型选择流程,考虑了四种不同的锚链状态,我们将其应用于对问题二的求解,并得到了理想的求解结果。
针对模型考虑之外的重物球质量调节,我们结合已知条件构造不等式,并利用线性规划求解了小球的重力围。
针对问题三 ,我们结合分段外推的数值求解方法,对非静海条件下的系泊系统求解控制方程,在考虑潮汐,不同风力和水深情况下钢桶、钢管的倾斜角度、锚链形状、浮标的吃水深度和游动区域。
首先,根据第一二问的基础模型再考虑外加因素来确定所求各项的值。
关键词:悬链线理论、浮标系泊系统静力计算、动态平衡一、问题重述1.1问题背景向海洋进军,利用开发海洋资源已经成为扩展人类生存资源,提高资源储备的主要方式。
随着人们对大海的研究越来越深刻,在近浅海海域人们需要实时观测天气、海风、海水流速等的情况变化。
这就需要人们建立大量的观测站,而这些观测站的传输节点是由浮标系统、系泊系统和水声通讯系统组成。
其中,系泊系统则是整个传输节点的关键。
1.2问题提出在设计系泊系统时,要求锚链末端与锚的连接处的切线方向和海平面的夹角不超过16度,以保证锚不会被拖行。
2016年全国大学生数学建模竞赛获奖论文
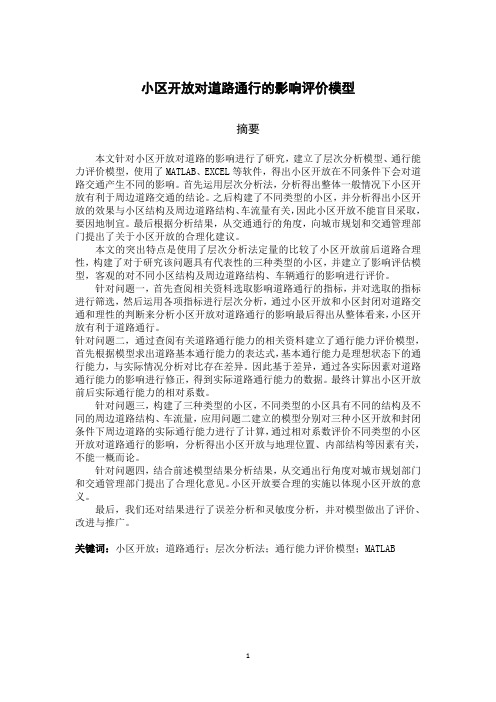
小区开放对道路通行的影响评价模型摘要本文针对小区开放对道路的影响进行了研究,建立了层次分析模型、通行能力评价模型,使用了MATLAB、EXCEL等软件,得出小区开放在不同条件下会对道路交通产生不同的影响。
首先运用层次分析法,分析得出整体一般情况下小区开放有利于周边道路交通的结论。
之后构建了不同类型的小区,并分析得出小区开放的效果与小区结构及周边道路结构、车流量有关,因此小区开放不能盲目采取,要因地制宜。
最后根据分析结果,从交通通行的角度,向城市规划和交通管理部门提出了关于小区开放的合理化建议。
本文的突出特点是使用了层次分析法定量的比较了小区开放前后道路合理性,构建了对于研究该问题具有代表性的三种类型的小区,并建立了影响评估模型,客观的对不同小区结构及周边道路结构、车辆通行的影响进行评价。
针对问题一,首先查阅相关资料选取影响道路通行的指标,并对选取的指标进行筛选,然后运用各项指标进行层次分析,通过小区开放和小区封闭对道路交通和理性的判断来分析小区开放对道路通行的影响最后得出从整体看来,小区开放有利于道路通行。
针对问题二,通过查阅有关道路通行能力的相关资料建立了通行能力评价模型,首先根据模型求出道路基本通行能力的表达式,基本通行能力是理想状态下的通行能力,与实际情况分析对比存在差异。
因此基于差异,通过各实际因素对道路通行能力的影响进行修正,得到实际道路通行能力的数据。
最终计算出小区开放前后实际通行能力的相对系数。
针对问题三,构建了三种类型的小区,不同类型的小区具有不同的结构及不同的周边道路结构、车流量,应用问题二建立的模型分别对三种小区开放和封闭条件下周边道路的实际通行能力进行了计算,通过相对系数评价不同类型的小区开放对道路通行的影响,分析得出小区开放与地理位置、内部结构等因素有关,不能一概而论。
针对问题四,结合前述模型结果分析结果,从交通出行角度对城市规划部门和交通管理部门提出了合理化意见。
小区开放要合理的实施以体现小区开放的意义。
优秀的数学建模论文范文(通用8篇)
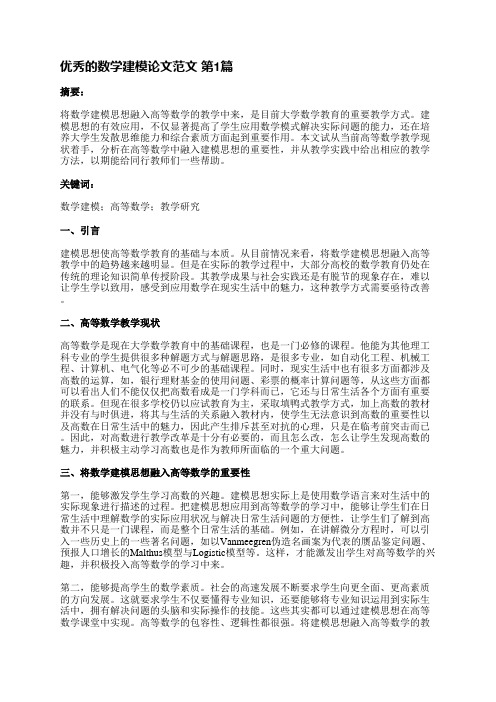
优秀的数学建模论文范文第1篇摘要:将数学建模思想融入高等数学的教学中来,是目前大学数学教育的重要教学方式。
建模思想的有效应用,不仅显著提高了学生应用数学模式解决实际问题的能力,还在培养大学生发散思维能力和综合素质方面起到重要作用。
本文试从当前高等数学教学现状着手,分析在高等数学中融入建模思想的重要性,并从教学实践中给出相应的教学方法,以期能给同行教师们一些帮助。
关键词:数学建模;高等数学;教学研究一、引言建模思想使高等数学教育的基础与本质。
从目前情况来看,将数学建模思想融入高等教学中的趋势越来越明显。
但是在实际的教学过程中,大部分高校的数学教育仍处在传统的理论知识简单传授阶段。
其教学成果与社会实践还是有脱节的现象存在,难以让学生学以致用,感受到应用数学在现实生活中的魅力,这种教学方式需要亟待改善。
二、高等数学教学现状高等数学是现在大学数学教育中的基础课程,也是一门必修的课程。
他能为其他理工科专业的学生提供很多种解题方式与解题思路,是很多专业,如自动化工程、机械工程、计算机、电气化等必不可少的基础课程。
同时,现实生活中也有很多方面都涉及高数的运算,如,银行理财基金的使用问题、彩票的概率计算问题等,从这些方面都可以看出人们不能仅仅把高数看成是一门学科而已,它还与日常生活各个方面有重要的联系。
但现在很多学校仍以应试教育为主,采取填鸭式教学方式,加上高数的教材并没有与时俱进,将其与生活的关系融入教材内,使学生无法意识到高数的重要性以及高数在日常生活中的魅力,因此产生排斥甚至对抗的心理,只是在临考前突击而已。
因此,对高数进行教学改革是十分有必要的,而且怎么改,怎么让学生发现高数的魅力,并积极主动学习高数也是作为教师所面临的一个重大问题。
三、将数学建模思想融入高等数学的重要性第一,能够激发学生学习高数的兴趣。
建模思想实际上是使用数学语言来对生活中的实际现象进行描述的过程。
把建模思想应用到高等数学的学习中,能够让学生们在日常生活中理解数学的实际应用状况与解决日常生活问题的方便性,让学生们了解到高数并不只是一门课程,而是整个日常生活的基础。
2016年数学建模竞赛A题优秀论文

(5-2-4)
5
(二)钢管的受力
图 5.2.2 钢管受力示意图
钢管 Pi ( 2 i 5 )受力如图 5.2.2 所示,首先对于底面直径为 d i ,轴向高度为 li 的 圆柱形钢管的浮力由阿基米德定律有Ti g di 4li4
(5-2-5)
物体静止不发生移动由牛顿第一定律有:
F0 0.625 S1v 2 S1 (l1 h)d1
(5-2-2)
其中 S1 为浮标在风向法平面的投影面积, l1 为浮标高度。 浮标下表面与第一节钢管铰接,钢管对浮标作用力的大小用 F2,1 表示,其与竖直方 向的夹角为 1 。此外,物体还受到竖直向下的重力 G1 。物体受力平衡根据牛顿第一定律 有浮标在 x, y 方向的合力为零,即:
(5-2-7)
05-2-8) (
对上式进行分离变量得到钢管倾斜角 i 关于上端点作用力的递推关系式:
i a r c t a n
(三)钢桶的受力
Fi 1 ,is i n i
1 i
0.5 T( i Gi ) F 1 i ,
c o si1
(5-2-9)
如图 5.2.3 所示,钢桶静止时共受到 6 个外力作用,其倾斜角度(与竖直方向夹角) 为 6 ,其上端与钢管 P5 铰接,钢管对钢桶作用力大小为 F5,6 ,倾角为 5 ;下端与锚链链 环 P8 铰接并悬挂一重物球,链环对钢管作用力大小为 F8,6 ,倾角为 6 。
i 1 F i 1 ,i s i n i 0 Fi 1 ,i s i n i1 G i F i1 , ic o s i1 , ic o s Ti F
i
0
(5-2-6)
2016年全国大学生数学建模竞赛获奖论文
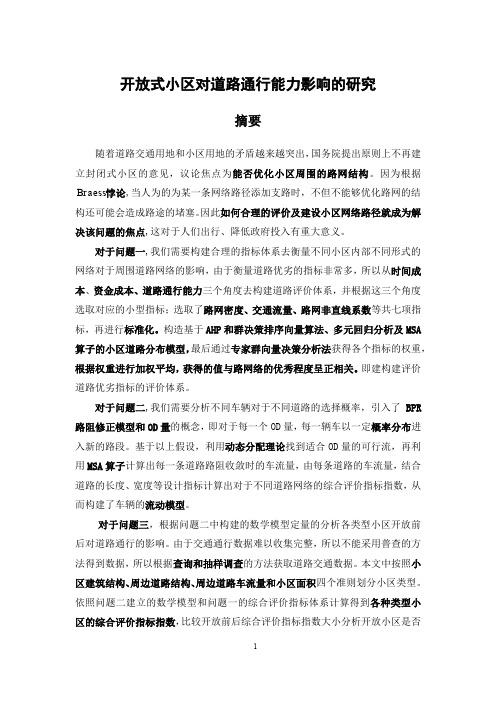
1.2 要解决的问题
针对题目所提出的要求,本文主要关注以下问题: 首先,针对“请选取合适的评价指标体系,用以评价小区开放对周边道路通 行的影响”问题,如何挑选出若干个有效的相关指标,作为道路通行情况的不同 属性, 采用可行的赋权方法为这些指标分别赋予权重,最后将这些指标加权汇总 为一个综合指标, 从而产生一个完整的评价指标体系,用以评价小区开放与否对 周边道路通行情况产生的影响。 其次, 如何尽量模拟真实交通环境, 充分考虑各种影响道路通车情况的因素,
设计通行能力是固定的,则高峰时段实际单位时 间内交通量越大道路越拥堵。一般在 0.5-0.7 比较合
起点与终点固定后,人类心理趋向是选择实际行 驶道路长度最短的路径,而当道路拥堵时,人们则会 绕路行驶,选择车流量较少的路径,则路网非直线系 数增大。所以路网非直线系数越大道路越拥堵。
M4
路网密度 M 5
M1
直观反映道路通行能力以及道路的实时路况,当 交通量超过某一数值时,则认为发生拥堵
M2
行驶总距离一定时,行程车速与行驶总时间成反 比关系,行驶总时间包括无障碍行驶时间、路阻时间
7
(km/h)
和交叉路口延误时间。所以当道路拥堵时,路阻时间 和交叉口延误时间增长,则行程车速降低。
饱和度 M 3 适。 路网非直线系数
M6
交通运行指数
交通状况越拥堵行程车速越小,拥堵里程比例越 大,则交通运行指数越大,得到拥堵等级越高。
sumc
h1 suml h2
mjl mjs
m n
1
T
4
xn
MSA 算法中 n 次循环后各个路段 分配的流量集合
d1
交叉口平均延误时间
四、模型的建立与分析 4.1 问题一综合评价指标体系的建立
2016数学建模国赛B题湖北赛区省一等奖论文_图文
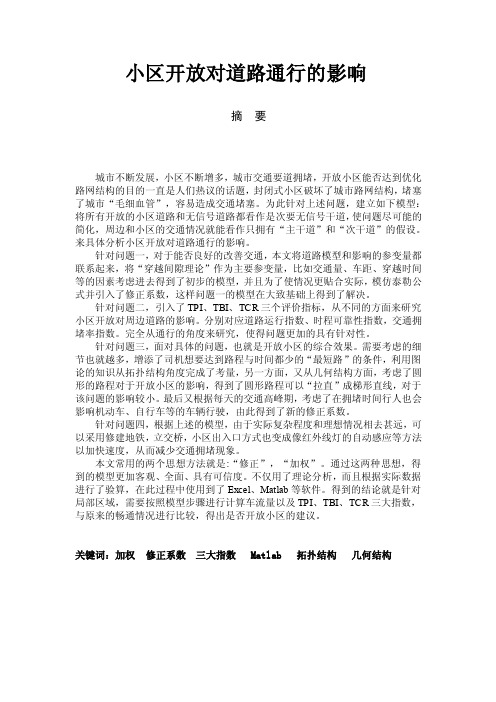
小区开放对道路通行的影响摘要城市不断发展,小区不断增多,城市交通要道拥堵,开放小区能否达到优化路网结构的目的一直是人们热议的话题,封闭式小区破坏了城市路网结构,堵塞了城市“毛细血管”,容易造成交通堵塞。
为此针对上述问题,建立如下模型:将所有开放的小区道路和无信号道路都看作是次要无信号干道,使问题尽可能的简化,周边和小区的交通情况就能看作只拥有“主干道”和“次干道”的假设。
来具体分析小区开放对道路通行的影响。
针对问题一,对于能否良好的改善交通,本文将道路模型和影响的参变量都联系起来,将“穿越间隙理论”作为主要参变量,比如交通量、车距、穿越时间等的因素考虑进去得到了初步的模型,并且为了使情况更贴合实际,模仿泰勒公式并引入了修正系数,这样问题一的模型在大致基础上得到了解决。
针对问题二,引入了TPI、TBI、TCR三个评价指标,从不同的方面来研究小区开放对周边道路的影响。
分别对应道路运行指数、时程可靠性指数,交通拥堵率指数。
完全从通行的角度来研究,使得问题更加的具有针对性。
针对问题三,面对具体的问题,也就是开放小区的综合效果。
需要考虑的细节也就越多,增添了司机想要达到路程与时间都少的“最短路”的条件,利用图论的知识从拓扑结构角度完成了考量,另一方面,又从几何结构方面,考虑了圆形的路程对于开放小区的影响,得到了圆形路程可以“拉直”成梯形直线,对于该问题的影响较小。
最后又根据每天的交通高峰期,考虑了在拥堵时间行人也会影响机动车、自行车等的车辆行驶,由此得到了新的修正系数。
针对问题四,根据上述的模型,由于实际复杂程度和理想情况相去甚远,可以采用修建地铁,立交桥,小区出入口方式也变成像红外线灯的自动感应等方法以加快速度,从而减少交通拥堵现象。
本文常用的两个思想方法就是:“修正”,“加权”。
通过这两种思想,得到的模型更加客观、全面、具有可信度。
不仅用了理论分析,而且根据实际数据进行了验算,在此过程中使用到了Excel、Matlab等软件。
2016年数学建模竞赛A题优秀论文
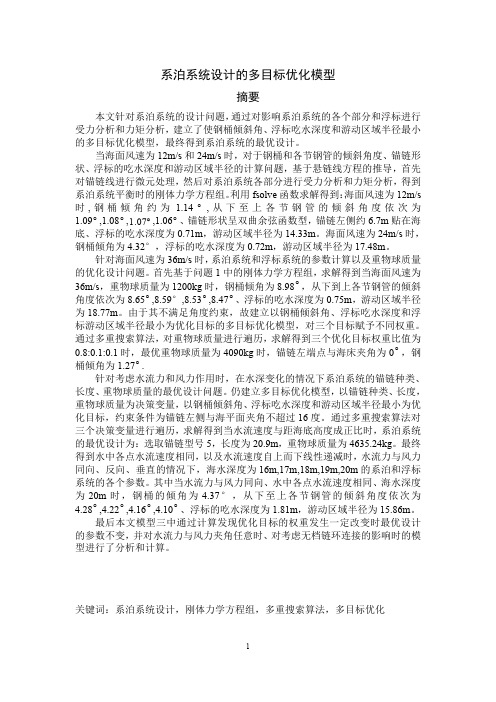
将(1)式代入得:
dy mg T1 sin 1
dx
T1 cos1
(2)
对于锚链,m=σs ,其中 s 是 AB 锚链的长度,σ是锚链的线密度,即单位长
度锚链的质量[1]。代入(2)式得:
dy sg T1 sin 1
(3)
dx
T1 cos1
根据勾股定理可以得到弧长公式:
ds
1
dy dx
dp dx
T1
cos1
g
1 p2
然后对 x 和 p 分离变量并对两端进行积分得到:
dp
1 p2
T1
g cos 1
dx
即:sinh 1
p
g T1
x
C1
(4)
其中 C1 可以由 x=0,y=0 时的值确定,原点 A 处 p y ' tan 1 ,可得 C1 为:
当海面风速一定且海水静止时,钢桶和各节钢管的倾斜角度、锚链形状、浮 标的吃水深度和游动区域,与锚链线的方程、系泊系统各部分之间的受力平衡和 力矩平衡的约束密切相关。由于传输节点各部分相互影响,根据力学相关知识, 可以按照锚链→钢桶和重物球→钢管→浮标的顺序依次进行受力分析,从而得到 各部分受力平衡时的定量解析式,通过这些表达式可以确定钢桶和各节钢管的倾 斜角度、锚链形状。由于吃水深度与浮标受力直接相关,还可以确定浮标的吃水 深度。对于浮标的游动区域,可以由稳定后系泊系统各个部分在水平方向投影的 总长度来计算游动区域的最大半径。
2016年大学生数学建模论文

2016年大学生数学建模论文数学建模就是通过计算得到的结果来解释实际问题,并接受实际的检验,来建立数学模型的全过程。
下文是店铺为大家整理的关于2016年大学生数学建模论文的范文,欢迎大家阅读参考!2016年大学生数学建模论文篇1试论数学建模方法目前数学教学与数学应用脱节的现象很突出,以至于学生认为学习数学没用,对数学学习失去兴趣,如何改变目前这种教学与应用脱节的现象,笔者认为,可以用数学模型法指导数学应用题教学,为学生用数学来解决问题提供经验和范式,从而探索出一条行之有效的教学途径。
一、什么是数学模型要突出应用,就应站在数学模型法的高度来认识并实施应用题教学。
什么是数学模型法?数学模型法就是把实际问题加以抽象概括,建立相应的数学模型,利用这些模型来研究实际问题的一般数学方法。
教师在应用题教学中要渗透这种方法和思想,要注重并强调如何从实际问题中发现并抽象出数学问题,如何用数学模型(包括数学概念、公式、方程、不等式函数等)来表达实际问题,如何用数学模型的解来解释实际问题的解。
以及为科学决策提供可信的依据并预测其发展趋势。
二、建模示范方法例谈在教学中我根据教学内容,选编一些应用问题进行例题教学,引导学生分析联想、抽象建模,培养学生的建模能力,提供经验和范式。
选编数学应用性例题的一般原则是:① 必须与教学内容密切联系;② 必须与学生的知识水平相适应;③ 必须符合科学性和趣味性;④ 取材应尽量涉及目前社会的热点问题,有时代气息,有教育价值。
1. 与其他相关学科有关的问题题1:化学中甲烷CH4的键角109°28′是怎样求出来的?题2:在大楼底层有一控制室,有三条导线和楼上某电器相连,设三连导线的电阻分别为x、y、z,现手头有一只电表可在控制室内测量电阻,试没计一种数学方法求这三根导线的电阻。
2. 发生在学生身边的数学问题题3:学校教学大楼,从一楼到二楼共13个台阶。
一位同学上楼梯可以一步上一个台阶,也可以一步上两个台阶。
2016年美国大学生数学建模E题中文版论文

2016年美国大学生数学建模E 题中文版论文摘要:本文建立了一个模型评定一个国家或地区提供清洁水的能力,找出水资源缺乏的原因,具体任务如下:任务一:建立一个模型,模型中考虑一个国家或地区的供水量由地表水量、地下水量、污水净化量提供,需水量由生活用水量、农业用水量、工业用水量提供。
在供水量中,地表水量受年均气温、年均降水量和森林覆盖率影响,地下水受年均气温、年均降水量影响;农业用水量受总人口数量和年均降水量影响,工业用水量与该地区的生产总值有关;运用多元非线性回归统计原理求出回归系数,确定其函数表达式,以供水量和需水量的比值作为衡量一个地区提供清洁水能力的强弱,通过比较清洁水供求量的比值与1的大小来反映能力的强弱。
任务二,该模型选取了中国山东省作为测试地区,对中国山东地区2005—2014年的统计数据进行分析,通过函数拟合和多元非线性回归的思想将模型具体化。
经过模型判断山东省提供清洁水的能力是为弱,接着从物理短缺和经济短缺两个方面分析中国山东缺水的原因,从而检验模型的可适用性。
对于任务三,本模型选取了几个影响供水量和需水量有代表性的因素即年降水量、年均气温、森林覆盖率、人口总数进行预测。
分别对中国山东省2005—2014这10年中各个因素与年份的数据进行分析,根据目前数据的发展趋势预测出未来15年这些因素的化,用本模型对山东省未来15年水资源的状况进行分析评价。
对于任务四,根据任务一中的模型,任务二的分析,找到影响中国山东省提供清洁水能力的主要因素,根据这些因素本文提出并制定来了相应的干预计划。
如针对年均降水量,提出了通过人工降雨来增加年均降水量;针对森林覆盖率,提出了植树造林、保护植被;针对污水净化量,提出了提高污水处理技术,来提高污水转化率和增加日处理污水量;针对人口总量,提出坚持实行计划生育政策;针对人均用水量,提出了规定每人每日用水量,并提出工业污水达到规定的指标后才能排放,研发海水淡化技术等计划,来增加清洁水的供应量。
2016年数学建模国赛A题一等奖论文

3
���������′���
表示钢桶到锚间第������个锚链与轴正向的夹角
������������������
表示钢管到钢桶间第������个零件对它上端第������ − 1个零件在������轴负 向的拉力
������������������
表示钢管到钢桶间第������个零件对它上端第������ − 1个零件在������轴负 向的拉力
三、模型假设
1. 浮标竖直漂浮在海面上不发生倾斜; 2. 海面上没有波浪不产生波生力; 3. 锚链在拉力作用下不伸长; 4. 锚链的体积很小可以忽略; 5. 重物球和锚链由铸钢材料构成; 6. 只有钢管、钢桶、锚链的侧面面积在水流速度法平面上产生投影; 7. 锚在海床上具有抓地力,不发生水平方向上的移动。
系泊系统的设计问题就是确定锚链的型号、长度和重物球的质量,使得浮标 的吃水深度和游动区域及钢桶的倾斜角度尽可能小。
1.2 问题提出
问题 1 某型传输节点选用Ⅱ型电焊锚链 22.05m,选用的重物球的质量为 1200kg 。 现 将 该 型 传 输 节 点 布 放 在 水 深 18m 、 海 床 平 坦 、 海 水 密 度 为 1.025×103kg/m3 的海域。若海水静止,分别计算海面风速为 12m/s 和 24m/s 时钢 桶和各节钢管的倾斜角度、锚链形状、浮标的吃水深度和游动区域。
二、问题分析
2.1 问题一分析
问题一作为整个问题的关键,需分析整个系泊系统在不同情况下全部变量间 关系进而建立相关模型。我们从整个系统最终的稳定状态开始分析,由上而下先 以上部钢管作为研究对象,利用理论力学的相关知识,建立稳定状态下的分力平 衡方程和力矩平衡方程,进而解得钢管倾斜角及下段钢管的相互作用拉力。继续 分析下段钢管,建立力与力矩的平衡方程,求解倾角和相互拉力,重复迭代计算 可得海床底部末节锚链的倾斜角。利用上述计算得到的各单元倾斜角度,可以得 出整个系统的垂悬高度,至此建立了适应本题的代数迭代模型,利用粒子群算法 优化上部浮标的吃水深度,进而改变倾斜角及相互拉力,最终迭代计算到满足题 目条件的吃水深度,最后计算了该吃水深下,不同海面风速时的钢桶和各节钢管
2016美国大学生数学建模大赛C题特等奖(原版论文)C42939Tsinghua University, China
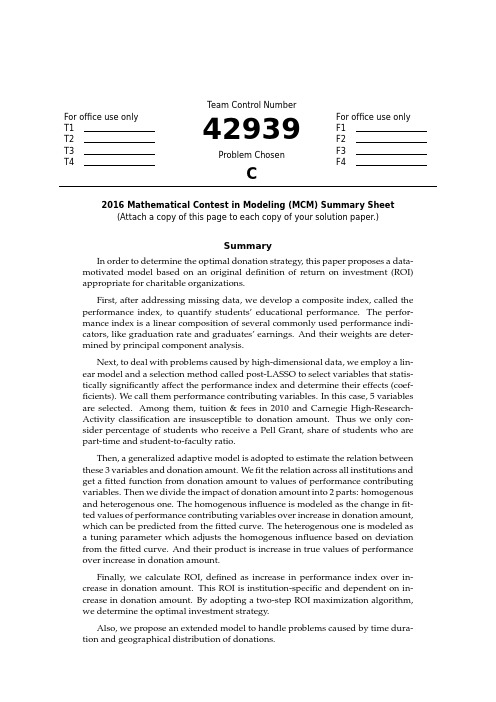
For office use only T1T2T3T4T eam Control Number42939Problem ChosenCFor office use onlyF1F2F3F42016Mathematical Contest in Modeling(MCM)Summary Sheet (Attach a copy of this page to each copy of your solution paper.)SummaryIn order to determine the optimal donation strategy,this paper proposes a data-motivated model based on an original definition of return on investment(ROI) appropriate for charitable organizations.First,after addressing missing data,we develop a composite index,called the performance index,to quantify students’educational performance.The perfor-mance index is a linear composition of several commonly used performance indi-cators,like graduation rate and graduates’earnings.And their weights are deter-mined by principal component analysis.Next,to deal with problems caused by high-dimensional data,we employ a lin-ear model and a selection method called post-LASSO to select variables that statis-tically significantly affect the performance index and determine their effects(coef-ficients).We call them performance contributing variables.In this case,5variables are selected.Among them,tuition&fees in2010and Carnegie High-Research-Activity classification are insusceptible to donation amount.Thus we only con-sider percentage of students who receive a Pell Grant,share of students who are part-time and student-to-faculty ratio.Then,a generalized adaptive model is adopted to estimate the relation between these3variables and donation amount.Wefit the relation across all institutions and get afitted function from donation amount to values of performance contributing variables.Then we divide the impact of donation amount into2parts:homogenous and heterogenous one.The homogenous influence is modeled as the change infit-ted values of performance contributing variables over increase in donation amount, which can be predicted from thefitted curve.The heterogenous one is modeled as a tuning parameter which adjusts the homogenous influence based on deviation from thefitted curve.And their product is increase in true values of performance over increase in donation amount.Finally,we calculate ROI,defined as increase in performance index over in-crease in donation amount.This ROI is institution-specific and dependent on in-crease in donation amount.By adopting a two-step ROI maximization algorithm, we determine the optimal investment strategy.Also,we propose an extended model to handle problems caused by time dura-tion and geographical distribution of donations.A Letter to the CFO of the Goodgrant FoundationDear Chiang,Our team has proposed a performance index quantifying the students’educational per-formance of each institution and defined the return of investment(ROI)appropriately for a charitable organization like Goodgrant Foundation.A mathematical model is built to help predict the return of investment after identifying the mechanism through which the donation generates its impact on the performance.The optimal investment strategy is determined by maximizing the estimated return of investment.More specifically,the composite performance index is developed after taking all the pos-sible performance indicators into consideration,like graduation rate and graduates’earnings. The performance index is constructed to represents the performance of the school as well as the positive effect that a college brings to students and the community.From this point of view, our definition manages to capture social benefits of donation.And then we adopt a variable selection method tofind out performance contributing vari-ables,which are variables that strongly affect the performance index.Among all the perfor-mance contributing variables we select,three variables which can be directly affected by your generous donation are kept to predict ROI:percentage of students who receive a Pell Grant, share of students who are part-time and student-to-faculty ratio.Wefitted a relation between these three variables and the donation amount to predict change in value of each performance contributing variable over your donation amount.And we calculate ROI,defined as increase in the performance index over your donation amount, by multiplying change in value of each performance contributing variable over your donation amount and each performance contributing variable’s effect on performance index,and then summing up the products of all performance contributing variables.The optimal investment strategy is decided after maximizing the return of investment according to an algorithm for selection.In conclusion,our model successfully produced an investment strategy including a list of target institutions and investment amount for each institution.(The list of year1is attached at the end of the letter).The time duration for the investment could also be determined based on our model.Since the model as well as the evaluation approach is fully data-motivated with no arbitrary criterion included,it is rather adaptable for solving future philanthropic educational investment problems.We have a strong belief that our model can effectively enhance the efficiency of philan-thropic educational investment and provides an appropriate as well as feasible way to best improve the educational performance of students.UNITID names ROI donation 197027United States Merchant Marine Academy21.85%2500000 102711AVTEC-Alaska’s Institute of Technology21.26%7500000 187745Institute of American Indian and Alaska Native Culture20.99%2000000 262129New College of Florida20.69%6500000 216296Thaddeus Stevens College of Technology20.66%3000000 229832Western Texas College20.26%10000000 196158SUNY at Fredonia20.24%5500000 234155Virginia State University20.04%10000000 196200SUNY College at Potsdam19.75%5000000 178615Truman State University19.60%3000000 199120University of North Carolina at Chapel Hill19.51%3000000 101648Marion Military Institute19.48%2500000187912New Mexico Military Institute19.31%500000 227386Panola College19.28%10000000 434584Ilisagvik College19.19%4500000 199184University of North Carolina School of the Arts19.15%500000 413802East San Gabriel Valley Regional Occupational Program19.09%6000000 174251University of Minnesota-Morris19.09%8000000 159391Louisiana State University and Agricultural&Mechanical Col-19.07%8500000lege403487Wabash Valley College19.05%1500000 Yours Sincerely,Team#42939An Optimal Strategy of Donation for Educational PurposeControl Number:#42939February,2016Contents1Introduction51.1Statement of the Problem (5)1.2Baseline Model (5)1.3Detailed Definitions&Assumptions (8)1.3.1Detailed Definitions: (8)1.3.2Assumptions: (9)1.4The Advantages of Our Model (9)2Addressing the Missing Values93Determining the Performance Index103.1Performance Indicators (10)3.2Performance Index via Principal-Component Factors (10)4Identifying Performance Contributing Variables via post-LASSO115Determining Investment Strategy based on ROI135.1Fitted Curve between Performance Contributing Variables and Donation Amount145.2ROI(Return on Investment) (15)5.2.1Model of Fitted ROIs of Performance Contributing Variables fROI i (15)5.2.2Model of the tuning parameter P i (16)5.2.3Calculation of ROI (17)5.3School Selection&Investment Strategy (18)6Extended Model186.1Time Duration (18)6.2Geographical Distribution (22)7Conclusions and Discussion22 8Reference23 9Appendix241Introduction1.1Statement of the ProblemThere exists no doubt in the significance of postsecondary education to the development of society,especially with the ascending need for skilled employees capable of complex work. Nevertheless,U.S.ranks only11th in the higher education attachment worldwide,which makes thefinancial support from large charitable organizations necessary.As it’s essential for charitable organizations to maximize the effectiveness of donations,an objective and systematic assessment model is in demand to develop appropriate investment strategies.To achieve this goal,several large foundations like Gates Foundation and Lumina Foundation have developed different evaluation approaches,where they mainly focus on spe-cific indexes like attendance and graduation rate.In other empirical literature,a Forbes ap-proach(Shifrin and Chen,2015)proposes a new indicator called the Grateful Graduates Index, using the median amount of private donations per student over a10-year period to measure the return on investment.Also,performance funding indicators(Burke,2002,Cave,1997,Ser-ban and Burke,1998,Banta et al,1996),which include but are not limited to external indicators like graduates’employment rate and internal indicators like teaching quality,are one of the most prevailing methods to evaluate effectiveness of educational donations.However,those methods also arise with widely acknowledged concerns(Burke,1998).Most of them require subjective choice of indexes and are rather arbitrary than data-based.And they perform badly in a data environment where there is miscellaneous cross-section data but scarce time-series data.Besides,they lack quantified analysis in precisely predicting or measuring the social benefits and the positive effect that the investment can generate,which serves as one of the targets for the Goodgrant Foundation.In accordance with Goodgrant Foundation’s request,this paper provides a prudent def-inition of return on investment(ROI)for charitable organizations,and develops an original data-motivated model,which is feasible even faced with tangled cross-section data and absent time-series data,to determine the optimal strategy for funding.The strategy contains selection of institutions and distribution of investment across institutions,time and regions.1.2Baseline ModelOur definition of ROI is similar to its usual meaning,which is the increase in students’educational performance over the amount Goodgrant Foundation donates(assuming other donationsfixed,it’s also the increase in total donation amount).First we cope with data missingness.Then,to quantify students’educational performance, we develop an index called performance index,which is a linear composition of commonly used performance indicators.Our major task is to build a model to predict the change of this index given a distribution of Goodgrant Foundation$100m donation.However,donation does not directly affect the performance index and we would encounter endogeneity problem or neglect effects of other variables if we solely focus on the relation between performance index and donation amount. Instead,we select several variables that are pivotal in predicting the performance index from many potential candidates,and determine their coefficients/effects on the performance index. We call these variables performance contributing variables.Due to absence of time-series data,it becomes difficult tofigure out how performance con-tributing variables are affected by donation amount for each institution respectively.Instead, wefit the relation between performance contributing variables and donation amount across all institutions and get afitted function from donation amount to values of performance contribut-ing variables.Then we divide the impact of donation amount into2parts:homogenous and heteroge-nous one.The homogenous influence is modeled as the change infitted values of performance contributing variables over increase in donation amount(We call these quotientsfitted ROI of performance contributing variable).The heterogenous one is modeled as a tuning parameter, which adjusts the homogenous influence based on deviation from thefitted function.And their product is the institution-specific increase in true values of performance contributing variables over increase in donation amount(We call these values ROI of performance contributing vari-able).The next step is to calculate the ROI of the performance index by adding the products of ROIs of performance contributing variables and their coefficients on the performance index. This ROI is institution-specific and dependent on increase in donation amount.By adopting a two-step ROI maximization algorithm,we determine the optimal investment strategy.Also,we propose an extended model to handle problems caused by time duration and geographical distribution of donations.Note:we only use data from the provided excel table and that mentioned in the pdffile.Table1:Data SourceVariable DatasetPerformance index Excel tablePerformance contributing variables Excel table and pdffileDonation amount PdffileTheflow chart of the whole model is presented below in Fig1:Figure1:Flow Chart Demonstration of the Model1.3Detailed Definitions&Assumptions 1.3.1Detailed Definitions:1.3.2Assumptions:A1.Stability.We assume data of any institution should be stable without the impact from outside.To be specific,the key factors like the donation amount and the performance index should remain unchanged if the college does not receive new donations.A2.Goodgrant Foundation’s donation(Increase in donation amount)is discrete rather than continuous.This is reasonable because each donation is usually an integer multiple of a minimum amount,like$1m.After referring to the data of other foundations like Lumina Foundation,we recommend donation amount should be one value in the set below:{500000,1000000,1500000, (10000000)A3.The performance index is a linear composition of all given performance indicators.A4.Performance contributing variables linearly affect the performance index.A5.Increase in donation amount affects the performance index through performance con-tributing variables.A6.The impact of increase in donation amount on performance contributing variables con-tains2parts:homogenous one and heterogenous one.The homogenous influence is repre-sented by a smooth function from donation amount to performance contributing variables.And the heterogenous one is represented by deviation from the function.1.4The Advantages of Our ModelOur model exhibits many advantages in application:•The evaluation model is fully data based with few subjective or arbitrary decision rules.•Our model successfully identifies the underlying mechanism instead of merely focusing on the relation between donation amount and the performance index.•Our model takes both homogeneity and heterogeneity into consideration.•Our model makes full use of the cross-section data and does not need time-series data to produce reasonable outcomes.2Addressing the Missing ValuesThe provided datasets suffer from severe data missing,which could undermine the reliabil-ity and interpretability of any results.To cope with this problem,we adopt several different methods for data with varied missing rate.For data with missing rate over50%,any current prevailing method would fall victim to under-or over-randomization.As a result,we omit this kind of data for simplicity’s sake.For variables with missing rate between10%-50%,we use imputation techniques(Little and Rubin,2014)where a missing value was imputed from a randomly selected similar record,and model-based analysis where missing values are substituted with distribution diagrams.For variables with missing rate under10%,we address missingness by simply replace miss-ing value with mean of existing values.3Determining the Performance IndexIn this section,we derive a composite index,called the performance index,to evaluate the educational performance of students at every institution.3.1Performance IndicatorsFirst,we need to determine which variables from various institutional performance data are direct indicators of Goodgrant Foundation’s major concern–to enhance students’educational performance.In practice,other charitable foundations such as Gates Foundation place their focus on core indexes like attendance and graduation rate.Logically,we select performance indicators on the basis of its correlation with these core indexes.With this method,miscellaneous performance data from the excel table boils down to4crucial variables.C150_4_P OOLED_SUP P and C200_L4_P OOLED_SUP P,as completion rates for different types of institutions,are directly correlated with graduation rate.We combine them into one variable.Md_earn_wne_p10and gt_25k_p6,as different measures of graduates’earnings,are proved in empirical studies(Ehren-berg,2004)to be highly dependent on educational performance.And RP Y_3Y R_RT_SUP P, as repayment rate,is also considered valid in the same sense.Let them be Y1,Y2,Y3and Y4.For easy calculation and interpretation of the performance index,we apply uniformization to all4variables,as to make sure they’re on the same scale(from0to100).3.2Performance Index via Principal-Component FactorsAs the model assumes the performance index is a linear composition of all performance indicators,all we need to do is determine the weights of these variables.Here we apply the method of Customer Satisfaction Index model(Rogg et al,2001),where principal-component factors(pcf)are employed to determine weights of all aspects.The pcf procedure uses an orthogonal transformation to convert a set of observations of pos-sibly correlated variables into a set of values of linearly uncorrelated variables called principal-component factors,each of which carries part of the total variance.If the cumulative proportion of the variance exceeds80%,it’s viable to use corresponding pcfs(usually thefirst two pcfs)to determine weights of original variables.In this case,we’ll get4pcfs(named P CF1,P CF2,P CF3and P CF4).First,the procedure provides the linear coefficients of Y m in the expression of P CF1and P CF2.We getP CF1=a11Y1+a12Y2+a13Y3+a14Y4P CF2=a21Y1+a22Y2+a23Y3+a24Y4(a km calculated as corresponding factor loadings over square root of factor k’s eigenvalue) Then,we calculate the rough weights c m for Y m.Let the variance proportions P CF1and P CF2 represent be N1and N2.We get c m=(a1m N1+a2m N2)/(N1+N2)(This formulation is justifiedbecause the variance proportions can be viewed as the significance of pcfs).If we let perfor-mance index=(P CF 1N 1+P CF 2N 2)/(N 1+N 2),c m is indeed the rough weight of Y m in terms of variance)Next,we get the weights by adjusting the sum of rough weights to 1:c m =c m /(c 1+c 2+c 3+c 4)Finally,we get the performance index,which is the weighted sum of the 4performance indicator.Performance index= m (c m Y m )Table 2presents the 10institutions with largest values of the performance index.This rank-ing is highly consistent with widely acknowledged rankings,like QS ranking,which indicates the validity of the performance index.Table 2:The Top 10Institutions in Terms of Performance IndexInstitutionPerformance index Los Angeles County College of Nursing and Allied Health79.60372162Massachusetts Institute of Technology79.06066895University of Pennsylvania79.05044556Babson College78.99269867Georgetown University78.90468597Stanford University78.70586395Duke University78.27719116University of Notre Dame78.15843964Weill Cornell Medical College 78.143341064Identifying Performance Contributing Variables via post-LASSO The next step of our model requires identifying the factors that may exert an influence on the students’educational performance from a variety of variables mentioned in the excel table and the pdf file (108in total,some of which are dummy variables converted from categorical variables).To achieve this purpose,we used a model called LASSO.A linear model is adopted to describe the relationship between the endogenous variable –performance index –and all variables that are potentially influential to it.We assign appropriate coefficient to each variable to minimize the square error between our model prediction and the actual value when fitting the data.min β1J J j =1(y j −x T j β)2where J =2881,x j =(1,x 1j ,x 2j ,...,x pj )THowever,as the amount of the variables included in the model is increasing,the cost func-tion will naturally decrease.So the problem of over fitting the data will arise,which make the model we come up with hard to predict the future performance of the students.Also,since there are hundreds of potential variables as candidates.We need a method to identify the variables that truly matter and have a strong effect on the performance index.Here we take the advantage of a method named post-LASSO (Tibshirani,1996).LASSO,also known as the least absolute shrinkage and selection operator,is a method used for variableselection and shrinkage in medium-or high-dimensional environment.And post-LASSO is to apply ordinary least squares(OLS)to the model selected byfirst-step LASSO procedure.In LASSO procedure,instead of using the cost function that merely focusing on the square error between the prediction and the actual value,a penalty term is also included into the objective function.We wish to minimize:min β1JJj=1(y j−x T jβ)2+λ||β||1whereλ||β||1is the penalty term.The penalty term takes the number of variables into con-sideration by penalizing on the absolute value of the coefficients and forcing the coefficients of many variables shrink to zero if this variable is of less importance.The penalty coefficient lambda determines the degree of penalty for including variables into the model.After min-imizing the cost function plus the penalty term,we couldfigure out the variables of larger essence to include in the model.We utilize the LARS algorithm to implement the LASSO procedure and cross-validation MSE minimization(Usai et al,2009)to determine the optimal penalty coefficient(represented by shrinkage factor in LARS algorithm).And then OLS is employed to complete the post-LASSO method.Figure2:LASSO path-coefficients as a function of shrinkage factor sFigure3:Cross-validated MSEFig2.displays the results of LASSO procedure and Fig3displays the cross-validated MSE for different shrinkage factors.As specified above,the cross-validated MSE reaches minimum with shrinkage factor between0.4-0.8.We choose0.6andfind in Fig2that6variables have nonzero coefficients via the LASSO procedure,thus being selected as the performance con-tributing variables.Table3is a demonstration of these6variables and corresponding post-LASSO results.Table3:Post-LASSO resultsDependent variable:performance_indexPCTPELL−26.453∗∗∗(0.872)PPTUG_EF−14.819∗∗∗(0.781)StudentToFaculty_ratio−0.231∗∗∗(0.025)Tuition&Fees20100.0003∗∗∗(0.00002)Carnegie_HighResearchActivity 5.667∗∗∗(0.775)Constant61.326∗∗∗(0.783)Observations2,880R20.610Adjusted R20.609Note:PCTPELL is percentage of students who receive aPell Grant;PPTUG_EF is share of students who are part-time;Carnegie_HighResearchActivity is Carnegie classifica-tion basic:High Research ActivityThe results presented in Table3are consistent with common sense.For instance,the pos-itive coefficient of High Research Activity Carnegie classification implies that active research activity helps student’s educational performance;and the negative coefficient of Student-to-Faculty ratio suggests that decrease in faculty quantity undermines students’educational per-formance.Along with the large R square value and small p-value for each coefficient,the post-LASSO procedure proves to select a valid set of performance contributing variables and describe well their contribution to the performance index.5Determining Investment Strategy based on ROIWe’ve identified5performance contributing variables via post-LASSO.Among them,tu-ition&fees in2010and Carnegie High-Research-Activity classification are quite insusceptible to donation amount.So we only consider the effects of increase in donation amount on per-centage of students who receive a Pell Grant,share of students who are part-time and student-to-faculty ratio.We denote them with F1,F2and F3,their post-LASSO coefficients withβ1,β2andβ3.In this section,wefirst introduce the procedure used tofit the relation between performance contributing variables and donation amount.Then we provide the model employed to calcu-latefitted ROIs of performance contributing variables(the homogenous influence of increase in donation amount)and the tuning parameter(the heterogenous influence of increase in dona-tion amount).Next,we introduce how to determine stly,we show how the maximiza-tion determines the investment strategy,including selection of institutions and distribution of investments.5.1Fitted Curve between Performance Contributing Variables and Donation AmountSince we have already approximated the linear relation between the performance index with the3performance contributing variables,we want to know how increase in donation changes them.In this paper,we use Generalized Adaptive Model(GAM)to smoothlyfit the relations. Generalized Adaptive Model is a generalized linear model in which the dependent variable depends linearly on unknown smooth functions of independent variables.Thefitted curve of percentage of students who receive a Pell Grant is depicted below in Fig4(see the other two fitted curves in Appendix):Figure4:GAM ApproximationA Pell Grant is money the U.S.federal government provides directly for students who needit to pay for college.Intuitively,if the amount of donation an institution receives from other sources such as private donation increases,the institution is likely to use these donations to alleviate students’financial stress,resulting in percentage of students who receive a Pell Grant. Thus it is reasonable to see afitted curve downward sloping at most part.Also,in commonsense,an increase in donation amount would lead to increase in the performance index.This downward sloping curve is consistent with the negative post-LASSO coefficient of percentage of students who receive a Pell Grant(as two negatives make a positive).5.2ROI(Return on Investment)5.2.1Model of Fitted ROIs of Performance Contributing Variables fROI iFigure5:Demonstration of fROI1Again,we usefitted curve of percentage of students who receive a Pell Grant as an example. We modeled the bluefitted curve to represent the homogeneous relation between percentage of students who receive a Pell Grant and donation amount.Recallfitted ROI of percentage of students who receive a Pell Grant(fROI1)is change in fitted values(∆f)over increase in donation amount(∆X).SofROI1=∆f/∆XAccording to assumption A2,the amount of each Goodgrant Foundation’s donation falls into a pre-specified set,namely,{500000,1000000,1500000,...,10000000}.So we get a set of possible fitted ROI of percentage of students who receive a Pell Grant(fROI1).Clearly,fROI1is de-pendent on both donation amount(X)and increase in donation amount(∆X).Calculation of fitted ROIs of other performance contributing variables is similar.5.2.2Model of the tuning parameter P iAlthough we’ve identified the homogenous influence of increase in donation amount,we shall not neglect the fact that institutions utilize donations differently.A proportion of do-nations might be appropriated by the university’s administration and different institutions allocate the donation differently.For example,university with a more convenient and well-maintained system of identifying students who needfinancial aid might be willing to use a larger portion of donations to directly aid students,resulting in a lower percentage of under-graduate students receiving Pell grant.Also,university facing lower cost of identifying and hiring suitable faculty members might be inclined to use a larger portion of donations in this direction,resulting in a lower student-to-faculty ratio.These above mentioned reasons make institutions deviate from the homogenousfitted func-tion and presents heterogeneous influence of increase in donation amount.Thus,while the homogenous influence only depends on donation amount and increase in donation amount, the heterogeneous influence is institution-specific.To account for this heterogeneous influence,we utilize a tuning parameter P i to adjust the homogenous influence.By multiplying the tuning parameter,fitted ROIs of performance con-tributing variables(fitted value changes)convert into ROI of performance contributing variable (true value changes).ROI i=fROI i·P iWe then argue that P i can be summarized by a function of deviation from thefitted curve (∆h),and the function has the shape shown in Fig6.The value of P i ranges from0to2,because P i can be viewed as an amplification or shrinkage of the homogenous influence.For example,P i=2means that the homogeneous influence is amplified greatly.P i=0means that this homogeneous influence would be entirely wiped out. The shape of the function is as shown in Fig6because of the following reasons.Intuitively,if one institution locates above thefitted line,when deviation is small,the larger it is,the larger P i is.This is because the institution might be more inclined to utilize donations to change that factor.However,when deviation becomes even larger,the institution grows less willing to invest on this factor.This is because marginal utility decreases.The discussion is similar if one institution initially lies under thefitted line.Thus,we assume the function mapping deviation to P i is similar to Fig6.deviation is on the x-axis while P i is on the y-axis.Figure6:Function from Deviation to P iIn order to simplify calculation and without loss of generality,we approximate the function。
数学建模论文(精选4篇)
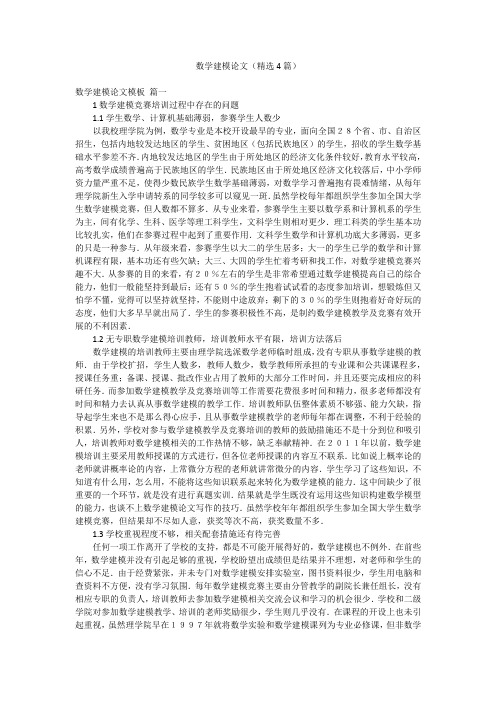
数学建模论文(精选4篇)数学建模论文模板篇一1数学建模竞赛培训过程中存在的问题1.1学生数学、计算机基础薄弱,参赛学生人数少以我校理学院为例,数学专业是本校开设最早的专业,面向全国28个省、市、自治区招生,包括内地较发达地区的学生、贫困地区(包括民族地区)的学生,招收的学生数学基础水平参差不齐.内地较发达地区的学生由于所处地区的经济文化条件较好,教育水平较高,高考数学成绩普遍高于民族地区的学生.民族地区由于所处地区经济文化较落后,中小学师资力量严重不足,使得少数民族学生数学基础薄弱,对数学学习普遍抱有畏难情绪,从每年理学院新生入学申请转系的同学较多可以窥见一斑.虽然学校每年都组织学生参加全国大学生数学建模竞赛,但人数都不算多.从专业来看,参赛学生主要以数学系和计算机系的学生为主,间有化学、生科、医学等理工科学生,文科学生则相对更少.理工科类的学生基本功比较扎实,他们在参赛过程中起到了重要作用.文科学生数学和计算机功底大多薄弱,更多的只是一种参与.从年级来看,参赛学生以大二的学生居多;大一的学生已学的数学和计算机课程有限,基本功还有些欠缺;大三、大四的学生忙着考研和找工作,对数学建模竞赛兴趣不大.从参赛的目的来看,有20%左右的学生是非常希望通过数学建模提高自己的综合能力,他们一般能坚持到最后;还有50%的学生抱着试试看的态度参加培训,想锻炼但又怕学不懂,觉得可以坚持就坚持,不能则中途放弃;剩下的30%的学生则抱着好奇好玩的态度,他们大多早早就出局了.学生的参赛积极性不高,是制约数学建模教学及竞赛有效开展的不利因素.1.2无专职数学建模培训教师,培训教师水平有限,培训方法落后数学建模的培训教师主要由理学院选派数学老师临时组成,没有专职从事数学建模的教师.由于学校扩招,学生人数多,教师人数少,数学教师所承担的专业课和公共课课程多,授课任务重;备课、授课、批改作业占用了教师的大部分工作时间,并且还要完成相应的科研任务.而参加数学建模教学及竞赛培训等工作需要花费很多时间和精力,很多老师都没有时间和精力去认真从事数学建模的教学工作.培训教师队伍整体素质不够强、能力欠缺,指导起学生来也不是那么得心应手,且从事数学建模教学的老师每年都在调整,不利于经验的积累.另外,学校对参与数学建模教学及竞赛培训的教师的鼓励措施还不是十分到位和吸引人,培训教师对数学建模相关的工作热情不够,缺乏奉献精神.在2011年以前,数学建模培训主要采用教师授课的方式进行,但各位老师授课的内容互不联系.比如说上概率论的老师就讲概率论的内容,上常微分方程的老师就讲常微分的内容.学生学习了这些知识,不知道有什么用,怎么用,不能将这些知识联系起来转化为数学建模的能力.这中间缺少了很重要的一个环节,就是没有进行真题实训.结果就是学生既没有运用这些知识构建数学模型的能力,也谈不上数学建模论文写作的技巧.虽然学校年年都组织学生参加全国大学生数学建模竞赛,但结果却不尽如人意,获奖等次不高,获奖数量不多.1.3学校重视程度不够,相关配套措施还有待完善任何一项工作离开了学校的支持,都是不可能开展得好的,数学建模也不例外.在前些年,数学建模并没有引起足够的重视,学校盼望出成绩但是结果并不理想,对老师和学生的信心不足.由于经费紧张,并未专门对数学建模安排实验室,图书资料很少,学生用电脑和查资料不方便,没有学习氛围.每年数学建模竞赛主要由分管教学的副院长兼任组长,没有相应专职的负责人,培训教师去参加数学建模相关交流会议和学习的机会很少.学校和二级学院对参加数学建模教学、培训的老师奖励很少,学生则几乎没有.在课程的开设上也未引起重视,虽然理学院早在1997年就将数学实验和数学建模课列为专业必修课,但非数学专业只是近几年才开始列为公选课开设,且选修率低.2针对存在问题所采取的相应措施2.1扩大宣传,重视数学和计算机公选课开设,举办数学建模学习讨论班最近两年,学院组建了数学建模协会,负责数学建模的宣传和参赛队员的海选,通过各种方式扩大了对数学建模的宣传和影响,安排数学任课教师鼓励数学基础不错的学生参赛.同时邀请重点大学具有丰富培训经验的老师来做数学建模专题讲座,交流经验.学院重视数学专业的基础课程、核心课程的教学,选派经验丰富的老教师、青年骨干教师担任主讲,随时抽查教学质量,教学效果.严抓考风学风,对考试作弊学生绝不姑息;学生上课迟到、早退、旷课一律严肃处理.通过这些举措,学生学习态度明显好转,数学能力慢慢得到提高.学校有意识在大一新生中开设数学实验、数学建模和相关计算机公选课,让对数学有兴趣的学生能多接触这方面的知识,减少距离感.选用的教材内容浅显而有趣味,主要目的是让同学们感受到数学建模并非高不可攀,数学是有用的,增加学生学习数学的热情和参加数学建模竞赛的可能性.为了解决学生学习数学建模过程中的遇到的困难,学院组织老师、学生参加数学建模周末讨论班,老师就学生学习过程中遇到的普遍问题进行讲解,学生分小组相互讨论,尽量不让问题堆积,影响后续学习积极性.通过这些措施,参赛学生的人数比以往有了大的改观,参赛过程中退赛的学生越来越少,参赛过程中的主动性也越来越明显.2.2成立数学建模指导教师组,分批培养培训教师,改进培训方法近年来,学院开始重视对数学建模培训教师的梯队建设,成立了数学建模指导教师组.把培训教师分批送出去进修,参加交流会议,学习其它高校的经验,并安排老教师带新教师,培训教师队伍越来越稳定、壮大.从去年开始,理学院组织学生进行了为期一个月的暑期数学建模真题实训,从8月初到8月底,培训共分为7轮.学生首先进行三天封闭式真题训练———其次答辩———最后交流讨论.效果明显,学生的数学建模能力普遍得到了提高,学习积极性普遍高涨.9月份顺利参加了全国大学生数学建模竞赛.从竞赛结果来看,比以前有了比较大的进步,不管是获奖的等次还是获奖的人数上都取得了历史性突破.有了这些可喜的变化,教师和学生的积极性都得到了提高,对以后的数学建模教学和培训工作将起着极大的促进作用.除了这种集训,今后,数学建模还需要加强平时的教学和培训工作.2.3学校逐渐重视,加大了相关投入,完善了激励措施最近几年,学校加大了对数学建模教学和培训工作的相关投入和鼓励措施.安排了专门的数学建模实验室,配备了学院最先进的电脑、打印机等设备,购买了数学建模相关的书籍.划拨了数学建模教学和培训专项经费.虽然数学建模教学还没有计入教学工作量,但已经考虑计入职称评定的相关工作量中,对参加数学建模教学和培训的老师减少了基本的教学工作量,使他们有更多的时间和精力投入到数学建模的相关工作中去.对参加全国大学生数学建模竞赛获奖的老师和学生的奖励额度也比以前有了很大的提高,老师和学生的积极性得到了极大的提高.3结束语对我们这类院校而言,最重要的数学建模赛事就是一年一度的全国大学生数学建模竞赛了.竞赛结果大体可以衡量老师和学生的付出与收获,但不是绝对的,教育部组织这项赛事的初衷主要是为了促进各个院校数学建模教学的有效开展.如果过分的看重获奖等次和数量,对学校的数学建模教学和组织工作都是一种伤害.参赛的过程对学生而言,肯定是有益的,绝大多数参加过数学建模竞赛的学生都认为这个过程很重要.这个过程可能是四年的大学学习过程中体会最深的,它用枯燥的理论知识解决了活生生的现实中存在的问题,虽然这种解决还有部分的理想化.由于我校地处偏远山区,教育经费相对紧张,投入不可能跟重点院校的水平比,只能按照自身实际来.只要学校、老师、学生三方都重视并积极参与这一赛事,数学建模活动就能开展的更好.数学建模论文模板篇二培养应用型人才是我国高等教育从精英教育向大众教育发展的必然产物,也是知识经济飞速发展和市场对人才多元化需求的必然要求。
数学建模论文(7篇)
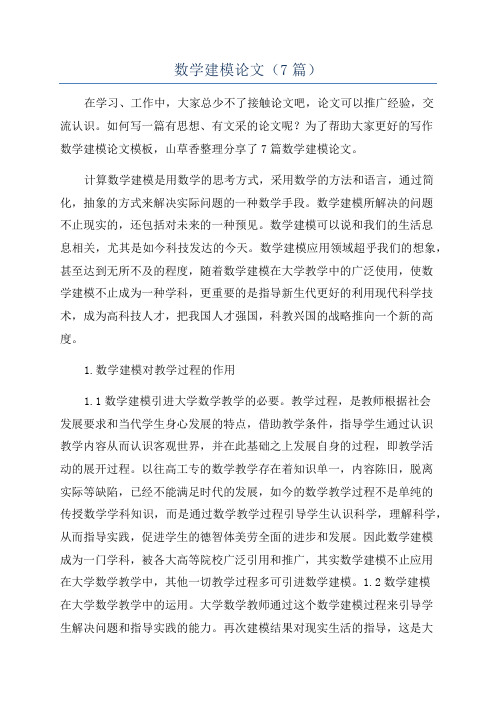
数学建模论文(7篇)在学习、工作中,大家总少不了接触论文吧,论文可以推广经验,交流认识。
如何写一篇有思想、有文采的论文呢?为了帮助大家更好的写作数学建模论文模板,山草香整理分享了7篇数学建模论文。
计算数学建模是用数学的思考方式,采用数学的方法和语言,通过简化,抽象的方式来解决实际问题的一种数学手段。
数学建模所解决的问题不止现实的,还包括对未来的一种预见。
数学建模可以说和我们的生活息息相关,尤其是如今科技发达的今天。
数学建模应用领域超乎我们的想象,甚至达到无所不及的程度,随着数学建模在大学教学中的广泛使用,使数学建模不止成为一种学科,更重要的是指导新生代更好的利用现代科学技术,成为高科技人才,把我国人才强国,科教兴国的战略推向一个新的高度。
1.数学建模对教学过程的作用1.1数学建模引进大学数学教学的必要。
教学过程,是教师根据社会发展要求和当代学生身心发展的特点,借助教学条件,指导学生通过认识教学内容从而认识客观世界,并在此基础之上发展自身的过程,即教学活动的展开过程。
以往高工专的数学教学存在着知识单一,内容陈旧,脱离实际等缺陷,已经不能满足时代的发展,如今的数学教学过程不是单纯的传授数学学科知识,而是通过数学教学过程引导学生认识科学,理解科学,从而指导实践,促进学生的德智体美劳全面的进步和发展。
因此数学建模成为一门学科,被各大高等院校广泛引用和推广,其实数学建模不止应用在大学数学教学中,其他一切教学过程多可引进数学建模。
1.2数学建模在大学数学教学中的运用。
大学数学教师通过这个数学建模过程来引导学生解决问题和指导实践的能力。
再次建模结果对现实生活的指导,这是大学数学教学中数学建模所需要达到的效果和要求。
不再停留在理论学习,而是通过理论指导实践,从而为科学的进步和人才综合水平的提高提供可能。
2.数学建模对当代大学生的作用2.2数学建模对学生综合能力的提高数学建模是大学数学教师运用数学科学去分析和解决实际问题,在数学建模学习的过程中,大学生的数学能力得到提高,其分析问题、解决问题的能力得到提高,这对大学生毕业走向社会具有着重大意义。
2016年全国大学生数学建模竞赛获奖论文

3470.00 3468.00 3466.00 3464.00 3462.00 3460.00 3458.00 3456.00 3454.00 3452.00 3450.00 3448.00 3446.00 3444.00 3442.00 3440.00 3438.00 3436.00 3434.00 3432.00 3430.00 3428.00 3426.00 3424.00 3422.00 3420.00 3418.00 3416.00 3414.00 3412.00 3410.00 3408.00 3406.00 3404.00 3402.00 3400.00 3398.00 3396.00 3394.00 3392.00 3390.00 3388.00 3386.00
电池剩余放电时间预测模型
摘要
本文针对放电曲线预测问题,在不同情况下,建立出合理模型,并依据所给 条件,运用 Matlab、Lingo、Excel 等工具,得出了问题的相关结果。 对于问题一, 本文首先剔除放电初期的不稳定数据, 建立了二元抛物线模型 一: U ( I , T) a(Tmax ( I ) T )b U m ,通过固定电流强度 I ,利用非线性参数拟合, 得出放电曲线;再利用三样条插值,得出插值点,计算出对应 MRE 为(0.15%, 0.20%,0.20%,0.15%,0.13%,0.26%,0.24%,0.25%) , U 9.8 下的剩余放电 时间为(937.96,608.06,440.50,333.37,280.54,261.65,237.75,212.82, 197.33 ) ;接着在模型一的基础上通过增加震荡函数,建立模型二:
其中 a, b 为系数。
(1)
4.1.2 模型一求解
2016年美国大学生数学建模竞赛C题H奖论文

Contents1.Introduction (1)1.1 Background (1)1.2 Foundation & ROI (1)2 Task (1)3 Fundamental assumptions (2)4 Definitions and Notations (2)5 Models (3)5.1 Filter data (3)5.2 Object Selection Model (Grey Relational Analysis) (4)5.2.1 Model analysis (4)5.2.2 Model solution (4)5.3 ROI Model (Principal Component Analysis) (5)5.3.1 Model analysis (5)5.3.2 Model solution (6)5.4 Verify the possibility (9)5.4.1 Comparison (9)5.4.2 External factor (10)5.5 Investment Forecast Model (11)5.5.1 Linear Regression Forecasting Model (11)5.5.2 School potential Prediction (TOPSIS) (12)5.5.3 Final investment (TOPSIS) (13)6 Conclusions (16)7 Strengths and Weaknesses (18)7.1 Strengths (19)7.2 Weaknesses (20)8 Letter to Mr. Alpha Chiang (21)9 References (22)1 Introduction1.1 BackgroundThe Goodgrant Foundation is a charitable organization that wants to help improve educational performance of undergraduates attending colleges and universities in the United States. To do this, the foundation intends to donate a total of $100,000,000 (US100 million) to an appropriate group of schools per year, for five years, starting July 2016. In doing so, they do not want to duplicate the investments and focus of other large grant organizations such as the Gates Foundation and Lumina Foundation.Our team has been asked by the Goodgrant Foundation to develop a model to determine an optimal investment strategy that identifies the schools, the investment amount per school, the return on that investment, and the time duration that the organi zation’s money should be provided to have the highest likelihood of producing a strong positive effect on student performance. This strategy should contain a 1 to N optimized and prioritized candidate list of schools you are recommending for investment bas ed on each candidate school’s demonstrated potential for effective use of private funding, and an estimated return on investment (ROI) defined in a manner appropriate for a charitable organization such as the Goodgrant Foundation.1.2 Foundation & ROIFoundation (charitable foundation) refers to the nonprofit legal person who uses the property of the natural persons, legal persons or other organizations to engage in public welfare undertakings. In terms of its nature, foundation is a kind of folk non-profit organizations.ROI is a performance measure used to evaluate the efficiency of an investment or to compare the efficiency of a number of different investments. ROI measures the amount of return on an investment relative to the investment’s cost. To calculate ROI, the benefit (or return) of an investment is divided by the cost of the investment, and the result is expressed as a percentage or a ratio.2 Task●One-page summary for our MCM submission●Using our models to achieve the candidate list of schools●Calculate the time durati on that the organization’s money should be provided to have thehighest likelihood of producing a strong positive effect on student performance●Calculate the investment amount Goodgrant Foundation would pay for each school●Calculate the ROI of the Goodgrant Foundation●Forecast the development of this kind of investment mode●Write a letter to the CFO of the Goodgrant Foundation, Mr. Alpha Chiang, that describesthe optimal investment strategy3 Fundamental assumptions1) The indexes of GRA (such as ACT 、SA T 、Pell Grant 、Graduation Rate 、Retention Rate 、Graduates income)are the most influential factor that affect the use potential of school funds, what’s more, the indexes have the same weight2) For four-year universities, their C200_L4_POOLED_SUPP 、RET_FTL4、RET_PTL4 arezero; For two-year colleges, their C150_4_POOLED_SUPP 、RET_FT4、RET_PT4 are zero3) For public institutions ,their NPT4_PRIV 、NPT41_PRIV 、NPT42_PRIV 、NPT43_PRIV 、NPT44_PRIV 、NPT45_PRIV are zero; For private for-profit and nonprofit institutions, their NPT4_PUB 、NPT41_PUB 、NPT42_PUB 、NPT43_PUB 、NPT44_PUB 、NPT45_PUB are zero4) We define “NULL” appears in the data except appears in 3) as the average of that series5) Ignore the influence of degree-conferring situation, race, religion, region6) Schools’ data of SA T and ACT d evelop in a linear trend4 Definitions and NotationsTable A: The Excel which contain the IPEDS UID for Potential Candidate SchoolsTable B: The Excel which contain the Most Recent Cohorts Data (Scorecard Elements) : The weight of the first k index)(k iξ: Grey relational coefficient r i : Grey weight relation: Standardized index value: Index value: Sample average: Sample standard deviation:Standardized index vector:The correlation coefficient y i: Main components : Rate of contribution:The cumulative contribution rate : The comprehensive scorek w ~a ij a ij j μj s ~x jr ijb j pαZ5 Models5.1 Filter dataBecause the topic has a large number of additional data, we should classify the data based on the College Scorecard Data Dictionary which we can find from the official website .And then, according to the flow diagram5.1.1 as follow, we can filtering data. By using that flow diagram, we set up limits to filter the data circularly. We use SPSS to achieve above purpose, after that we get the valid data of 2936 potential candidate schools.InputTable A,TableBBased on the ID in TableA, merge Table A and BTable A has theID which TableB don’tDelete that IDin Table AOutputTable AOutputTableAFigure 5.1.1 Flow Diagram5.2 Object Selection Model (Grey Relational Analysis)5.2.1 Model analysisNot only there is a large amounts of missing in the original data, but also even after a preliminary screening, the data volume is still very large. We find there are more than 50 factors which affect us optimizing the school , what ’s more, the link between each factor we can't find accurately. So the normal model has no use to predict and evaluate the data .But Grey correlation analysis method is both suitable for irregular data and normal data. The quantitative results are consistent with qualitative analysis perfectly. Therefore, we choose that method to further narrowing the scope of the data.Grey correlation analysis is based on the similarity degree of various factors ’ changing curve , it can judge the correlation degree of each index .Through the quantitative analysis of the dynamic process, we can get the geometrical relationship of statistical data in the system and the grey correlation degree between the reference sequence and compare sequence. The greater the comparative sequence ’s correlation degree is , the closer the relationship between the reference sequence and compare sequence will be.The basic idea is to standardized the original observation and calculate the correlation coefficient, correlation degree. And then rank the indexes according to the size of the correlation. The application of GRA involves many fields, especially in the field of social economy, such as the ROI of the national economy departments , analyzing regional economic advantages , industrial structure adjustment, GRA has a good application effect.5.2.2 Model solution1) We have 2936 objects (potential candidate schools )and 7 evaluation indexes (ACT 、SAT 、Pell Grant 、Graduation Rate 、Retention Rate 、Graduates income ),the reference sequence is}7,...,2,1|)({00==k k x x , the compare sequence is 2936,...,2,1},7,...,2,1|)({===i k k x i i x ;2) The weight of every index is ],...,[71w w w =,)7,...,2,1(=k w k means the first k index ’sweight , in that model we assume every index ’s weight is equal.3) |)()(|max max |)()(||)()(|max max |)()(|min min )(0000t x t x t x k x t x t x t x t x k s t s i s t s s t s i-+--+-=ρρξ 4) )(71k i k i i w r ξ∑==5) By using MATLAB, we can finish above data calculation process ,and then we can makethe figure of each evaluation objects’ grey weight relatio n as follow:Figure 5.2.1 Distribution Diagram of Grey Weight Relation According to Figure 5.2.1,we find that 90% objects’grey weight relation is under 0.60.We use MA TLAB to rank the evaluation objects, because the greater the grey weight relation is, the better the evaluation result is. We choose the first 300 objects as the new potential candidate schools.But during the process of GRA, the weight of evaluation indexes has deviation, we can’t get the accurate solution, so we need build another model to analyze in detail.5.3ROI Model (Principal Component Analysis)5.3.1 Model analysisAccording to the topic’s requirements, we should choose the potential candidate schools by their money using ability, but there are so many indexes influence the resul t, we can’t get reliable evaluation only by one factor.In the study of that practical problem, in order to comprehensively and systematically analyze problems, we must consider many factors. These involved factors generally referred to the index, also known as a variable in the multivariate statistical analysis. Because each variable reflects some information of the research question and all of them has a certain correlation , the information one index reflects may overlap another.PCA is to use less variables to explain most variables of the original data, it transforms many high correlation variables into uncorrelated variables. Usually the number of new variables is smaller than the original variables, we call it principal component and use it to explain the comprehensive index.This method simplify the problem, at the same time make the result more scientific and effectiveTherefore ,it seems easier to use PCA solving the Optimization problem. By reducing dimension, we transform many indexes(such as Net Price, Repayment of Debt, Repayment Rate, Graduates Income) into a few principal components.5.3.2 Model solution1) Standardized the original data,15,...,2,1,300,...,2,1,~==-=j i s j j ij ij a a μ;15,...,2,1,)(13001,3001300123001=--==∑∑==j a a i j ij j i ij j s μμ 15,...,2,1,~=-=j s x x j j jjμ2) Calculate the correlation coefficient matrix,15*15)(r ij R =,15,...,2,1,,1300*~3001~=-=∑=j i a a r kj k ki ij3) Calculate the eigenvalue and the feature vectors,By using SPSS, we calculate the eigenvalue of R ,0...1521≥≥≥≥λλλ,And the standardized feature vector ,,...,,1521μμμby using the feature vector, we get 15 newindexes ,,......,,...,...~151515~2215~111515~15152~222~1122~15151~221~1111x x x x x x x x x y yyμμμμμμμμμ+++=+++=+++= 4) Choose P (15≤P )principal component ,calculate the comprehensive score①Calculate the contribution rate of )15,...,2,1(=j j λ and cumulative contribution rate∑∑====151151,j j p k k j j b b αλλ By using SPSS, we get the contribution rate of 15 eigenvalue as follow:Table 5.3.1 Contribution RateAnalyzing Table 5.3.1,we find the cumulative contribution rate of the first three eigenvalue is more than 90%, the model has a well result.②Choose the first three principal component for a comprehensive evaluation,y b j p j j Z ∑==1By using SPSS, we get the feature vectors of the first three characteristic root,shows in Table 5.3.2Table 5.3.2 The Feature VectorsWe make the first three principal components ’ contribution rate as weight, build up the principal component comprehensive evaluation model,y y y Z 3211031.01153.06892.0++= Put every optimized school’s three principal components into above equation ,we get the comprehensive evaluation result of 300 new potential candidate schools, shows in figure5.3.3 ,Fac_1 on behalf ofy 1,Fac_2 on behalf of y 2,Fac_3 on behalf of y 3, Total onbehalf of Z ,Figure 5.3.3 Comprehensive evaluation result of 300 schoolsWe choose the first 40 schools as the final potential candidates list ,the comprehensive evaluation result shows in figure 5.3.4,Figure 5.3.4 Comprehensive evaluation result of 40 final potential schoolsBecause the indexes (such as Net Price, Repayment of Debt, Repayment Rate, Graduates Income) of this model are closely related to ROI, we use the comprehensive score to evaluate schools’ ROI. We ranked it in Table 5.3.3,Table 5.3.3 Rank5.4 Verify the possibility5.4.1 ComparisonThrough comparison we found that many famous universities such as Harvard University does not appear in our preferred list, this can’t help but let us create confusion, what is the reason causes this kind of phenomenon ?Considering that phenomenon, we analyze from the model itself. The topic asks us to optimize the school list which is based on the potential of fund using .We've learned from the related literature , there is a positive correlation between the income of graduate individual and donation. As their income level become higher, the possibility and amount of donation is greater. According to statistics, every 1% increase in income, the possibility to donation increase 0.35% ~ 0.5%; when the personal income increased $10000, donation amount can increased 2%; per $10000 increase in household income, donation amount can increased 9%.So at the beginning, we choose Net Price, Repayment of Debt, Repayment Rate, Graduates Income as evaluation indexes.We standardized the indexes, and then compare the data of final potential schools with Harvard University, the result shows in Figure 5.4.1Figure 5.4.1 ComparisonAccording to Figure 5.4.1, we find the average net price and the monthly repayment of the debt are significantly less than final potential schools. The bigger the average net price and the monthly repayment of the debt are, the higher the students’ needs for money are, so the school has high potential to use the fund.Like Harvard University, there are many famous foundations invest it, its students have enough funds ,so there is no doubt that it has low average net price and monthly repayment of the debt. That is the reason which makes Harvard University get low comprehensive score in our model.5.4.2 External factorBecause the Goodgrant Foundation do not want to duplicate the investments and focus of other large grant organizations, we check out the Gates foundation's donation list of schools and compare it with the potential candidates list which we get in Figure 5.3.4, what’s more, we eliminate repetitive schools to get the final candidates list, shows in Table 5.4.2(the red name means that school should remove from the potential candidates list),Table 5.4.2 Real rank5.5 Investment Forecast Model5.5.1 Linear Regression Forecasting Model1)We find previous years’ (09-13years) Reference data which is closely related to students’performance(such as ACT,SAT, Enrollment of undergraduate degree-seeking students) from “https:///ipeds/datacenter/Default.aspx”.According to Table 5.4.2,we use that 36 schools’ data to do the linear regression prediction.2)There are many schools’ data lost in the table,so how to deal with those “NULL”?We choose other years data of that school, doing the linear regression prediction, and then getthe data of that year. If other years data have also lost , we take the average data of other schools as the lost data.3)Because the data we can use is limited(no more than 5 years),we can’t doing theComplex forecast. We choose Linear Regression prediction, by analyzing the variation trend of 36 schools’ data and forecast 2016~2020 years’ ACT and SCT . Based on forecast data, We make the diagram of 2016 ~ 2020 data variation trend which shows as Figure 5.5.1 and Figure 5.5.2 ,analyze the development of student performance primarily.Figure 5.5.1 Figure 5.5.25.5.2 School potential Prediction (TOPSIS)1)Based on forecast data which we get from Linear Regression Forecasting Model, we useTOPSIS method to evaluate the effect which The Goodgrant Foundation’s investment can takes in the future 5years.2)We take five factors (such as ACT and SAT mark、the growing trend of the mark and thegrowing trend of undergraduate degree-seeking students’ number) as indexes, evaluate the potential of its annual school development . We calculate the comprehensive evaluation score, and then sorting every candidate school by score.3)According to the influence the five factors have, we define the weight of every factor asfollow: ACT mark--0.25, SAT mark--0.25,both the growing trend of ACT and SAT are0.2, the growing trend of undergraduate degree-seeking students’ number--0.1.4)We use MA TLAB to calculate the comprehensive evaluation score and show the result inFigure 5.4.3,analyze the Figure, we find between 2016 and 2020 every school’s score changes little, it means every school’s development capacity is stable. What’s more, the score of the first 20 schools which get the higher score in 2016 are always higher than other school from 2017 to 2020,so we choose those 20 schools as the reliable potentialcandidate list, we think those school both has the better ROI and development potential.Figure 5.5.3 ScoreThe score of those 30 schools from2016 to 2020 is showed in Table 5.5.1ID Z[SP]_2016Z[SP]_2017Z[SP]_2018Z[SP]_2019Z[SP]_2020 1213090.581 0.653 0.591 0.595 0.600 1226120.574 0.609 0.575 0.576 0.577 1229310.684 0.656 0.683 0.682 0.681 1311590.543 0.527 0.526 0.518 0.511 1523180.543 0.490 0.616 0.610 0.604 1630460.628 0.490 0.512 0.509 0.506 1649880.520 0.571 0.561 0.555 0.550 1656620.574 0.725 0.601 0.598 0.596 1666560.607 0.497 0.639 0.645 0.652 1791590.548 0.551 0.534 0.528 0.523 1868670.734 0.734 0.737 0.739 0.740 1912410.611 0.549 0.602 0.598 0.594 1939000.694 0.585 0.683 0.678 0.673 1948240.556 0.524 0.549 0.547 0.544 2024800.584 0.594 0.583 0.582 0.582 2112910.624 0.526 0.613 0.607 0.602 2114400.740 0.654 0.737 0.735 0.732 2165970.601 0.488 0.587 0.580 0.573 2174930.546 0.483 0.535 0.530 0.525 2232320.611 0.595 0.607 0.605 0.603Table 5.5.1 Z[SP]5.5.3 Final investment (TOPSIS)5.5.3.1 Model AnalysisAccording to 5.5.2,we get the final 20 candidate school list, after that ,we will undertake the key process--investment allocation.There are many factors affect investment, but through the step-by-step modeling process, those factors finally can be summed up as follow : The comprehensive score of ROI model(Z[ROI]) ;The comprehensive score of School potential Prediction(Z[SP]) ;Percent of all federal undergraduate students receiving a federal student loan(PCTFLOAN);Median debtof the student (MD);The number of undergraduates(NG).Because TOPSIS method allows us to analyze the scheme by our own ideas which give us enough free space, what’s more,the result of TOPSIS method is clear, it has well operational flexibility, so we choose that to solve our problem.Weight distribution: all of the factors in 1) have great effects on investment, but both PCTFLOAN and MD belong to debt ,those share the weight of debt, so we can distribute the weight as follow:Z[ROI]--0.25,Z[SP]--0.25,NG--0.25,PCTFLOAN--0.125,MD--0.1255.5.3.2Model SolutionNG is changing with time ,we show it in Table 5.5.2,id NG_2016NG_2017NG_2018NG_2019NG_20201213092597263326692705274112261270777304753077577983122931551755795642570457671311597159725073427434752615231824102497258526722759163046414142004260431943791649881657016587166041662216639165662393740104083415642291666565866655172377923860817915985708711885389949136186867294430433142324133401912418505857886518724879719390022951231832341623648238811948245177514051045067503020248083688528868888489009211291348934853481347734732114405977601460506087612321659768916891689168916891217493203620482061207320862232321394914218144861475415023Table 5.5.2 NGWe can also get Z[ROI],PCTFLOAN,MD from previous data,ID Z[ROI]PCTFLOAN MD1213090.73034350.641249511226120.802319610.5115250001229310.765134390.3726205001311590.786649810.460323500152318 1.004578520.5776270001630460.845330140.5407270001649880.854815810.4235270001656620.924753020.567823312166656 1.112655080.783250001791590.808487460.348250001868670.785503970.6589270001912410.811966360.5467250001939000.982760460.4131232501948240.916736310.586627835.52024800.747331260.6791268632112910.767629720.4098270002114400.854345660.3964250002165970.771433880.435627000217493 1.154260170.390726024.52232320.825762390.491625281Table 5.5.3By using MATLAB, we calculate the final comprehensive score (Z [FN]) of the 20 candidate schools. According to the score, we decide how much money The Goodgrant Foundation should invest for each school. But Z[SP] is changing every year,so the investment to each school is changing. The final comprehensive score and investment from 2016 to 2020 is showed as follow table:ID Z[FN]_2016Z[FN]_2017Z[FN]_2018Z[FN]_2019Z[FN]_2020 1213090.252 0.339 0.268 0.274 0.280 1226120.280 0.343 0.292 0.298 0.303 1229310.329 0.301 0.334 0.332 0.331 1311590.211 0.225 0.206 0.204 0.203 1523180.373 0.370 0.430 0.426 0.423 1630460.366 0.288 0.281 0.281 0.282 1649880.426 0.489 0.457 0.453 0.449 1656620.304 0.460 0.337 0.336 0.336 1666560.514 0.472 0.560 0.575 0.589 1791590.264 0.293 0.262 0.261 0.261 1868670.451 0.445 0.456 0.457 0.457 1912410.351 0.317 0.347 0.344 0.342 1939000.674 0.605 0.670 0.667 0.663 1948240.367 0.371 0.366 0.365 0.364 2024800.352 0.381 0.358 0.360 0.362 2112910.311 0.250 0.304 0.299 0.295 2114400.465 0.382 0.464 0.461 0.457 2165970.321 0.269 0.312 0.307 0.302 2174930.423 0.416 0.420 0.419 0.419 2232320.437 0.446 0.442 0.444 0.446Table 5.5.4 Z[FN]ID INV_2016INV_2017INV_2018INV_2019INV_2020 121309$3,377,595$4,545,294$3,535,471$3,623,230$3,704,440 122612$3,753,266$4,594,187$3,863,051$3,934,467$4,003,692 122931$4,406,572$4,033,955$4,406,992$4,394,449$4,376,854 131159$2,817,760$3,009,942$2,716,954$2,696,351$2,682,999 152318$4,988,693$4,951,707$5,679,022$5,635,768$5,596,019 163046$4,893,351$3,860,524$3,716,334$3,720,512$3,726,668 164988$5,703,381$6,549,593$6,041,218$5,985,171$5,932,065 165662$4,069,234$6,165,503$4,455,247$4,447,100$4,439,571 166656$6,875,631$6,324,965$7,406,247$7,598,343$7,789,734 179159$3,533,653$3,922,658$3,462,914$3,456,195$3,453,114 186867$6,033,507$5,963,478$6,031,848$6,038,227$6,038,962 191241$4,695,197$4,249,163$4,587,144$4,551,653$4,517,746 193900$9,026,607$8,102,416$8,859,888$8,814,570$8,766,089 194824$4,917,651$4,973,672$4,833,172$4,824,265$4,817,097 202480$4,711,203$5,106,241$4,727,869$4,760,523$4,791,968 211291$4,167,850$3,355,796$4,021,230$3,959,898$3,900,586 211440$6,218,948$5,124,833$6,134,486$6,091,854$6,041,917 216597$4,301,641$3,607,390$4,124,471$4,055,891$3,990,970 217493$5,664,556$5,577,679$5,554,513$5,543,635$5,536,138 223232$5,843,702$5,981,004$5,841,930$5,867,899$5,893,373Table 5.5.5 INV6. ConclusionsThe candidate list of schools:Table 6.1During the evaluation of ROI Model, we get those40 schools as the first optimization list; When we compare the first optimization list with other Big Foundations’ list, the red names are deleted;During the evaluation of Investment Forecast Model, the blue names are deleted; In theend, the final candidate schools are the last 20 black names.Considering the distribution of that 40 optimized school, we can find that half of them are located in large city, the more developed the city is, the more the optimized schools locate in.Figure 6.1 LocaleThe investment list is showed in Table 6.2,We transform the data in Table 6.2 into a line chart which is showed in Figure 6.2.According to Figure 6.2 we can find , in different years The Goodgrant Foundation should undertakes different Investment strategy.Figure 6.2 INV7.Strengths and WeaknessesFor this model, we have used Grey Correlation Analysis, Principal Component Analysis, Comprehensive Evaluation Method(TOPSIS),Linear Regression Model. There are nearly 3000 schools' data with 50 index. In addition to the given data, we can't able to make a comprehensive evaluation for all university’ indexes.When we choose university at first time , the principle that according to is the ability to school’s cultivate, we can set aside most of the university due to the size of the numerical . The powerful of the university ability could be determined according to the size of the numerical. In the given index, we chose seven indicators related to the students' ability to predict the ability of university. However, these indicators without a typical distribution, so we can not use the exact formula or model to accurately judge the powerful of each university training students' ability.7.1StrengthsGrey Correlation Analysis Method, as a kind of Comprehensive Evaluation Method, it has not a exactly requirement for size of sample, also do not need the typical distribution, and the relatively small amount of calculation, the result will be the same as those of qualitative analysis, the advantages of simple and reliable. The most important thing is that it can build a relational sequence which can obtain correlation quantificationally. In this model, we can application the grey correlation method to get correlation, the size of the correlation willrepresent the strength of the school training students' ability. If size of the correlation more bigger, the evaluation results will be better.After the primary screening. we used Principal Component Analysis (PCA). Principal Component Analysis (PCA) can eliminate the mutual influence between the indexes, it also can reduce the workload of index selection, we can use a handful of composite indicator to replace the original indicators for evaluation. In this screening, we apply the indicator of return on investment regard as the university ability, we should select multiple indicators for principal component analysis, in order to determine the comprehensive variables. The return on investment required to our ability can be used a general comprehensive index to in place , according to the size of the index, we can select the list of schools that we need again. This method overcomes the defect of identified weighting, and having a standard calculation. Using the software can be implemented on the computer, the most important is that the comprehensive index value is objective and reasonable.Finally, using linear regression to forecast, then use TOPSIS to decide the last list. The reason is that we need five years list, so it must be make predictions. Linear regression method deal with multi-factor model is more simple and convenient, and the regression analysis applicable is easy to be affected by many factors. When Our model need predict index, simple easy to use regression analysis. At the end of the forecast, we using TOPSIS method to judge the development potential of the school by predict the index and the given index as influ ence factors. TOPSIS can join the evaluator’ like, it can analyze base on the director of the preference, and it can be carried out in accordance with the investors' willingness to change; The calculation results is more clear, and high maneuverability. The value that acquire by TOPSIS, can represent the development potential of the university. we can according to the size of the values to determine the amount of distribution, in order to distribution rationalization, and have a maximum functionality.7.2WeaknessesWhen we use grey correlation analysis, we should assume the value by ourselves. So it must have exist error, and the accuracy is not high.Principal component analysis model have disadvantage too. The Explain of meaning of the principal component have fuzziness, clear and exact compare with the original data.When predict date, there have a lot of missing, it must influential to forecast results.。
2016年美国大学生数学建模竞赛题论文

2016
MCM/ICM Summary Sheet (Your team's summary should be included as the first page of your electronic submission.) Type a summary of your results on this page. Do not include the name of your school, advisor, or team members on this page.
For office use only T1 ________________ T2 ________________ T3 ________________ T4 ________________
Team Control Number
52557
Problem Chosen
E
For office use only_ F3 ________________ F4 ________________
Team # 52557
i
Contents
1 Introduction ........................................................................................................................................... 1 1.1 Problem Statement..........................................................................................................................1 1.2 Problem Analysis............................................................................................................................1 1.2.1 Task 1 Analysis ........................................................................................................................ 1 1.2.2 Task 2 Analysis ........................................................................................................................ 1 1.2.3 Task 3 Analysis ........................................................................................................................ 2 1.2.4 Task 4 Analysis ........................................................................................................................ 2 1.2.5 Task 4 and 5 Analysis .............................................................................................................. 2
2016数学建模C题论文
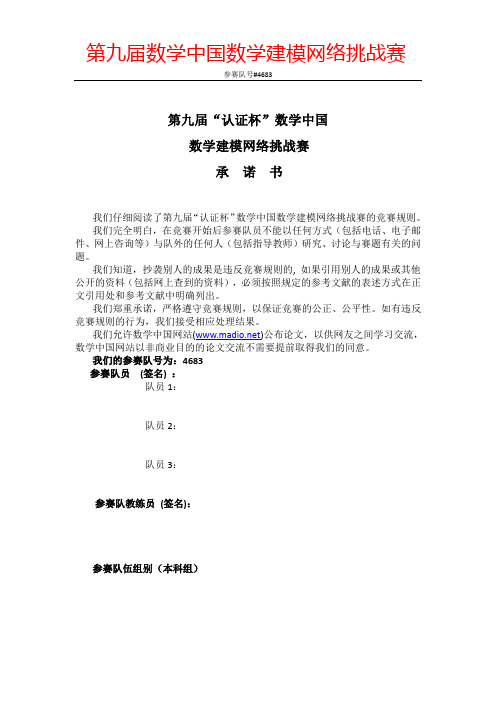
8到10岁
生命正向
生命负向
生活正向
生活负向
娱乐正向
娱乐负向
A的频数
100
139
152
140
173
162
B的频数
163
124
108
123
90
101
11到12岁
生命正向
生命负向
生活正向
生活负向
娱乐正向
娱乐负向
A的频数
152
114
174
102
2016年研究生数学建模竞赛优秀论文选-《具有遗传性疾病和性状的遗传位点分析》2-27
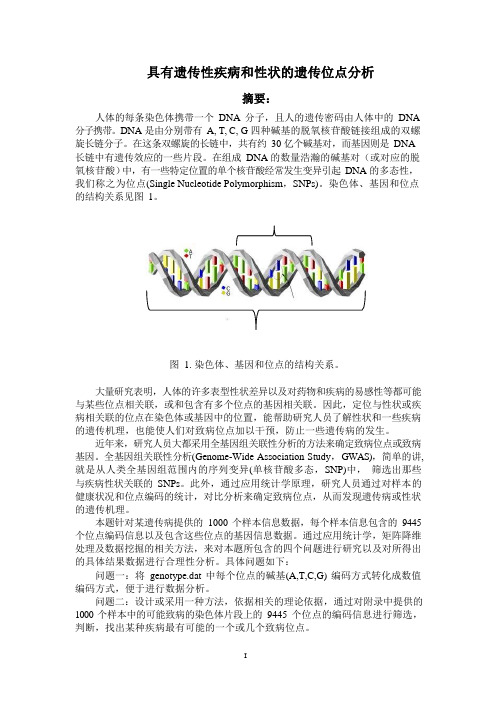
具有遗传性疾病和性状的遗传位点分析摘要:人体的每条染色体携带一个DNA 分子,且人的遗传密码由人体中的DNA 分子携带。
DNA 是由分别带有A, T, C, G 四种碱基的脱氧核苷酸链接组成的双螺旋长链分子。
在这条双螺旋的长链中,共有约30 亿个碱基对,而基因则是DNA 长链中有遗传效应的一些片段。
在组成DNA 的数量浩瀚的碱基对(或对应的脱氧核苷酸)中,有一些特定位置的单个核苷酸经常发生变异引起DNA 的多态性,我们称之为位点(Single Nucleotide Polymorphism,SNPs)。
染色体、基因和位点的结构关系见图1。
图 1. 染色体、基因和位点的结构关系。
大量研究表明,人体的许多表型性状差异以及对药物和疾病的易感性等都可能与某些位点相关联,或和包含有多个位点的基因相关联。
因此,定位与性状或疾病相关联的位点在染色体或基因中的位置,能帮助研究人员了解性状和一些疾病的遗传机理,也能使人们对致病位点加以干预,防止一些遗传病的发生。
近年来,研究人员大都采用全基因组关联性分析的方法来确定致病位点或致病基因。
全基因组关联性分析(Genome-Wide Association Study,GWAS),简单的讲, 就是从人类全基因组范围内的序列变异(单核苷酸多态,SNP)中,筛选出那些与疾病性状关联的SNPs。
此外,通过应用统计学原理,研究人员通过对样本的健康状况和位点编码的统计,对比分析来确定致病位点,从而发现遗传病或性状的遗传机理。
本题针对某遗传病提供的1000 个样本信息数据,每个样本信息包含的9445 个位点编码信息以及包含这些位点的基因信息数据。
通过应用统计学,矩阵降维处理及数据挖掘的相关方法,来对本题所包含的四个问题进行研究以及对所得出的具体结果数据进行合理性分析。
具体问题如下:问题一:将genotype.dat 中每个位点的碱基(A,T,C,G) 编码方式转化成数值编码方式,便于进行数据分析。
2016全国大学生数学建模竞赛A题论文
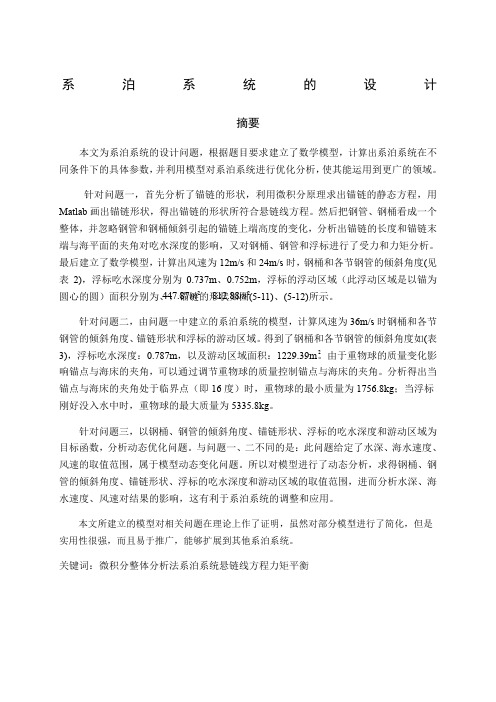
系泊系统的设计摘要本文为系泊系统的设计问题,根据题目要求建立了数学模型,计算出系泊系统在不同条件下的具体参数,并利用模型对系泊系统进行优化分析,使其能运用到更广的领域。
针对问题一,首先分析了锚链的形状,利用微积分原理求出锚链的静态方程,用Matlab 画出锚链形状,得出锚链的形状所符合悬链线方程。
然后把钢管、钢桶看成一个整体,并忽略钢管和钢桶倾斜引起的锚链上端高度的变化,分析出锚链的长度和锚链末端与海平面的夹角对吃水深度的影响,又对钢桶、钢管和浮标进行了受力和力矩分析。
最后建立了数学模型,计算出风速为12m/s 和24m/s 时,钢桶和各节钢管的倾斜角度(见表2),浮标吃水深度分别为0.737m 、0.752m ,浮标的浮动区域(此浮动区域是以锚为圆心的圆)面积分别为、,锚链的形状如图(5-11)、(5-12)所示。
针对问题二,由问题一中建立的系泊系统的模型,计算风速为36m/s 时钢桶和各节钢管的倾斜角度、锚链形状和浮标的游动区域。
得到了钢桶和各节钢管的倾斜角度如(表3),浮标吃水深度:0.787m ,以及游动区域面积:1229.39m 。
由于重物球的质量变化影响锚点与海床的夹角,可以通过调节重物球的质量控制锚点与海床的夹角。
分析得出当锚点与海床的夹角处于临界点(即16度)时,重物球的最小质量为1756.8kg ;当浮标刚好没入水中时,重物球的最大质量为5335.8kg 。
针对问题三,以钢桶、钢管的倾斜角度、锚链形状、浮标的吃水深度和游动区域为目标函数,分析动态优化问题。
与问题一、二不同的是:此问题给定了水深、海水速度、风速的取值范围,属于模型动态变化问题。
所以对模型进行了动态分析,求得钢桶、钢管的倾斜角度、锚链形状、浮标的吃水深度和游动区域的取值范围,进而分析水深、海水速度、风速对结果的影响,这有利于系泊系统的调整和应用。
本文所建立的模型对相关问题在理论上作了证明,虽然对部分模型进行了简化,但是实用性很强,而且易于推广,能够扩展到其他系泊系统。
- 1、下载文档前请自行甄别文档内容的完整性,平台不提供额外的编辑、内容补充、找答案等附加服务。
- 2、"仅部分预览"的文档,不可在线预览部分如存在完整性等问题,可反馈申请退款(可完整预览的文档不适用该条件!)。
- 3、如文档侵犯您的权益,请联系客服反馈,我们会尽快为您处理(人工客服工作时间:9:00-18:30)。
针对问题一,利用需求预测得到观看 DVD 的人数服从二项分布, 并计算出多种可靠度下购买 DVD 的数量(由问卷调查结果得到每种 DVD 被租赁的概率 p,应用二项分布,在多种可靠度下计算出了购买 DVD 的数量)。
山东大学控制科学与工程学院
2017/8/2 15
山东 大学
五、撰写论文需要重视的问题
摘要---摘要范例
本文针对出版社资源配置建立了相应的数学模型。根据出版社历年的统 计资料和问卷调查数据,以9个分社的72们课程为研究对象,利用线性回 归的方法预测2006年每种书号实际销售量,从均值角度统计其市场满意 度和市场占有率,并计算出书号申请计划的准确度。 从经济效益,市场占有率和市场满意度三方面来理解所谓的“强势产 品”,兼顾计划准确度建立了一个多目标整数规划模型。利用“极差标 准化法”和“指派方法”读经济效益指标和满意度指标进行了标准化处 理。最后禁止综合加权将多目标转化为单目标。以人力资源和书号申请 量为约束条件,引进“惩罚因子”和“平衡因子”对分社的申请量进行 调整,使决策更具有效性。利用LINGO软件求解,得到了总社明确的书 号配置方法,并分析了在资源配置中人力资源的关键性作用。针对市场 经济条件下出版社的发展趋势和该出版社所暴露出来的问题提出了一些 参考性建议。
• • • • 摘要应理解为详细摘要; 提纲挈领表达严谨、简捷; 思路清新格式符合规范; 严禁暴露身份。
清晰性
山东大学控制科学与工程学院
2017/8/2
11
山东 大学
a.文章结构
四、数学建模论文的文章结构
摘要 1.问题重述 2.模型的假设 3.符号说明 4.模型建立与求解 5.模型检验 6.模型的评价 参考文献 附录(计算框图,程序)
山东大学控制科学与工程学院
2017/8/2
13
山东 大学
五、撰写论文需要重视的问题
字数控制在A4纸1页之内 语言精简,用词准确 阐述细致具体的方法 列出主要结论 写出三至五个关键词
摘要------重中之重
山东大学控制科学与工程学院
2017/8/2
14
山东 大学
五、撰写论文需要重视的问题
摘要---摘要范例
• 1. 同一问题有多种模型可以选择时,不宜给出太多,一至二 个为宜。 • 2. 实际做,可以多个,写时选取最好的。其他模型可放在推 广讨论中,与前面作比较分析,切忌列出多个、结果又不好 的模型.
问题分析时…….
• 1. 阐述、分析建模构想和建模思路,列出关键步骤和要点; • 2. 分析要清晰,层次分明,条理清楚,逻辑性强; • 3. 尤其创新之处的陈述要斟酌,确切无误。
山东大学控制科学与工程学院
2017/8/2
20
山东 大学
3、 符号说明
五、撰写论文需要重视的问题
以表格的形式比较清楚,建议采用.
符号 • H • c • L
意义
单位
• 游标表示的油深 • 油浮子标杆距左侧油罐壁水平距离 • 罐体的水平长度
• mm • mm • mm
山东大学控制科学与工程学院
2017/8/2
山东大学控制科学与工程学院
2017/8/2 16
山东 大学
五、撰写论文需要重视的问题
摘要---摘要范例
白酒质量控制数学模型的研究与应用
采用 GC-MS 对同一档次不同批次酒样进行分析 , 建立该档次酒的标 准指纹图谱库 , 运用向量夹角余弦法建立待测 GC-MS 指纹图谱相似
度评价的数学模型,并通过比对实验评价了该数学模型的可行性。
山东大学控制科学与工程学院
2017/8/2
8
山东 大学
二、数学建模论文写作的重要性
1
论文是评定竞赛成绩高低及获奖级别的依据 培养科技写作能力的一种有效方法 对撰写毕业论文,撰写科技论文,科技报告、 课题项目申请等有帮助作用
2
3
山东大学控制科学与工程学院
2017/8/2
9
山东 大学
三、数学建模竞赛论文的评阅原则
结果表明 , 利用 GC-MS 建立的白酒指纹图谱能全面、稳定测定白酒 香味物质的组成情况 , 具有较强的特征性、典型性和唯一性 , 在一
定程度上反映出白酒的质量及其风格特点。利用向量夹角余弦法
对GC-MS技术获取白酒指纹图谱进行处理的数学模型能快速、准确 表达出白酒的质量及其风格特点 , 既能鉴定白酒的真伪 , 又能评价
山东大学控制科学与工程学院
2017/8/2
3
山东 大学
一、全国研究生数学建模竞赛简介
b、历届概况
1、南京师范大学 2004;
2、东南大学 2005;
3、同济大学 2006; 4、国防科技大学 2007;
5、第二炮兵工程学院 2008;
6、武汉大学 2009; 7、中山大学 2010;
8、东北大学 2011;
9、上海交通大学 2012
山东大学控制科学与工程学院
2017/8/2
4
山东 大学
一、全国研究生数学建模竞赛简介
c、历届赛题
2005年 A: Highway Traveling Time Estimate and Optimal Routing B:空中加油 C:城市交通管理中的出租车规划 D:仓库容量有限条件下的随机存贮管理
山东大学控制科学与工程学院
2017/8/2
7
山东 大学
一、全国研究生数学建模竞赛简介
c、历届赛题
2010年 A:确定肿瘤的重要基因信息--提取基因图谱信息方法的研究 B:与封堵溃口有关的重物落水后运动过程的数学建模 C:神经元的形态分类和识别 D:特殊工件磨削加工的数学建模
2011年 A:基于光的波粒二象性一种猜想的数学仿真 B:吸波材料与微波暗室问题的数学建模 C:小麦发育后期茎秆抗倒性的数学模型 D:房地产行业的数学建模
样品质量的相似性或稳定性,适合应用于白酒质量控制。
山东大学控制科学与工程学院
2017/8/2 17
山东 大学 1、问题重述
五、撰写论文需要重视的问题
1.1 问题背景
• 简单地说明问题的情景,说清事情的来龙去脉
1.2 目标任务
• 列出必要数据,提出要解决的问题,并给出 研究对象的关键信息的内容。
山东大学控制科学与工程学院
Click to add Title 撰写论文需要重视的问题
Click to add Title 论文格式要求
山东大学控制科学与工程学院
2017/8/2
2
山东 大学
一、全国研究生数学建模竞赛简介
a、全国研究生数学建模竞赛的由来
2003年,东南大学、南京大学、中国科技大学、合肥工业大学等 12 所高校研究生会联合发起 “南京及周边地区研究生数学建模”,有20 所学校、近200名研究生参加。 2004 年由包括山东大学等 26 所高校研究生院一致决定联合发起全 国部分高校研究生数学建模竞赛,成立了竞赛组织委员会和竞赛评审委 员会,制定了竞赛的章程和规则。 2008年初,教育部研究生司正式批示,将全国研究生数学建模创新 能力培养改革和举办全国研究生数学建模竞赛列入研究生创新教育计划 项目,对竞赛产生巨大的推动作用。
2017/8/2
18
山东 大学
五、撰写论文需要重视的问题
1
2
• 论文中的假设要以严格、确切的数学语言表达 • 所提出的假设为建立数学模型所必需的, 而不是与建立模型无关 • 假设应验证其合理性
• 合理性可以从分析问题过程中得出,例如从问题的性质 出发作出合乎常识的假设;或者由观察所给数据的图象, 得到变量的函数形式;也可以参考其他资料由类推得到, 但要指出参考文献的相关内容
以会员的最大满意度为目标函数,建立一个整数规划模型( 0 - 1 规划,利用lingo 软件求解),得到问题二(会员的)分配方案,并计 算出前30 位会员的分配结果。 在问题三中,我们考虑到60%的会员由于两次租赁而导致DVD可重 复利用,因而采用了两阶段购买的策略,在每个购买阶段都建立了双目 标整数规划,从而得到的购买量比原来网站拥有量小,并且会员的满意 度达到99.38%。 本文最后还给出了考虑归还 DVD 周期的情形下购买与分配的模型。
• • • • 方法有创造性 能用初等方法解决的、尽可能不用高级方法 能用简单方法解决的,尽可能不用复杂方法 能用被更多人看懂、理解的方法,尽可能不用只能少数人看 懂、理解的方法。
2017/8/2 24
山东大学控制科学与工程学院
山东 大学
五、撰写论文需要重视的问题
模型的建立与求解中应注意的问题
多个模型的取舍问题
山东大学控制科学与工程学院
2017/8/2
12
山东 大学
四、数学建模论文的文章结构
b.采用多层次式的标题
1 2 3
• 每个标题能够反映该标题下本节的主题 • 每段句首能够概括本段的主要内容;使评 阅人把握此文的脉络和要点,快速、准确 理解全文. • 凡有特色、有创新的建模方法、或求解方 法、或数据处理方法、或结果分析方法, 都可以在标题中明确的体现
2、模型的假设
3 4
5
• 主要假设以3~5条为宜
2017/8/2 19
山东大学控制科学与工程学院
山东 大学
五、撰写论文需要重视的问题 Nhomakorabea模型假设举例
1) 忽略温度对原油体积与对油罐壳体的影响; 2) 忽略液体的静压力作用会引起钢板油罐壳体的膨胀和收缩; 3) 假设本题所给数据均为在同一变位参数的情况下测得的,即 在测量的时候地下储油罐发生变位的可能性和程度极小,忽略不 计。