HypothesisTesting统计学假设检验
HypothesisTesting统计学假设检验
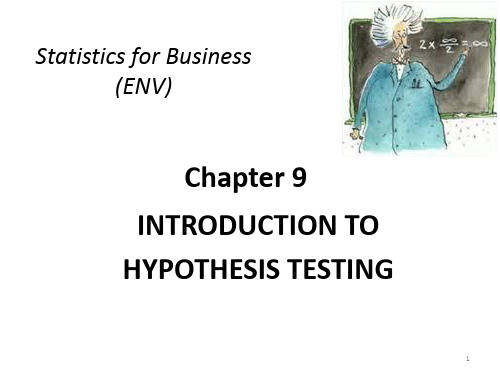
• These two hypotheses are mutually exclusive and exhaustive.
7
Determined by the level of significance or the alpha level
Step Two: Select a Level of Significance,
Level of Significance,
Measures the max probability of rejecting a true null hypothesis
and s).
With “=” sign Say, “ = 2” or “ 2”
Alternative Hypothesis H1: A statement that is accepted
if H0 is false
Without “=” sign Say, “ 2” or “ < 2”
17
Step 1: State the null and alternate hypotheses
4
The basic experimental situation for using hypothesis testing is presented here. It is assumed that the parameter is known for the population before treatment. The purpose of the experiment is to determine whether or not the treatment has an effect. Is the population mean after treatment the same as or different from the mean before treatment? A sample is selected from the treated population to help answer this question.
假设检验应用场景
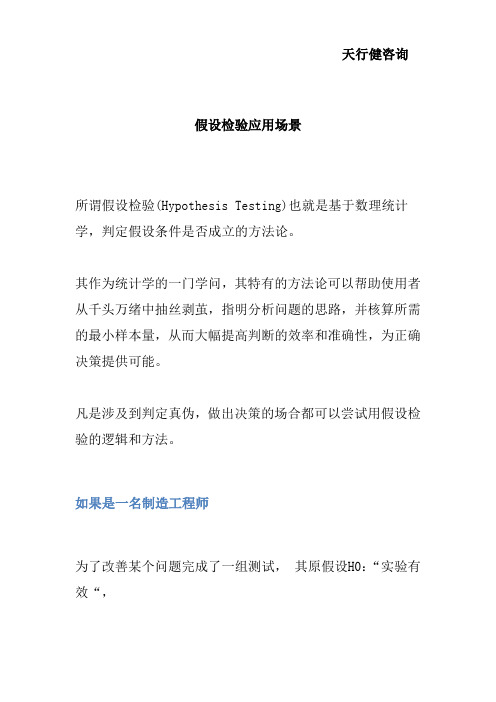
假设检验应用场景
所谓假设检验(Hypothesis Testing)也就是基于数理统计学,判定假设条件是否成立的方法论。
其作为统计学的一门学问,其特有的方法论可以帮助使用者从千头万绪中抽丝剥茧,指明分析问题的思路,并核算所需的最小样本量,从而大幅提高判断的效率和准确性,为正确决策提供可能。
凡是涉及到判定真伪,做出决策的场合都可以尝试用假设检验的逻辑和方法。
如果是一名制造工程师
为了改善某个问题完成了一组测试,其原假设H0:“实验有效“,
如果做出了错误的判断会导致:
I类错误
试验有效,但判定无效.造成错失改善机会.
均值不等,但判定相等.后果同上.
标准差不等但判定相等后果同上
II类错误
试验无效,但判定有效,造成无效的措施被采纳. 均值相等,但判定不等,后果同上.
标准差相等,但判定不等,后果同上.
管理者如何面对有疑问的说辞
如果是一名管理者面对有疑问的说辞:原假设是“相信此人是诚实/正确的”,
做出了错误的判断会导致:
I类错误
错过好的改善或者盈利的机会
II类错误
可能使得企业遭受或大或小的损失,随着企业对管理人员的
容错范围在收窄,对其职业生涯会产生直接影响。
这也是管理者一般不轻信别人的原因。
如果是一名法官
庭审上面对疑犯的原假设H0是“疑犯无罪”(注意律政的原则是疑罪从无),
做出了错误的判断会导致:
I类错误
清白的人进监狱,需要特别谨慎,一般选择5%
II类错误
罪犯逍遥法外,一般选择10%
这些就是假设检验的一般应用场合,更多请关注天行健咨询!。
第十七章 假设检验(hypothesis testing)

A B 40 73 69 182
非参数检验(nonparametric test )
概念:在总体分布不明确或明显偏离正态情况下,
对总体进行差异性推断的一种统计方法,其检验的
是分布,而非参数。
应用范围:
配对资料的秩和检验 两样本成组比较秩和检验 多样本比较的秩和检验 等级分组资料的秩和检验
定结果是否不同?
表2 两种方法对乳酸饮料中脂肪含量的测定结果(%)
编号
哥特里-罗紫法
脂肪酸水解法
1
0.840
2
0.591
3
0.674
4
0.632
5
0.687
6
0.978
7
0.750
8
0.730
9
1.200
10
0.870
0.580 0.509 0.500 0.316 0.337 0.517 0.454 0.512 0.997 0.506
两样本成组比较的秩和检验
例3 为了研究血铁蛋白与肺炎的关系,随机抽取了肺炎患 者和正常人若干名,测得血铁蛋白(μg/L),数据如下表, 请问两种人血铁蛋白总体分布是否相同?
cards; 68 100 83 101 69 120 100 180 110 100 180 240 55 120 200 170 210 300 120 105 ; proc univariate;
var x; run;
例2 用两种方法测定肺炎患者的尿铁蛋白,测量结果如下 表所示,试问,这两种方法测的结果是否有差别?
一、样本均数与总体均数差异的t检验 x
1.数学模型 t 2. SAS过程(编程) S X
用means过程,检验μd = μ- μ0 =0,其检 验相当于总体均数μ=μ0 。
假设检验(hypothesis testing)
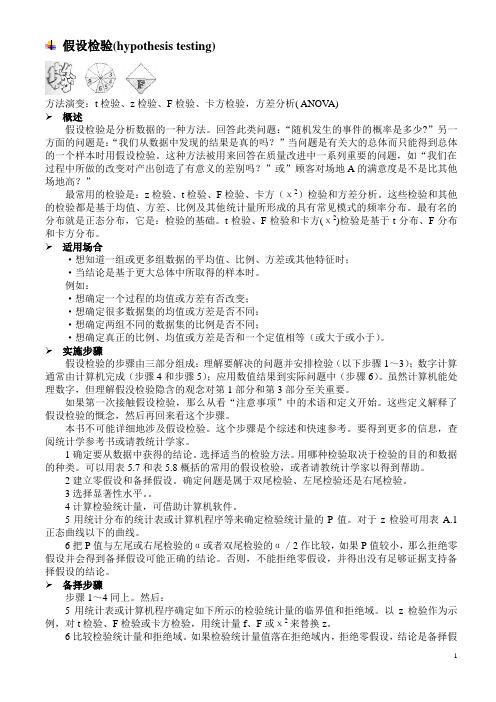
假设检验(hypothesis testing)方法演变:t检验、z检验、F检验、卡方检验,方差分析( ANOV A)➢概述假设检验是分析数据的一种方法。
回答此类问题:“随机发生的事件的概率是多少?”另一方面的问题是:“我们从数据中发现的结果是真的吗?”当问题是有关大的总体而只能得到总体的一个样本时用假设检验。
这种方法被用来回答在质量改进中一系列重要的问题,如“我们在过程中所做的改变对产出创造了有意义的差别吗?”或”顾客对场地A的满意度是不是比其他场地高?”最常用的检验是:z检验、t检验、F检验、卡方(χ2)检验和方差分析。
这些检验和其他的检验都是基于均值、方差、比例及其他统计量所形成的具有常见模式的频率分布。
最有名的分布就是正态分布,它是:检验的基础。
t检验、F检验和卡方(χ2)检验是基于t分布、F分布和卡方分布。
➢适用场合·想知道一组或更多组数据的平均值、比例、方差或其他特征时;·当结论是基于更大总体中所取得的样本时。
例如:·想确定一个过程的均值或方差有否改变;·想确定很多数据集的均值或方差是否不同:·想确定两组不同的数据集的比例是否不同;·想确定真正的比例、均值或方差是否和一个定值相等(或大于或小于)。
➢实施步骤假设检验的步骤由三部分组成:理解要解决的问题并安排检验(以下步骤1~3);数字计算通常由计算机完成(步骤4和步骤5);应用数值结果到实际问题中(步骤6)。
虽然计算机能处理数字,但理解假没检验隐含的观念对第1部分和第3部分至关重要。
如果第一次接触假设检验,那么从看“注意事项”中的术语和定义开始。
这些定义解释了假设检验的慨念,然后再回来看这个步骤。
本书不可能详细地涉及假设检验。
这个步骤是个综述和快速参考。
要得到更多的信息,查阅统计学参考书或请教统计学家。
1确定要从数据中获得的结论。
选择适当的检验方法。
用哪种检验取决于检验的目的和数据的种类。
《数理统计》第三章 假设检验
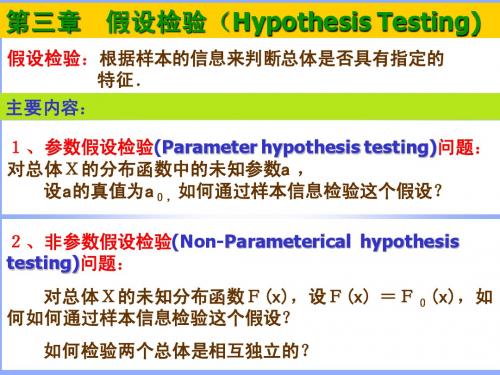
P328
P329
第三章 1.2 参数假设检验Parameter hypothesis testing
一个正态总体方差的假设检验
第三章 1.2 参数假设检验Parameter hypothesis testing
一个正态总体方差的假设检验
两个正态总体方差比的假设检验 两个正态总体方差比的假设检验 方差比
两个正态总体方差比的假设检验 两个正态总体方差比的假设检验 方差比
P393
P393
第三章 1.2 参数假设检验Parameter hypothesis testing
两个正态总体均值,方差的假设检验举例 两个正态总体均值,方差的假设检验举例
第三章 1.2 参数假设检验Parameter hypothesis testing
一个正态总体均值的假设检验( 检验 检验) 一个正态总体均值的假设检验(U检验)
第三章 1.2 参数假设检验Parameter hypothesis testing
一个正态总体均值的假设检验( 检验) 一个正态总体均值的假设检验(U检验)表示
两个正态总体均值差假设检验举例 两个正态总体均值差假设检验举例
第三章 1.2 参数假设检验Parameter hypothesis testing
两个正态总体均值差假设检验举例 两个正态总体均值差假设检验举例
两个正态总体方差比的假设检验
第三章 1.2 参数假设检验Parameter hypothesis testing
总体分布函数的假设检验
1.3 非参数假设检验(Non-Parameter hypothesis testing) 非参数假设检验 Parameter
HypothesisTesting(统计学假设检验)PPT课件

2
Hypothesis testing-1
Researchers usually collect data from a sample and then use the sample data to help answer questions about the population. Hypothesis testing is an inferential statistical process that uses limited information from the sample data as to reach a general conclusion about the population.
example, we might hypothesize that the mean IQ for UIC students is = 110.
2. Next, we obtain a random sample from the population. For example,
we might select a random sample of n = 100 UIC students.
4
The basic experimental situation for using hypothesis testing is presented here. It is assumed that the parameter is known for the population before treatment. The purpose of the experiment is to determine whether or not the treatment has an effect. Is the population mean after treatment the same as or different from the mean before treatment? A sample is selected from the treated population to help answer this question.
Chapter7假设检验Hypothesistesting.

Chapter 7 Hypothesis testingStatistical hypothesis testing is another core issue in statistical inference . Wecalled the thesis about population distribution the statistical assumptions in Mathematical Statistics . A statistical hypothesis is an assertion about the distribution of one or more random variables. A test of statistical hypothesis is a rule which, when the experimental sample values have been obtained, leads to a decision to accept or to reject the hypothesis under consideration. Hypothesis testing owns an important status both in theoretical research and practical applications . In this chapter we will introduce the basic concepts of hypothesis testing and the hypothesis testing methods in normal population station .§7.1 The basic concepts of hypothesis testingThe theses about the population distribution have two forms. One is the thesis aboutthe population distribution types, known as non-parametric hypothesis ; another is thatthe type of population distribution is known but with unknown parameters, we called the thesis about the unknown parameters parametric hypothesis. We will only introduce parametric hypothesis testing in this chapter .1 An exampleIn this section, we will introduce some important concepts th rough an example ofhypothesis test.Example 7.1 We selected 20 newborns randomly from a region in 2002, the average weight of them is 3160g, the sample standard deviation of the weigh t is 300g, and based on past statistics, the average weight of newborn is 3140g. If the weight of X obeys normal distribution. Is there any significant difference about the weight between the newborn in 2002 and the old ones?Let X denote the weight of newborn, then based on the hypothesis , wehave 2~(,)X N μσ.The problem is that whether the mean of population is equal to 3140g or not, which can be expressed as0:3140H μ=,This hypothesis is called zero hypotheses or the original hypothesis. If 0:3140H μ=is not correct, so 1:3140H μ≠ is correct. This hypothesis could be called the alternativehypothesis . The above hypothesis testing problem is often expressed as01:3140:3140H H μμ=↔≠.2 significant testAccording to statistics, in the past, the average weight of newborns is 3140g,while in 2002, the average weight of newborn samples is 3160g, a difference in 20g, this difference may arise in two situations. O ne is that there is no essential difference in them, the difference in 20g is only caused by the randomness of the sample; another is caused by the essential difference in them . So the point is whether the difference can be explained by the randomness of sample or not.The sample mean is a good estimation of the population mean , if 3140μ=,|3140|X -should be relatively small, we should establish a reasonable limit C , When|3140|X C -<,we accept the zero hypothesis ;Otherwise ,we will accept alternativehy pothesis .We know that~(1)X T t n =-,Setting α=0.01,0.005(||(1))0.01P T t n α≥-==, if the observation of ||T satisfies||t 0.005(1)t n ≥-,that is to say the small probability event 0.005{||(1)}T t n ≥- occur.Generally speaking, small probability events will not occur in one experiment, so we believe 0H is unreasonable, we call 0.005{||(1)}t t n ≥- critical region . Otherwise, we have not enough evidence to reject 0H ,so we accept 0H .Which is called significant test . In this example,0.00520,(19) 2.861,n t ==and 0.298 2.861,x t ==<So we cannot reject 0H ,thus wecan believe no significant difference between the newborn in 2002 and the old ones.Noticing 0.005||(1)T t n ≥-is equivalent to 0.005||(1)X t n S μ-≥-0.005(1)C t n S =-,Afterward, we can make judgment only by the sample value of ||T .3 Two types of errorsAs mentioned above, a significant test is based on the fact that small probability event will not occur in one experiment. However, small probability events still may occur, therefore using the above hypothesis testing method still may make wrong judgments, there are two situations:1. The original hypothesis is actually correct, but the test result wrongly reject it, which commit "abandoning true" errors, often referred to as type I error.2. The original hypothesis is not right, but the test result wrongly accept it, which commit "maintaining false " errors, often referred to as type II error.As the sample is random, so we are committing two types of errors on certain probability . In statistics, we call the probability of committing type I error the significance level , abbreviated as the level. Naturally, people desire the probability of committing two types of errors as small as possible, but for a given sample size, We can not reduce the probability of committing two types of errors simultaneously, Commonly, we often fix the upper bound of the probability of committing type I error , and then select a test with smaller probability of committing type II error .§7.2 single normal population hypothesis testingLet 1(,,)n X X denote a random sample from a normal distribution 2~(,)X N μσ,α issignificance level.1 Test of mean1.1 Variance knownThe problem as follows0010::H H μμμμ=↔≠When 0H is true, then~(0,1)X U N =,thus/2(||)P U u αα≥=,So the critical region is /2{||}u u α≥.Example 7.2 Let us assume 2(4.55,0.108)X N ,we have nine random samples,and themean is 4.484,suppose the variance have no change, testing the following problem with the significance level 0.05,α=01: 4.55: 4.55H H μμ=↔≠.0.025u =1.96, 0.108, 4.484,9x n σ===,we get4.484 4.55|||| 1.83 1.960.108/3u -==<,Then 0H can be accepted.1.2 Variance unknownThe problem as follows0010::H H μμμμ=↔≠,When 0H is true, then~(1)X T t n =-,Thus/2(||(1))P T t n αα≥-=,So the critical region is /2{||(1)}t t n α≥-.Example 7.3 Let us assume X have normal distribution,we have 25 random samples,and themean is 66.5,and the standard deviation of sample is 15, testing the following problem with the significance level 0.05,α=01:70:70H H μμ=↔≠.25n =,0.025(24)t =2.06,66.5x =,we get66.570|||| 1.167 2.0615/5t -==<,Then 0H can be accepted.There are still two other kinds of problem of testing mean as follows0010::H H μμμμ=↔>; 0010::H H μμμμ=↔<.Using similar methods above to discuss,we can get the result in table 7.12 Test of varianceThe problem as follows22220010::H H σσσσ=↔≠,When 0H is true, then2222(1)~(1)n S n χχσ-=-,thus22221/2/2((1)(1))P n n ααχχχχα-≤-≥-=或,So the critical region is 22221/2/2{(1)}{(1)}n n ααχχχχ-≤-≥-.Example 7.4 Let us assume X have normal distribution,we have 5 random samples,1.32, 1.55, 1.36, 1.40, 1.44,and the standard deviation is 0.048σ=, testing the following problem with the significance level 0.05,α=222201:0.048:0.048H H σσ=↔≠.5n =,220.0250.975(4)11.14,(4)0.484χχ==,Fromthe givensample,we have20.00778S =,hence2240.0077813.5111.140.048χ⨯==>,So we reject 0H .There are still two other kinds of problem of testing variance as follows22220010::H H σσσσ=↔>; 22220010::H H σσσσ=↔<.Using similar methods above to discuss,we can get the result in table 7.2§7.3 double normal population hypothesis testingLet 112(,,...,)n X X X denote a random sample of size 1n from a distribution that is211(,),N μσ 212(,,...,)n Y Y Y denote a random sample of size 2n from a distributionthat is 222(,),N μσwhere 211,μσ ,222,μσ are unknown parameters, the two random samples are independent1.2212σσ=, testing of 12μμ-The problem as follows012112::H a H a μμμμ-=↔-≠,When 0H is true, then12~(2)X Y T t n n =+-,Thus/212(||(2))P T t n n αα≥+-=,So the critical region is /212{||(2)}T t n n α≥+-.Example 7.4 Let us assume X and Y both have normal distribution with equalvariances,we have the following random samples,X 24.3, 20.8, 23.7, 21.3, 17.4; Y 18.2, 16.9, 20.2, 16.7.testing the following problem with the significance level 0.05,α=012112::H H μμμμ=↔≠.0a =.125,4n n ==,0.025(7) 2.365t =,221221.5,7.505,18, 2.593X S Y S ====,hence2.324S ω==,| 2.245 2.365|T =<=,So we accept 0H .Using similar methods above to discuss the following problems012112::H a H a μμμμ-≤↔->; 012112::H a H a μμμμ-≥↔-<.we can get the result in table 7.3.2 testing of 2212/σσThe problem as follows2222012112::H H σσσσ=↔≠,When 0H is true, then221212(1,1)F S S F n n =--,hence1212212((1,1)(1,1))P F F n n F F n n ααα-≤--≥--=或,So the critical region is 112212{(1,1)(1,1)}F F n n F F n n αα-≤--≥--或. Using similar methods above to discuss the following problems,2222012112::H H σσσσ≤↔>,we can get the result in table 7.4.1(1,F n -1(1,F n -Example 7.5 There are two team A and B participate a paper contest, A team have 9 people, andB team have 8 people, the score as follows:A team 85, 59, 66, 81, 35, 57, 76, 63, 78,B team 65, 72, 69, 65, 58, 68, 52, 64,Can we believe the variance of B team is significantly greater than A team ’s concerning the significance level 0.05?Testing the following problem2222012112::,H H σσσσ≤↔>0.025(8,7) 3.73F =,so the critical region is { 3.73}F ≥,from the given sample we have2212240.75,40.98S S ==,hence21225.875 3.73S F S ==>,So we can believe the variance of B team is significantly greater than A team ’s.Exercises1.Let us assume that the life of a tire in mile, say X ,is normally distributed with mean θ and standard deviation 5000.Past experience indicates that 3000θ=.The manufacturer claims that the tires made by a new process have mean 3000θ>,we observe 10 independent values of X ,the sample mean is 5100,under the significance level 0.05,can we believe the manufacturer ’s claim?2. Let us assume the temperature of certain substance is normally distributed, we have 5 sample values:1250, 1265, 1245, 1260, 1275,Can we believe the temperature of this substance is 1277?(α=0.05).3.Let us assume that the life of a electronic product is normally distributed, and standard deviation 1.6.Now the technique is improved, we draw 9 products in the new products, the mean life is 52.8,sample standard deviation is 1.19,can we believe the variance of the life is changed? (α=0.05).4. Seeds of a particular variety of plant were randomly assigned to either a nutritionally rich environment (the treatment) or standard conditions (the control). After a predetermined period, allProviding that the two sample are from normal population, concluding that is there any difference between the mean weights due to environmental conditions? (α=0.05) 5.Let population (,9)XN μ,μ is unknown parameter, 125(,,)X X is a samplefrom population, considering the testing problem:0010::H H μμμμ=↔≠,Critical region is 1250{(,,)|||}C x x x c μ=-≥.Please find the constant c ,thesignificance level is 0.05.。
六西格玛工具HypothesisTest假设检验完整版
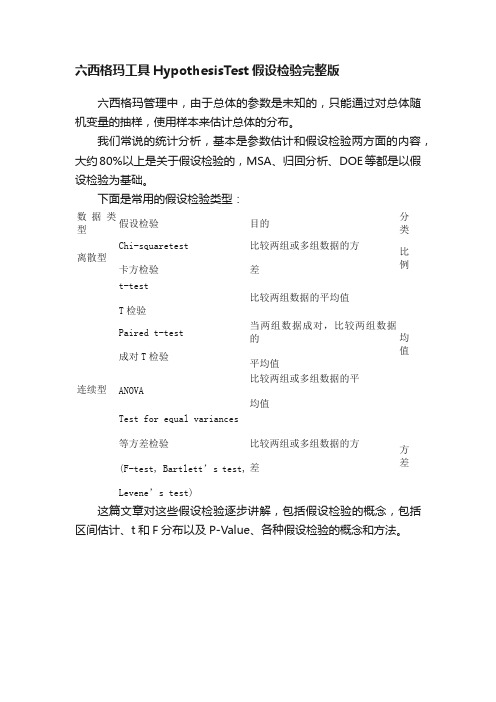
六西格玛工具HypothesisTest假设检验完整版
六西格玛管理中,由于总体的参数是未知的,只能通过对总体随机变量的抽样,使用样本来估计总体的分布。
我们常说的统计分析,基本是参数估计和假设检验两方面的内容,大约80%以上是关于假设检验的,MSA、归回分析、DOE等都是以假设检验为基础。
下面是常用的假设检验类型:
数据类型假设检验目的
分
类
离散型
Chi-squaretest
卡方检验比较两组或多组数据的方
差
比
例
连续型t-test
T检验
比较两组数据的平均值
均
值Paired t-test
成对T检验
当两组数据成对,比较两组数据
的
平均值
ANOVA
比较两组或多组数据的平
均值
Test for equal variances
等方差检验
(F-test, Bartlett’s test,
Levene’s test)
比较两组或多组数据的方
差
方
差
这篇文章对这些假设检验逐步讲解,包括假设检验的概念,包括区间估计、t和F分布以及P-Value、各种假设检验的概念和方法。
Hypothesis-Testing(统计学假设检验).ppt

Null and Alternative Hypotheses
• The null hypothesis, denoted H0, is a statement of the basic proposition being tested. It generally represents the status
quo (a statement of “no effect” or “no difference”, or a statement of equality) and is not rejected unless there is convincing sample evidence that it is false.
9.2 z Tests about a Population with known s 9.3 t Tests about a Population with unknown s
2
Hypothesis testing-1
Researchers usually collect data from a sample and then use the sample data to help answer questions about the population. Hypothesis testing is an inferential statistical process that uses limited information from the sample data as to reach a general conclusion about the population.
3. Finally, we compare the sample data with the hypothesis. If the data are consistent with the hypothesis, we will conclude that the hypothesis is reasonable. But if there is a big discrepancy between the data and the hypothesis, we will decide that the hypothesis is wrong.
假设检验

例2:某种零件的尺寸,要求其平均长度为4厘米,大于或小于4 厘米均属于不合格。该企业生产的零件平均长度是4厘米吗?
提出原假设 H0: = 4厘米
提出备择假设 H1: 4厘米
单边检验
例1:某灯泡制造商声称,该企业所生产的灯泡的平均使用 寿命在1000小时以上。该批产品的平均使用寿命超过1000小 时吗?
x 0 t ~ t (n 1) s n
正态总体、方差未知、小样本情况下,样本统计量的抽样分布
t
正态 分布
X S n
~ t (n 1)
正态分布 t (df = 13) t (df = 5)
t 分布
Z
X
t 分布与正态分布的比较
不同自由度的t分布
t
总体均值的检验—— t 检验(双边)
提出原假设H0: 1000 选择备择假设 H1: < 1000
例2:学生中通宵上网的人数超过25%吗?
提出原假设H0: 25%
选择备择假设 H1: 25%
例3:消费者协会接到消费者投诉,指控某品牌纸包装饮料 容量不足,有欺骗消费者之嫌。消费者协会从市场上随机抽 取50盒该品牌纸包装饮品,包装上标明的容量为250毫升, 但测试发现平均含量为248毫升,小于250毫升。这是生产中 正常的波动,还是厂商的有意行为?消费者协会能否根据该 样本数据,判定饮料厂商欺骗了消费者呢?
2 2
Z 1.96
2
决策准则
当 Z Z ,即Z Z 或Z Z 时 拒绝H 0
2 2 2
当 Z Z ,即 Z Z Z 时 接受H 0
统计-假设检验
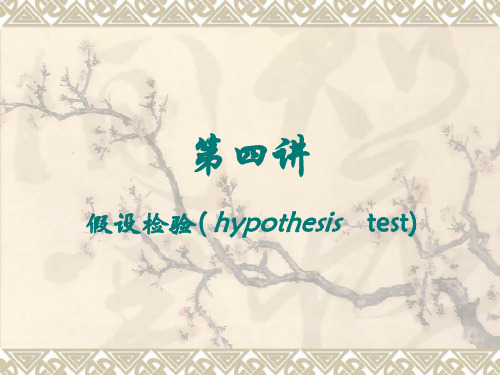
假设
一种是原假设(或零假设),通常是“相等性假
设”,例如假定总体均值等于μ ,总体方差等于 σ ,总体分布为标准正态分布等,记为H0; 另一种是在原假设被拒绝后可供选择的假设,称 为备择假设,记为H1。备择假设H1是和原假设H0 不相容的。
7
两均数比较的t检验
应用条件
两组计量资料差异比较
第四讲
假设检验( hypothesis test)
讲解内容
假设检验的概念 假设检验的思想 假设检验的步骤
假设检验的方法
两均数比较的t检验 两均数比较的u检验
假设检验注意的问题
2
假设检验的概念
由于个体差异的存在,即使从同一总体中严格的随机抽样,
X1, X 2 , X 3 , X 4 ,... 不同。
•先作方差齐性检验
•作 t 或 t 检验 (根据 P 值)
23
方差齐性检验
H0:两总体方差齐 H1:两总体方差不齐
计算F值
F
2 S1 较大 2 S2 较小
2 1.79 0.562
10.22
查附表2 (方差分析表,方差齐性检验用)F0.05(9,49) =2.39 因为F 大于F0.05(9,49) 所以 P<0.05,拒绝H0 。 认为因为两总体方差的差异有统计学意义,故不 宜用 t 检验而要用 t 检验或非参数检验。
配对设计
将条件相同或相近的两个受试对象配成对子,再
将每对中的两个受试对象随机分配到不同处理组 同一受试对象分别接受两种不同的处理 同一受试对象处理前后比较
推断目的:差值d的总体均数是否为0 应用条件:要求差值d服从正态分布
什么是假设检验
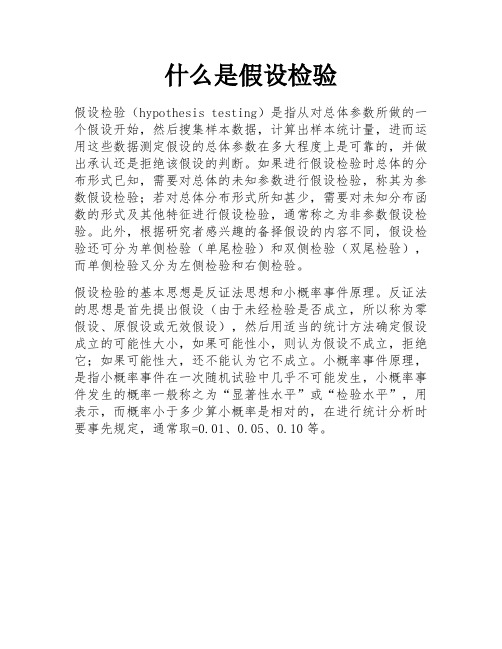
什么是假设检验
假设检验(hypothesis testing)是指从对总体参数所做的一个假设开始,然后搜集样本数据,计算出样本统计量,进而运用这些数据测定假设的总体参数在多大程度上是可靠的,并做出承认还是拒绝该假设的判断。
如果进行假设检验时总体的分布形式已知,需要对总体的未知参数进行假设检验,称其为参数假设检验;若对总体分布形式所知甚少,需要对未知分布函数的形式及其他特征进行假设检验,通常称之为非参数假设检验。
此外,根据研究者感兴趣的备择假设的内容不同,假设检验还可分为单侧检验(单尾检验)和双侧检验(双尾检验),而单侧检验又分为左侧检验和右侧检验。
假设检验的基本思想是反证法思想和小概率事件原理。
反证法的思想是首先提出假设(由于未经检验是否成立,所以称为零假设、原假设或无效假设),然后用适当的统计方法确定假设成立的可能性大小,如果可能性小,则认为假设不成立,拒绝它;如果可能性大,还不能认为它不成立。
小概率事件原理,是指小概率事件在一次随机试验中几乎不可能发生,小概率事件发生的概率一般称之为“显著性水平”或“检验水平”,用表示,而概率小于多少算小概率是相对的,在进行统计分析时要事先规定,通常取=0.01、0.05、0.10等。
最常用的统计学分析方法--假设检验
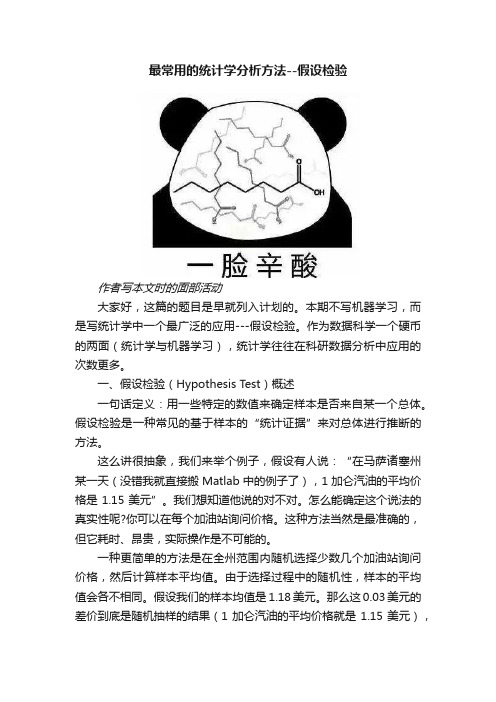
最常用的统计学分析方法--假设检验作者写本文时的面部活动大家好,这篇的题目是早就列入计划的。
本期不写机器学习,而是写统计学中一个最广泛的应用---假设检验。
作为数据科学一个硬币的两面(统计学与机器学习),统计学往往在科研数据分析中应用的次数更多。
一、假设检验(Hypothesis Test)概述一句话定义:用一些特定的数值来确定样本是否来自某一个总体。
假设检验是一种常见的基于样本的“统计证据”来对总体进行推断的方法。
这么讲很抽象,我们来举个例子,假设有人说:“在马萨诸塞州某一天(没错我就直接搬Matlab中的例子了),1加仑汽油的平均价格是1.15美元”。
我们想知道他说的对不对。
怎么能确定这个说法的真实性呢?你可以在每个加油站询问价格。
这种方法当然是最准确的,但它耗时、昂贵,实际操作是不可能的。
一种更简单的方法是在全州范围内随机选择少数几个加油站询问价格,然后计算样本平均值。
由于选择过程中的随机性,样本的平均值会各不相同。
假设我们的样本均值是1.18美元。
那么这0.03美元的差价到底是随机抽样的结果(1加仑汽油的平均价格就是1.15美元),还是1加仑汽油的平均价格实际上大于1.15美元的重要证据?此时就可以用假设检验的方法,用于做出此类决策。
假设检验有很多不同种类,不同的假设检验对数据中被抽样的随机变量的分布做出不同的假设(都有哪些假设后面讲)。
而在选择方法时,必须考虑这些假设。
所有的假设检验都有相同的基本术语和结构。
1.零假设:也称为原假设,是关于你想检验的总体的某一种判断。
它在某种意义上是“无效”的,因为它通常代表着一种“现状”。
它通过“断言”一个总体参数或总体参数的组合具有一定的值来形式化。
在我们的例子中,零假设是“整个州的平均汽油价格就是1.15美元”。
零假设写作H0,那么H0:µ=1.15。
2.备择假设:是一种与原假设相反的关于总体的断言。
在我们的例子中,可能的备择假设有:H1:µ≠1.15 即州平均价格不是1.15美元(对应双尾检验)H1:µ>1.15 -即州平均价格大于1.15美元(对应右尾检验)H1:µ<1.15 -即州平均价格小于1.15美元(对应左尾检验)从这里面选一个,作为你的备择假设。
hypothesis test
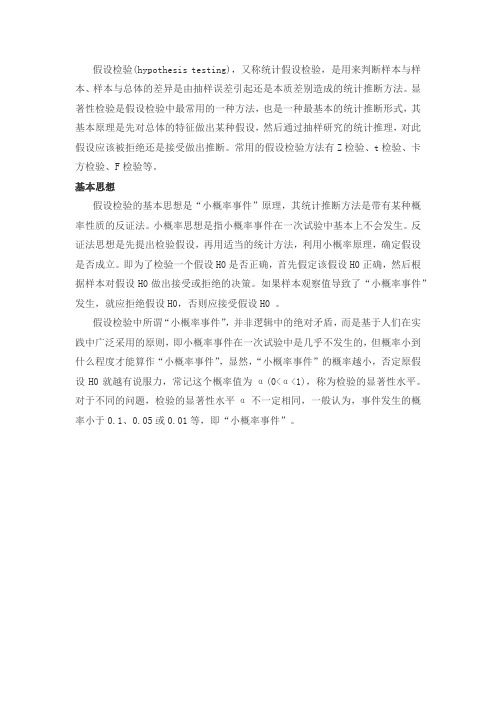
假设检验(hypothesis testing),又称统计假设检验,是用来判断样本与样本、样本与总体的差异是由抽样误差引起还是本质差别造成的统计推断方法。
显著性检验是假设检验中最常用的一种方法,也是一种最基本的统计推断形式,其基本原理是先对总体的特征做出某种假设,然后通过抽样研究的统计推理,对此假设应该被拒绝还是接受做出推断。
常用的假设检验方法有Z检验、t检验、卡方检验、F检验等。
基本思想
假设检验的基本思想是“小概率事件”原理,其统计推断方法是带有某种概率性质的反证法。
小概率思想是指小概率事件在一次试验中基本上不会发生。
反证法思想是先提出检验假设,再用适当的统计方法,利用小概率原理,确定假设是否成立。
即为了检验一个假设H0是否正确,首先假定该假设H0正确,然后根据样本对假设H0做出接受或拒绝的决策。
如果样本观察值导致了“小概率事件”发生,就应拒绝假设H0,否则应接受假设H0 。
假设检验中所谓“小概率事件”,并非逻辑中的绝对矛盾,而是基于人们在实践中广泛采用的原则,即小概率事件在一次试验中是几乎不发生的,但概率小到什么程度才能算作“小概率事件”,显然,“小概率事件”的概率越小,否定原假设H0就越有说服力,常记这个概率值为α(0<α<1),称为检验的显著性水平。
对于不同的问题,检验的显著性水平α不一定相同,一般认为,事件发生的概率小于0.1、0.05或0.01等,即“小概率事件”。
假设检验(HypothesisTesting)
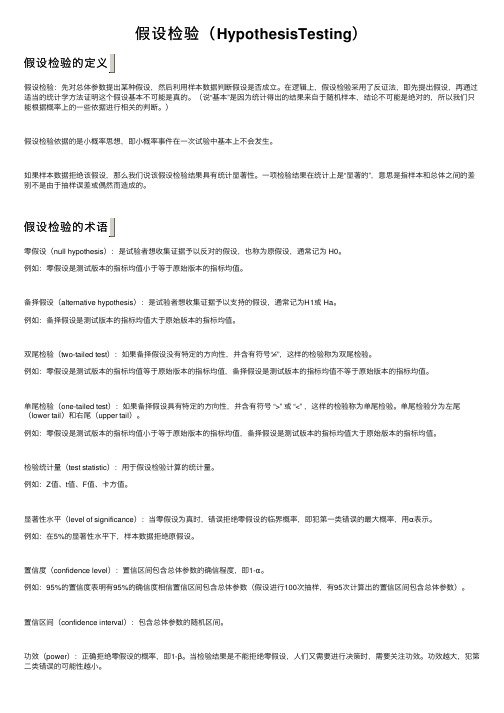
假设检验(HypothesisTesting)假设检验的定义假设检验:先对总体参数提出某种假设,然后利⽤样本数据判断假设是否成⽴。
在逻辑上,假设检验采⽤了反证法,即先提出假设,再通过适当的统计学⽅法证明这个假设基本不可能是真的。
(说“基本”是因为统计得出的结果来⾃于随机样本,结论不可能是绝对的,所以我们只能根据概率上的⼀些依据进⾏相关的判断。
)假设检验依据的是⼩概率思想,即⼩概率事件在⼀次试验中基本上不会发⽣。
如果样本数据拒绝该假设,那么我们说该假设检验结果具有统计显著性。
⼀项检验结果在统计上是“显著的”,意思是指样本和总体之间的差别不是由于抽样误差或偶然⽽造成的。
假设检验的术语零假设(null hypothesis):是试验者想收集证据予以反对的假设,也称为原假设,通常记为 H0。
例如:零假设是测试版本的指标均值⼩于等于原始版本的指标均值。
备择假设(alternative hypothesis):是试验者想收集证据予以⽀持的假设,通常记为H1或 Ha。
例如:备择假设是测试版本的指标均值⼤于原始版本的指标均值。
双尾检验(two-tailed test):如果备择假设没有特定的⽅向性,并含有符号“=”,这样的检验称为双尾检验。
例如:零假设是测试版本的指标均值等于原始版本的指标均值,备择假设是测试版本的指标均值不等于原始版本的指标均值。
单尾检验(one-tailed test):如果备择假设具有特定的⽅向性,并含有符号 “>” 或 “<” ,这样的检验称为单尾检验。
单尾检验分为左尾(lower tail)和右尾(upper tail)。
例如:零假设是测试版本的指标均值⼩于等于原始版本的指标均值,备择假设是测试版本的指标均值⼤于原始版本的指标均值。
检验统计量(test statistic):⽤于假设检验计算的统计量。
例如:Z值、t值、F值、卡⽅值。
显著性⽔平(level of significance):当零假设为真时,错误拒绝零假设的临界概率,即犯第⼀类错误的最⼤概率,⽤α表⽰。
假设检验名词解释
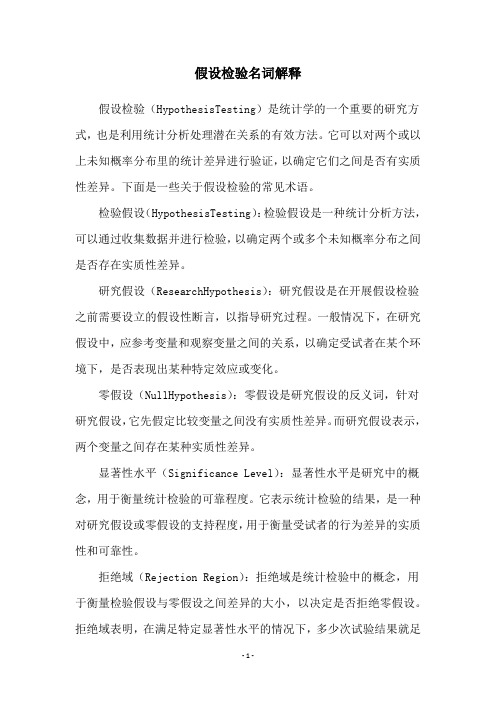
假设检验名词解释假设检验(HypothesisTesting)是统计学的一个重要的研究方式,也是利用统计分析处理潜在关系的有效方法。
它可以对两个或以上未知概率分布里的统计差异进行验证,以确定它们之间是否有实质性差异。
下面是一些关于假设检验的常见术语。
检验假设(HypothesisTesting):检验假设是一种统计分析方法,可以通过收集数据并进行检验,以确定两个或多个未知概率分布之间是否存在实质性差异。
研究假设(ResearchHypothesis):研究假设是在开展假设检验之前需要设立的假设性断言,以指导研究过程。
一般情况下,在研究假设中,应参考变量和观察变量之间的关系,以确定受试者在某个环境下,是否表现出某种特定效应或变化。
零假设(NullHypothesis):零假设是研究假设的反义词,针对研究假设,它先假定比较变量之间没有实质性差异。
而研究假设表示,两个变量之间存在某种实质性差异。
显著性水平(Significance Level):显著性水平是研究中的概念,用于衡量统计检验的可靠程度。
它表示统计检验的结果,是一种对研究假设或零假设的支持程度,用于衡量受试者的行为差异的实质性和可靠性。
拒绝域(Rejection Region):拒绝域是统计检验中的概念,用于衡量检验假设与零假设之间差异的大小,以决定是否拒绝零假设。
拒绝域表明,在满足特定显著性水平的情况下,多少次试验结果就足以表明两个变量之间存在某种实质差异。
样本大小(SampleSize):样本大小是指在进行统计检验时,受试者的数量。
样本越大,获得更多有意义结论的可能性就越大,但是样本越大,所需时间就越长。
p值(pValue):p值是一个概念,用于衡量统计检验结果的可靠性,它表示有多少可能性发生统计检验中参与变量之间存在的差异是由于随机性,而不是真实差异。
p值用于确定零假设是否应被拒绝,只有当p值小于显著性水平,才能够拒绝零假设。
假设检验是一种有效的统计分析方法,在决策过程中有许多应用,比如市场营销决策、投资决策、政策决策等。
研究生统计学教案:假设检验
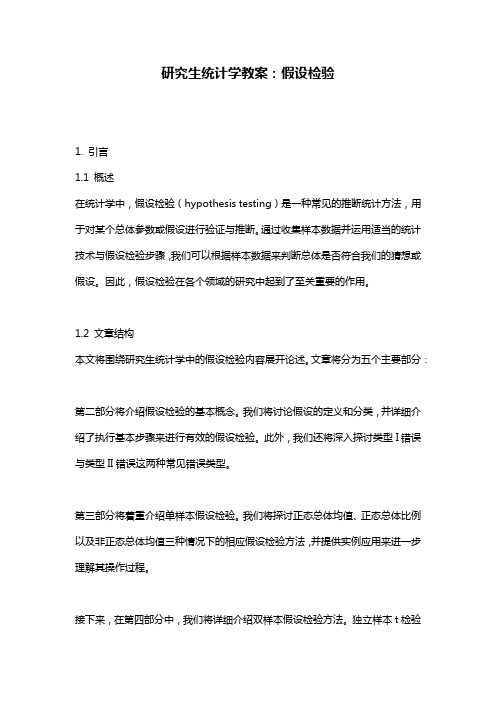
研究生统计学教案:假设检验1. 引言1.1 概述在统计学中,假设检验(hypothesis testing)是一种常见的推断统计方法,用于对某个总体参数或假设进行验证与推断。
通过收集样本数据并运用适当的统计技术与假设检验步骤,我们可以根据样本数据来判断总体是否符合我们的猜想或假设。
因此,假设检验在各个领域的研究中起到了至关重要的作用。
1.2 文章结构本文将围绕研究生统计学中的假设检验内容展开论述。
文章将分为五个主要部分:第二部分将介绍假设检验的基本概念。
我们将讨论假设的定义和分类,并详细介绍了执行基本步骤来进行有效的假设检验。
此外,我们还将深入探讨类型I错误与类型II错误这两种常见错误类型。
第三部分将着重介绍单样本假设检验。
我们将探讨正态总体均值、正态总体比例以及非正态总体均值三种情况下的相应假设检验方法,并提供实例应用来进一步理解其操作过程。
接下来,在第四部分中,我们将详细介绍双样本假设检验方法。
独立样本t检验与成对样本t检验分别针对两个独立样本和配对样本的假设检验进行讨论,同时也会涉及到非参数方法的应用。
最后,在第五部分,我们将总结前述的重要观点,并回顾文章中所探讨的内容。
此外,我们还将提出对该教案的改进和展望,以便在今后的学习中进一步完善相关的统计学知识。
1.3 目的通过本文,读者将能够全面了解研究生统计学中与假设检验相关的知识与技巧。
我们将深入讲解基本概念、步骤和错误类型,并提供具体实例来帮助读者更好地理解和应用这一研究方法。
希望通过阅读本文,读者能够在统计分析中准确运用假设检验并获得可靠推断结果,从而为其学术研究或实际问题提供有力支持。
2. 假设检验的基本概念2.1 假设的定义和分类在统计学中,假设是对总体或样本的某种特征所作出的陈述或主张。
根据提出假设的性质及其内容,可以将假设分为两类:原假设(H0)与备择假设(H1)。
原假设是关于总体参数或分布性质的一个主张,而备择假设则是对原假设提出的另一种可能性进行陈述。
- 1、下载文档前请自行甄别文档内容的完整性,平台不提供额外的编辑、内容补充、找答案等附加服务。
- 2、"仅部分预览"的文档,不可在线预览部分如存在完整性等问题,可反馈申请退款(可完整预览的文档不适用该条件!)。
- 3、如文档侵犯您的权益,请联系客服反馈,我们会尽快为您处理(人工客服工作时间:9:00-18:30)。
Hypothesis Testing
Step 1: State null and alternate hypotheses
Step 2: Select a level of significance
Step 3: Identify the test statistic
Step 4: Formulate a decision rule
3. Set the decision criteria by locating the critical region
13
Alpha level of .05 -- the probability of rejecting the null hypothesis when it is true is no more than 5%.
Statistics for Business (ENV)
Chapter 9 INTRODUCTION TO HYPOTHESIS TESTING
1
Hypothesis Testing
9.1 Null and Alternative Hypotheses and Errors in Testing
9.2 z Tests about a Population with known s 9.3 t Tests about a Population with unknown
s
2
Hypothesis testing-1
Researchers usually collect data from a sample and then use the sample data to help answer questions about the population. Hypothesis testing is an inferential statistical process that uses limited information from the sample data as to reach a general conclusion about the population.
we might hypothesize that the mean IQ for UIC students is = 110.
2. Next, we obtain a random sample from the population. For example,
we might select a random sample of n = 100 UIC students.
Step Two: Select a Level of Significance,
Level of Significance,
Measures the max probability of rejecting a true null hypothesis
4
The basic experimental situation for using hypothesis testing is presented here. It is assumed that the parameter is known for the population before treatment. The purpose of the experiment is to determine whether or not the treatment has an effect. Is the population mean after treatment the same as or different from the mean before treatment? A sample is selected from the treated population to help answer this question.
6
Null and Alternative
Hypotheses
• The null hypothesis, denoted H0, is a statement of the basic proposition being tested. It generally represents the status quo (a statement
11
H0 : µ=18
12
1. State the hypotheses The null hypothesis states that exposure to alcohol has no effect on birth weight. The alternative hypothesis states that alcohol exposure does affect birth weight.
and s).
With “=” sign Say, “ = 2” or “ 2”
Alternative Hypothesis H1: A statement that is accepted
if H0 is false
Without “=” sign Say, “ 2” or “ < 2”
17
Step 1: State the null and alternate hypotheses
Three possibilities
regarding means
H0: = 0 H1: = 0
a constant
H0: < 0 H1: > 0
The null hypothesis
always
contains
H0: > 0 H1: < 0
equality.
3 hypotheses about means 18
3
Hypothesis testing-2
• A hypothesis test is a formalized procedure that follows a standard series of operations.
• In this way, researchers have a standardized method for evaluating the results of their research studample, arrive at a decision
Do not reject null
Reject null and accept alternate
Step 1: State the null and alternate hypotheses
Null Hypothesis H0: A statement about the value of a population parameter (
of “no effect” or “no difference”, or a statement of equality) and is not rejected unless there is convincing sample evidence that it is false.
• The (scientific or) alternative hypothesis, denoted Ha (or H1) , is an alternative (to the null hypothesis) statement that will be accepted
2. Select the Level of Significance (alpha) level We will use an alpha level of .05. That is, we are taking a 5% risk of committing a Type I error, or, the probability of rejecting the null hypothesis when it is true is no more than 5%.
10
Example: Alcohol appears to be involved in a variety of birth defects, including low birth weight and retarded growth. A researcher would like to investigate the effect of prenatal alcohol on birth weight. A random sample of n = 16 pregnant rats is obtained. The mother rats are given daily doses of alcohol. At birth, one pup is selected from each litter to produce a sample of n = 16 newborn rats. The average weight for the sample is 15 grams. The researcher would like to compare the sample with the general population of rats. It is known that regular newborn rats (not exposed to alcohol) have an average weight of m = 18 grams. The distribution of weights is normal with sd = 4.
8
Z
Alpha level of .05 -- the probability of rejecting the null hypothesis when it is true is no more than 5%.
9
The locations of the critical region boundaries for three different levels of significance