An Empirical Investigation of the Impact of Discretization on Common Data Distributions
执业医师资格分阶段考试实证分析
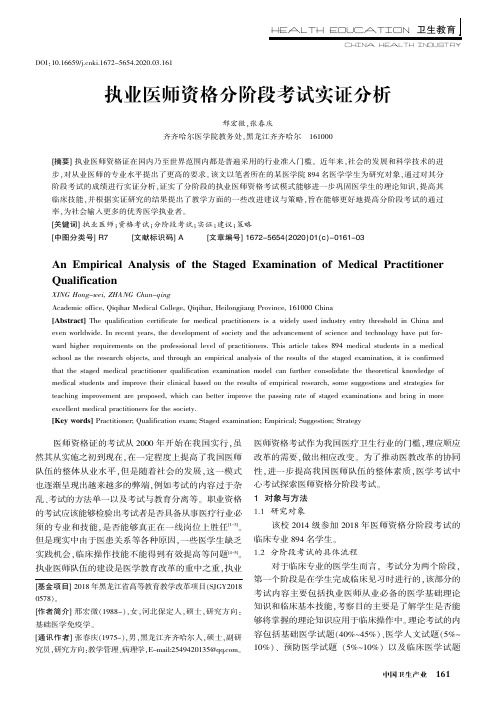
中国卫生产业CHINA HEALTHINDUSTRY[基金项目]2018年黑龙江省高等教育教学改革项目(SJGY20180578)。
[作者简介]邢宏微(1988-),女,河北保定人,硕士,研究方向:基础医学免疫学。
[通讯作者]张春庆(1975-),男,黑龙江齐齐哈尔人,硕士,副研究员,研究方向:教学管理、病理学,E-mail:*****************。
医师资格证的考试从2000年开始在我国实行,虽然其从实施之初到现在,在一定程度上提高了我国医师队伍的整体从业水平,但是随着社会的发展,这一模式也逐渐呈现出越来越多的弊端,例如考试的内容过于杂乱、考试的方法单一以及考试与教育分离等。
职业资格的考试应该能够检验出考试者是否具备从事医疗行业必须的专业和技能,是否能够真正在一线岗位上胜任[1-3]。
但是现实中由于医患关系等各种原因,一些医学生缺乏实践机会,临床操作技能不能得到有效提高等问题[4-5]。
执业医师队伍的建设是医学教育改革的重中之重,执业医师资格考试作为我国医疗卫生行业的门槛,理应顺应改革的需要,做出相应改变。
为了推动医教改革的协同性,进一步提高我国医师队伍的整体素质,医学考试中心考试探索医师资格分阶段考试。
1对象与方法1.1研究对象该校2014级参加2018年医师资格分阶段考试的临床专业894名学生。
1.2分阶段考试的具体流程对于临床专业的医学生而言,考试分为两个阶段,第一个阶段是在学生完成临床见习时进行的,该部分的考试内容主要包括执业医师从业必备的医学基础理论知识和临床基本技能,考察目的主要是了解学生是否能够将掌握的理论知识应用于临床操作中。
理论考试的内容包括基础医学试题(40%~45%)、医学人文试题(5%~10%)、预防医学试题(5%~10%)以及临床医学试题DOI:10.16659/ki.1672-5654.2020.03.161执业医师资格分阶段考试实证分析邢宏微,张春庆齐齐哈尔医学院教务处,黑龙江齐齐哈尔161000[摘要]执业医师资格证在国内乃至世界范围内都是普遍采用的行业准入门槛。
intellectual capital

Analysing value added as an indicator of intellectual capital and its consequences on company performanceDaniel Ze´ghal and Anis Maaloul CGA –Accounting Research Centre,Telfer School of Management,University of Ottawa,Ottawa,CanadaAbstractPurpose –The purpose of this paper is to analyse the role of value added (VA)as an indicator of intellectual capital (IC),and its impact on the firm’s economic,financial and stock market performance.Design/methodology/approach –The value added intellectual coefficient (VAIC e )method is used on 300UK companies divided into three groups of industries:high-tech,traditional and services.Data require to calculate VAIC e method are obtained from the “Value Added Scoreboard”provided by the UK Department of Trade and Industry (DTI).Empirical analysis is conducted using correlation and linear multiple regression analysis.Findings –The results show that companies’IC has a positive impact on economic and financial performance.However,the association between IC and stock market performance is only significant for high-tech industries.The results also indicate that capital employed remains a major determinant of financial and stock market performance although it has a negative impact on economic performance.Practical implications –The VAIC e method could be an important tool for many decision makers to integrate IC in their decision process.Originality/value –This is the first research which has used the data on VA recently calculated and published by the UK DTI in the “Value Added Scoreboard”.This paper constitutes therefore a kind of validation of the ministry data.Keywords Intellectual capital,Value added,Company performance,United KingdomPaper type Research paperI.IntroductionIntellectual capital (IC),innovation and value creation (or value added (VA))are nowadays the object of particular attention by managers,investors,economic institutions and governments;as they are also the object of several studies recently realised in academic and professional environments.According to the Organisation for Economic Cooperation and Development (OECD)(2008),many companies invest nowadays in employee training,research and development (R&D),customer relations,computer and administrative systems,etc.These investments,often referred to as IC,are growing and they are competing with physical and financial capital investments in some countries[1].Several authors,suchas Stewart (1997)and Ze´ghal (2000),ascribed this change in investment structure to theThe current issue and full text archive of this journal is available at/1469-1930.htmThe authors gratefully acknowledge the support of the CGA –Accounting Research Centre at the University of Ottawa.AnalysingVA as an indicator of IC 39Journal of Intellectual Capital Vol.11No.1,2010pp.39-60q Emerald Group Publishing Limited 1469-1930DOI 10.1108/14691931011013325advent of a new knowledge-based economy.Other authors,such as Edvinsson (1997),Sveiby (1997)and Lynn (1998)emphasised the importance of IC which they consider to be the main source of value creation in the new economy.However,it is difficult to measure IC since it is intangible and non-physical in nature.The traditional accounting model,which is conceived for companies operating in an industrial economy,remains focussed on physical and financial assets andignores most IC assets.Interestingly,even the International Accounting Standards/International Financial Reporting Standards (IAS/IFRS)[2],including the ones recently modified by the International Accounting Standards Board,did not contribute to redefining many of the concepts,principles and valuation methods of IC assets.The relative lack of IC accounting recognition and its growing role in the value creation process,imply that financial statements have lost some of their value for shareholders and many other users (Canibano et al.,2000;Ashton,2005;OECD,2006,2007).Many studies,often conducted within a resource-based theory framework,have tried to analyse the problem of accounting for IC.The first studies began with the identification,representation,and classification of IC components (Edvinsson andMalone,1997;Ze´ghal,2000;Guthrie et al.,2004).Other studies were interested rather in the practices of IC reporting in companies’annual reports (Williams,2001;Abdolmohammadi,2005;Kristandl and Bontis,2007).Some studies also focussed on the problem of IC measurement not being recorded in financial statements (Stewart,1997;Pulic,1998,2004;Gu and Lev,2003;Chen et al.,2004).Finally,a number of studies were related to the validation of IC in a decision-making context,notably in terms of its usefulness to investors on a capital market (Lev and Sougiannis,1996;Cazavan-Jeny,2004;Casta et al.,2005;Lev et al.,2007).In this study,we aim to extend the efforts made by researchers and practitioners to find an appropriate measure of IC.We propose therefore the concept of “value added”as an indicator of IC measurement in a company.This idea is based on the “value added intellectual coefficient –VAIC e ”method developed by Pulic (1998,2004).This method is still in the early stages of its application in management accounting practices and needs to be empirically validated with a large number of companies in a decision-making context.This is precisely the objective of our study according to which we attempt:.to empirically analyse the role of VA as an indicator of IC;and.to empirically validate the method of VAIC e to assess the impact of IC on economic,financial and stock market performance.Our study is motivated by the conceptual link which could exist between IC and VA.Indeed,a recent survey carried out by the UK Department of Trade and Industry (DTI)(2004)[3]shows that successful UK companies recognise that investing in IC is essential to their ability to create world-class VA products and services.Other reports edited by the OECD (2006,2007)considered IC as the main important source of value creation in a company.More recently,the UK DTI (2007)also reported that,on average,UK companies create much more VA than other European companies.In fact,UK companies’ability to compete in the global economy largely and increasingly depends on creating higher levels of VA through investments in IC.JIC 11,140The concept of“value added”used in the present study for measuring IC has a strong historical past in the UK(Morley,1979;Pong and Mitchell,2005).For many years,UK companies have revealed information about their VA in the“Value Added Statement”.At present,information on the VA of UK companies is also revealed by the UK DTI in the“Value Added Scoreboard”.All this explains our choice to carry out our study in a UK context through the analysis of a sample of300companies listed on the London Stock Exchange(LSE)during2005.We also chose,in this study,to divide our sample into three groups of sectors representing high-tech industries,traditional industries and services.Our expectation is that the contribution of IC to a company’s performance differs from sector to sector.The remainder of the present paper is organised as follows:Section2briefly describes the theoretical and conceptual framework for both IC and VA and outlines related prior research.Section3presents our research objective and the different research hypotheses.Section4describes the methodology used in this study and the results of the data analysis are detailed in Section5.Thefinal section summarises and concludes with suggestions for future research.II.Intellectual capital and value added1.Intellectual capitalUntil now,there has been no generally accepted definition or classification of IC (Canibano et al.,2000;Bhartesh and Bandyopadhyay,2005;OECD,2006).It was only in the late1990s that professionals and researchers in management began to attempt to define and to classify the IC components.Stewart(1997,p.67)defined IC simply as“packaged useful knowledge”.Edvinsson and Malone(1997,p.358)broadened the definition of IC to“knowledge that can be converted into value”.Following these authors we define IC,in this study,as being “the sum of all knowledge a company is able to use in the process of conducting business to create value–a VA for the company”.The studies conducted by Stewart(1997)and Edvinsson and Malone(1997)led to a similar classification of IC components.According to their classification,the company’s IC,in a broad sense,comprises human capital(HC)and structural capital (SC).HC is defined as the knowledge,qualifications and skills of employees and the fact that companies cannot own or prevent those employees from going home at night;SC refers to the knowledge that remains with the company after the employees go home at night.It includes production processes,information technology,customer relations, R&D,etc.This classification of IC components is,according to Ashton(2005),the one most used in literature up until the present time.There are also other classifications which subdivide the SC into organisational capital and customer capital(Sveiby,1997; Guthrie et al.,2004;Youndt et al.,2004).The IC(both human and structural)is viewed by resource-based theory(Wernerfelt, 1984;Barney,1991;Peteraf,1993)as being a strategic resource in the same way as capital employed(physical andfinancial)is viewed as a strategic resource.This theory considers that companies gain competitive advantage and superiorfinancial performance through the acquisition,holding and efficient use of strategic resources.However,many authors such as Riahi-Belkaoui(2003)and Youndt et al.(2004) underline that capital employed(physical andfinancial)is not strategic because it simply constitutes a generic resource.It is precisely IC that is viewed as being Analysing VA as an indicator of IC41a strategic resource allowing the company to create VA.For these authors,a resource is considered to be strategic when it distinguishes itself from others by the difficulty of imitation,substitution and by its imperfect mobility.This point of view is consistent with Reed et al.(2006)who recently developed an IC-based theory.Reed and her colleagues view their theory as a mid-range one because it represents one specific aspect of the more general resource-based theory.Althoughthey have the same objective,to explain corporate performance by the effective and efficient use of a company’s resources,the IC-based theory considers IC as being the only strategic resource to allow a company to create VA.This theory does not break away from its origins and is always analysed along with resource-based theory.2.Value added as an indicator of IC (the VAIC e method)Taking into consideration the increasing importance of the role played by IC in value creation,Pulic (1998,2004),with colleagues at the Austrian IC Research Centre,developed a new method to measure companies’IC which they called the “value added intellectual coefficient”(VAIC e ).This method is very important since it allows us to measure the contribution of every resource –human,structural,physical and financial –to create VA by the company.The calculation of the VAIC e method follows a number of different steps.The first step is to calculate the company’s ability to create VA.In accordance with the stakeholder theory[4](Meek and Gray,1988;Donaldson and Preston,1995;Riahi-Belkaoui,2003;DTI,2006),the VA is calculated as follows:VA ¼OUT 2IN ð1ÞOutputs (OUT)represent the revenue and comprise all products and services sold on the market;inputs (IN)include all expenses for operating a company,exclusive of employee costs which are not regarded as costs.The second step is to assess the relation between VA and HC.The value added human capital coefficient (VAHU)indicates how much VA has been created by one financial unit invested in employees.For Pulic (2004),employee costs are considered as an indicator of HC.These expenses are no longer part of the inputs.This means that expenses related to employees are not treated as a cost but as an investment.Thus,the relation between VA and HC indicates the ability of HC to create value in a company[5]:VAHU ¼VA =HC ð2ÞThe third step is to find the relation between VA and SC.The value added structural capital coefficient (STVA)shows the contribution of SC in value creation.According to Pulic (2004),SC is obtained when HC is deducted from VA.As this equation indicates,this form of capital is not an independent indicator.Indeed,it is dependent on the created VA and is in reverse proportion to HC.This means that the greater the share of HC in the created VA,the smaller the share of SC.Thus,the relation between VA and SC is calculated as:STVA ¼SC =VA ð3ÞThe fourth step is to calculate the value added intellectual capital coefficient (VAIN),which shows the contribution of IC in value creation.Given that IC is composed of HC and SC,the VAIN is obtained by adding up VAHU and STVA:JIC 11,142VAIN¼VAHUþSTVAð4ÞThen,thefifth step is to assess the relation between VA and physical andfinancial capital employed(CA).For Pulic(2004),IC cannot create value on its own.Therefore,it is necessary to takefinancial and physical capital into account in order to have a full insight into the totality of VA created by a company’s resources.The value added capital employed coefficient(VACA)reveals how much new value has been created by one monetary unit invested in capital employed.Thus,the relation between VA and CA indicates the ability of capital employed to create value in a company:VACA¼VA=CAð5ÞFinally,the sixth step is to assess each resource that helps to create or produce VA. Therefore,VAIC e measures how much new value has been created per invested monetary unit in each resource.A high coefficient indicates a higher value creation using a company’s resources,including its IC.Thus,VAIC e is calculated as follows:VAIC e¼VAINþVACAð6ÞThere has been some criticism of the VAIC e method,mainly by Andriessen(2004) who suggested that the method’s basic assumptions are problematic and thus it produces dissatisfying results.However,an increasingly large number of researchers (such as Chen et al.,2005;Shiu,2006;Kujansivu and Lonnqvist,2007;Tan et al.,2007; Yalama and Coskun,2007;Kamath,2007,2008;Chan,2009)adopted the VAIC e method which remains,in their view,the most attractive among the suggested methods to measure IC.For instance,Chan(2009,p.10)mentions at least a dozen favourable arguments for the VAIC e method,and concludes that the VAIC e is the most appropriate method to measure IC.Also,according to Kamath(2007,2008),the VAIC e method has compellingly proved its suitability as a tool for the measurement of IC.Finally,the fact that the UK DTI makes data available to facilitate the use of the VAIC e method contributes significantly,in our opinion,to the practical and empirical validity of this method.3.Prior researchRecent empirical studies have attempted to show that IC significantly contributes to VA created by the company,and therefore is positively associated with its performance.In this context,Pulic(1998),using data from Austrian companies,found that VA is very highly correlated with IC,whereas the correlation between VA and capital employed(physical andfinancial)is low.These results suggest that IC has become the main source of value creation in the new knowledge-based economy. Furthermore,Stewart(1997,2002),showed that a company’s performance depends on the ability of its resources to create VA.Similarly,Riahi-Belkaoui(2003),using a sample of US multinationalfirms,discovered a significantly positive association between IC and future performance.He considers IC as a strategic resource,able to create VA for the company.In a study based on4,254Taiwanese listed companies during the period1992-2002, Chen et al.(2005)found that IC and capital employed have a positive impact on market value,as well as on current and futurefinancial performance.In other words,the results indicated that investors place higher value onfirms with greater IC which are Analysing VA as an indicator of IC43considered more competitive than other firms.More recently,Tan et al.(2007)confirmed these ing data from 150publicly traded companies in Singapore,their findings showed that IC is positively associated with current and future financial performance.They also found that the contribution of IC to a company’s performance differs by industry.However,the studies of Firer and Williams (2003)and Shiu (2006)providedgenerally limited and mixed ing data from 75publicly traded companies in South Africa,Firer and Williams demonstrated that IC is negatively associated with traditional measures of corporate performance,while the association between these measures of performance and capital employed (physical and financial)is positive.In other words,the empirical results suggested that capital employed remains the most significant underlying resource of corporate performance in South Africa.Similar results were found by Shiu who examined the same model using data from 80Taiwanese technology firms.III.Research objective and hypothesesThe objective of this study is to empirically analyse the role of VA as an indicator of IC.We attempt also to empirically validate the method of VAIC e to assess the impact of IC under the following triptych:economic performance (Model 1),financial performance (Model 2),and stock market performance (Model 3).Our assumption is that if the company is economically successful,it is going to exhibit a healthy and profitable financial state of affairs,which is going to reverberate on its stock market performance.1.Economic performance modelMany authors suggest that IC investment allows the company to enhance its economic performance (Lev and Sougiannis,1996;Lev and Zarowin,1998;Casta et al.,2005;Bismuth and Tojo,2008).This performance is defined by the operating profitability which represents an economic surplus or an economic margin acquired by the difference between income and production costs (Cappelletti and Khouatra,2004).For instance,Nakamura (2001)suggested that if companies invest in IC,the success of these investments should permit companies on average to reduce their production costs and/or increase any kind of operational margins (or mark-ups).In this sense,highly skilled HC can enhance department store sales,and an efficient process of R&D can cut a factory’s production costs.In their model of IC valuation,Gu and Lev (2003)propose a new methodology based on the economic notion of “production function”.This methodology considers that a company’s economic performance is generated by three kinds of resources:physical,financial and intellectual.However,the UK DTI (2006)considers that,in a value creation context,the company’s economic performance depends not only on amounts invested in physical,financial and intellectual resources,but rather on the ability of these resources to create ing the VAIC e method measures,we will test the following hypothesis:H1a.There is a positive association between “value added intellectual capitalcoefficient”and economic performance.JIC 11,144H1b.There is a positive association between“value added capital employed (physical andfinancial)coefficient”and economic performance.2.Financial performance modelMany authors believed strongly that IC could have a positive effect on the company’s financial performance(Riahi-Belkaoui,2003;Youndt et al.,2004;Chen et al.,2005;Tan et al.,2007).This performance was defined by profitability,an expression of the ability of invested capital to earn a certain level of profit.Following resource-based theory,Chen et al.(2005)suggested that if IC is a valuable resource for a company’s competitive advantages,it will contribute to the company’s financial performance.This assumption is also shared by Youndt et al.(2004)who stated that IC intensive companies are more competitive than other companies and are, therefore,more successful.However,in order to constitute a sustained competitive advantage,and therefore a determinant offinancial performance,Riahi-Belkaoui(2003)and Firer and Williams (2003)assumed that IC,as well as the physical andfinancial capital employed,is used in an effective and efficient way.This efficiency is assessed,according to Pulic(2004), in terms of the resources’ability to create VA for the company.Using the VAIC e method measures,we will investigate the following hypothesis: H2a.There is a positive association between“value added intellectual capital coefficient”andfinancial performance.H2b.There is a positive association between“value added capital employed (physical andfinancial)coefficient”andfinancial performance.3.Stock market performance modelSome authors considered that the increasing gap between a company’s market and book value can be a consequence of not taking IC into account infinancial statements (Edvinsson and Malone,1997;Lev and Sougiannis,1996;Lev,2001;Skinner,2008). This gap,generally exhibited in market-to-book(MB)ratio,indicates that investors perceive IC as a source of value for a company,even though it is not present in the company’s book value.In this context,Firer and Williams(2003)and Chen et al.(2005)suggested that if the market is efficient,investors will place higher value on companies with greater IC.This assumption is also shared with Youndt et al.(2004)and Skinner(2008)who stated that IC intensive companies are valued more in the stock market than are other companies.However,Ze´ghal(2000)and the UK DTI(2006)considered that,in a value creation context,investors do not limit their investments to companies with greater IC.Rather they will try to select for their portfolios those companies that have a track record of continuous creation of VA in an efficient and sustainable way.Using the VAIC e method measures,we will test the following hypothesis:H3a.There is a positive association between“value added intellectual capital coefficient”and stock market performance.H3b.There is a positive association between“value added capital employed (physical andfinancial)coefficient”and stock market performance.Analysing VA as an indicator of IC45IV.Methodology 1.Data and sample selection The sample of companies used in this study was based on all UK companies which were listed on the LSE and which were available on the “Value Added Scoreboard”database provided by the UK DTI.The original data sample consisted of about 342companies[6]that had realised the biggest contribution to the VA in the UK during2005.The following selection criteria were then applied to the original data sample:.Following Firer and Williams (2003)and Shiu (2006),companies with negative book value of equity,or companies with negative HC or SC values were excluded from the sample..Companies for which some data were missing (unavailability of annual reports in consequence of merger,repurchase,suspension,delisting)were also excluded..Finally,in order to control for the presence of extreme observations or “outliers”in our sample,companies with selected variables situated at the extremities of every distribution were eliminated.This sample selection process led us to a final sample composed of 300UK companies (Table I).In contrast with previous studies which examined only a single sector (Firer and Williams,2003;Pulic,2004;Shiu,2006),the originality of our study consists in the examination of all sectors.In fact,the “new economy”literature shows that every sector of the economy has felt the impact of increased IC in value creation (Lynn,1998;Bhartesh and Bandyopadhyay,2005;Ashton,2005).However,it is likely that the contribution of IC to a company’s performance differs by industry (Abdolmohammadi,2005;Tan et al.,2007).For instance,the OECD (2006)suggested the application of industry-specific standards to accommodate the very different role of IC from sector to sector.Considering these suggestions,we chose in this study to divide our sample into different sub-samples to allow a cross-sectional analysis.After a review of classification criteria of sectors used in the literature,we opted to follow the rigorous classification provided by the UK DTI (2006,p.49).This classification consists in organising the 39industry classification benchmark (ICB)[7]sectors into three groups:high-tech industries,traditional industries and services (see the classification criteria in Table AI).Table II shows the sample distribution by sector group and stock index.As can be seen,our sample is dominated by the group of services (53.67per cent of the whole sample).The two other groups,i.e.traditional industries and high-tech industries,respectively,represent 30and 16.33per cent of the whole sample.Initial sample342Companies with negative book value215Companies missing data on selected variables214Extreme observations or “outliers”29Companies with negative human capital or structural capital values24Final sample 300Table I.Sample selection proceduresJIC 11,146Table II also shows that our sample of companies represents the different levels of market capitalization.The largest component of the sample(51.33per cent of whole sample)belongs to the Financial Times Stock Exchange(FTSE)250index which is characterised by medium market capitalization.The two other components of the sample(29and19.67per cent of whole sample)belong,respectively,to the FTSE100 index which is characterised by large market capitalization and other stock indexes which are characterised by small market capitalization(such as,for example,the FTSE small cap index).2.Definition of variables2.1Dependent variables.To conduct the relevant analysis in the present study,three dependent variables of operating income/sales(OI/S),return on assets(ROA)and MB were used as proxy measures for economic,financial and stock market performance, respectively.These variables were defined as:.OI/S.Ratio of the operating income divided by total sales,used as a proxy for economic performance(Lev and Sougiannis,1996;Nakamura,2001;Lev,2004)..ROA.Ratio of the earnings before interest and taxes divided by book value of total assets,used as a proxy forfinancial performance(Firer and Williams,2003;Chen et al.,2005;Shiu,2006)..MB.Ratio of the total market capitalization(share price times number of outstanding common shares)to book value of net assets,used as a proxy for stock market performance(Sougiannis,1994;Firer and Williams,2003;Cazavan-Jeny,2004).2.2Independent ing the VAIC e method measures,two coefficients were selected to measure both independent variables under consideration:(1)VAIN:the value added intellectual capital coefficient.where:VAIN¼VAHUþSTVAVAHU¼VA=HC;Value added human capital coefficientVA¼OUT2INHC¼employee costs2R&D costsðprincipally costs of employees working in R&DÞSTVA¼SC=VA;Value added structural capital coefficientSC¼VA2HC;structural capitalSector group Stock indexSector group Vol.Percentage Index Vol.PercentageHigh-tech industries4916.33FTSE1008729.00 Traditional industries9030.00FTSE25015451.33 Services16153.67Others5919.67 Total300100Total300100Table II.Sample distributionby sector groupand stock index Analysing VA as an indicator of IC47(2)VACA:the value added capital employed coefficient.,where:VACA ¼VA =CACA ¼book value of the net assets2.3Control variables .Two control variables were used in this study to control for theireffect on company performance:(1)Size of the company (Size):measured by the natural log of book value of total assets (Riahi-Belkaoui,2003).(2)Leverage (Lev):measured by the ratio of book value of total assets to book value of common equity (Lev and Sougiannis,1996).3.Research modelsIn order to respond to our research objective,we propose to empirically test the following three equations relating to the economic (Model 1),financial (Model 2)and stock market (Model 3)performance models:OI =S ¼b 0þb 1VAIN þb 2VACA þb 3Size þb 4Lev þmðModel 1ÞROA ¼b 0þb 1VAIN þb 2VACA þb 3Size þb 4Lev þmðModel 2ÞMB ¼b 0þb 1VAIN þb 2VACA þb 3Size þb 4Lev þm ðModel 3ÞV.Empirical results1.Descriptive statisticsTable III presents the means,standard deviations,medians,minimum and maximum values of all the variables.The mean MB is about 3.5,indicating that investors generally valued the sample companies in excess of the book value of net assets as reported in financial statements.Consequently,nearly 70per cent of a company’s market value is not reflected on financial statements.The comparison between VAIN and VACA values suggests that during 2005the sample companies were generally more effective in creating VA from their IC (VAIN ¼2.946)than from physical and financial capital employed (VACA ¼1.402).This finding is consistent with priorliterature (Ze´ghal,2000;Pulic,2004)suggesting that,in the new economic era,we accord much more value to wealth created by intellectual resources than that created by physical and financial resources.The mean of aggregate VAIC e which is4.348indicates that UK companies studied in this paper created £4.348for every £employed.To compare between groups of sectors,a one-way ANOVA was conducted on the three groups.Results from this test are shown in Table IV.These results again suggest that each group (high-tech industries,traditional industries and services)was generally more effective in creating VA from its IC than from physical and financial capital employed (VAIN .VACA).This finding is consistent with the “new economy”literature showing that every sector of the economy has felt the impact of increased IC in value creation and economic wealth,and that value creation applies to any sector (Lynn,1998;Bhartesh and Bandyopadhyay,2005;Ashton,2005).It is also consistent JIC 11,148。
新视野大学英语2(第三版)unit8 textA课文翻译
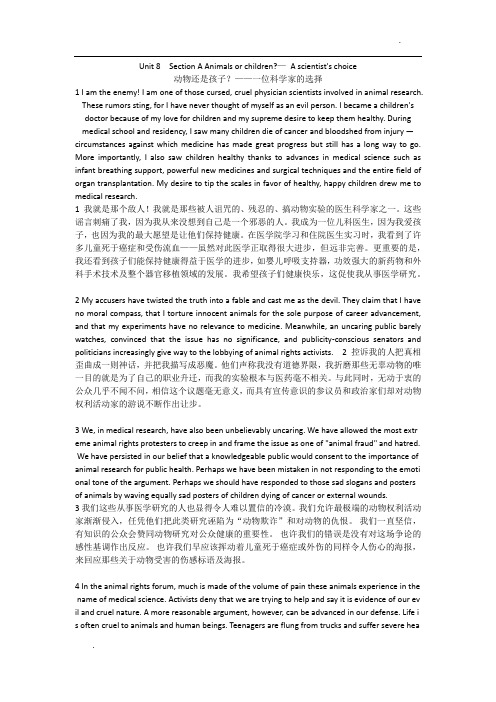
Unit 8 Section A Animals or children?—A scientist's choice动物还是孩子?——一位科学家的选择1 I am the enemy! I am one of those cursed, cruel physician scientists involved in animal research. These rumors sting, for I have never thought of myself as an evil person. I became a children's doctor because of my love for children and my supreme desire to keep them healthy. During medical school and residency, I saw many children die of cancer and bloodshed from injury —circumstances against which medicine has made great progress but still has a long way to go. More importantly, I also saw children healthy thanks to advances in medical science such as infant breathing support, powerful new medicines and surgical techniques and the entire field of organ transplantation. My desire to tip the scales in favor of healthy, happy children drew me to medical research.1 我就是那个敌人!我就是那些被人诅咒的、残忍的、搞动物实验的医生科学家之一。
预期后悔对网上冲动性购买行为影响的实证研究

Modern Marketing 现代市场营销, 2017, 7(1), 1-14Published Online February 2017 in Hans. /journal/mom https:///10.12677/mom.2017.71001文章引用: 耿黎辉, 严茂洋, 向金金. 预期后悔对网上冲动性购买行为影响的实证研究[J]. 现代市场营销, 2017, 7(1):Empirical Research on the Impact of Anticipated Regret on Impulse Buying Behavior on InternetLihui Geng 1, Maoyang Yan 1, Jinjin Xiang 21School of Economics and Management, Southwest Jiaotong University, Chengdu Sichuan2School of Economics and Management, Nanchang Institute of Science and Technology, Nanchang JiangxiReceived: Jan. 13th , 2017; accepted: Feb. 6th , 2017; published: Feb. 9th, 2017AbstractDrawing on the theory of impulse buying behavior and anticipated regret, this study explores the relationship between anticipated regret and impulse buying behavior on internet, investigates different factors (price promotion, graphic display and online comment) and impulsivity trait influencing impulse buying behavior on internet. Samples collected are empirically tested by SPSS17.0 and multiple group regression analysis is conducted with anticipated regret as a mod-erator variable. The results indicated that anticipated regret can directly influence online impulse buying. In the effect of price promotion, graphic display and impulsive impulsivity trait on con-sumer impulse buying, the moderating effect of anticipated regret is significant. In the effect of on-line comment on consumer impulse buying, the moderating effect of anticipated regret is not sig-nificant.KeywordsImpulse Buying, Anticipated Regret, External Stimuli, Impulsivity Trait, Moderated Function预期后悔对网上冲动性购买行为影响的 实证研究耿黎辉1,严茂洋1,向金金21西南交通大学,经济管理学院,四川 成都 2南昌工学院,经济管理学院,江西 南昌耿黎辉等收稿日期:2017年1月13日;录用日期:2017年2月6日;发布日期:2017年2月9日摘要基于冲动性购买、预期后悔等相关理论,探讨了预期后悔和电子商务环境下消费者冲动性购买行为的关系,研究了不同的刺激因素(价格促销、图文展示、他人评论)和冲动性特质对网上冲动性购买行为产生的影响。
EmpiricalEvidence:ADefinitionLiveScience

EmpiricalEvidence:ADefinitionLiveScienceEmpirical evidence is information acquired by observation or experimentation. Scientists record and analyze this data. The process is a central part of the scientific method.The scientific methodThe scientific method begins with scientists forming questions, or hypotheses, and then acquiring the knowledge through observations and experiments to either support or disprove a specific theory. 'Empirical' means 'based on observation or experience,' according to the Merriam-Webster Dictionary. Empirical research is the process of finding empirical evidence. Empirical data is the information that comes from the research.Before any pieces of empirical data are collected, scientists carefully design their research methods to ensure the accuracy, quality and integrity of the data. If there are flaws in the way that empirical data is collected, the research will not be considered valid.The scientific method often involves lab experiments that are repeated over and over, and these experiments result in quantitative data in the form of numbers and statistics. However, that is not the only process used for gathering information to support or refute a theory.Types of empirical research'Empirical evidence includes measurements or data collected through direct observation or experimentation,' said Jaime Tanner, a professor of biology at Marlboro College in Vermont. There are two research methods used to gather empirical measurements and data: qualitative and quantitative.Qualitative research, often used in the social sciences, examines the reasons behind human behavior, according to Oklahoma State University. It involves data that can be found using the human senses. This type of research is often done in the beginning of an experiment.Quantitative research involves methods that are used to collect numerical data and analyze it using statistical methods, according to the IT University of Copenhagen. Quantitative numerical data can be any data that uses measurements, including mass, size or volume, according to Midwestern State University, in Wichita Falls, Texas. This type of research is often used at the end of an experiment to refine and test the previous research.Identifying empirical evidenceIdentifying empirical evidence in another researcher's experiments can sometimes be difficult. According to the Pennsylvania State University Libraries, there are some things one can look for when determining if evidence is empirical:•Can the experiment be recreated and tested?•Does the experiment have a statement about the methodology, tools and controls used?•Is there a definition of the group or phenomena being studied?BiasThe objective of science is that all empirical data that has been gathered through observation, experience and experimentation is without bias. The strength of any scientific research depends on the ability to gather and analyze empirical data in the most unbiased and controlled fashion possible.However, in the 1960s, scientific historian and philosopherThomas Kuhn promoted the idea that scientists can be influenced by prior beliefs and experiences, according to the Center for the Study of Language and Information.Because scientists are human and prone to error, empirical data is often gathered by multiple scientists who independently replicate experiments. This also guards against scientists who unconsciously, or in rare cases consciously, veer from the prescribed research parameters, which could skew the results.The recording of empirical data is also crucial to the scientific method, as science can only be advanced if data is shared and analyzed. Peer review of empirical data is essential to protect against bad science, according to the University of California. Empirical law vs. scientific lawEmpirical laws and scientific laws are often the same thing. 'Laws are descriptions — often mathematical descriptions — of natural phenomenon,' Peter Coppinger, associate professor of biology and biomedical engineering at the Rose-Hulman Institute of Technology, told Live Science. Empirical laws are scientific laws that can be proven or disproved using observations or experiments, according to the Merriam-Webster Dictionary. So, as long as a scientific law can be tested using experiments or observations, it is considered an empirical law. Empirical, anecdotal and logical evidenceEmpirical, anecdotal and logical evidence should not be confused. They are separate types of evidence that can be used to try to prove or disprove and idea or claim.Logical evidence is used proven or disprove an idea using logic. Deductive reasoning may be used to come to a conclusion to provide logical evidence. For example, 'All men are mortal. Harold is a man. Therefore, Harold is mortal.'Anecdotal evidence consists of stories that have been experienced by a person that are told to prove or disprove a point. For example, many people have told stories about their alien abductions to prove that aliens exist. Often, a person's anecdotal evidence cannot be proven or disproven.Additional resources•U.S. National Library of Medicine: Empirical Evidence of Bias •Mississippi State University: Empirical Research Tutorial•National Bureau of Economic Research: Persuasion- Empirical Evidence•Texas Education Agency: Differentiate Among Empirical, Anecdotal and Logical Evidence。
Sepsis-induced myopathy
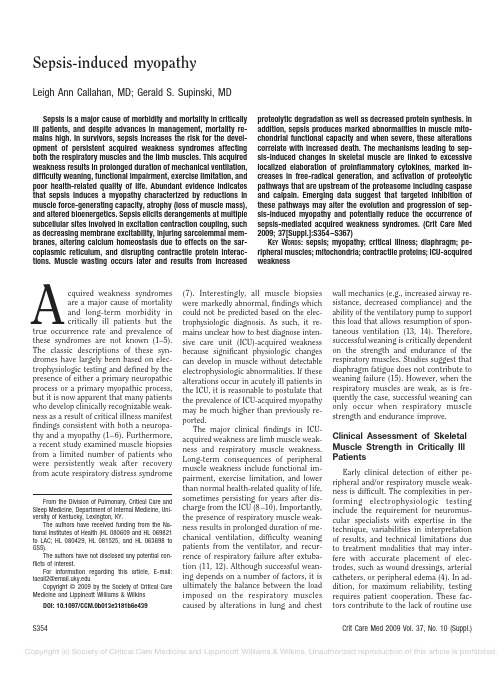
Sepsis-induced myopathyLeigh Ann Callahan,MD;Gerald S.Supinski,MDAcquired weakness syndromes are a major cause of mortality and long-term morbidity in critically ill patients but thetrue occurrence rate and prevalence of these syndromes are not known (1–5).The classic descriptions of these syn-dromes have largely been based on elec-trophysiologic testing and defined by the presence of either a primary neuropathic process or a primary myopathic process,but it is now apparent that many patients who develop clinically recognizable weak-ness as a result of critical illness manifest findings consistent with both a neuropa-thy and a myopathy (1–6).Furthermore,a recent study examined muscle biopsies from a limited number of patients who were persistently weak after recovery from acute respiratory distress syndrome(7).Interestingly,all muscle biopsies were markedly abnormal,findings which could not be predicted based on the elec-trophysiologic diagnosis.As such,it re-mains unclear how to best diagnose inten-sive care unit (ICU)-acquired weakness because significant physiologic changes can develop in muscle without detectable electrophysiologic abnormalities.If these alterations occur in acutely ill patients in the ICU,it is reasonable to postulate that the prevalence of ICU-acquired myopathy may be much higher than previously re-ported.The major clinical findings in ICU-acquired weakness are limb muscle weak-ness and respiratory muscle weakness.Long-term consequences of peripheral muscle weakness include functional im-pairment,exercise limitation,and lower than normal health-related quality of life,sometimes persisting for years after dis-charge from the ICU (8–10).Importantly,the presence of respiratory muscle weak-ness results in prolonged duration of me-chanical ventilation,difficulty weaning patients from the ventilator,and recur-rence of respiratory failure after extuba-tion (11,12).Although successful wean-ing depends on a number of factors,it is ultimately the balance between the load imposed on the respiratory muscles caused by alterations in lung and chestwall mechanics (e.g.,increased airway re-sistance,decreased compliance)and the ability of the ventilatory pump to support this load that allows resumption of spon-taneous ventilation (13,14).Therefore,successful weaning is critically dependent on the strength and endurance of the respiratory muscles.Studies suggest that diaphragm fatigue does not contribute to weaning failure (15).However,when the respiratory muscles are weak,as is fre-quently the case,successful weaning can only occur when respiratory muscle strength and endurance improve.Clinical Assessment of Skeletal Muscle Strength in Critically Ill PatientsEarly clinical detection of either pe-ripheral and/or respiratory muscle weak-ness is difficult.The complexities in per-forming electrophysiologic testing include the requirement for neuromus-cular specialists with expertise in the technique,variabilities in interpretation of results,and technical limitations due to treatment modalities that may inter-fere with accurate placement of elec-trodes,such as wound dressings,arterial catheters,or peripheral edema (4).In ad-dition,for maximum reliability,testing requires patient cooperation.These fac-tors contribute to the lack of routine useFrom the Division of Pulmonary,Critical Care and Sleep Medicine,Department of Internal Medicine,Uni-versity of Kentucky,Lexington,KY.The authors have received funding from the Na-tional Institutes of Health (HL 080609and HL 069821to LAC;HL 080429,HL 081525,and HL 063698to GSS).The authors have not disclosed any potential con-flicts of interest.For information regarding this article,E-mail:lacall2@Copyright ©2009by the Society of Critical Care Medicine and Lippincott Williams &Wilkins DOI:10.1097/CCM.0b013e3181b6e439Sepsis is a major cause of morbidity and mortality in critically ill patients,and despite advances in management,mortality re-mains high.In survivors,sepsis increases the risk for the devel-opment of persistent acquired weakness syndromes affecting both the respiratory muscles and the limb muscles.This acquired weakness results in prolonged duration of mechanical ventilation,difficulty weaning,functional impairment,exercise limitation,and poor health-related quality of life.Abundant evidence indicates that sepsis induces a myopathy characterized by reductions in muscle force-generating capacity,atrophy (loss of muscle mass),and altered bioenergetics.Sepsis elicits derangements at multiple subcellular sites involved in excitation contraction coupling,such as decreasing membrane excitability,injuring sarcolemmal mem-branes,altering calcium homeostasis due to effects on the sar-coplasmic reticulum,and disrupting contractile protein interac-tions.Muscle wasting occurs later and results from increasedproteolytic degradation as well as decreased protein synthesis.In addition,sepsis produces marked abnormalities in muscle mito-chondrial functional capacity and when severe,these alterations correlate with increased death.The mechanisms leading to sep-sis-induced changes in skeletal muscle are linked to excessive localized elaboration of proinflammatory cytokines,marked in-creases in free-radical generation,and activation of proteolytic pathways that are upstream of the proteasome including caspase and calpain.Emerging data suggest that targeted inhibition of these pathways may alter the evolution and progression of sep-sis-induced myopathy and potentially reduce the occurrence of sepsis-mediated acquired weakness syndromes.(Crit Care Med 2009;37[Suppl.]:S354–S367)K EY W ORDS :sepsis;myopathy;critical illness;diaphragm;pe-ripheral muscles;mitochondria;contractile proteins;ICU-acquired weaknessof these techniques particularly because the availability of neurologists with this expertise is limited.As a result,electro-diagnostic testing is usually requested af-ter clinical recognition of weakness, which typically occurs late in the evolu-tion of the disease.Peripheral muscle involvement is usu-ally recognized clinically when patients are recovering from the acute phase of illness,and upon awakening,are noted to be weak.The severity of peripheral mus-cle weakness is widely variable and can be profound in some cases,manifesting symptoms of paralysis(16).The most widely used clinical tool to assess periph-eral muscle strength at the bedside is the Medical Research Council examination, which incorporates strength testing of three muscle groups in each limb with assignment of scores on a scale of1to5.A Medical Research Council score ofϽ48 is indicative of clinically significant weak-ness(11).Although it has been suggested that these measurements are reliable and reproducible,patients must be awake and fully cooperative.This restricts the utility of this tool in determining the presence of weakness,particularly early in the course of acute illness.Similarly,recognition of acquired re-spiratory muscle weakness most often oc-curs clinically when patients are difficult to wean from mechanical ventilation. Bedside assessment of respiratory muscle strength in critically ill patients typically involves measurements of maximal in-spiratory pressure generation and maxi-mal expiratory pressure generation(17). However,in mechanically ventilated pa-tients,measurements are often inaccu-rate because they are highly dependent both on the operator as well as the voli-tional effort of the patient.Even when standardized techniques are used by trained investigators,these measure-ments are unreliable(18).As a result,it is difficult,using these methods,to diag-nose acquired respiratory muscle weak-ness early in the course of critical illness.On the other hand,it is important to recognize that magnetic stimulation techniques are available to objectively measure respiratory muscle strength and peripheral muscle strength in critically ill mechanically ventilated patients(19). These techniques can be performed re-producibly without the limitation of an awake cooperative patient and also pro-vide the opportunity to detect early re-ductions in muscle strength.Several studies have utilized anterolateral mag-netic phrenic nerve stimulation to mea-sure diaphragm pressure generation inresponse to supramaximal twitch stimu-lation(T w P di)in mechanically ventilatedpatients in the ICU(15,20).In patientswho were thought to be ready for wean-ing from mechanical ventilation,Laghi etal found marked decrements in T w P di val-ues which averaged8to10cm H2O(15).Watson et al also measured T w P di in33critically ill mechanically ventilated pa-tients with a variety of diagnoses andduration of ventilation,and found valuesaveraged10.14cm H2O(20).These datareveal diaphragm strength is profoundlyreduced in critically ill patients,with val-ues averaging between23%and36%ofnormal(20,21).In addition,these stud-ies reported a wide range in the severityof respiratory muscle weakness withsome patients showing T w P di values aslow as1to2cm H2O(20).These nonvo-litional objective measurements have alsobeen used to assess peripheral musclestrength in a number of patient popula-tions including ICU patients.Harris et alassessed adductor pollicus strength in asmall group of critically ill patient andnoted severe peripheral muscle weakness,with values as low as30%of controls(22).Magnetic stimulation has also beenused to objectively measure the strengthof lower limb muscles(19,23).Use ofsuch techniques is therefore likely to pro-vide clinically relevant information re-garding the physiologic evolution ofweakness in critically ill patients,partic-ularly in the early stages of acute illness.Electrodiagnostic testing,use of theMedical Research Council examination,and measures of maximal inspiratorypressure and maximal expiratory pres-sure generation all require an awake co-operative patient,whereas magneticstimulation techniques provide objectivemeasurements of strength independentof these confounding variables.Nonethe-less,there has been a fair amount of in-terest in developing more simplified ap-proaches to assess weakness in criticallyill patients(24–26).Recently,it has beenargued that,because the therapeutic ar-mamentarium for ICU-acquired weaknessis virtually nonexistent,it is not essentialto make a precise physiologic diagnosis(24).On the other hand,it is important torealize that no study has systematicallyemployed objective nonvolitional func-tional measurements of muscle strengthto determine the prevalence or the timecourse of development of respiratorymuscle dysfunction and/or limb muscleweakness in critically ill patients.Emerg-ing data suggest that use of pharmaco-logic agents and other interventions mayvery well impact the occurrence and pro-gression of ICU-acquired weakness syn-dromes,and potentially improve recov-e of a simplistic,less sophisticatedapproach in clinical trials,however,isunlikely to result in sufficiently scientif-ically rigorous outcome measurementsthat would provide clear evidence as towhether or not these agents or interven-tions improve strength or prevent wast-ing.Furthermore,the fact that ourprogress in management and treatmentof these syndromes is in its infancy pro-vides a strong rationale for an approachthat systematically incorporates both theavailable scientific evidence and best di-agnostic tools to critically address theseissues,rather than retreating to simplis-tic tactics.Failure to use more sophisti-cated methods may very well impede ad-vancements in understanding anddevelopment of effective treatments forcritical care-acquired weakness.Sepsis Is a Major Risk forAcquired Weakness in CriticallyIll PatientsAlthough a number of factors havebeen associated with prolonged weaknessin critically ill patients,sepsis(includingseptic shock,systemic inflammatory re-sponse syndrome of infectious and non-infectious etiologies,multiple organ fail-ure)is a major risk(4,27).AlthoughBolton et al(28)were thefirst to suggestthat critical illness-acquired weaknesssyndromes were associated with sepsis,systemic inflammatory response syn-drome,and multiple organ failure,addi-tional prospective and retrospective clin-ical studies confirm this observation(29–31).Importantly,the reported frequencyof prolonged weakness in sepsis is ex-tremely high,occurring in70%to100%of these patients(29–31).Furthermore,these studies provide clear evidence thatsepsis produces profound decrements inboth limb and respiratory muscle func-tion.In the last decade,much attention hasfocused on studies showing that mechan-ical ventilation per se induces deleteriouseffects on the diaphragm(32–35).How-ever,the clinical importance of ventila-tor-induced diaphragm dysfunction andits relationship to ICU-acquired weaknessis still unclear.For example,ventilator-induced diaphragm dysfunction does notproduce peripheral muscle weakness (36).Furthermore,the mode of ventila-tion is a major factor in producing ven-tilator-induced diaphragm dysfunction (37–39).Although Levine et al elegantly validated that diaphragm atrophy devel-ops in brain dead patients who were me-chanically ventilated using a controlled mode(32),this study did not include assessments of diaphragm strength.In addition,an international survey sug-gested that controlled mechanical venti-lation is not commonly used in the ICU (40).Finally,if mechanical ventilation is the major cause of respiratory muscle weakness in critically ill patients,venti-latory support theoretically would pro-hibit successful weaning in any patient who requires mechanical ventilation be-cause of persistent ongoing respiratory muscle injury.As such,more studies are required to address the importance of ventilator-induced diaphragm dysfunc-tion in patients and its relationship to ICU-acquired weakness.In contrast,both animal and human data showed that sep-sis-induced respiratory and limb muscle weakness is a major risk for development of ICU-acquired weakness. Manifestations of Sepsis-Induced MyopathyThere is abundant scientific evidence that sepsis induces a myopathy character-ized by reductions in force-generating ca-pacity,atrophy(loss of muscle mass),and altered bioenergetics.A variety of animal models including cecal ligation perfora-tion(CLP),endotoxin administration, live bacteria injection,pneumonia,and cytokine induction have been used to as-sess skeletal muscle function in sepsis. Interestingly,most functional assess-ments of skeletal muscle in sepsis have examined the respiratory muscles;fewer data exist for limb muscles.The following sections will review the manifestations of sepsis-induced changes in muscle. Sepsis Reduces SkeletalMuscle-Specific Force GenerationIt is important to understand that muscle strength is dependent on two fac-tors:muscle force generation for a given amount of muscle mass and total muscle mass.These factors represent two dis-tinct aspects of muscle function and can alter muscle strength independently of each other.This is a critical concept be-cause the processes that regulate andmodulate muscle force generation for agiven amount of muscle mass(force percross-sectional area)and the processesthat regulate and modulate total musclemass are different.Most of the studiesdescribed in this section evaluated phys-iologic function in muscle by assessingmuscle-specific force generation,a mea-sure of muscle strength where force isnormalized to muscle cross-sectionalarea,thereby taking into account anychanges in muscle mass.Furthermore,muscle wasting can occur without pro-ducing changes in muscle-specific forcegeneration.For example,in a model ofprolonged nutritional deprivation,Lewiset al showed that diaphragm mass wasreduced by50%,but diaphragm-specificforce generation was normal(41).In ad-dition,Le Bourdelles et al showed thatafter48hrs of mechanical ventilation,limb muscle mass is reduced significantlywithout concomitant reductions in limbmuscle-specific force generation(36).Therefore,although force reductions andmuscle wasting often occur simulta-neously,it is important to understandthat reductions in muscle-specific forcegeneration may exist in the absence ofatrophy and atrophy may occur withoutreducing muscle-specific force genera-tion.Likewise,enhancing muscle massdoes not necessarily improve muscle-specific force generation,as demon-strated in studies that assessed musclecontractile performance in myostatinknockout animals(42).Finally,experi-mental evidence suggests that pharmaco-logic interventions can differentially af-fect muscle-specific force generation andwasting(43,44).In one of thefirst studies evaluatingrespiratory muscle contractile perfor-mance in sepsis,Hussain et al showedthat injection of Escherichia coli endo-toxin in spontaneously breathing dogs in-duced hypercapneic respiratory failure(45).Subsequently,a number of investi-gators demonstrated that diaphragm con-tractile performance is profoundly re-duced in several different animal modelsof sepsis and infection,including CLP,endotoxin administration and pneumonia(46–53)(Fig.1).Declines in respiratorymuscle strength occur within hours ofinduction of sepsis and are rapidly pro-gressive.Importantly,these decrementsin diaphragm-specific force generation(force normalized per muscle cross-sectional area)occur before any evidenceof reductions in diaphragm mass or dia-phragm protein content(54).Similar data indicate that sepsis alsoalters peripheral muscle-specific forcegeneration.Supinski et al examined theeffects of varying doses of endotoxin andobservedϾ50%reductions in force-generating capacities of both the dia-phragm and limb muscles(55)(Fig.2).Recently,Eikermann et al examined forcegeneration and fatiguability in the adduc-tor pollicus muscle of critically ill septicpatients,comparing these functional pa-rameters in patients with normal individ-uals before and after cast immobilization(56).Importantly,2wks of lower armimmobilization had no effects on forcegeneration in controls,but forcegenera-Figure 1.Diaphragm force-frequency relation-ships in endotoxin-treated animals.Diaphragmforce-frequency curves for control(E)and low(F),medium(Œ),and high(f)dose endotoxingroups.As shown,endotoxin elicited a dose-dependent reduction in respiratory muscle-specific force generation.*Control group signif-icantly different from the three endotoxingroups.Modified and reproduced with permis-sion from Supinski et al(55).Figure2.Limb muscle force-frequency relation-ships in endotoxin-treated animals.Limb muscle(flexor hallucis longus)force-frequency relation-ships for control(E)and low(F),medium(Œ),and high(f)dose endotoxin groups.Endotoxinreduced limb muscle-specific force generation ina dose-dependent fashion.*Control group signif-icantly different from the three endotoxingroups;#control group significantly differentfrom low and high endotoxin dose groups;**con-trol group significantly different from low endo-toxin dose group.Modified and reproduced withpermission from Supinski et al(55).tion was severely reduced in septic pa-tients (Fig.3).In contrast,pneumonia markedly im-pairs diaphragm force generation,but pe-ripheral muscle force is preserved (51).Likewise,cardiac specific overexpression of tumor necrosis factor (TNF)-␣reduces diaphragm strength without altering limb muscle function (57).Studies also indicate that sepsis induces more abun-dant cytokine levels in the diaphragm compared with limb muscle,raising the question as to whether or not the respi-ratory muscles are more susceptible to sepsis-induced injury (58).If this is true,it is conceivable that respiratory muscle weakness may represent the earliest man-ifestation of sepsis-induced myopathy.Sepsis Reduces Skeletal Muscle MassThe earliest important physiologic al-teration in response to acute sepsis is a reduction in muscle-specific force-gener-ating capacity.However,in more pro-longed models of sepsis,muscle wasting is a prominent feature and is thought to result from increased proteolysis and de-creased protein synthesis.A number of recent reviews have highlighted the cur-rent knowledge regarding the mecha-nisms underlying muscle atrophy in a variety of conditions including sepsis (59–63).For example,in the CLP model,data indicate that limb muscle protein content diminishes due to increased pro-teolysis (64).Rossignol et al also found reductions in limb muscle force genera-tion and mass,using a prolonged model of CLP (65).Wasting also occurs in both respiratory and limb muscles with pro-longed endotoxemia (66,67)and in other models of protracted infection (68).Al-though experimental models of pneumo-nia have not completely addressed atro-phy and protein loss,Langen et al described significant muscle wasting and impaired muscle regeneration in a trans-genic mouse model of chronic pulmonary inflammation (69).These data,therefore,provide evidence that loss of muscle mass is also an important aspect of sepsis-induced muscle weakness.Sepsis Alters Skeletal Muscle BioenergeticsAlterations in mitochondrial bioener-getic function are thought to play a crit-ical role in sepsis-induced organ failure and there are a number of recent reviews on this topic (70–75).Both human and animal data indicate that sepsis inducesmitochondrial dysfunction in respiratory and limb muscles,and these alterations are thought to be important in the patho-genesis of sepsis-induced muscle dys-function.Brealey et al demonstrated de-creased respiratory chain complex I activity and lower adenosine triphosphate (ATP)levels in skeletal muscle biopsies taken from critically ill patients in septic shock (76).Furthermore,they showed a significant correlation between muscle mitochondrial dysfunction and death in sepsis.In follow-up studies,this group reported similar alterations in complex I activity and ATP levels in hind-limb mus-cle of rodents as well as significant dec-rements in bioenergetics in other tissues (77).Interestingly,they also demon-strated that,despite severe metabolic dis-turbances in muscle,gross histologic damage or evidence of cell death is ab-sent;this underscores the importance of performing physiologic assessments when evaluating the effects of sepsis in muscle.Although bioenergetic function in sepsis is markedly abnormal,the ex-tent to which these mitochondrial de-rangements participate in the develop-ment and progession of sepsis-induced prolonged ICU-acquired weakness,or in the recovery process,however,are yet to be determined.Subcellular Sites of Skeletal Muscle Dysfunction in SepsisSepsis-induced myopathy may produce muscle dysfunction by effecting a number of sites involved in excitation contraction coupling including the sarcolemma,the sarcoplasmic reticulum,the contractile ap-paratus,and the mitochondria.SarcolemmaMuch attention has been given to the discovery that muscle becomes electri-cally inexcitable during the development of acquired weakness in critically ill pa-tients (78–81).This has led to the con-cept that ICU-associated weakness repre-sents an acquired channelopathy involving dysregulation of sodium chan-nels.It is important to note,however,that much of these data with respect to critical illness myopathy has been gener-ated in animal models using denervation with concomitant administration of high-dose steroids and should not be extrapo-lated to sepsis.However,Rossignol et al recently showed evidence of decreased muscle membrane excitability andup-Figure 3.Force-frequency relationships in patients with sepsis and multiple organ failure.Force-frequency curves of adductor pollicis muscle during supramaximal ulnar nerve stimulation before performing the fatigue protocol (mean Ϯstandard deviation).Force is significantly lower in patients with sepsis and multiple organ failure (full circles ;n ϭ13)compared with healthy volunteers (triangles ;n ϭ7)both before (open triangles )and after (solid triangles )2wks of immobilization of their lower arm and thumb.*p Ͻ.01vs.volunteers before and after immobilization;#p Ͻ.05vs.volunteers before immobilization.These data indicate that sepsis significantly reduced muscle force generation in peripheral muscle whereas immobilization had no effect.Reproduced with permission from Eikermann et al (56).regulation of Nav 1.5in a chronic sepsis model,corroborating findings in the dener-vation steroid model (82).Additional stud-ies will be needed to determine whether this process plays a central role in the pathogenesis of sepsis-induced myopathy.On the other hand,there is clear evi-dence that sepsis causes marked sar-colemmal injury in muscles (83–85).Us-ing a fluorescent tracer dye (procion orange)which does not enter cells with intact membranes,Lin et al found that diaphragm myofibers of both lipopolysac-charide treated and CLP animals demon-strated a markedly greater level of sar-colemmal damage than in control animals (83)(Fig.4).In addition,sar-colemmal damage also increased in the soleus muscles of the CLP group.Fur-thermore,these alterations were linked to excessive nitric oxide generation and alterations in diaphragm myofiber mem-brane potential (83).Importantly,insti-tution of mechanical ventilation in septic animals prevented sarcolemmal damage in the diaphragm (84),suggesting that mechanical ventilation under these con-ditions was not harmful but,in fact,pro-tective.Although it is not known if sepsis-induced sarcolemmal injury occurs in pa-tients,this is nevertheless an important issue to consider.For example,the ben-eficial effect of exercise on skeletal mus-cle are well described;however,exercise increases cytokines and free-radical gen-eration in muscle (86).In addition,Du-mont et al recently showed that reloading muscles induces inflammatory cell infil-tration and worsens sarcolemmal injury (87).As a result,it is conceivable that exercising patients with damaged mus-cles could potentially propagate muscle inflammation and injury,or delay recov-ery.Such issues should be carefully ex-amined in the context of early mobiliza-tion and exercise interventions,and particularly in patients with sepsis-induced acquired weakness.Sarcoplasmic ReticulumThe skeletal muscle ryanodine recep-tor (RyR1)is a highly redox-senstive ion channel,modulated by hydrogen perox-ide and nitric oxide (NO)under physio-logic conditions (88–90).However,a number of studies suggest that resting intracellular calcium (Ca ϩϩ)levels are increased in muscle in sepsis,and al-though most studies have examined this issue in cardiac muscle,there are data for skeletal muscle.For example,Benson et al showed that Ca ϩϩuptake and content in limb skeletal muscle are increased dur-ing sepsis and that these high Ca ϩϩcon-centrations are critically involved in reg-ulation of muscle protein breakdown in sepsis (91).On the other hand,Liu inves-tigated the in vivo effects of lipopolysac-charide on the Ca ϩϩ-dependent mechan-ical activity and ryanodine response in isolated sarcoplasmic reticulum mem-brane vesicles from the mouse dia-phragm,demonstrating that Ca ϩϩre-lease and [3H]ryanodine binding in sarcoplasmic reticulum membrane vesi-cles of lipopolysaccharide-treated animals were significantly depressed (92)(Fig.5).Taken together,these data suggest that complex alterations in calcium ho-meostasis are present in septic skeletal muscle,with evidence that calcium in-creases in some subcellular compart-ments and decreases in others.Additional data will be needed to determine whether sarcoplasmic reticulum alterations are important in the pathogenesis of sepsis-induced myopathy and ICU-acquired weakness.Contractile ProteinsOne mechanism by which sepsis re-duces skeletal muscle force generation in sepsis is by directly alteringcontractileFigure 4.Sepsis induces skeletal muscle sarcolem-mal injury.Representative micrographs illustrating the effects of sepsis on sarcolemmal integrity in the diaphragm.a ,Control group.Very little tracer dye uptake within individual myofibers was found,al-though intense staining of extracellular connective tissue indicates good dye diffusion throughout the tissue.b ,Lipopolysaccharide group.Note the pres-ence of numerous myofibers with sarcolemmal damage,as indicated by their inability to prevent entry of the low molecular weight tracer dye.c ,Cecal ligation perforation group.Myofibers with sarcolemmal damage are characterized by variable degrees of intracellular fluorescent staining,similar to the findings in the lipopolysaccharide group.Reproduced with permission from Lin et al(83).Figure 5.Sepsis induces alterations in skeletal muscle sarcomplasmic reticulum calcium han-dling.Ca2ϩrelease and [3H]ryanodine binding were studied using sarcoplasmic reticulum mem-brane vesicles isolated from skeletal muscles of control and lipopolysaccharide (LPS )(7.5mg/kg)-treated animals.Some rats were pretreated with polymyxin B (PMB ),the polycationic anti-biotic that neutralizes LPS before the application of LPS.A )Ryanodine (2M)-induced Ca2ϩre-lease from sarcoplasmic reticulum was signifi-cantly reduced in muscles from LPS-treated an-imals compared with controls.B )[3H]ryanodine binding was also significantly reduced in animals treated with LPS.Pretreatment with polymyxin B abated LPS-induced changes in Ca2ϩrelease and [3H]ryanodine binding.Data are presented as mean Ϯstandard error of the mean.*p Ͻ.05as compared with control;**p Ͻ.05as compared with LPS group.Reproduced with permission from Liu et al (92).。
脑卒中 血压控制 pressure control
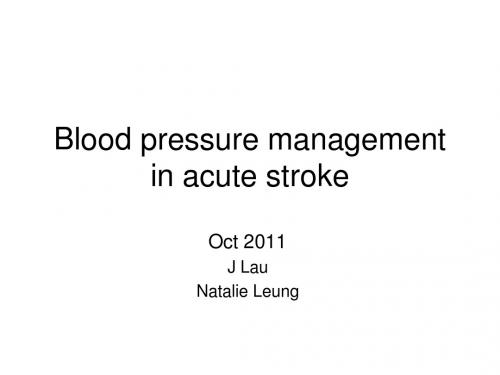
• A normal CBF and oxygen consumption are maintained at the expense of a marked increase in the cerebro-vascular resistance
– Resulting in decreased tolerance for relative hypotension, as the capacity to maintain a constant CBF at the lower end of the BP spectrum is impaired
Cerebral auto-regulation
Cerebral auto-regulation
• Ischaemic penumbra
– Tissue with the lowest CBF would be irreversibly damaged and constitutes the core of the infarct – The regions surrounding the core, the ‘penumbra’, are ischaemic and dysfunctional, but potentially salvageable (with timely re-perfusion) – This hypothesis suggests that BP reduction in the setting of acute ischaemic stroke may worsen hypoperfusion of the penumbra and hasten extension of the infarct
2 recent studies question this relationship…
投资者关注与IPO首日超额收益——基于双边随机前沿分析的新视角
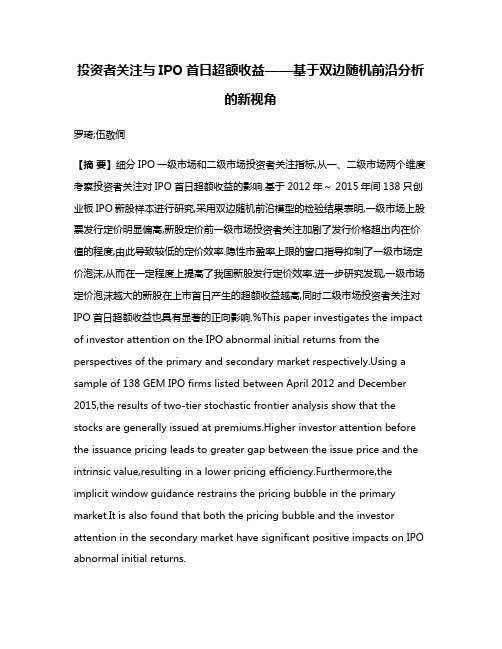
投资者关注与IPO首日超额收益——基于双边随机前沿分析的新视角罗琦;伍敬侗【摘要】细分IPO一级市场和二级市场投资者关注指标,从一、二级市场两个维度考察投资者关注对IPO首日超额收益的影响.基于2012年~ 2015年间138只创业板IPO新股样本进行研究,采用双边随机前沿模型的检验结果表明,一级市场上股票发行定价明显偏高,新股定价前一级市场投资者关注加剧了发行价格超出内在价值的程度,由此导致较低的定价效率.隐性市盈率上限的窗口指导抑制了一级市场定价泡沫,从而在一定程度上提高了我国新股发行定价效率.进一步研究发现,一级市场定价泡沫越大的新股在上市首日产生的超额收益越高,同时二级市场投资者关注对IPO首日超额收益也具有显著的正向影响.%This paper investigates the impact of investor attention on the IPO abnormal initial returns from the perspectives of the primary and secondary market ing a sample of 138 GEM IPO firms listed between April 2012 and December 2015,the results of two-tier stochastic frontier analysis show that the stocks are generally issued at premiums.Higher investor attention before the issuance pricing leads to greater gap between the issue price and the intrinsic value,resulting in a lower pricing efficiency.Furthermore,the implicit window guidance restrains the pricing bubble in the primary market.It is also found that both the pricing bubble and the investor attention in the secondary market have significant positive impacts on IPO abnormal initial returns.【期刊名称】《管理科学学报》【年(卷),期】2017(020)009【总页数】15页(P46-60)【关键词】投资者关注;IPO首日超额收益;随机前沿分析;定价效率;溢价【作者】罗琦;伍敬侗【作者单位】武汉大学经济与管理学院,武汉430072;武汉大学经济与管理学院,武汉430072【正文语种】中文【中图分类】F830首次公开发行的新股在上市首日出现高额回报的异象广泛存在于各国资本市场上,学者们对这一异象进行了不懈探索. 早期关于IPO 首日超额收益异象的研究汇集成一系列基于一级市场抑价的理论,包括信号理论[1]、“赢者诅咒”假说[2]、市场反馈假说[3]以及代理理论[4]等. 一级市场抑价理论假定二级市场是有效的,新股上市后价格会迅速向内在价值回归,但这一观点无法解释互联网泡沫期间激增的IPO首日超额收益. 学者们进而对二级市场有效性产生质疑,并基于投资者非理性提出了二级市场溢价理论,包括卖空限制与意见分歧假说[5]、投资者情绪理论[6,7]、正向反馈交易者理论[8]等.近年来,一些学者基于投资者关注的视角对IPO首日超额收益异象进行解释和预测. 投资者关注理论认为,精力有限的投资者无法对所有有关股票的信息充分反应,而是倾向于购买吸引其注意力的股票,从而推动这些股票价格上涨. 在互联网时代,投资者运用网络手段获取信息,股票信息检索行为反映了其对股票的关注. Da等[9]借助网络技术收集股票搜索行为数据,并据此构建投资者关注指标,实证检验发现投资者关注对IPO首日超额收益具有很强的解释能力. 中国大陆资本市场(以下称为中国资本市场)尚处于成长阶段,其有效程度还远不能与国外成熟市场相比,股票价格容易受到投资者行为因素的影响而产生非理性波动[10,11]. 与发达国家相比,我国资本市场上IPO首日超额收益明显偏高[12],投资者关注理论能否用来解释我国新股上市首日超额收益呢?特别地,我国新股IPO往往吸引大量投资者的注意力,长期存在的超高打新收益更加促使投资者狂热参与[13]. 在二级市场非有效的情况下,投资者对新股的大量关注驱动股票上市后价格上涨似乎是一种合理的解释,但仅从二级市场的角度进行研究可能是不全面的. 投资者关注不仅可能引发新股价格反应过度从而造成二级市场溢价,而且作为在一级市场上可观察到的信息可能对发行人、承销商以及机构投资者的定价报价策略产生影响. 如果投资者关注引发了对新股的狂热需求,明知股票价格将上涨的发行人和承销商为什么还要在定价阶段“把钱留在桌子上”呢?在前人研究的基础上,本文综合一级市场利益相关者行为和二级市场投资者行为考察投资者关注对IPO首日超额收益的影响. 借鉴以往文献中投资者关注的指标设计方法,本文使用百度搜索量指数构建关注度的代理变量,并根据IPO进程细分一级市场投资者关注和二级市场投资者关注. 本文双边随机前沿分析的结果显示,我国新股发行一级市场上存在价格泡沫,并且投资者关注助长了价格泡沫. 我国资本市场上IPO投机氛围浓厚,发行首日超高收益的现象十分突出,发行人和承销商倾向于制定高于股票内在价值的发行价格. 新股定价前的投资者关注是发行人、承销商和机构投资者能够获取的信息,这一信息使得他们能够估计投资者对股票的需求并预期投资者过度反应的程度,从而制定较高的股票发行价格.2014年6月IPO重启之后,新股发行市盈率普遍不超过23倍,本文将这种证监会实际中实施而无明文规定的市盈率管制定义为“隐性”窗口指导. 相比于2012年4月和2014年1月的IPO改革中明确提出的窗口指导标准,隐性窗口指导使得证监会对IPO公司的管制力度进一步加强. 这一管制手段大大约束了发行人和承销商的自主定价能力,从而削弱了投资者关注在新股定价中发挥的作用. 实证结果显示,隐性窗口指导有效减少了新股高定价的情况,并且实施隐性窗口指导后投资者关注对IPO定价效率的不利影响减弱了.在分析一级市场定价效率的基础上,本文进一步考察二级市场投资者关注对IPO 首日超额收益的影响. 投资者关注提高了股票的购买需求但对新股供给的影响微乎其微,同时还加重了投资者关于股票价值的意见分歧. 在卖空限制下,股票价格更多地体现乐观投资者信念,从而导致股价上升. 本文实证结果表明,在控制一级市场定价效率的情况下,二级市场投资者关注显著提高了IPO首日超额收益,并且新股定价泡沫与首日超额收益也呈正相关关系.本文的贡献主要体现在以下3个方面:1)使用双边随机前沿模型对创业板IPO公司的定价效率进行判断,并将一级市场投资者关注作为效率因子引入模型,研究发现新股发行定价存在泡沫,一级市场投资者关注降低了定价效率. 2)结合我国资本市场和监管政策的实际情况,考察了隐性窗口指导实施前后投资者关注对IPO定价效率影响的差异,研究发现隐性窗口指导约束了投资者关注对IPO定价效率的不利影响. 3)从一级市场定价效率和和二级市场有效程度两个维度考察投资者关注对IPO首日超额收益的影响,研究发现一级市场定价效率和二级市场投资者关注均与新股上市首日超额收益显著相关.本文拓展了以往文献仅从上市后股票溢价角度出发的投资者关注理论.Kahneman[14]认为可以视注意力为一种稀缺资源,人们只能在一定能力范围内处理信息,而不可能对所有信息充分反应. 由于精力、资质和判断能力的有限性,投资者无法分析所有股票相关信息,因此在处理引发自身关注的信息过程中容易对相应股票反应过度,从而导致股票价格上涨[15]. 一些学者致力于探讨投资者关注与股票价格行为之间的关系,研究发现两者之间存在显著的正向联系[16-18]. Da等[9]进一步考察了投资者关注对IPO首日超额收益的影响,研究发现投资者关注导致股票价格反应过度,从而产生新股上市首日超额收益. 宋双杰等[19]用我国A股IPO数据对关注理论进行了检验,研究表明投资者关注能较好地解释我国股票市场的首日超额收益异象.已有文献大多基于二级市场溢价的角度考察投资者关注对股票价格的作用机制,而没有考虑投资者关注可能引起一级市场新股发行定价偏离其内在价值,进而对IPO 首日超额收益产生影响. Colaco等[20]指出,发行人、承销商等利益相关方能够观察到个体投资者对新股的关注度信息并产生股票上市后价格上涨的预期,从而有动机制定较高的发行价格. 一些学者意识到,研究 IPO首日超额收益异象应该在测度首日超额收益构成的基础上综合一级市场和二级市场两个维度进行考察. Hunt-McCool等[21]首次将随机前沿模型引入到IPO首日超额收益的分析中,并通过估计股票的内在价值来判断新股发行一级市场是否存在系统性折价. 刘煜辉和沈可挺[22]将随机前沿分析方法应用于我国股票市场,并按照热销和非热销时段划分子样本,研究发现我国新股IPO总体上不存在折价发行的现象. Hu等[23]进一步将IPO 首日超额收益分解为故意折价和市场错误定价两个部分,研究表明首日超额收益主要源自二级市场上投资者非理性造成的溢价.基于生产函数的随机前沿模型只能判断新股发行价格是否低于其内在价值,而不适用于存在定价泡沫的情形. 为了解决这一问题,学者们采用引入成本函数的双边随机前沿模型考察IPO定价效率. 郭海星和万迪昉[24]、Wu[25]发现,我国新股发行价格显著高于其内在价值,一级市场存在价格泡沫,IPO首日超额收益是二级市场溢价与一级市场定价泡沫之间的差值. 陈训波和贺炎林[26]对新股发行定价效率进行测度,并进一步引进效率因子对随机前沿模型进行改进,研究发现招股期间市场回报率等因素会对新股定价效率产生影响.上述研究成果表明,投资者对新股IPO的大量关注导致股票上市后价格迅速上涨,从而产生较高的首日回报. 我国IPO一级市场普遍存在价格泡沫,新股上市首日超额收益不仅取决于二级市场溢价,而且会受到发行定价泡沫的影响. 需要加以说明的是,已有文献没有将投资者关注如何影响一级市场定价效率纳入到IPO首日超额收益的分析框架中. 本文使用双边随机前沿模型对新股上市首日超额收益的构成进行分析,并在此基础上从一级市场和二级市场这两个维度考察投资者关注与IPO 首日超额收益之间的内在联系,从而对投资者关注理论和IPO异象研究进行了有益拓展.我国资本市场有效程度较低,IPO投机氛围浓厚,发行人和承销商往往高价发行股票以获取超额募集资金[27]. 本文的理论分析建立在IPO一级市场存在定价泡沫的基础上,并且价格泡沫越严重,新股发行定价效率则越低.2.1 一级市场投资者关注一些学者研究指出,投资者非理性因素不仅会造成二级市场股票价格异常波动,而且可能影响一级市场利益相关者的定价策略. Cornelli等[28]和Derrien[7]等学者探讨了投资者情绪对IPO发行价格的影响,研究表明散户投资者表现出来的情绪轰动会直接影响发行人和承销商的定价行为,并导致较高的新股发行价格. 李冬昕等[29]从询价机构意见分歧的角度分析我国IPO一级市场定价问题,研究发现询价机构报价过程中意见分歧越严重,一级市场定价过髙的问题就越突出.自2005年以来,我国逐步探索和完善IPO询价制度. 在询价制度下,发行人和承销商通过初步询价确定发行价格区间,并通过向机构投资者等累计投标询价最终决定发行价格. Colaco等[20]研究认为,如果IPO定价前投资者对新股的关注程度较高,发行人和承销商则有动机制定高于内在价值的发行价以获取额外利益. 一级市场投资者关注在一定程度上反映了个体投资者对新股的需求量信息,发行人和承销商能够观测到这一信息并倾向于抬高发行价格区间的上下限[30]. 另一方面,询价机构预期到个体投资者的过度反应可能导致股票上市后价格上涨,因而有动机在累计投标询价中报出高价. 据此,本文提出如下实证假说.假设1 一级市场投资者关注显著提高新股发行价格,并导致IPO定价效率降低.2.2 隐性窗口指导自2012年4月《关于进一步深化新股发行体制改革的指导意见》发布以来,证监会加强了对新股发行市盈率的行政指导. 2014年1月,证监会发布文件《关于加强新股发行监管的措施》,进一步加大了对新股发行市盈率的管制力度,将“同行业上市公司的一个月静态平均市盈率”作为新股发行定价上限的指导标准. 2014年6月IPO重启之后,证监会对IPO公司实施了“隐性”窗口指导. 虽然监管层没有明文规定市盈率上限,但发行价制定过高可能会影响公司获得批文. 从股票市场实际情况来看,隐性窗口指导生效后绝大多数IPO公司的发行市盈率不超过23倍,高市盈率发行的现象明显减少. 相对于2012年4月和2014年1月的市盈率管制政策,这一隐性窗口指导对新股定价的限制作用更为明显.投资者关注对新股定价效率的影响建立在询价制度的基础上,其大小取决于一级市场利益相关者的自主定价能力. 隐性窗口指导实施后,证监会对新股发行定价的管制力度进一步加强. 如果发行人和承销商有动机根据一级市场投资者关注制定定价策略,那么受到隐性窗口指导的IPO公司选择的发行价格应该较低,并且隐性窗口指导对IPO定价泡沫产生抑制作用. 另一方面,由于隐性窗口指导实施后发行人和承销商的自主定价能力受到限制,投资者关注提高新股发行价格的作用也应该受到约束. 基于上述分析,本文提出如下假说.假设2 隐性窗口指导抑制了IPO定价泡沫,并约束了投资者关注对新股定价效率的不利影响.2.3 二级市场投资者关注投资者关注引起股票价格上涨的解释有两种代表性观点:1)股票供求假说. Barber和Odean[16]认为,个体投资者受限于自身的信息处理能力,在构建股票池的过程中无法充分了解所有股票的信息,而只会考虑买进引起自己关注的股票. 然而,投资者的卖出决策只需要对自己所持有的有限只股票进行分析,因此投资者关注对股票供给的影响十分有限. 随着投资者关注度的提高,股票购买需求增加,而股票供给几乎保持不变,最终导致股票价格上涨. 2)异质信念假说. Chemmanur 和Yan[31]认为,关注度的提高可能增加投资者关于股票价值信念的异质性,进而导致投资者意见分歧加重. 当异质信念的投资者受到卖空限制时,悲观投资者将很难进行空头操作,股票价格更多地体现乐观投资者信念而被高估.新股上市前的投资者关注直接反映了股票上市后过度反应的可能性,引起更多关注的新股在交易开始后受到个体投资者的购买压力可能会更大,由此导致股票价格更大幅度的上涨. 由于存在新股发行价格泡沫,IPO一级市场的错误定价可能对新股上市首日价格行为产生影响. 为此本文使用随机前沿模型估计一级市场定价效率,并将其作为控制变量进行回归. 由此,本文提出如下假说.假设3 在控制新股定价效率的基础上,二级市场投资者关注显著提高了IPO首日超额收益.3.1 样本选择与数据来源本文选择的样本区间为2012年4月至2015年12月,研究样本为这一区间内在深交所创业板首次公开发行股票的公司. 创业板市场上机构投资者的投资规模较小,散户投资者是市场交易的主要参与者[32]. 散户投资者获取信息的渠道较为有限,往往需要借助互联网搜索来了解上市公司的相关信息,这使得网络搜索量数据较好地反映了创业板市场上的投资者关注. 本文使用的搜索量数据来源于百度指数,由于部分公司未被百度指数收录,本文剔除搜索量数据缺失的观测值,最终得到的样本为138家创业板IPO公司. 除搜索量数据以外,承销商声誉数据取自证监会网站,市场交易数据和其他财务数据分别来源于同花顺数据库和国泰安数据库.3.2 投资者关注指标设计Da等[9]使用谷歌趋势提供的股票搜索量数据刻画投资者关注度,但谷歌趋势不适用于我国资本市场. 一方面,谷歌搜索在国内受众不高. 谷歌于2010年退出大陆市场,并于2014年在我国内地被禁止访问,其市场份额大幅降低,这导致谷歌趋势提供的搜索量数据不能真实反映中国投资者的关注情况. 另一方面,谷歌趋势只能以周为时间单位提供个股的搜索量数据. 我国大陆股票市场新股申购与上市之间存在一定的时间间隔,在此期间市场上新产生的有关公司IPO的信息可能影响投资者关注和网络搜索行为,周度数据不能很好地刻画这段较短时间间隔内投资者关注的变化.中国网民进行网络搜索的选择较为集中,投资者使用百度搜索获取信息远远超过其他搜索方式*根据中国互联网络信息中心(CNNIC)发布的《2013年中国网民搜索行为研究报告》,百度在渗透率和网民常用率上仍然高居首位,过去半年使用过百度的搜索网民比例达97.9%,在网民常用的搜索引擎中,百度占据了84.5%以上的份额,网民选择较为集中.. 百度是我国第一大搜索引擎,且能够提供搜索量的日度数据,因而成为国内学者广泛采用的数据来源[33-35]. 本文将公司IPO 时的简称作为关键词输入百度指数,并根据抓取到的股票搜索量数据构建投资者关注的代理变量.本文借鉴Colaco等[20]的研究成果,根据新股发行上市的时间进程选取定价日和上市日这两个重要时点. 如图1所示,本文根据定价日前30天(t1)至定价日(t2)这一段时间间隔内的股票搜索量构建一级市场投资者关注指标,并根据定价日(t2)至上市日(t3)这一段时间间隔内的股票搜索量构建二级市场投资者关注指标. 此外,本文选取定价日前30天(t1)至上市日(t3) 整个期间的股票搜索量来反映IPO过程中投资者关注度的总体水平.宋双杰等[19]选定申购日前第9周到第2周为基准期,IPO申购日前1周为观察期,用观察期和基准期内搜索量对数的差值来构建异常搜索量指数(abnormal search volume index),并以此作为投资者关注度的指标. 这种指标设计方法实质上认为基准期的搜索量水平代表了正常的关注程度,以搜索量的相对变化来衡量投资者关注水平,但忽视了基准期内不同新股关注度差异带来的影响[20]. 本文借鉴俞庆进和张兵[32]、张维等[34]的研究成果,使用观察期内搜索量的绝对水平来反映投资者对股票的关注程度,一级市场投资者关注度、二级市场投资者关注度和投资者关注的总体水平指标设计分别如式(1)~式(3)所示PASVI=ln[average(SV It1,…,SVIt2)]SASVI=ln[average(SVIt2,…,SVIt3)]TASVI=ln[average(SVIt1,…,SVIt3)]3.3 模型与变量定义3.3.1 双边随机前沿模型自Aigner等[36]与Meeusen和Broeck[37]分别独立提出随机前沿模型以来,这一分析方法逐步由生产效率测度转而应用于经济金融领域. Hunt-McCool等[21]首次在IPO定价效率研究中运用随机前沿生产模型,模型将新股发行价格视为产出,将影响新股定价的各类因素(公司财务状况、风险和市场环境等)视为投入要素,从而估计出新股价格的有效前沿. 有效价格可以视为对新股内在价值的无偏估计,如果IPO发行价恰好等于股票的内在价值,则其与有效价格的差异仅由随机项造成,不存在系统性的偏差. 如果新股实际发行价格系统性地低于潜在最大发行价格,那么两者之间的偏差将会以残差有偏的形式出现,此时最小二乘法不再适用. 基于生产函数的随机前沿模型如式(4)所示ln Pi=β ln Xi+νi-μi, i=1,…,n式中Pi为新股实际发行价格;Xi是影响公司i新股发行定价的公司特征变量和发行相关变量;β是待估参数. νi是随机误差项,代表实际发行价格与潜在最大价格之间的随机偏差,服从标准正态分布;μi是系统误差项,代表IPO实际发行价格与潜在最大价格之间的系统性偏差,假定其为非负随机变量且与νi相互独立.这两个误差项组成模型误差项.如前所述,随机前沿生产函数模型只能识别一级市场折价发行的情况. 为了检验新股定价泡沫是否存在,本文借鉴郭海星和万迪昉[24]、邹高峰等[38]及Wu[25]的研究成果采用引入成本函数的双边随机前沿模型对IPO定价行为进行分析. 基于成本函数的随机前沿模型如式(5)所示ln P i=β ln Xi+νi+μi, i=1,…,n模型(5)中所有的变量和参数设定均与基于生产函数的随机前沿模型一致,只有μi 的符号发生改变. 在传统的技术效率分析中,μi被假定服从零处截尾的正态分布|,用来描述公司的生产效率. Pitt和Lee[39]运用随机前沿模型估计出每家公司的生产效率,并用这些生产效率的估计值对公司层面的变量进行回归分析,以考察公司生产效率出现差异的原因. Battese和Coelli[40]认为使用效率估计值的回归分析违背了系统误差项μi的同分布假设,并提出一种μi的新分布从而解决了估计不一致的问题. 在此基础上,本文假定μi服从原点截断的正态分布是影响新股定价效率的因素. 如果参数δ的估计值大于0且在一定水平上显著,那么效率因子zi对μi 的均值有正向影响.本文采用极大似然方法对双边随机前沿模型的参数进行估计,由此得到每只股票发行价格偏离有效价格的估计值,并在此基础上对一级市场定价效率进行测度. 为了将生产函数模型和成本函数模型纳入统一的分析框架,本文定义IPO定价非效率(inefficiency)为新股发行价格对有效价格估计值的相对偏离,其计算方法如式(6)所示=|exp(±μi)-1|容易证明,双边随机前沿模型中INEFFi值与μi值呈正向变动关系. μi越大意味着新股发行价偏离有效价格的幅度也越大,一级市场定价效率就越低. 如果μi=0,新股i的发行定价是充分有效的,那么INEFFi等于0,这意味着公司IPO一级市场的定价效率达到最大. 此外,参数用于判断系统性偏差相对于随机误差的偏离程度,γ显著异于零表明系统性偏差是存在的.在变量选取上,本文借鉴Koop和Li[41]的研究成果,将随机前沿模型中的解释变量分为影响新股内在价值的“定价因子”(pricing factor s,Xi)和影响新股定价效率的“效率因子”(misvaluation factors,zi). 为了考察新股发行定价效率产生差异的原因,本文将一级市场投资者关注指标和隐性窗口指导虚拟变量放入效率因子中并作为解释变量. 在对以往文献进行借鉴、比较和判别的基础上,选取其他定价因子和效率因子作为控制变量. 双边随机前沿模型所用到的变量及其定义如表1中的A组所示.3.3.2 超额收益回归模型为了检验二级市场投资者关注对IPO首日超额收益的影响,本文在控制一级市场定价效率的基础上,拟构建如式(7)所示的回归模型AIRi=α+θ1SASVIi+θ2INEFFi+θ3Yi+εi式中AIRi为被解释变量,是新股上市首日超额收益;SASVIi为二级市场投资者关注指标;INEFFi为随机前沿模型估计的定价非效率. Yi为控制变量,其中换手率用来作为投资者情绪的衡量指标[42],超额认购倍数度量市场需求[43]. 此外,本文还控制了上市时的流通股市值、总资产、非流通股比例、承销商声誉等变量[19]. IPO发行至上市存在一定的时间间隔,首日超额收益的计算需要经过这段时间市场收益率的调整. 本文用创业板指数计算市场收益率,并用上市首日新股收益率减去对应期间的市场收益率来计算IPO首日超额收益率. 为了避免上市首日涨跌幅限制所带来的影响,还考虑借鉴Burrowes和Jones[44]的研究成果,用类似的方法计算上市后5日超额收益率. 上市首日和上市后5日超额收益率分别定义如下。
Should Banks Be Diversified
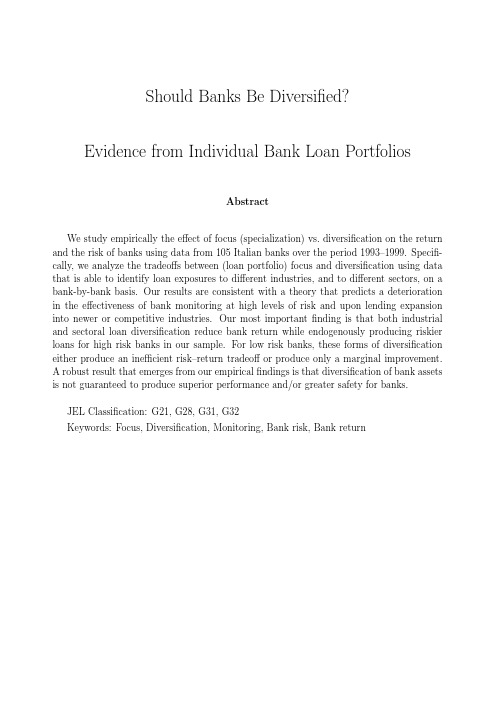
Should Banks Be Diversified?Evidence from Individual Bank Loan PortfoliosAbstractWe study empirically the effect of focus(specialization)vs.diversification on the return and the risk of banks using data from105Italian banks over the period1993–1999.Specifi-cally,we analyze the tradeoffs between(loan portfolio)focus and diversification using data that is able to identify loan exposures to different industries,and to different sectors,on a bank-by-bank basis.Our results are consistent with a theory that predicts a deterioration in the effectiveness of bank monitoring at high levels of risk and upon lending expansion into newer or competitive industries.Our most importantfinding is that both industrial and sectoral loan diversification reduce bank return while endogenously producing riskier loans for high risk banks in our sample.For low risk banks,these forms of diversification either produce an inefficient risk–return tradeoffor produce only a marginal improvement.A robust result that emerges from our empiricalfindings is that diversification of bank assets is not guaranteed to produce superior performance and/or greater safety for banks.JEL Classification:G21,G28,G31,G32Keywords:Focus,Diversification,Monitoring,Bank risk,Bank return1IntroductionShouldfinancial institutions(FIs)and banks be focused or diversified?Does the extent of focus or diversification affect the quality of their loan portfolios?Does diversification, based on traditional portfolio theory wisdom,lead to greater safety for FIs and banks? In this paper,we undertake an empirical investigation of these questions.The evidence we present suggests that,in contrast to the recommendations of traditional portfolio and banking theories,diversification of bank assets is not guaranteed to produce superior return performance and/or greater safety for banks.There are several reasons why the focus vs.diversification issue is important in the context of FIs and banks.First,FIs and banks face several(often conflicting)regulations that create incentives either to diversify or focus their asset portfolios,such as the imposition of capital requirements that are tied to the risk of assets,branching and asset investment restrictions, etc.Hence,from a policy standpoint,it is interesting to ask if FIs and banks benefit or get hurt from diversification of their loan portfolios.In addition,the very nature of an intermediary’s business activities makes the question of focus versus diversification an interesting economic issue to explore.FIs and banks act as delegated monitors in the sense of Diamond(1984),and acquire proprietary information about thefirms they lend to,as noted by Fama(1980,1985),and James(1987),and as modelled by Rajan(1992)and Sharpe(1990).The quality of monitoring and information acquisition is however an endogenous choice of FIs and banks.This choice is governed by the extent of agency conflict between equity holders(bank owners)and creditors of an FI. As explained below,this agency conflict is affected by the downside risk of the FI and by the extent of the FI’s focus or diversification.For the sake of illustration,consider the extreme case where the FI’s downside risk is extremely high so that on an expected basis most benefits from monitoring accrue only to its creditors(uninsured depositors and providers of borrowed funds).In this case,bank owners have little incentive to monitor.All else being equal,the FI’s under-investment in monitoring will be more severe the greater is its downside risk of failure.Under such an incentive structure,can FIs and banks monitor their loans effectively as they expand into different industries and segments of the loan markets?How does the decision to be focused or diversified affect their monitoring incentives and the endogenous quality,i.e.,the risk and the return,of their loans?To answer these questions,we examine data on the asset and loan portfolio composition of individual Italian banks during the period1993–1999.The choice of Italian banks is driven by the availability of detailed data on the industrial and sectoral composition of their balance-sheets.By contrast,in the United States,publicly available data on bank loan1portfolios is restricted to call reports which do not contain such“fine”asset decompositions. In particular,U.S.regulators do not provide a breakdown of individual(or aggregate)bank lending to specific industries or industrial sectors.Instead,the general level of disaggregation is highly coarse in nature,specifically into household sector loans,commercial and industrial loans,etc.We obtain results that are sufficiently striking and robust to warrant a closer look at the wisdom of simply advocating banks to diversify as much as possible.In turn, they suggest that a more careful assessment needs to be made of the costs and benefits of diversification in banking in general.Some of these issues have been examined at a theoretical level in a recent paper by Winton (1999).Traditional arguments based on Diamond(1984)suggest that banks should be as diversified as possible.This precludes any agency problem between bank owners and bank creditors.In practice,however,banks cannot fully diversify all their risks.Winton presents a theoretical framework that allows for a residual agency problem between bank owners and bank creditors and investigates the merit of the proverbial wisdom of not putting all your eggs in one basket.1The model provides a number of testable empirical hypotheses that are central to the focus versus diversification debate in banking.We state below the empirical hypotheses we test and discuss the economic intuition for them in Section2.H.1The relationship between bank return and diversification is non–linear in bank risk (inverted U–shaped).To be precise,diversification across loan sectors helps a bank’s return most when loans have moderate exposure to sector downturns(downside risk)2;when loans have low downside risk,diversification has little benefit;when loans have sufficiently high downside risk,diversification may actually reduce returns.H.2A bank’s monitoring effectiveness may be lower in newly entered and competitive sectors,and thus,diversification can result in an increase in the downside risk of the bank’s loan portfolio.Broadly speaking,these hypotheses reflect the view that a bank’s credit risk depends on its monitoring incentives(and effectiveness)as well as on its degree of portfolio diversification.1Winton motivates the issue by comparing the following two advices:“It’s the part of a wise man to keep himself today for tomorrow and not venture all his eggs in one basket”by Miguel de Cervantes(Don Quixote de la Mancha,1605),and,Behold the fool saith“Put not thine eggs in one basket”-which is but a manner of saying,“Scatter your money and attention”;but the wise man saith“Put all your eggs in one basket and watch that basket”by Mark Twain(Pudd’nhead Wilson,1894).2By portfolio“downside risk,”we mean the likelihood that the portfolio return will be lower than a given threshold(e.g.,level of deposits in the bank’s capital structure),an event that constitutes a“default.”In the paper,we have employed several measures of downside risk,both expected and unexpected,based on their availability and measurability.2Overall,our results provide support for these two hypotheses.We measure focus using the Herfindahl index for a bank’s(i)non-financial and housing loan portfolio(I–HHI),(ii)overall asset sector portfolio(A–HHI),and(iii)geographical portfolio(G–HHI).3Thus,a decrease in HHI implies an increase in diversification and a reduction in focus.Since geographic focus measure,G-HHI,is notfine enough to produce a sufficient variation across banks,we focus our study on industrial and sector focus measures,I-HHI and A-HHI,respectively.Wefind that the relationship between focus and bank return is non–linear in the risk of the bank and may in fact be U–shaped as implied by hypothesis H.1.Specifically,increased industrial diversification appears to decrease return for banks with high risk levels,and produces either a relatively smaller decrease or only a small increase in return for banks with moderate risk levels.The effect of increased asset sectoral diversification is analogous,hurting returns of high risk banks and producing only a marginal effect on returns of low risk banks.These results are robust to measurement of bank risk and to endogeneity of focus measures.Specifically,we proxy for bank risk using a variety of measures:(i)realized risk measured as a bank’s doubtful and non–performing loans to assets ratio(DOUBT),(ii)unexpected risk measured as the sample standard deviation of DOUBT and the total as well as the idiosyncratic volatility of the bank’s stock market returns(if it is publicly traded),and (iii)expected risk measured as the ratio of loan-loss provisions to assets.Our results are qualitatively robust across these different measures of bank risk.We also correct for the endogeneity of industrial and asset sectoral focus by conducting a simultaneous equations estimation of bank return and bank focus where additional variables are employed to serve as instruments that explain focus.The U–shaped relationship of hypothesis H.1is robust to this correction,and in fact,statistically stronger in most cases.We test hypothesis H.2by examining endogenous loan quality(risk)and treating risk as a dependent variable that is affected by the extent of focus(diversification).Our empirical results suggest that increased focus in terms of industrial sector or asset sectoral exposure (high values for I–HHI and A–HHI)improves loan quality(reduces risk).This effect is also robust to treating focus measures as endogenous variables.Further,the reduction in risk upon an increase in industrial focus is greater,the greater the competition for loans that the bank faces in the industries it lends to.We alsofind some evidence supporting the hypothesis that when banks enter as lenders into“newer”industries or industries where they had less exposure before,there is a contemporaneous deterioration in a bank’s loan quality(increase in its risk).4This deterioration is smaller,the greater the industrial focus of the bank’s loan3The Herfindahl index is the sum of the squared weights corresponding to a bank’s exposure to different in-dustries,sectors,or geographical areas,a higher value corresponding to greater focus or lower diversification.4We use the qualifier“newer”for industries in the sense that they are newer to the bank,i.e.,previous exposures of the bank to these industries had been lower or non–existent,rather than being newer in the sense of technological changes produced by the industries.3portfolio.These results underscore the importance of“watching the basket”of loans and the improvement in monitoring effectiveness of banks from specialization.From the combined results on bank loan return and risk,we conclude that increased industrial and sectoral loan diversification both result in an inefficient risk–return tradeofffor the(Italian)banks in our sample,the effect being strongest for banks with relatively high levels of risk.5We conduct additional robustness checks by separating the sample into state-owned and private banks,into money center national and non-national banks,and finally,into banks that are members of a consortium group and those that are not.The results are supportive of our main conclusions.These results have important and direct implications for the optimal size and scope of banks.While traditional banking theory based on a delegated monitoring argument recommends that it is optimal for a bank to be fully diversified across sectors or“projects”(see,for example,Boyd and Prescott,1986),our results suggest that there are diseconomies of scope that arise through weakened monitoring incentives and a poorer quality loan portfolio when a risky bank expands into additional industries and sectors.This complements the agency theory based analysis of the boundaries of a bank’s activities as proposed in Cerasi and Daltung(2000),Stein(2002)and Berger et al.(2001).6From a normative standpoint, our results sound a cautionary note to the adoption of regulatory mechanisms that encourage bank–level portfolio and/or activity diversification,or attempt to measure credit portfolio risk through traditional diversification measures.The issue of focus versus diversification has not been addressed thoroughly in the con-text offinancial institutions and banks,although it has a long history in the corporate finance literature.7This is primarily because it has been difficult to obtain bank-level(cross-5While we do not report these results due to the lack of richness in the measure of geographic diversifi-cation,wefind that geographic diversification does result in an improvement in the risk–return tradeofffor banks with low or moderate levels of risk.6We believe that the agency theories based on conflicts acrossfirm segments proposed in corporate finance to explain the poor performance of conglomerates cannot completely explain the perverse effect of diversification on bank returns and risk.A bank’s lending to different industries is much more centralized than is the operation of a typical conglomerate’s operating segments.Stein(2002)and Berger et al.(2001), however,tie incomplete contracting to the inability of large banks to process“soft”information about their borrowers.This potentially leads to diseconomies of scale for FIs and banks.7The early evidence in this literature seemed to suggest that diversification destroys value on average leading to what is popularly known as the“diversification discount.”See,for example,Lang and Stulz (1994),Comment and Jarrell(1995),Berger and Ofek(1995),Lins and Servaes(1999).The issue of there being a discount on average is,however,disputed.Campa and Kedia(2000),Villalonga(2001),Graham, Lemmon and Wolf(2002),Maksimovic and Phillips(2002)examine the endogeneity of the decision to focus or diversify and question this early evidence,both on empirical as well as economic grounds.Several theories have been proposed to explain why focus(diversification)may affectfirm value.These are based on managerial risk-aversion(Amihud and Lev,1981),agency problems between managers and4sectional)portfolio data and construct measures of industrial and geographical diversification that are as“fine”or“micro”as those employed in this paper.Using somewhat coarser measures,Hughes,Lang,Mester and Moon(1996),Saunders and Wilson(2001),and Berger and DeYoung(2001)examine geographical diversification.Caprio and Wilson(1997)examine cross–country evidence for a relationship between on–balance sheet concentration and bank insolvency.Klein and Saidenberg(1998)present portfolio simulations to compare lending by multi–bank bank holding companies and their pro forma “pure–play”benchmark banks.Berger,Demsetz and Strahan(1999)find that consolidation infinancial services industry has been consistent with greater diversification of risks on average but with little or no cost efficiency improvements.DeLong(2001)examines the merger offinancialfirms in the U.S.andfinds that bank mergers that are focusing in terms of geography and activity produce superior economic performance relative to those that are diversifying.Finally,Stiroh(2002)finds that during the period from late1970s to2001,a greater reliance on non-interest income by the U.S.banks,particularly on trading revenue, is associated with higher risk and lower risk-adjusted profits at the individual bank level.Section2discusses the economic intuition behind the empirical hypotheses.Section3 describes our data.Section4formalizes the hypotheses and presents our empirical results. Section5provides a discussion and concludes.2Empirical HypothesesWe restate the empirical hypotheses of Winton(1999)and provide their economic underpin-nings.The essence of Winton’s model lies in understanding that the quality of bank loan portfolios is endogenous:it is determined,in part,by the levels of monitoring induced by a change in the bank’s focus or diversification.H.1The relationship between bank return and diversification is non–linear in bank risk (inverted U–shaped).To be precise,diversification across loan sectors helps a bank’s return most when loans have moderate exposure to sector downturns(downside risk);when loans have low downside risk,diversification has little benefit;when loans have sufficiently high downside risk,diversification may actually reduce returns.INSERT FIGURE1HERE.shareholders(Denis,Denis and Sarin,1997,and Cornett et al.,2001),the inefficiency of internal capital markets(Scharfstein and Stein,2000),and power-struggles between different segments of afirm(Rajan, Servaes and Zingales,2000).5From traditional portfolio theory,we know that diversification increases the central ten-dency of the distribution of a loan portfolio.However,as Winton(1999)notes,when debt is risky and the central tendency of distribution is low relative to the level of debt,diversifica-tion can in fact increase the probability of default.This would occur for example if downside risk of bank loans is substantial.For the sake of illustration,Figure1plots the cumulative probability function for two normal distributions with different standard deviations and with a common mean of zero.Suppose these distributions correspond to(suitably scaled)two possible distributions for realization on bank loans.Suppose further that the level of debt varies along the x-axis.If the level of debt is to the left of zero(under a suitable scale),e.g.,at x=−1,then a decrease in standard deviation,by reducing the likelihood of events in the left tail of the distribution(the“default”states),reduces the probability of default.However,if the level of debt is to the right of zero,e.g.,at x=1,then a decrease in standard deviation,by reducing the likelihood of events in the right tail of the distribution(the“no-default”states),in fact increases the probability of default.The left skewed nature of a typical loan portfolio’s return distribution implies that the level of debt,in fact,may not need to be too high for this effect to arise.An additional impact bolstering hypothesis(H.1)arises from the interaction of this per-verse effect of diversification on bank risk and the bank’s monitoring incentives.The conflict of interest between bank owners and bank creditors(similar to the equity holder vs.creditor conflictsfirst described in Jensen and Meckling,1976,and Myers,1977)implies that an increase in the probability of default reduces the incentives of bank owners to monitor their loans.If the loan portfolio has high downside risk,then an improvement in loan monitoring and,in turn,in loan quality produces greater benefits to the creditors than to the bank owners.Since the cost of monitoring is borne by the bank owners(the residual claimants), it follows that if the loan portfolio has high downside risk,then an increase in diversification leads to weaker incentives for bank owners to monitor loans.This,in turn,leads to lower bank returns reinforcing hypothesis H.1.H.2A bank’s monitoring effectiveness may be lower in newly entered and competitive sectors,and thus,diversification can result in an increase in the downside risk of the bank’s loan portfolio.There are at least three reasons why this might arise.First,banks may lack the moni-toring expertise in lending to a new sector when learning costs are present.Second,when the loan sector to which banks migrate is already being supplied with credit by other banks, the new bank entrants may be subject to adverse selection and a“winner’s curse”effect.88In addition to Winton(1999),several papers have discussed the adverse effect of competition on bank6This suggests that diversification could lower returns on bank loans and increase the risk of failure to a greater degree when the sectors into which the bank expands are subject to greater competition.Third,diversification can cause a bank to grow in size,subjecting it to agency–based scale inefficiencies discussed in the corporatefinance literature.Thus,diversification per se is no guarantee of a reduced risk of failure.By the same token,regulatory requirements to diversify are no assurance of greater banking system safety or stability.9In this paper,we empirically test these hypotheses using bank-by-bank data on focus(diversification),return,and risk for Italian banks.3Data3.1Data sourcesData for the industrial,asset,and geographic decompositions of the portfolios of Italian banks in our study are taken from the regulatory reports submitted by these banks to the Bank of Italy,the Italian Bankers’Association(ABI),and the Interbank Deposit Protection Fund of Italy(FITD).The latter is the Italian equivalent of the U.S.Federal Deposit Insurance Corporation(FDIC).Our sample starts with a base of105primarily commercial banks that reported their asset portfolio and other data during the entire1993–1999period.The sample period starts in1993since the banking law of August27,1993(consolidating act) marked a regime shift in the Italian banking structure.It revolutionized the Italian banking system by encouraging the new model of a“full-service”financial institution.It eliminated the distinction between specialized lending institutions(medium and long-term credit)and retail banks(short-term credit),as opposed to the pre-existing system of specialized banks.A complete list of the banks and the ones that are traded publicly during our sample period is shown in Appendix A along with the average size of each bank over the sample period.These105banks constitute over80percent of the total banking assets of Italy.A few of the banks in our sample undertook acquisitions of other banks.The data set, however,does not provide any details as to which were these acquiring banks and which banks they acquired.Furthermore,the data set does not include foreign bank operations in Italy.Over our sample period,the foreign bank penetration of the Italian banking market is however weak largely due to the prohibition on foreign banks from accepting deposits of Italian residents.loan quality.These include Gehrig(1998),Dell’Arricia,Friedman,and Marquez(1999),Boot and Thakor (2000),and Hauswald and Marquez(2002)for theory,and Shaffer(1998)for empirical results.9For example,in the U.S.,regulations restrict a bank’s lending to any one counterparty to a maximum of15%of that bank’s capital.7In terms of size,8of these105banks are“very large”(as defined by the Bank of Italy), 7are“large,”15are“medium,”and the remaining75are“small.”In terms of geographical scope of banking activities,9of these banks are“national,”18are“regional,”13are“intra–regional,”10are“local,”and the remaining55are“provincial.”Finally,34of these banks are publicly traded and62of them were state–owned at the beginning of1993.10While there are natural differences between the banking sectors of any two countries, there are several dimensions along which the Italian banking system is similar to that in the U.S.:(1)Unlike other banking systems in Continental Europe,Italy has a large number of banks(about850at the beginning of our sample)giving rise to a less concentrated banking system like that of the U.S.(2)The branching restrictions on banks in Italy were removed in 1990as they were in the U.S.in the mid1980s.(3)There has been a wave of consolidation in the banking system in1990s mirroring that in the U.S.(4)The banking system comprises of a few very large banks and a large number of medium-to-small sized banks as in the U.S. In addition,the risk levels of Italian banks in our sample exhibit economically significant variability,from being very safe to being very risky,which lends an element of robustness and generality to our results.Finally,Italy differs from the U.S.in that many of its banks are state-owned although state-ownership has been steadily declining over the past decade following the Amato-Carli law.However,our results are found to hold for both the privately-owned and the state-owned samples of banks(see Section4.4).These stylized facts and the use of Italian banking data to address other important economic issues such as the benefit of relationship banking(Degatriache et al.,2000)and the effect of bank mergers on loan contracts(Sapienza,2002a)lead us to believe that our results would generalize to banking sectors of other countries,including the U.S.11For each bank in our sample,data is available to calculate the following portfolio decom-positions:1.A disaggregated industrial sector decomposition based on each bank’s topfive indus-trial sector exposures with a sixth exposure comprising of the sum of the remaining exposures,where the exposures could be to any of the23industries among:(1)Agri-cultural,Forestry,and Fishing products,(2)Energy products,(3)Iron and non–iron Material and Ore,(4)Ores and products based on non-metallic minerals,(5)Chemicals,(6)Metal products,apart from machinery and means of conveyance,(7)Agriculturaland Industrial machinery,(8)Office,EDP Machinery,and others,(9)Electric mate-10We are very grateful to Paola Sapienza for supplying us the state–ownership dummy for our sample based on her work on Italian banks in Sapienza(2002b).11Descriptions of the Italian banking sector can be found in Degatriache et al.(2000)and Sapienza(2002a). Industry perspectives on the developments of the Italian banking system can also be found in BNP Paribas (2001)and Goldman Sachs(2001).8rial,(10)Transport,(11)Food products,Beverages,and Tobacco-based products,(12) Textile,Leather,Shoes,and Clothing products,(13)Paper,Publishing,and Print-ing products,(14)Rubber and Plastic products,(15)Other Industrial products,(16) Construction,(17)Services trade and similar,(18)Hotel and Publicfirms products,(19)Internal Transport services,(20)Sea and Air Transport,(21)Transport relatedservices,(22)Communication services,and(23)Other Sales related services.Note that in aggregate these exposures(collectively defined in the data as Non–financial and Household exposures)constitute the dominant part of each bank’s portfolio.2.A broad asset sector decomposition based on exposures to(1)Sovereigns,(2)Othergovernmental authorities,(3)Non–financial corporations,(4)Financial institutions,(5)Households,and(6)Other counterparties.Note that the size of bank lending to a particular sector or industry in our data set is net of loans that are already classified as either doubtful or non–performing.Unfortunately,our data set does not provide more detailed loan-by-loan or borrower-by-borrower information within these decompositions.The Financial Statement variables and capital structure variables are obtained from the Bank of Italy and Bankscope data bases.Stock market data items for the34banks that are publicly traded were taken from the Datastream and Milan Stock exchange information bases on Italian Banks.A few banks had to be discarded from the sample due to missing values of relevant variables,e.g.,doubtful and non–performing loans.3.2Construction of Herfindahl indicesWe measure focus(diversification)by employing a Hirschman Herfindahl Index(HHI)mea-sure.HHI is the sum of the squares of exposures as a fraction of total exposure under a given classification.In our case,we construct two different kinds of HHI’s,which consist of Industrial and Household sector HHI,more simply referred to as Industrial sector HHI (I–HHI)and Broad Asset sector HHI(A–HHI).I–HHI is based on the5top industries where loans were made for each bank.The6th exposure considers the rest of the industrial loan portfolio.For the6th exposure,we em-ployed two conventions:first,where the6th exposure is treated as a separate“hypothetical”industry,and second,where the6th exposure is treated as being equally divided among the remaining18industries.Our results were not sensitive to this choice.Hence,we report results with I–HHI computed using the6th exposure as a hypothetical industry.Thus,if the proportional exposures to six industries are X1,X2,X3,X4,X5,and X6,respectively,then I–HHI equals 6i=1(X i/Q)2,where Q= 6i=1X i.Note that the HHI has a maximum of1 when all loans are made to a single industry.9。
corporate ownership around the world
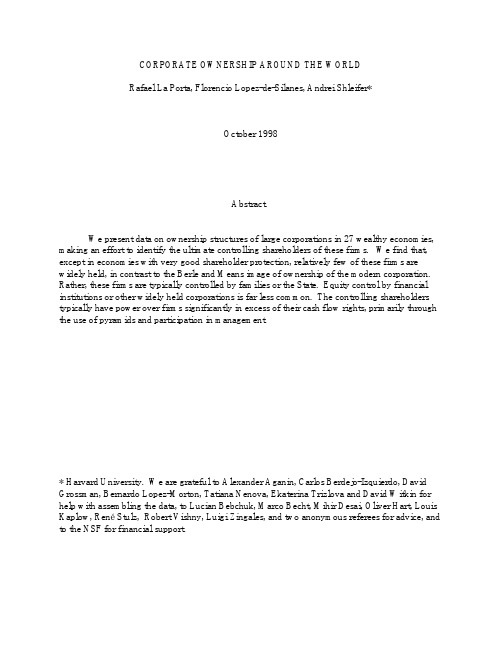
CORPORATE OWNERSHIP AROUND THE WORLDRafael La Porta, Florencio Lopez-de-Silanes, Andrei Shleifer*October 1998Abstract.We present data on ownership structures of large corporations in 27 wealthy economies, making an effort to identify the ultimate controlling shareholders of these firms. We find that, except in economies with very good shareholder protection, relatively few of these firms are widely held, in contrast to the Berle and Means image of ownership of the modern corporation. Rather, these firms are typically controlled by families or the State. Equity control by financial institutions or other widely held corporations is far less common. The controlling shareholders typically have power over firms significantly in excess of their cash flow rights, primarily through the use of pyramids and participation in management.* Harvard University. We are grateful to Alexander Aganin, Carlos Berdejo-Izquierdo, David Grossman, Bernardo Lopez-Morton, Tatiana Nenova, Ekaterina Trizlova and David Witkin for help with assembling the data, to Lucian Bebchuk, Marco Becht, Mihir Desai, Oliver Hart, Louis Kaplow, René Stulz, Robert Vishny, Luigi Zingales, and two anonymous referees for advice, and to the NSF for financial support.In their 1932 classic, “The Modern Corporation and Private Property,” Adolph Berle and Gardiner Means called attention to the prevalence of widely held corporations in the United States, in which ownership of capital was dispersed between small shareholders, yet control was concentrated in the hands of managers. For at least two generations, their book fixed the image of the modern corporation as one run by professional managers unaccountable to shareholders. The book stimulated an enormous “managerialist” literature on the objectives of such managers, including the important work of Baumol (1959), Marris (1964), Penrose (1959), and Williamson (1964), as well as Galbraith’s (1967) popular and influential account. More recently, the modern field of corporate finance has developed around the same image of a widely held corporation, as can be seen in the central contributions of Jensen and Meckling (1976) or Grossman and Hart (1980). The Berle and Means image has clearly stuck.In recent years, several studies have begun to question the empirical validity of this image. Eisenberg (1976), Demsetz (1983), Demsetz and Lehn (1985), Shleifer and Vishny (1986), and Morck, Shleifer and Vishny (1988) have shown that, even among the largest American firms, there is a modest concentration of ownership. Holderness and Sheehan (1988) have found several hundred publicly-traded firms with majority (greater than 51 percent) shareholders in the United States. Holderness, Kroszner and Sheehan (1998) have moreover found that management ownership in the United States today is higher than it was when Berle and Means wrote their study.Studies of other rich countries discovered more significant concentration of ownership in Germany (Franks and Mayer (1994), Gorton and Schmid (1996)), Japan (Prowse (1992), Berglof and Perotti (1994)), Italy (Barca (1995)), and seven OECD countries (European CorporateGovernance Network (1997)). In developing economies, ownership is also heavily concentrated (La Porta et al. (1998a)). This research suggests not only that, in many countries, large corporations have large shareholders, but also that these shareholders are active in corporate governance (e.g., Kang and Shivdasani (1995), Yafeh and Yosha (1996)), in contrast to the Berle and Means idea that managers are unaccountable.1Thanks to this research, the Berle and Means image of the modern corporation has begun to show some wear. Still, we have relatively little systematic evidence on the ownership patterns of large publicly traded firms in different countries, and lack a comparative perspective on the relevance of the Berle and Means description of the firm. This paper attempts to provide some such evidence. Specifically, we look at the ownership structures of the twenty largest publicly traded firms in each of the 27 (generally richest) economies, as well as of some smaller firms so that we can keep size constant across countries. We focus on the largest firms in the richest economies precisely because, for these firms, the likelihood of widely-dispersed ownership is the greatest (this is indeed the case). Our principal contribution is to find wherever possible the identities of the ultimate owners of capital and of voting rights in firms, so when shares in a firm are owned by another company, we examine the ownership of that company, and so on.2 For most countries, this is the only way to understand the relationship between ownership and control. These data enable us to address, in a comparative perspective, four broad questions related to the Berle and Means thesis.First, how common are widely held firms in different countries, as opposed to firms that have owners with significant voting rights? Second, to the extent that firms have significant owners, who are they? Are they families, the government, financial institutions, or other --possibly widely held -- firms? How often do banks control companies -- a big issue in corporate finance in light of the extensive discussion of the German corporate governance model? Third, how do these owners maintain their power? Do they use shares with superior voting rights that enable them to exercise control with only limited ownership of capital? Alternatively, do they create complicated cross-ownership patterns to reduce the threat to their control? Or do they build pyramids, whereby they control firms through a chain of companies -- another form of separating ownership of capital and control? By answering these questions empirically, we hope to provide a comprehensive description of ownership patterns of large firms in rich countries.The fourth question we address is: what explains the differences between countries in their ownership patterns? Why, for example, is the Berle and Means image of a widely held firm so much more descriptive of the United States than of Mexico or Italy? Our earlier work (La Porta et al. (1997, 1998a)) suggests that the widely held Berle and Means corporation should be more common in countries with good legal protection of minority shareholders (which are often rich common law countries). In these countries, controlling shareholders have less fear of being expropriated themselves in the event that they ever lose control through a takeover or a market accumulation of shares by a raider, and so might be willing to cut their ownership of voting rights by selling shares to raise funds or to diversify. In contrast, in countries with poor protection of minorities, losing control involuntarily and thus becoming a minority shareholder may be such a costly proposition in terms of surrendering the private benefits of control that the controlling shareholders would do everything to keep control. They would hold more voting rights themselves, and have less interest is selling shares in the market.3 In view of this analysis, we assess the relationship between ownership concentration and minority shareholder protection interms of the voting rights of the principal shareholders rather than their cash flow rights.4 Relatedly, we evaluate the relationship between shareholder protection and the incidence of various control arrangements, including cross-shareholdings, differential voting rights and pyramids. The theory in this area is not completely developed, but some articles do help us think about the data. Grossman and Hart (1988) and Harris and Raviv (1988) suggest that deviations from one-share-one-vote should be larger when private benefits of control are higher, which must be the case in countries with poorer shareholder protection. Wolfenzon (1998) argues that pyramids should also be more common in countries with poor shareholder protection, because it is easier for controlling shareholders there to make minority shareholders in existing firms pay for starting up new firms as partial subsidiaries without fully sharing with these minorities the benefits of a new venture. Pyramids and multiple classes of stock are of course two different ways of separating cash flow and control rights in firms.The controlling shareholders face strong incentives to monitor managers and maximize profits when they retain substantial cash flow rights in addition to control. These incentives, emphasized by Jensen and Meckling (1976) and Shleifer and Vishny (1986), also restrain the diversion of corporate resources by the controlling shareholders, and enhance the value of minority shares.In our empirical work, we find that the Berle and Means corporation is far from universal, and is quite rare for some definitions of control. Similarly, the so-called German model of bank control through equity is uncommon. Instead, controlling shareholders -- usually the State or families -- are present in most large companies. These shareholders have control rights in firms in excess of their cash flow rights, largely through the use of pyramids, but they also participate inmanagement. The power of these controlling shareholders is evidently not checked by other large shareholders. The results suggest that the theory of corporate finance relevant for most countries should focus on the incentives and opportunities of controlling shareholders to both benefit and expropriate the minority shareholders.The next section of the paper describes our data, and presents a number of examples of ownership patterns in particular companies. Section II presents the basic results on the incidence of various ownership structures around the world. Section III concludes.I. Data.A. Construction of the DatabaseThis paper is based on a new database of ownership structures of companies from 27 countries. As we detail below, the data on corporate ownership are often difficult to assemble, and this limitation determines many of the choices we make. We generally use the richest countries based on 1993 per capita income, but exclude a number of them that do not have significant stock markets (e.g., Kuwait, United Arab Emirates, Saudi Arabia).5 For each country, we collect two samples of firms. The first sample consists of the top 20 firms ranked by market capitalization of common equity at the end of 1995 (with some exceptions detailed below). This sample runs into the objection that the largest companies in some countries are much larger than the largest companies in other countries. This is a particularly serious issue for a study of ownership because larger companies presumably have less concentrated ownership, and hence we should be careful that our measures of block ownership do not simply proxy for size. Accordingly, the second sample collects, whenever possible, the smallest 10 firms in each countrywith market capitalization of common equity of at least $500 million at the end of 1995. We call the first sample “large firms” and the second sample “medium firms.” For countries with small stock markets, the two samples intersect. Moreover, for six countries (Argentina, Austria, Ireland, New Zealand, Greece, and Portugal) we do not have 10 publicly traded firms with capitalizations above $500 million. Overall, we have 540 large firms in the large firm sample, and a total of 691 different firms (out of a possible maximum of 810).6There are a few further restrictions on these samples of companies. First, for both samples, we exclude all affiliates of foreign firms. A firm is defined as an affiliate of a foreign company if at least 50 percent of its votes are directly controlled by a single foreign corporate owner. In addition, we exclude banks and utilities from the sample of medium firms, to prevent the domination of this sample by these two industries. Finally, by construction, neither sample includes companies that are owned either wholly privately or wholly by the government, and therefore are not listed. This restriction biases our results toward finding fewer firms with significant government and family ownership than actually exist.As a rule, our companies come from WorldScope database. In four countries for which WorldScope coverage is limited (Argentina, Israel, Mexico, and the Netherlands), we use other sources (see Appendix A for data sources). We generally rely on annual reports, 20-F filings for companies with American Depositary Receipts (ADRs), proxy statements, and -- for several countries -- country-specific books that detail ownership structures of their companies. We also found the INTERNET to be very useful because many individual companies (e.g., in Scandinavia), as well as institutions (e.g., the Paris Bourse and The Financial Times) have Websites that contain information on ownership structures. Virtually all of our data are for 1995and 1996, though we have a few observations where the data come from the earlier years, and a few from 1997. Since ownership patterns tend to be relatively stable, the fact that the ownership data do not all come from the same year is not a big problem.For several countries, our standard procedures do not work because disclosure is so limited. For Greece and Mexico, we can not work with the 20 largest firms because we do not have enough ownership data. For Greece, we take the 20 largest corporations for which we could find ownership data (mostly in Bloomberg). For Mexico, we take the 20 largest firms that have ADRs. For Israel, we rely almost entirely on Lexis/Nexis and INTERNET sources. For Korea, different sources offer conflicting information on corporate ownership structures of chaebols. We were advised by Korean scholars that the best source for chaebols contains information as of 1984, so we use the more stale but reliable data.To describe control of companies, we generally look for all shareholders who control over 10 percent of the votes. The cutoff of 10 percent is used because (1) it provides a significant threshold of votes; and (2) most countries mandate disclosure of 10 percent, and usually even lower, ownership stakes. For most countries and companies, we have some information on smaller shareholdings, but focus only on shareholders who control over 10 percent of the votes. In many cases, the principal shareholders in our firms are themselves corporate entities and financial institutions. We then try to find the major shareholders in these entities, then the major shareholders in the major shareholders, and so on, until we find the ultimate controllers of the votes. In some cases, the ultimate controller is the State, a widely held financial institution, or a widely held corporation. In other cases, it is an individual or a family. We do not attempt to get inside families, and assume that every family owns and votes its shares collectively.B. Definitions of VariablesWe ask whether firms have substantial owners. We do not try to measure ownership concentration, because a theoretically appropriate measure requires a model of the interactions between large shareholders, which we do not have. Rather, we try to define owners in a variety of ways, summarized in Table I and discussed in this subsection. In the following subsection, we illustrate these definitions using several companies from our sample.Our definitions of ownership rely on voting rights rather than cash flow rights. Recall that Berle and Means want to know who controls the modern corporation: shareholders or managers. We too want to know whether corporations have shareholders with substantial voting rights, either directly or through a chain of holdings. This idea motivates our definitions.We divide firms into those that are widely held and those with ultimate owners. We allow for five types of ultimate owners: 1) a family or an individual, 2) the State, 3) a widely held financial institution such as a bank or an insurance company, 4) a widely held corporation, or 5) miscellaneous, such as a cooperative, a voting trust, or a group with no single controlling investor. State control is a separate category because it is a form of concentrated ownership in which the State uses firms to pursue political objectives, while the public pays for the losses (Shleifer and Vishny (1994)). We also give widely held corporations and widely held financial institutions separate categories as owners because it is unclear whether the firms they control should be thought of as widely held or having an ultimate owner. A firm controlled by a widely held corporation or financial institution can be thought of either as widely held since the management of the controlling entity is not itself accountable to an ultimate owner, or as controlled by that management. For these reasons (and because bank ownership is of independentinterest), we keep these categories separate.As a first cut, we say that a corporation has a controlling shareholder (ultimate owner) if this shareholder’s direct and indirect voting rights in the firm exceed 20 percent. A shareholder has x percent indirect control over firm A if: (1) it directly controls firm B which, in turn, directly controls x percent of the votes in firm A; or (2) it directly controls firm C which in turn controls firm B (or a sequence of firms leading to firm B each of which has control over the next one, i.e., they form a control chain), which directly controls x percent of the votes in firm A. Table I provides a more precise definition. The idea behind using 20 percent of the votes is that this is usually enough to have effective control of a firm. Indeed, below we present evidence that, in the majority of cases, our ultimate owners are also part of the management of the firm.In the simplest case, each sample firm would have an ultimate owner of the above five types. There may, alternatively, be a legal entity that has over 20 percent voting rights in our sample firm, which itself has a shareholder with over 20 percent of the votes, and so on. We classify all firms that do not have such a 20 percent chain of voting rights as widely held, and firms with such a chain as having owners. On this definition, if company B has 23 percent of the votes in company A, and individual C has 19 percent of the votes in B, we still call A controlled by a widely held corporation (unless C has additional indirect control in A -- see the discussion of Korea below). In addition to the definition of ultimate owners using this 20 percent of votes rule, we consider a second definition that relies on a chain of over 10 percent of voting rights.The above definitions give us a reasonably conservative way to answer the question: do firms have shareholders with a substantial amount of control, or ultimate owners? But this is not the only interesting aspect of ownership. To evaluate the potential for agency problems betweenultimate owners and minority shareholders, we also want to know whether the cash flow ownership rights of the controlling shareholders are substantially different from their voting rights. One way in which the ultimate owners can reduce their ownership below their control rights is by using shares with superior voting rights; another way is to organize the ownership structure of the firm in a pyramid. Finally, the ultimate owners might wish to solidify their control through cross-shareholdings: having the firm own shares in its shareholders.We describe the role of multiple classes of shares in the simplest possible way. For each firm in the sample, we ask what is the minimum percentage of its capital at par value that the immediate shareholder (who might be different from the ultimate owner) needs to own to have 20 percent of the voting rights under the existing structure of share types of that firm (as opposed to what might be allowed by law). For example, if a firm has 50 percent of its capital in the form of shares that have 100 percent of voting rights, and 50 percent in the form of non-voting shares, we would say that a shareholder must own at least 10 percent of capital (in the form of the first kind of shares) to have 20 percent of the votes. Note that we are only computing this measure for the firms in the sample; we do not capture a deviation from one-share-one-vote if a publicly held corporate shareholder in our sample firm itself has multiple classes of stock.We say that a firm’s ownership structure is a pyramid (on the 20 percent definition) if: (1) it has an ultimate owner, and (2) there is at least one publicly traded company between it and the ultimate owner in the chain of 20 percent voting rights. Thus if a publicly traded firm B has 43 percent of the votes in a sample firm A, and an individual C has 27 percent of the votes in firm B, we would say that C controls A, and that the ownership structure is a pyramid. But if B is 100 percent owned by C, we would still call C the ultimate owner, but would not call the ownershipstructure a pyramid. Pyramids require publicly traded intermediate companies. We also use a parallel definition of pyramids with 10 rather than 20 percent of voting rights.We say that there is cross-shareholding by sample firm A in its control chain if A owns any shares in its controlling shareholder or in the companies along that chain of control. So, if firm B has 20 percent of the votes in A, a publicly held firm C owns 20 percent of the votes in B, and A owns two percent of the votes in C, we would say that C is the ultimate owner of A, that A is owned through a pyramid, and that there is a cross-shareholding by A. On the other hand, if, instead of A owning two percent in C, it were the case that B owned two percent in C, we would not call this a cross-shareholding by A because B is not a firm in our sample. We do not look for cross-shareholdings by firm A in firms outside its control chain because of data limitations.We use some further measures of ownership, which are summarized in Table I, but introduce them later as we present our findings in Section II. First, we present some examples.C. Examples of Ownership StructuresTo describe the database and to illustrate our variables, we present several cases of ownership structures of individual companies, in roughly increasing order of complexity.Begin with the United States. The three most valuable firms in the US at the end of 1995, General Electric, AT & T, and Exxon, are all widely held. The fourth most valuable, Microsoft, has three large shareholders (Figure 1): the co-founders Bill Gates (with 23.7 percent of the votes as well as shares) and Paul Allen (with 9 percent), and Steven Ballmer (with 5 percent). We say that Microsoft has an ultimate owner on the 20 percent (as well as on the 10 percent) definition, namely Bill Gates, and is a family-owned firm. It is obviously not a pyramid, does not have cross-shareholdings, and it takes 20 percent of the capital to amass 20 percent of the votes.The fourth most valuable company in Canada is Barrick Gold, and it has a more complex ownership structure (Figure 2). Its founder, Chairman, and CEO is Peter Munk, who is also Chairman and CEO of a holding company called Horsham, that owns 16.3 percent of votes and capital in Barrick Gold. Mr. Munk controls the publicly-traded Horsham with 79.7 percent of its votes, but only 7.3 percent of capital. Even though Munk evidently controls Barrick, we say that Barrick Gold is widely held on the 20 percent definition of control, since Horsham only has 16.3 percent of the votes. On the 10 percent definition, Barrick Gold, has an ultimate owner, a family. Since Horsham is publicly traded, we call Barrick’s ownership structure a pyramid on the 10, but not the 20, percent definition. Finally, even though Horsham has multiple classes of stock, it takes 20 percent of Barrick’s capital to have 20 percent of the votes, and so the company has a one-share-one-vote structure.7The next example is Hutchison Whampoa, the third most valuable company in Hong Kong (Figure 3). It is 43.9 percent controlled by Cheung Kong Holdings, which happens to be the fifth largest publicly traded company in Hong Kong and is therefore also in our sample. In turn, the Li Ka Shing family owns 35 percent of Cheung Kong. Hutchison Whampoa and Cheung Kong are thus both family controlled companies, except the former is owned through a pyramid but the latter is not. Note that Li Ka Shing controls three of the 20 largest companies in Hong Kong (also the 11th largest Hong Kong Electric Holdings), a number that we keep track of. After the State-controlled NT & T, Toyota Motor is the most valuable company in Japan (Figure 4). Toyota has several non-trivial shareholders, but none of them is very large. Four of these shareholders (Sakura Bank, Mitsui Fire and Marine, Mitsui T & B, and Mitsui Life) are part ofthe Mitsui Group and together control 12.1 percent of both capital and votes in Toyota. This is a common situation in Japan, and we say that Toyota is widely held on the 20 percent definition, but “miscellaneous” on the 10 percent definition, because that is where we put business groups as well as voting trusts. There are no pyramids or deviations from one-share-one-vote here, but Toyota has cross-shareholdings in firms in the Mitsui Group.8Ownership in Japanese companies is straightforward relative to that in Korean ones, as the example of Korea’s second largest firm, Samsung Electronics (Figure 5), illustrates. Samsung’s founder, Lee Kun-Hee controls 8.3 percent of Samsung Electronics directly. But he also controls 15 percent of Samsung Life, which controls 8.7 percent of Samsung Electronics, as well as 14.1 percent of Cheil Jedang, which controls 3.2 percent of Samsung Electronics directly but also 11.5 percent of Samsung Life. Lee Kun-Hee has additional indirect stakes in Samsung Electronics as well. Because there are no 20 percent ownership chains, we call Samsung Electronics widely held on the 20 percent definition. But because between his direct holdings and holdings in Samsung Life Lee Kun-Hee controls over 10 percent of the votes in Samsung Electronics, it is a family-controlled firm on the 10 percent definition. It is also controlled through a pyramid on that definition because, for example, Samsung Life is publicly traded.Finally, to illustrate the really complicated cases, we consider ownership structure of five companies from Continental Europe. We begin with Germany, where the most valuable company is Allianz Insurance (Figure 6). Allianz is a one-share-one-vote company with several large shareholders, of whom the largest, with a 25 percent stake, is Munich Reinsurance, the third most valuable company in Germany. However, Allianz has cross-shareholdings in most of its large shareholders, including a 25 percent stake in Munich Reinsurance (Allianz also has a 22.5 percentstake in Dresdner Bank, which has a 10 percent stake in Munich Reinsurance). Allianz presents a difficult case: one could argue that it is widely held because it controls its controlling shareholder, that it is controlled by a widely held financial institution, or that it belongs in the “miscellaneous”category. We allocate it to the first category, while (happily) recognizing that there are only four such controversial cases in the sample, including Munich Reinsurance itself.The fourth largest company in Germany is Daimler Benz (Figure 7). It is 24.4 percent owned by Deutsche Bank, so its ultimate owner is a widely held financial institution (the largest shareholder in Deutsche Bank is Allianz, with five percent). Other shareholders of Daimler Benz form an enormous pyramid, but we would not call its ownership structure a pyramid because it does not involve publicly traded firms in the control chain and does not lead to the ultimate owner. While there are other over 10 percent shareholders, and chains of shareholders, in Daimler Benz, for the purposes of most of our analysis we only look at the largest shareholder, namely Deutsche Bank. Also, by looking only at the banks’ own equity ownership, we ignore the voting arrangements which enable Deutsche Bank and other German banks to vote the shares they hold in custody for their brokerage clients, thereby biasing our results in favor of Berle and Means.The fourth most valuable company in Sweden is ABB (Figure 8). Like five of the top ten most valuable companies in Sweden, ABB is controlled by the Wallenberg family, characteristically through a pyramid of companies that have shares with differential cash flow and voting rights. Incentive, the 17th most valuable company in Sweden, owns 24.3 percent of capital and has 32.8 percent of the votes in ABB. The Wallenberg Group owns 32.8 percent of the capital, but has 43.1 percent of the votes in Incentive. The Wallenberg Group is a voting。
英文:坏的习惯会影响你的大脑

The New Theory On Weight Loss: Your Bad Diet Has Damaged Your BrainYou’ve tried and tried to lose weight, but it just doesn’t happen. Or you lose a bit, then gain it back with a vengeance. Sound familiar?Recently, while reporting a story on 3 new supplements studied for weight loss, I had the opportunity to speak with nationally known obesity expert Louis Aronne, M.D., Director of the Comprehensive Weight-Control Program at New York-Presbyterian HospitalAccording to Aronne, scientists are finally finding answers to the mystery that has stumped them for so long: Why do some people seem to find it impossible to lose weight, despite numerous serious attempts to get slim using diets and exercise?And what they’ve discovered might surprise you: Years of eating – and overeating – the typical American diet actually changes the brain. More specifically, it damages the signaling pathways in the hypothalamus, the part of the brain that regulates metabolism.“The evidence is quite convincing – eating fattening foods causes inflammatory cells to go into the hypothalamus, ” explains Aronne. “This overloads the neurons and causes neurological damage.”A groundbreaking study in the British Journal of Nutrition published in February, 2013 is one example of the kind of high-quality, on-target research that’s proving the theory of hypothalamic damage and thus paving the way to new weight loss strategies, Aronne says.A team of scientists at the University of Liverpool analyzed a body of research that included studies of different weight loss diets. What they found was that a diet high in saturated fat and simple carbohydrates sets in motion a chain reaction of “metabolic dysfunction” involving the appetite regu lating hormones leptin and ghrelin. (Leptin’s job is to suppress appetite, ghrelin’s to increases it.) In addition, a fatty high-carb diet resulted in “alterations in structural plasticity” – i.e. brain changes.Over time, consuming too many calories from fat and simple sugars damages the nerves that conduct signals through the hypothalamus, affecting thefunction of leptin and ghrelin, and thus the body’s ability to regulate weight and metabolism, says Aronne. “Because of this damage, the signals don’t get through about how much fat is stored.”In other words, your brain has gone haywire and you can no longer trust the messages it’s sending you about appetite, hunger, and fullness. “It’s like your gas gauge points to empty all the time, whether or not the t ank is full, ” says Aronne. “So you keep stopping for gas, and then eventually you start filling up gas cans and storing them in the back of your car because you’re so convinced you could run out of gas at any moment.”So What Does Work for Weight Loss?Ch ange your diet, and change it fast. “It’s about biology, ” Aronne is fond of saying. While some damage to the hypothalamus may be permanent, it’s possible to reverse much of it. “If less fatty food comes in, it reduces the rate of damage, ” he explains, noting that it doesn’t matter so much which specific diet you follow, as long as it’s one that cuts calories, reduces fat, and reduces simple carbohydrates.Of course, there are lots of trendy diets, such as the Fast Diet currently making headlines. And ther e’s no reason not to try a new approach and see if it works better for you than the ones you’ve tried in the past. But work with your body, not against it, Aronne says, and the weight will come off much faster.But wait, there’s more. Retooling your diet t o be rich in health-promoting foods can stop and even reverse the damage done by an unhealthy one. In the above-mentioned study at the University of Liverpool, the researchers also looked at the impact of omega-3 fatty acids, known to be beneficial to brain health. And sure enough, fish oil appears to modulate some of the negative effects of the saturated fats and carbs.What that means, in effect, is that switching to a healthy diet can heal the hypothalamic damage that’s playing havoc with your hunger and satiety cues. Not surprisingly, Aronne has authored his own diet book (with coauthor Alisa Bowman), The Skinny: The Ultimate Guide to Weight Loss Success (2010). It features lean meat, plenty of seafood, lots of vegetables and fruit, and unprocessed grain s. There’s also more information on Aronne and his views on brain signaling and weight loss available on the Weill Cornell Medical College website.But here’s secret number two: Permanent weight loss takes time. Aronne is quick to point out that many of th ose who’ve dropped massive amounts of weight on The Biggest Loser have gained most of it back again within a year ortwo. Once again, science suggests the problem is that it takes time for thebrain’s metabolic messaging system to heal.Weight Loss Medications May HelpIf you’re in the group of people who’ve tried (really tried) controlling yourweight with diet and exercise, Aronne says it’s worth considering taking aprescription weight loss medication. Doing so can reset your brain to begin healing the hypothalamic damage. The two new diet drugs that just entered the market, Qsymia (formerly Qnexa) and Belviq, have the potential to do this, Aronne says.“Qsymia supports neuropeptide Y, amplifying the signals that come from the hypothalamus, ” Aronne says, adding that Belviq (lorcaserin) and the migraine drug Topamax also have a beneficial effect on brain signaling. In other words, these drugs at least partially fix the broken gas gauge.Along with being overweight comes insulin and leptin resistance, Aronne says.“When inflammatory cells go into the hypothalamus, they prevents leptin –which signals to the brain that the stomach is full from getting in, ” Aronne says. Among other things, Belviq stimulates the effect of leptin, Aronne says.“We don’t know exactly how it works, but it’s possible the mechanisms are hypothalamic. Belviq appears to amplify signals that go to the critical area of injury.”Now I know I’ve reported in the past on concerns about side effects fromQsymia and Belviq. Qsymia has been looked at for potential heart valvedamage because phentermine, one of the two drugs in the combination, was part of the notorious Fen Phen diet drug combo that caused serious heartvalve damage in the 1980s and early 90s.But from Aronne’s perspective, the drugs have been studied very thoroughly and these concerns are unfounded. And more importantly, the seriousness of the health problems – and the increased risk of death – associated withobesity outweigh the risks of the drugs.n. 机制;原理,途径;进程;机械装置;技巧∙appetite['æpitait]videon. 食欲;嗜好∙reset[,ri:'set, 'ri:set]videovi. 重置;清零vt. 重置;重新设定;重新组合n. 重新设定;重新组合;重排版∙overeat[,əuvə'ri:t]videovt. 使吃过量vi. 吃得过多n. 药物;药物治疗;药物处理∙pathway['pɑ:θwei,'pæθ-]videon. 路,道;途径,路径∙insulin['insjulin, 'insə-]videon. [生化][药] 胰岛素∙stimulate['stimjuleit]videovt. 刺激;鼓舞,激励vi. 起刺激作用;起促进作用∙saturate['sætʃəreit, 'sætʃərit]videovt. 浸透,使湿透;使饱和,使充满adj. 浸透的,饱和的;深颜色的∙analyze['ænəlaiz]videovt. 对…进行分析,分解(等于analyse)。
风险感知的要素 Sjoberg 2000_factors in risk perception
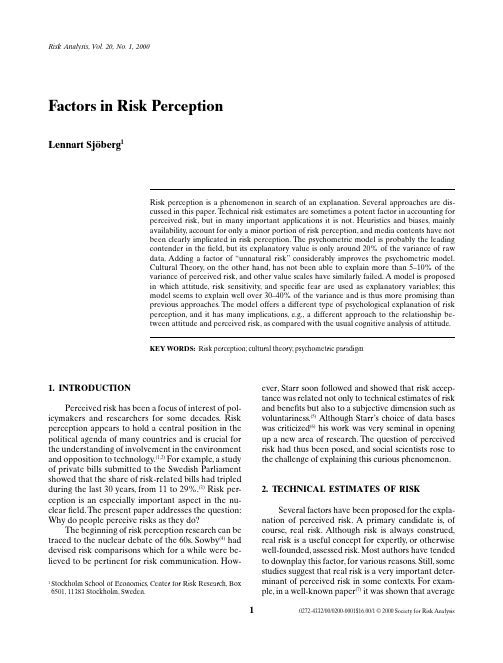
Risk Analysis, Vol. 20, No. 1, 200010272-4332/00/0200-0001$16.00/1 © 2000 Society for Risk AnalysisFactors in Risk PerceptionLennart Sjöberg1cussed in this paper. Technical risk estimates are sometimes a potent factor in accounting for perceived risk, but in many important applications it is not. Heuristics and biases, mainly availability, account for only a minor portion of risk perception, and media contents have not been clearly implicated in risk perception. The psychometric model is probably the leading contender in the field, but its explanatory value is only around 20% of the variance of raw data. Adding a factor of “unnatural risk” considerably improves the psychometric model.Cultural Theory, on the other hand, has not been able to explain more than 5–10% of the variance of perceived risk, and other value scales have similarly failed. A model is proposed in which attitude, risk sensitivity, and specific fear are used as explanatory variables; this model seems to explain well over 30–40% of the variance and is thus more promising than previous approaches. The model offers a different type of psychological explanation of riskperception, and it has many implications, e.g., a different approach to the relationship be- 1.INTRODUCTIONPerceived risk has been a focus of interest of pol-icymakers and researchers for some decades. Risk perception appears to hold a central position in the political agenda of many countries and is crucial for the understanding of involvement in the environment and opposition to technology. (1,2) For example, a study of private bills submitted to the Swedish Parliament showed that the share of risk-related bills had tripled during the last 30 years, from 11 to 29%. (3) Risk per-ception is an especially important aspect in the nu-clear field. The present paper addresses the question:Why do people perceive risks as they do?The beginning of risk perception research can be traced to the nuclear debate of the 60s. Sowby (4) had devised risk comparisons which for a while were be-lieved to be pertinent for risk communication. How-ever, Starr soon followed and showed that risk accep-tance was related not only to technical estimates of risk and benefits but also to a subjective dimension such as voluntariness. (5) Although Starr’s choice of data bases was criticized (6) his work was very seminal in opening up a new area of research. The question of perceived risk had thus been posed, and social scientists rose to the challenge of explaining this curious phenomenon.2.TECHNICAL EST IMAT ES OF RISKSeveral factors have been proposed for the expla-nation of perceived risk. A primary candidate is, of course, real risk. Although risk is always construed,real risk is a useful concept for expertly, or otherwise well-founded, assessed risk. Most authors have tended to downplay this factor, for various reasons. Still, some studies suggest that real risk is a very important deter-minant of perceived risk in some contexts. For exam-ple, in a well-known paper (7) it was shown that average 1Stockholm School of Economics, Center for Risk Research, Box 6501, 11383 Stockholm, Sweden.2Sjöbergestimated mortality rates for a number of common ill-nesses and accidents were strongly related to statistical data. There was also, to be sure, some systematic devi-ation from the trend—small risks were overestimated and large risks were underestimated—but the general trend was the one just stated: risks were perceived, by the average person, in a rather veridical manner. Others(8,9) have replicated these findings.The type of risks where realistic perception can be expected appear to be the risks with which people have some experience, direct or indirect.(10) In addi-tion, it must be noted that the risk ratings of the cited work concerned general risk, i.e., the risk to others, or to people in general. The risk target is a factor of great importance in risk perception, to be treated in a subsequent section.3.HEURISTICS, BIASES, AND RISKPERCEPTIONWhether people perceive a risk the same as tech-nical risk estimates can be seen as a question of the veridicality of subjective probability. It was conceived in that way in the 1970s, and the work on heuristics and probability judgment biases(11) was often cited as pertinent.(12) Of the three heuristics—representative-ness, availability, and anchoring—it was most often ar-gued that availability(13) was important for understand-ing risk perception. There is an obvious relationship here to mass media and the idea that frequent media exposure gives rise to a high level of perceived risk.(14) This work is no longer regarded as of primary importance for risk perception, however. Fischhoff and others showed early on that the public’s risk per-ception was much more multidimensional—subjec-tive probability is only one of many factors.(15) The mass media role in risk perception is still very much under debate.(16,17)The heuristics tradition relied heavily on a pre-sumption that belief distortion is a matter of cogni-tion. It has been found, however, that beliefs and values correlate strongly.(18,19) This phenomenon is hard to account for in a purely cognitive framework, and it suggests that beliefs are value driven. The bias of be-liefs is largely a question of a tendency towards black-and-white construals and wishful thinking. Mont-gomery pointed to the pervasive tendency of decision makers to construe options in such a manner as to ob-tain a dominant or quasi-dominant alternative; the choice of such an alternative is of course very easily justified to oneself and others.(20)It can be added that the work on heuristics reliedgreatly on probability calculus problems that hadbeen set up to have counterintuitive answers. How-ever interesting such a method may be for revealinghow intuition differs from probability calculus, it hasprobably limited applicability to how people perceiveprobabilities and risks in applied settings.4.RISK TARGETPeople do not make the same estimate when theyrate the risk to themselves, to their family, or to peoplein general. For example, in a study with a large repre-sentative sample of the Swedish population, thesethree risk targets were studied for a set of fifteen haz-ards.(21) The respondents were instructed to rate therisks on a scale from 0 (no risk at all) to 6 (an extremelylarge risk), with five categories lying in between thesetwo extremes and defined as 1, 2, 3, 4, and 5. The meanratings (Nϭ 1,224, 72% response rate) are given in Fig. 1. Drastic differences are seen, particularly betweengeneral risk and personal or family risk.Note that the difference is not only a question oflevel. The rank order between risks is not the sameacross targets.It is clear that not everybody can be right in say-ing that he or she is subjected to a smaller risk thanpeople in general (even if some respondents of courseare right in saying so). The fact that people most oftenclaim to be less subjected to risk than others can betermed risk denial. Risk denial is a very important fea-ture of risk perception. The phenomenon is related towhat has been called unrealistic optimism.(22)I t is also important, of course, to ask what cancause this variation in perceived risk across risk tar-gets. Respondents have also been asked to rate the Fig. 1.Average risk ratings: personal, family, and general risk.Factors in Risk Perception 3degree of control they felt they had over each of the hazards—to what extent they could protect them-selves against it. (23) Mean differences between general and personal risk (risk denial) are plotted in Fig. 2against mean control ratings.Hence, at this level of analysis, control is appar-ently an important variable in accounting for risk denial.For individual data, the correlation is much weaker.In many risk perception studies the target is not explicitly spelled out. The respondents are simply asked to rate “the risk,” with no further specification.That instruction was compared with several others in a study of 309 subjects who were divided at random into groups getting different instructions. (24) They rated 27 hazards. The results are seen in Fig. 3.I t was found that the no-target condition gave about the same result as rating the general risk. Fur-thermore, rating the risk to “any one person” also gave the same result, refuting the sometimes-heard explanation of the general–personal difference that it reflects the fact that general risk ratings refer to many more persons than personal risk ratings.Personal risk and general risk have different con-sequences. Consider, by way of illustration, a study of attitudes to alcohol sales liberalization in Sweden. 22At present, alcoholic beverages can be sold only by state monopoly stores, which are rather few and keep quite restricted business hours. Taxation keeps prices very high. The system has been in place since the 1950s, when it replaced a still more restrictive system.An unpublished master’s thesis from the Center for Risk Research reported a study of perceived risk and the attitude to legislation enabling ordinary grocery stores to sell wine. (25) The correlations between per-ceived personal risk of alcohol-caused injury and the corresponding general risk on the one hand and atti-tude toward liberalization of sales on the other were Ϫ 0.59 for general risk and Ϫ 0.15 for personal risk. (In both cases, a larger risk was associated with a more negative attitude.) Hence, policy attitude was proba-bly driven by general risk rather than personal risk.The risk target is of paramount importance in risk studies. This fairly simple fact seems not to be generally known and many studies still work with nonspecified risk targets. This means that they proba-bly miss out on the need to understand perceived per-sonal risk, and it introduces some uncertainty as to what target they actually do study. Some authors argue explicitly against the introduction of a target and seem to regard the choice by the subject as to target as an interesting factor. (26) Although it is true that studying the choice of target in a situation with ambiguous or nonspecific instruction as to target could be interest-ing, this is simply not possible in a design where people just make nonspecific risk ratings. Additional infor-mation about the choice of target, not collected in Marris et al. , (26) is needed.5.THE PSYCHOMETRIC MODELThis model was launched in a 1978 paper by Fisch-hoff et al. (27)The empirical work reported in that paper was later followed up with several other studies that were more extensive, both in terms of scales and thenumber of respondents.Fig. 2.Risk denial (general minus personal risk) plotted against perceived control over risks. Each point corresponds to one haz-ard; mean ratings are plotted.Fig. 3.Mean ratings of 27 hazards plotted for different targets;independent groups rated each target.4SjöbergThe model is based on a number of explana-tory scales (9 to begin with, later 18) such as New–Old, Voluntary–I nvoluntary, etc. The subjects areasked to rate a number of hazards on each of thescales. Mean ratings are computed for each hazardon each scale, and the resultant Scales ϫ Hazards matrix is factor analyzed. Usually, three factorssuffice to explain a large share of the variance, per-haps around 80%. The factors that have beenfound in many studies are New–Old, Dread, andNumber of Exposed.The scales, or the factors, usually account forsome 60–70% of the variance of perceived risk or riskacceptance, when entered as average ratings for anumber of hazards in multiple regression analyses.This model has been used as a basis for extensivework on risk communication.(28) The famous “map” ofhazards in the two major dimensions of Dread andNew–Old(29) shows, e.g., how nuclear power is locatedin a position where it is highly loaded on the Dreaddimension and also seen as New or Unknown. Thisfinding apparently seemed to be a self-evident expla-nation of the strong public opposition to nuclearpower and is still often quoted as such.This is curious indeed because no data actuallylinked opposition to nuclear power to the psychomet-ric dimensions. Apparently it was sufficient that it justseemed to be the explanation. As a counterexample,the perceived risk of nuclear waste in Swedish data isaccounted for neither by dread nor newness.(30) I n-deed, nuclear power is not perceived as “new.” Per-haps the 15 years since the first U.S. studies accountfor that. But opposition to nuclear power is everpresent today, just as it was at the end of the 1970s.One wonders just how important the factor of new-ness is, or was.Furthermore, the model is not at all so powerfulas its proponents have claimed. Several reasons forthis statements can be given:1.Factor analysis of a matrix with only 9 or even18 scales is bound to give few factors. The fact thatthese few factors account for some 80% of the vari-ance of the scales is not surprising and does not at allimply that they can also account for the perceivedlevel of risk to same extent. The latter factor couldbe unrelated to the scales, and the scales could still bewell described by 3 factors.2.The scales were based on a compilation offactors suggested in the earlier risk literature of the70s, which in turn was stimulated by Starr’s work.(5)Although 18 scales may seem like a lot, they missedat least one important aspect, namely, interference with nature (tampering with nature, immoral andunnatural risk), which will be further describedsubsequently.3.The finding that a very large share of the vari-ance of perceived risk could be explained by thesefactors is due to the fact that the authors analyzedmean ratings, not raw data.(31) Mean data are less sub-ject to error than raw data and “smooth,” hence modelsof various kinds are much more likely to fit. One could,of course, claim that mean risk ratings are the focus ofinterest, not raw data, which reflect more directly therisks actually perceived by the subjects. However, forpractical and theoretical purposes it would seem to beobvious that the individual ratings should be of primaryinterest. The analysis of behavior should not be of aver-age behavior but of the ratings that people actuallygive. And for a practitioner, the inflated levels of ex-planatory power given by the results reported forthe analyses of means simply are very misleading.The raw data can only be explained to about 20–30% by the traditional psychometric model,(32,33) andthis means that many other factors are just as impor-tant as, or more important than, the psychometricfactors devised by Fischhoff et al.(27)For one such dimension, consider results show-ing that a fourth factor, denoted as Unnatural andImmoral Risk, has been discovered in recent work.(34)This work is a continuation of earlier studies in whichthe moral factor was emphasized(35,36) and shown to bestrongly related to perceived risk. Morality is indeedmissing from much of the work on risk perception,(37)and the reason might be that hazards are conceived assuch, rather than as the outcome of human actions.In a study of perceived nuclear waste, risk datawere obtained from a representative sample of theSwedish population, response rate 53.2% (Nϭ541).(34) A factor analysis of 21 psychometric scalesapplied to nuclear waste risk gave a four-factor solu-tion with 66% explained variance. Oblimin rotationresulted in four factors, three of which were tradi-tional, namely New Risk, Dreaded Risk, and Numberof Exposed. A fourth factor also emerged, loaded insuch items as Unnatural Risk, I mmoral Risk, andHuman Arrogance. A multiple regression of per-ceived nuclear waste risk (a pooled index, no targetspecified) against these four factors gave the resultsshown in Table I.Only the factor of Unnatural and I mmoralRisk had a significant  value. Note that the psycho-metric model performed, in its three-factor version, at the usual 20% level and that its performance was strongly improved by the introduction of the newFactors in Risk Perception5fourth factor, which then carried the whole of its ex-planatory power.Could these findings be replicated in a different setting and with different hazards? Another set of data analyzed here are from a current European Union project called RI SKPERCOM in which 798 respondents (response rate 61.0%), representative of the Swedish population, answered an extensive risk perception questionnaire. Results are given for per-sonal and general risk of domestic nuclear power and factor scores of four factors (explaining 61% for the variance) of psychometric scales measuring the per-ception of a Chernobyl-type accident. Twenty-two scales were once more compressed to four factors: New Risk, Dreaded Risk, Many Exposed, and Un-natural and Immoral Risk (including risk as a warn-ing of worse to come). The results are given in Table I I. The reason for the rather low values of R2adjis probably that a rather specific psychometric target was studied, namely “A Chernobyl-type accident.”The conclusion drawn about the psychometric model is that, in its traditional three-factor form, it explains only a rather modest share of the variance of perceived risk. The widespread credibility that the model apparently enjoys seems to be based largely on the fact that analysis was done on averages rather than raw data, i.e., correlations reported were based on means and therefore very high, thereby giving an impression that virtually all of risk perception was ac-counted for.The fourth factor throws quite a different light on perceived nuclear risk than the three traditional factors. It is no longer a question of new or dreaded risk, but a more elaborated perspective having to do with notions about tampering with nature and moral questions. Other current concerns such as genetic en-gineering and the BSE (“mad cow disease”) come easily to mind in this connection and the fourth factor therefore seems worthy of serious consideration in further risk perception work.It is sometimes argued that scales of the psycho-metric type are trivially related to risk perception and that semantic overlap is the true reason for success in accounting for risk perception. I tems that have the same or very similar denotation can of course be ex-pected to correlate quite strongly. I n the present context, however, this argument is exaggerated. Level of perceived risk is not strictly semantically overlapping with, say, newness or immorality of a hazard. The fact that properties of a construct or ob-ject are statistically related to a global assessment such as attitude or level of risk may be seen as likely but is not an artifact. Yet, many researchers voice their interest in more “distal” explanations of risk, i.e., in constructs that are contentwise less obviously related. A second major attempt at explaining risk perception is provided by Cultural Theory, and that theory tries to deliver such nonproximal explana-tory concepts of risk perception. A discussion of that theory and some related approaches of a distal na-ture follows.6.CULT URAL T HEORY OF RISKPERCEPT IONThe Cultural Theory of risk perception was spelled out in a book by Douglas and Wildavsky(38) and made operational for quantitative study by Dake.(39,40) The theory specifies that there are four types of people: egalitarian, individualistic, hierarchic, and fatalistic. These types of people will “choose” to be concerned with different types of hazards:•Egalitarians: technology and the environment•Individualists: war and other threats to themarkets•Hierarchists: law and order•Fatalists: with none of the aboveTable I.Multiple Regression Analysis of Perceived Nuclear Waste Risk as a Function of Psychometric Explanatory FactorsExplanatory variable Value (four factor model)Many exposed to risk0.090New risk0.044 Unnatural and immoral risk0.452 Dreaded riskϪ0.006R2adjthree traditional factors0.200R2adjfour factors0.280Table II.Regression Analyses of Personal and General Risk of Domestic Nuclear Power as a Function of Four PsychometricFactors, and R2adjfor the Three Traditional Factors ValuePersonal risk General risk Many exposed to risk0.0160.054 New risk0.000Ϫ0.013 Unnatural and immoral risk0.4360.419 Dreaded risk0.0140.000R2adjthree traditional factors0.0860.094R2adjfour factors0.1990.1986SjöbergThese four types of people were originally sug-gested by a group-grid analysis. The potent social context—in an abstract and formal sense—of a per-son is assumed to be governing his or her beliefs. A full empirical and quantitative investigation of the theory demands a study also of these social phenomena—no such investigation has so far been published.The theory is a rich conceptual structure and has several variations; see Boholm’s critical analysis of the theory from the standpoint of an anthropolo-gist.(41) Boholm’s conclusions as to the theoretical sta-tus of the theory are quite negative. The present paper will concentrate on what can be said about the empir-ical support claimed for the theory in quantitative studies of the relationship between the four types of people noted previously and risk perception. A fuller discussion is available elsewhere.(42)First, however, a few words about theory. The success of Cultural Theory is largely an example of the persuasive power of speculation. The theory has now existed for some 15 years and it has been, in the words of a supporter, “immensely influen-tial.”(43) Yet, the only empirical evidence offered before Dake’s work was available in anecdotal ac-counts, a few anthropological observations, and some observations using more or less informal qualitative methods.The initial empirical support for the theory was described by Wildavsky and Dake.(44) They used scales measuring the four concepts illustrated by the types noted previously and variously termed cultural biases or world views. The scale scores were corre-lated with a number of “social concerns,”3 which can perhaps be seen as a kind of risk ratings, and reports were given for a selection of the concerns that were significantly correlated with the scales. The pattern of relationships provided by these correlations was roughly in accordance with predictions from the the-ory, but only bivariate correlations were given, so judging how much of the variance of perceived con-cerns would have been explained by multiple regres-sion of the scale scores is impossible.However, the same scales and concerns were investigated in a later study of U.S. subjects by Sjöberg,(45) in which explicit risk ratings were also ob-tained. I t was found that concerns were explained, on average, to about 10% of the variance, risk ratings to only half as much. European studies, using a re-3Although this research has been suggested as strong evidence for a Cultural Theory apporach to understanding opposition to tech-nology, the social concerns actrully studied were mostly related to economic and social problems, not problems of technology.vised form of the scales, have shown that the scales account for about 5% of the variance, on aver-age.(46:26) Correlations with single items have turned out to give a very low amount of explained variance when added to other explanatory constructs in models of risk perception.(37,47)Yet the pattern of relationships with social con-cerns observed by Dake is apparently robust.(26,45) The question is what to conclude from that finding of a weak but systematic pattern of relationships. Several authors have suggested that much stronger relation-ships are likely if the scales are improved. However, correcting for measurement error yields only trivial increase of the correlations. (Reliabilities of the U.S. scales are not reported in Dake’s work but can be found in Sjöberg.(45) They were moderate.)The Dake scales are examples of scales of politi-cal values and attitudes, and they function much in the same manner as traditional political attitude scales when related to risk perception, i.e., quite weakly.Asking if value scales in general are similarly weak in accounting for risk perception is natural. Sjöberg first approached this question by investigat-ing lifestyle scales and relating them to risk percep-tion.(48) Lifestyles are extremely popular in marketing and there are many claims about their strong explan-atory value in various commercial contexts. How-ever, they were found to carry little or no explanatory power for risk perception.A second approach, investigating some cur-rently popular general value scales in the environ-mental field, is summarized here. To achieve this pur-pose, a number of value scales were included in a questionnaire aiming at the measurement of attitudes to Swedish membership in the European Union. The study was conducted during the month before the Swedish referendum (November 13, 1994). A mail survey design was used. Sixty percent of a random sample of the adult Swedish population responded, thus yielding a data set with 1,224 respondents.Twenty two risks, all personal, were included in the study,4 and representing a broad selection of threats to health, environment, and economy. They were judged on a category scale with 7 steps, 0–6, where 0 denoted a nonexistent risk and 6 an ex-tremely large risk.The value scales were Swedish translations of the following:4Personal and general risk perception correlate highly and have si-milar relationships to other variables, so it is unlikely that general risk perception data would have been more strongly related to value dimensions.Factors in Risk Perception71.Kahle’s(49,50) List of Values (8 items)2.Dunlap’s New Environmental Paradigm(51)(15 items)3.Inglehart’s(52) postmaterialism items (12 items)4.Three subsets of items from Schwartz’s(53)value scale. These were 14 items selected onthe basis of a previous study to cover the fac-tors of the scale in a representative manner, 9associated with environmental responsibilityand 9 with consequences of environmentalrisks, in all 32 items.5.Nine items from a study of American valuesby Kempton(54)6.Eleven items measuring current left–rightconflict or traditional political ideology Multiple correlations (squared, adjusted) were computed for each set of value items and each per-ceived personal risk. In addition, R2adjor eta2 were com-puted for the risks and the following background data:•Sex•Education•Income•Size of residential community•Political party preferenceIn Table III, the mean amounts of variance explained, computed across the 22 risks, as well as minimum andmaximum values of R2adjor eta2 are reported.These results show clearly that general value di-mensions are not very promising for understanding risk perception. Even in the best case, only a modest average of 6–7% of the variance was explained.It is also important to note that background factors were very weakly related to risk perception. Survey studies often display a bias of some kind, e.g., in terms of educational level of the respondents, but results such as the present ones (and they are rather typical) suggest that such bias is not serious in studies of risk perception.Several other objections to the critique of Cul-tural Theory need to be discussed. It should first be noted that the results reported here are not all that different from the ones reported by Dake. The differ-ences are mostly a question of the conclusions drawn, not the results per se.Defenders of Cultural Theory have suggested a number of objections to the present conclusions, namely that the results are due to restriction of range or differentiation (too few steps) of the variables used, lack of reliability, the choice of risk perception rather than demand for risk mitigation, linguistic dif-ficulties or cultural differences; or it is argued that other ways of making the theory operational could result in research supporting the theory. None of these ad hoc objections holds up under closer scrutiny, however. The purely statistical objections (restriction of range, lack of reliability, etc.) have been checked and found to be of minor importance. The differences in political culture between Europe and the United States may be the reason why the Dake scales are somewhat more strongly related to risk perception in the United States than in Europe.5 The notion that the theory may be supported in other, as yet untried, ways is log-ically correct but an uninteresting type of objection 5The left in Europe is more strongly associated with the estab-lished power and takes responsibility for technologies such as nu-clear power. Hence, egalitarian values cannot be expected to be as strongly correlated with antitechnology values as in the United States.Table III.Mean and Range of Explained Variance of 22 Personal Risks,on the Basis of Background Variables and Value DimensionsExplanatory variable Minimum Maximum Average Sex.000.042.011 Education.000.030.007 Size of residential community.000.010.003 Income.000.039.008 Political party preference.004.163.051 Kahle’s list of values.000.070.024 Dunlap’s New Environmental Paradigm.010.104.046 Inglehart’s post-materialism.001.095.038 Schwartz’s 14 general items.017.129.067 Schwartz’s responsibility items.001.093.026 Schwartz’s awareness of consequences items.000.260.058 Kempton items.000.088.035 Left-right political items.005.113.050。
光材料学英文文献(1)
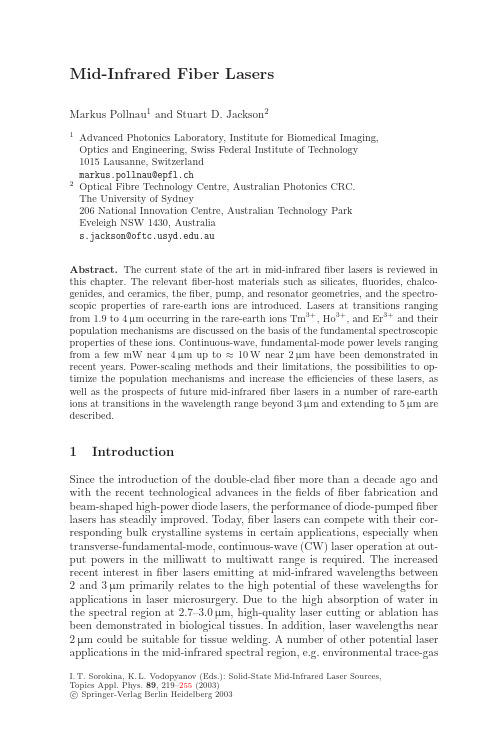
Mid-Infrared Fiber LasersMarkus Pollnau1and Stuart D.Jackson21Advanced Photonics Laboratory,Institute for Biomedical Imaging,Optics and Engineering,Swiss Federal Institute of Technology1015Lausanne,Switzerlandmarkus.pollnau@epfl.ch2Optical Fibre Technology Centre,Australian Photonics CRC.The University of Sydney206National Innovation Centre,Australian Technology ParkEveleigh NSW1430,Australias.jackson@.auAbstract.The current state of the art in mid-infraredfiber lasers is reviewed in this chapter.The relevantfiber-host materials such as silicates,fluorides,chalco-genides,and ceramics,thefiber,pump,and resonator geometries,and the spectro-scopic properties of rare-earth ions are sers at transitions ranging from1.9to4µm occurring in the rare-earth ions Tm3+,Ho3+,and Er3+and their population mechanisms are discussed on the basis of the fundamental spectroscopic properties of these ions.Continuous-wave,fundamental-mode power levels ranging from a few mW near4µm up to≈10W near2µm have been demonstrated in recent years.Power-scaling methods and their limitations,the possibilities to op-timize the population mechanisms and increase the efficiencies of these lasers,as well as the prospects of future mid-infraredfiber lasers in a number of rare-earth ions at transitions in the wavelength range beyond3µm and extending to5µm are described.1IntroductionSince the introduction of the double-cladfiber more than a decade ago and with the recent technological advances in thefields offiber fabrication and beam-shaped high-power diode lasers,the performance of diode-pumpedfiber lasers has steadily improved.Today,fiber lasers can compete with their cor-responding bulk crystalline systems in certain applications,especially when transverse-fundamental-mode,continuous-wave(CW)laser operation at out-put powers in the milliwatt to multiwatt range is required.The increased recent interest infiber lasers emitting at mid-infrared wavelengths between 2and3µm primarily relates to the high potential of these wavelengths for applications in laser microsurgery.Due to the high absorption of water in the spectral region at2.7–3.0µm,high-quality laser cutting or ablation has been demonstrated in biological tissues.In addition,laser wavelengths near 2µm could be suitable for tissue welding.A number of other potential laser applications in the mid-infrared spectral region,e.g.environmental trace-gas I.T.Sorokina,K.L.Vodopyanov(Eds.):Solid-State Mid-Infrared Laser Sources,Topics Appl.Phys.89,219–255(2003)c Springer-Verlag Berlin Heidelberg2003220Markus Pollnau and Stuart D.Jacksondetection,are currently becoming increasingly important.In all these appli-cations fiber lasers may find their niches.The high development costs of fabricating fibers with sufficiently low losses in the mid-infrared spectral region has impeded the necessary research efforts in the field of mid-infrared fiber lasers.The currently available fiber materials that are suitable as host materials for specific rare-earth-doped fiber lasers in the spectral region 2–5µm will be introduced in Sect.2.More than any other idea,the invention of the double-clad fiber geometry has accelerated the output-power scaling and hence the success of fiber lasers.The various aspects of the fiber,pump,and resonator geometries will be de-scribed in Sect.3.A significant number of spectroscopic investigations has led to a better understanding of the population mechanisms of rare-earth-doped laser systems.The fundamental spectroscopic properties of rare-earth ions in solid-state host materials will be reviewed in Sect.4.Equipped with this general information,the performance of the most important mid-infrared fiber laser transitions in the wavelength range 2–3µm can be understood in detail.Sect.5will be devoted to the Tm 3+fiber lasers at 1.9and 2.3µm,whereas the Ho 3+fiber lasers at 2.1and 2.9µm will be discussed in Sect.6.An impressive example of the variety of population mechanisms and operational regimes in a single system is the Er 3+2.7µm fiber laser transition that will be investigated in Sect.7.At wavelengths beyond 3µm,it becomes increasingly difficult to find suitable host materials for actively doped laser systems.This statement holds true for glass fibers in the same way as for crystalline materials.The prospects of future mid-infrared fiber lasers in this wavelength range will be discussed in Sect.8.Besides general introductions to the different topics of lasers [1,2]that include many aspects relevant also to mid-infrared fiber lasers,a comprehen-sive introduction to the field of rare-earth-doped fiber lasers can be found in [3].2Fiber MaterialsThe choice of the fiber material involves a number of considerations:the maximum phonon energy,the environmental durability,the draw ability,the rare-earth solubility,and the purity of the starting materials.The maximum phonon energy of the glass sets the overall infrared transparency range of the fiber and the multiphonon relaxation rates which influence the quantum efficiency.The multiphonon relaxation rates for the common fiber glasses as a function of the energy gap between energy levels are shown in Fig.1.The optical transparency range relates to both the size of the band gap and also the infrared absorption cut-off,hence to the vibrational frequency νof the anion–cation bonds of the glass.For an ordered structure,ν=(1/2π) k/M ,(1)Mid-Infrared Fiber Lasers221101010M u l t i p h o n o n R e l a x a t i o n R a t e (s -1)Energy Gap (cm )Fig.1.Calculated and measured multiphonon relaxation rates as a function of the energy gap between energy levels for glasses with different maximum phonon energies.(Data taken from [4,5])where M =m 1m 2/(m 1+m 2)is the reduced mass for two bodies m 1,m 2vibrating with an elastic restoring force k .While for disordered structures like glass,this is not an accurate expression,nevertheless,it does highlight the important contributions to the glass transparency.The relative cation–anion bond strength is intimated by the field strength Z /r 2,where Z is the valence state of the cation or anion and r is the ionic radius.Generally,glasses composed of large anions and cations with low field strengths display high transparency in the mid-infrared spectral region.The important physical properties of the popular glasses used for optical fibers are shown in Table 1.Table 1.Properties of popular fiber materialsFibermaterialMax.phonon energy (cm −1)Infrared transparency (µm)Propagation losses (λat minimum)(dB/km)Thermal conductivity (W/K m)Silica1100[4]<2.50.2(1.55µm) 1.38[6]ZBLAN550[7]<6.00.05(2.55µm)0.7–0.8[8]GLS 425[5]<8.00.5(3.50µm)0.43–0.5[9]2.1SilicatesThis glass is perhaps the most important material used for optical fiber pro-duction [3,10],however,the maximum phonon energy is high (≈1100cm −1)and has so far limited the emission wavelength of mid-infrared fiber lasers us-ing this material to ≈2.2µm [11].Silica is robust and involves the very effec-222Markus Pollnau and Stuart D.Jacksontive modified chemical vapor deposition(MCVD)technique forfiber fabrica-tion.Reducing the OH−content in the glass,which has two main absorption peaks in the range1.3–2.0µm[12],improves the near-to-mid-infrared utility. Rare-earth ions such as Nd3+and Er3+which have highfield strengths have low solubility in silicate glass which can lead to clustering and micro-scale phase separation.2.2FluoridesThe use offluoride glasses,especially the heavy-metalfluorides[13,14],as host materials for mid-infraredfiber lasers has found wide acceptance.The most common form of heavy-metalfluoride glass is thefluorozirconate(ZrF4) composition and the most widespreadfluoridefiber material is ZBLAN[15], a mixture of53mol.%ZrF4,20mol.%BaF2,4mol.%LaF3,3mol.%AlF3, and20mol.%NaF.Since it can be readily drawn into single-mode optical fiber[16]it is particularly important to mid-infraredfiber lasers[17].The large atomic weight of the zirconium atom combined with relatively weak bonding provides a maximum phonon energy for ZBLAN of≈550cm−1and allows for high infrared transparency up to≈6µm.Multiphonon relaxation, however,becomes significant for transitions at wavelengths longer than≈3µpared to silica,ZBLAN has a lower damage threshold and a lower level of inhomogeneous spectral-line broadening(Sect.4.1)because the rare-earth ion is placed in sites of a less perturbed network.The crystal-field strength is also comparatively weaker[18].An overview of the spectroscopic properties of rare-earth ions doped into ZBLAN has been given in[7].2.3ChalcogenidesChalcogenides are composed of the chalcogen elements S,Se and Te[19,20,21]. They are environmentally durable,have a low toxicity and have reasonably large glass forming regions.When the rare-earth ions are doped into these glasses[22],the radiative transition probabilities and,therefore,the absorp-tion and emission cross-sections are high as a result of the high refractive in-dex(≈2.6)of the glass and the high degree of covalency of the rare-earth ion with the surrounding medium.Maximum phonon energies of300–450cm−1 produce low rates of multiphonon relaxation,see Fig.1,and therefore high quantum efficiencies.The low thermal conductivity,see Table1,is however an important factor to be considered in the design of chalcogenide-based lasers. Of the large number of rare-earth chalcogenides studied for luminescent emis-sion,the most important glasses are the sulfide glasses GaLaS(GLS)[23]and GeGaS[24]because of the reasonably high rare-earth solubility.2.4CeramicsStudies into the use of ceramics as host materials for the rare earths have recently made a lot of progress[25].These ceramics are composed of nano-Mid-Infrared Fiber Lasers223 crystallites of materials such as Y3Al5O12(YAG)and can be produced ina simple and cost-efficient process at relatively low temperatures.This allows the fabrication of materials with very high melting points[26]that are difficultto grow by other techniques such as the Czochralski method[27].This class ofmaterials is also available infiber geometry[28].Ceramicfibers combine the characteristics of crystalline materials such as high absorption and emissioncross-sections,large thermal conductivity,and even the possibility of doping with transition-metal ions[28]with the convenience of guiding the pump andsignal light in afiber.Currently,the losses of thesefibers are comparativelyhigh,but further improvement can be expected.3Fiber,Pump,and Resonator GeometriesThe light oscillating in afiber-laser resonator can be either free running or deliberately modulated depending on whether CW or pulsed output,re-spectively,is desired.Consequently,a large number of techniques for pulsedoperation including Q-switching and mode locking offiber lasers have been explored.These techniques have been investigated intensively for the commonlaser transitions at1µm in Nd3+and Yb3+and at1.5µm in Er3+,and are usually described in combination with these lasers.The smallfiber size limitsthe peak power through the damage-threshold intensity(propagating powerper core area)and,hence,crystalline lasers in bulk geometries or optical parametric processes are often preferred when high-energy short pulses areneeded.This argument accounts especially for mid-infrared ZBLAN-basedfiber lasers,because thesefibers possess a lower damage threshold compared to silicafibers.The description of mid-infraredfiber lasers is,therefore,con-fined to CW operation and specific techniques for pulsed operation offiber lasers are not discussed in this chapter.In an analogous way to the optical excitation of bulk gain media,dopedopticalfibers can be either end pumped(core pumped)or side pumped (cladding pumped).The former method is less scalable since it relies onthe use of expensive high-beam-quality pump sources because core areas areusually<100µm2.On the other hand,the larger cladding area(>104µm2) allows for high-power diode-array pumping[29,30,31,32,33].The obvious sim-plicity of the core-pumping method negates further explanation and we will concentrate on the cladding-pumping technique:one of the most important developments infiber-laser technology.3.1Fiber Designs for Cladding PumpingIn the design offibers for cladding pumping,the core of thefiber is gener-ally made to guide a single-transverse LP01mode.The shape of the mul-timode pump cladding,see Fig.2,however,remains somewhatflexible and can be shaped with a number of considerations in mind.The pump cladding,224Markus Pollnau and Stuart D.Jackson (a)(b)(d)CorePump cladding Outer cladding Jacket Fig.2.Principal double-clad fiber geometries which include (a )circular shaped pump cladding with axially positioned core,(b )circular shaped pump cladding with off-axially positioned core,(c )rectangular shaped pump cladding and (d )D-shaped pump claddingwhich in turn is surrounded by a low-refractive-index transparent polymer or glass,provides a high numerical aperture (NA)of 0.3–0.55for the pump cladding.There are three main double-clad-fiber layouts:circular,circular with offset core,and rectangular as shown schematically in Fig.2.Maxi-mum pump-light absorption sees the core near the outer edge of the circular pump cladding [34]because a portion of the launched light is skew to the fiber axis and produces an inner caustic and never crosses the central re-gion of the pump cladding.Scrambling these skew rays by bending [35]or by using a graded and slightly elliptical pump cladding [36]increases the pump-absorption efficiency as does spatially varying refractive-index fluctuations in inhomogeneous pump claddings [37].Inner caustics can be avoided by rectilinearly shaping the pump cladding [38]which has the ancillary advantage of matching the shape of diode-array output.The overall absorption coefficient of the fiber is reduced by the ratio of the core area to the area of the pump cladding [34].The propagation losses for the rectangular-shaped pump cladding are higher and the effective numerical aperture lower as compared to the circular shape [39];however,in certain cases higher dopant concentrations can provide shorter fiber lengths that also lead to reduced nonlinear effects.A D-shaped or trun-cated circular pump cladding [40],see Fig.2d,is also effective while be-ing easier to make than rectangular preforms.The circular-multimode pump cladding may also have the gain medium distributed in a ring around the edge of the pump cladding either discretely or continuously in multi-core [41]and M-profile [42]arrangements,respectively.The effective absorption coef-ficient is now further increased while maintaining high-beam-quality output.A large-mode-area core [43]can also increase the effective absorption coeffi-cient of the fiber.Recently,double-clad pump schemes have been demonstrated also with holey fibers [44].These structures offer the additional advantage of single-mode guiding over a broad spectral range [45].Mid-Infrared Fiber Lasers 2253.2Fiber-Laser ResonatorsTypical free-running fiber-laser resonators are shown schematically in Fig.3.In the simplest resonator,see Fig.3a,the pump light passes through a dichroic mirror that is highly reflective for the oscillating laser light.Fresnel reflection at the cleaved output end facet of the fiber can provide sufficient feedback for laser oscillation;however,with an output-coupler mirror –and pump retro-reflector –placed at the output end of the fiber the optical efficiency can be maximized.In an alternative arrangement,the pump light can be launched into the output end of the fiber,see Fig.3b.A dichroic mirror oriented at 45◦to the fiber axis extracts the laser output and a broadband highly reflecting mirror is placed at the rear fiber end.To scale the output power,each end of the fiber can be pumped,see Fig.3c.Periodic V-grooves [46]or prism coupling [47]along the fiber to distribute the pump access allow one to further scale the output power and are useful for pumping fiber ring resonators.Spectrally combining the output from a number of separate fiber lasers is also a promising power-scaling technique [48,49,50].The highest reported fiber-laser output powers of 110W in a singly Yb 3+-doped fiber [51]and 150W in a Nd 3+,Yb 3+-codoped fiber [52]have been obtained using arrangements as shown schematically in Fig.3c.Bragg gratings can substitute the fiber-butted mirror if spectrally well-defined output is required.PumpPump Pump Pump Output Output OutputMM M MMFiberFiberFiber(a)(b)(c)Fig.3.Schematic diagram of resonators used for free-running fiber lasers with (a )a single-end co-propagating pump,(b )a single-end counter-propagating pump and (c )dual end pumps.M represents the mirror226Markus Pollnau and Stuart D.Jackson3.3Thermal IssuesAs higher pump powers become available from laser-diode systems,it is gen-erally recognized that thermal and thermo-optical issues set limitations to the power scalability of end-pumped bulk-laser systems.Owing to the unfavor-able temperature dependence of thermal and thermo-optical parameters[53], the large heat load in the crystal leads,firstly,to a significant temperature increase in the rod,secondly,to strong thermal lensing with pronounced spherical aberrations,and ultimately,to rod fracture in a high-average-power end-pumped system.Due to its geometry,thefiber provides potentially high pump-and signal-beam intensities without the drawbacks of significant thermal and thermo-optical effects.Its large surface-area-to-volume ratio means that the heat generated from multiphonon relaxation in the core is dissipated effectively by radiation and convection from the outer surface of thefiber.This is es-pecially true for single-clad,core-pumped single-modefibers where this ratio is highest[54].Double-cladfibers have a relatively smaller surface-area-to-volume ratio and thermal issues need to be taken into account[6,55,56]. Thermal management will be required when very high output powers are desired.In particular,for high-power mid-infrared operation,thermal man-agement may be very important because of the decreased quantum efficiency and the consequently higher amount of heat dissipation.4Spectroscopic and Laser Propertiesof Rare-Earth IonsThe structure of a glass is less well defined as compared to a crystalline mate-rial.The local variation of the chemical environment of active ions in a glass has a number of consequences.Most important,the active ions may undergo chemical reactions during the fabrication process and be incorporated in the host in several oxidation states with different spectroscopic properties.Oxi-dation states other than the desired one may act as impurities that introduce undesired optical effects such as parasitic pump absorption,the reabsorption of oscillating laser light,the lifetime quenching of the laser ion,and the trap-ping of the excitation energy.A stable oxidation state of the optically active ion is thus highly desirable.The necessity of a stable oxidation state excludes a number of transition-metal ions from the list of suitable dopants in glass environments.This is one of the possible reasons why examples of transition-metal-ion-doped lasers in glass hosts are rare.On the other hand,most of the rare-earth ions prefer to stabilize in the trivalent oxidation state and are, therefore,suitable candidates as glass andfiber dopants.This chapter will, therefore,concentrate on the rare-earth ions as active dopants offiber lasers.Mid-Infrared Fiber Lasers227 4.1Spectra of Rare-Earth Ions in GlassesThe optical transitions of lanthanide(rare-earth)ions in the visible and in-frared spectral region occur within the4f subshell.This subshell is shielded by the outer5s and5p subshells and the influence of the host material isrelatively small compared to,e.g.,the3d transitions in transition-metal ions.The electronic structure of trivalent rare-earth ions derives from the perturba-tion of the4f energy level in the central-field approximation by the noncen-trosymmetric electron–electron interaction,the spin–orbit interaction,andthe crystal-field splitting(Stark effect);see the example of the energy-level scheme of Er3+in Fig.4.The spin–orbit multiplets are commonly denotedby their2S+1L J terms in Russell–Saunders coupling,although the4f elec-trons of lanthanide ions exhibit intermediate coupling and the total angularmomenta J of the spin–orbit multiplets are linear combinations of the totalorbital angular momenta L and total spins S.Single crystal-field(Stark)tran-sitions between two spin–orbit multiplets cannot be distinguished in glasses at ambient temperature,because inhomogeneous spectral-line broadening oc-curs due to the local variation of the ligand electricfield.Also homogeneous (lifetime)broadening mechanisms are relevant in a number of glasses.This spectral-line broadening makes glasses the preferred hosts when broadband,Fig.4.Energy-level scheme of triva-lent erbium indicating the splitting ofthe4f11configuration in the central-field approximation by the noncen-trosymmetric electron–electron inter-action,the spin–orbit interaction,andthe Stark splitting by the local elec-tricfield of the host material(indi-cated only for selected spin–orbit mul-tiplets)228Markus Pollnau and Stuart D.Jacksoncontinuous tunability of lasers is desired.On the other hand,the spectral-line broadening leads to lower absorption and emission cross-sections for the same transition in glasses compared to single-crystalline hosts.The reducedcross-sections lead to generally higher pump threshold of laser transitions inglasses,a fact that is compensated infiber geometry because a high pump confinement is achieved over the wholefiber length.4.2Intraionic ProcessesGenerally,the probability of an allowed electric-dipole transition is seven or-ders of magnitude larger than that of an allowed magnetic-dipole transition.Since electric-dipole transitions within the4f subshell are parity forbidden,the intensities of radiative transitions in rare-earth ions are weak and the radiative lifetimes of the emitting states are long,typically in the ms range.Mixing of the4f states with higher-lying(typically5d)electronic states of opposite parity at ion sites without inversion symmetry,however,means thatelectric-dipole transitions become partially allowed and are usually the dom-inant transitions between4f electronic states.The oscillator strengths f and integrated absorption and emission cross-sectionsσof these spin–orbit mul-tiplet-to-multiplet transitions can be calculated with the help of the semi-empirical Judd–Ofelt theory[57,58].If the degree of inhomogeneous spectral-line broadening is relatively small and the absorption and emission spectraremain structured,as is the case for ZBLAN,the cross-sectionsσ(λ)at in-dividual wavelengths that are relevant to pump absorption and stimulatedemission of narrow laser lines must be determined experimentally.Besides ground-state absorption(GSA),excited-state absorption(ESA) of pump photons,see Fig.5a,can play a significant role infiber lasers,specif-ically in the case of high-intensity core pumping.An experimental examplewill be given later in Sect.7.1.Since the absorption increases exponentially with the absorption coefficientα(λP)=Nσ(λP),ESA becomes relevant forthe population dynamics of a laser when(a)the ESA and GSA cross-sectionsσ(λP)are comparable at the pump wavelengthλP and(b)the population density N of the excited state in which the second pump-absorption steporiginates becomes a significant fraction of the density of ions in the ground state,i.e.,a large degree of ground-state bleaching must be present for ESA to play a significant role.A radiative transition from an excited state i to a lower-lying state j is characterized by the radiative rate constant A ij.If the decay occurs to sev-eral lower-lying states,the overall radiative rate constant A i is the sum of all individual rate constants.The branching ratio of each radiative transition is defined asβij=A ij/A i.Radiative decay of excited states is in competition with nonradiative decay by interaction with vibrations of the host material, called multiphonon relaxation.The rate constant of a multiphonon relaxation process decreases exponentially with the energy gap to the next lower-lying state and with the order of the process,i.e.,the number of phonons required(a)Ion (b)12Donor Ion Acceptor IonAcceptor Ion (c)Sensitizing Ion ALaser Ion B(d)1Laser Ion AQuenching Ion B (e)Donor Ion Acceptor Ion(f)1Donor Ion Acceptor Ion Fig.5.Intra-and interionic processes infiber lasers:(a)excited-state absorption (ESA);(b)energy migration;(c)sensitization and(d)quenching of a laser ion by an ion of a different type;(e)cross-relaxation and(f)energy-transfer upconversionto bridge the energy gap[59,60].This fact is illustrated in Fig.1for differ-ent glasses.The rate constant of multiphonon relaxation increases with host temperature.The measurable luminescence lifetimeτi of an excited state i is the inverse of the sum of the overall radiative rate constant A i and the rate constant of multiphonon relaxation,W i.The radiative quantum efficiency is defined asη=A i/(A i+W i).The influence of multiphonon relaxations is stronger in oxides as com-pared tofluorides because of the smaller atomic mass m2of the anion and the larger elastic restoring force k,see(1),due to stronger covalent bonds in oxides[3],both resulting in larger maximum phonon energies in oxides.A brief example:The luminescence lifetime of the4I11/2upper laser level of the erbium3µm laser(Sect.7)is partly quenched by multiphonon relaxation. Typically,nonradiative decay becomes dominant iffive or less phonons are required to bridge the energy gap.With an energy gap between the4I11/2and the next lower lying4I13/2levels of≈3400–3500cm−1,radiative decay pre-vails for phonon energies below≈600cm−1,roughly the maximum phonon energy of ZBLAN,see Table1.Fluorides are,therefore,preferred over oxides as host materials for most of the mid-infrared laser transitions.Like absorption,the strength of a stimulated-emission process is char-acterized by the emission cross-sectionσ(λL)of the laser transition.From a simple analysis,for one resonator round-trip of oscillating laser photons, the productτσ(λL)withτthe luminescence lifetime of the upper laser level, is identified as a“figure of merit”for a possible laser transition.The larger this product,the lower is the expected pump threshold of the laser transition. This“figure of merit”,however,does not take into account the numerous par-asitic effects that can occur in the population dynamics of a laser system,such as pump ESA,reabsorption of laser photons,and energy-transfer processes. It is often these parasitic processes that lead to surprising performance char-acteristics–as likely in the negative as in the positive sense–and make the interpretation of rare-earth-doped solid-state lasers challenging.Examples will be discussed in Sects.5–7.4.3Interionic ProcessesIn addition to intraionic excitation and decay mechanisms,radiative en-ergy transfer due to reabsorption of emitted photons by other active ions in the sample and nonradiative energy-transfer processes due to multipole–multipole or exchange interactions between neighboring active ions can occur. Radiative energy transfer leads to an increase in the luminescence lifetime. Among the nonradiative energy-transfer processes,most common is the elec-tric dipole–dipole interaction,which can occur as a direct[61]or phonon-assisted[62]energy transfer.A direct energy transfer requires spectral reso-nance between the involved emission and absorption transitions whereas an indirect transfer can also be nonresonant,i.e.,an existing energy gap between the emission and absorption transitions involved in the transfer is bridged by。
文献研读的一些建议

文献研读的一些建议1、文献研读通常比我们想象地复杂;2、阅读必须和批判性思考相结合,没有批判性思考的阅读,无异于用眼睛不假思索地扫描。
3、分类按主题研读文献是非常重要的;它对于你的选题极为重要;4、文献研读本身就是一种学习,向他人学习,向有经验的人学习。
5、在研读的时候,一定要想想,如果你是作者,你会怎么处理。
6、在阅读文献时思考的问题作者解决了什么问题?为什么这些问题是重要的?作者真正做了些什么?(与作者声称的相对照)这个研究工作的贡献是什么?(对领域来说是新的或者有趣的东西有哪些)作者使用了什么样的方法(具体数据收集)?如果是你,你会怎么解决这个问题?这篇研究报告的逻辑是否严谨完整?这篇报告的结论是什么?是否完成了预定目标?7、文献阅读必须与写作相结合。
将你的灵感、评论、感言、想法写下来,这非常重要。
8、阅读与检索相结合,这往往是阅读的经常伴随状态。
9、文献研读之后,如必要,可以与作者进行联系交流和讨论。
10、你不必从头到尾地阅读。
建议的阅读顺序是:标题、摘要、引言(或问题的提出)、审视文章的结构、结论、文章主体、通读。
如果必要再重点研读和重读。
11、阅读技巧阅读标题(这篇文章是关于什么的?)阅读摘要(将会给你一个梗概)阅读引言(看看动机、相关的其他工作、更详细的背景)看看文章的结构(剩余部分主要强调的是什么?各个部分之间的逻辑联系)阅读结论(它们的结论是什么?新知识?)阅读文章的主体(细节重读、研读、查读)12参考文献并非可有可无。
有的时候,对你真正有价值的东西是在参考文献中。
13阅读文献就是学习。
学习该研究发现的新知识(结论),学习作者提出问题的方法、学习作者对问题的表述、学习作者是如何收集和处理数据的(研究方法)。
文献研读的第一步是选择合适的高质量的文献;有些教育硕士,读文献就只读以前的教育硕士的学位论文,这样自己的论文水准能提高多少?值得怀疑。
有些同学选择文献只依照方便原则。
文献不够集中。
新视野大学英语第五册单词(注释+例句)~
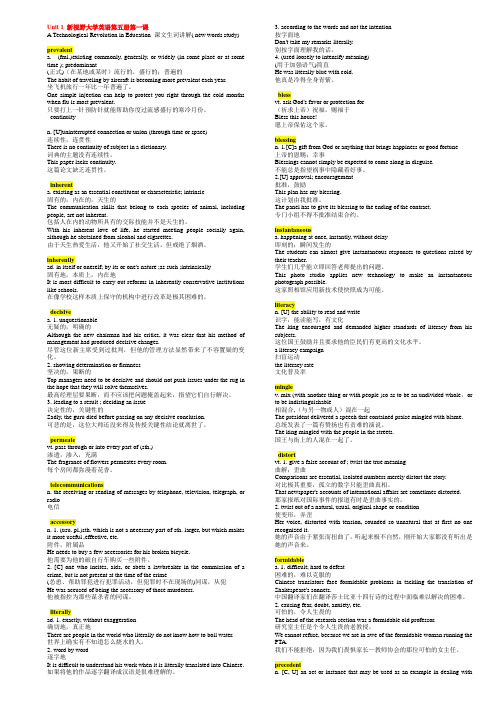
Unit 1 新视野大学英语第五册第一课A Technological Revolution in Education 课文生词讲解( new words study) prevalenta. (fml.)existing commonly, generally, or widely (in some place or at some time ); predominant(正式)(在某地或某时)流行的,盛行的;普遍的The habit of traveling by aircraft is becoming more prevalent each year.坐飞机旅行一年比一年普遍了。
One simple injection can help to protect you right through the cold months when flu is most prevalent.只要打上一针预防针就能帮助你度过流感盛行的寒冷月份。
continuityn. [U]uninterrupted connection or union (through time or space)连续性,连贯性There is no continuity of subject in a dictionary.词典的主题没有连续性。
This paper lacks continuity.这篇论文缺乏连贯性。
inherenta. existing as an essential constituent or characteristic; intrinsic固有的,内在的,天生的The communication skills that belong to each species of animal, including people, are not inherent.包括人在内的动物所具有的交际技能并不是天生的。
脑炎影像表现英文版护理讲座
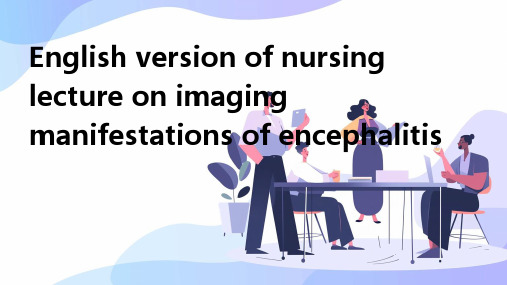
目录
• Overview of ethics • Imaging presentations of ethics • Encephalitis care • Encephalitis English Professional
Classification
Encephalitis is classified based on the cause, with virtual ethics being the most common type Other types include bacterial, fungal, and parasitic ethics
Provide comfort measures
Ensure that the patient is comfortable, and provide pay relief or sedimentation if necessary
Rehabilitation care
Physical rehabilitation
Pathogenesis
The pathogenes of ethics involve the invasion of viruses into the central nervous system (CNS) and the subsequence immediate response mounted by the body to clear the infection This immediate response can lead to inflation and damage to the brain issue
有关911的英文介绍
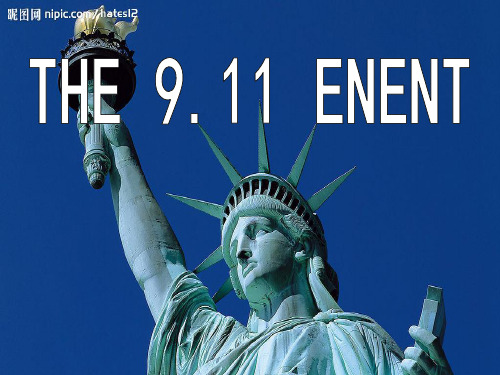
9/11 conspiracy theories attribute the planning and execution of the September 11th,2oo1attacks against the United States to parties other than, or in addition to, alQaeda or claim there was advance knowledge of the attacks among high-level government officials. Government investigations and independent reviews have found no evidence for the theories. Proponents of these conspiracy theories claim there are inconsistencies in the official conclusions, or evidence which was either ignored or overlooked.
An_empirical

Business financing in China 959Management Research News Vol.31No.12,2008pp.959-975#Emerald Group Publishing Limited0140-9174DOI 10.1108/01409170810920666An empirical evidence of small business financing in ChinaJunjie WuSchool of Accountancy and Financial Services,Leeds Metropolitan University,Leeds,UK Jining SongBusiness School,Central University of Finance and Economics,Beijing,People’s Republic of China,andCatherine ZengDeloitte Touche Tohmatsu LLP,London,UKAbstractPurpose –The purpose of this paper is to provide empirical quantitative evidence concerning small business financing in China and highlight the financing problems faced by small to medium-sized enterprises (SMEs)in developing their businesses.Design/methodology/approach –A semi-structured questionnaire survey was conducted to collect data from a sample of 60small businesses in three cities in China.Descriptive methods and the SPSS statistical software package were used to analyse the data and interpret the results.Findings –The data gathered covered current topic in research including the capital structure of SMEs at start-up,the types and extent of funding shortage,the preference of financial resources as SMEs grow,the significant factors,which help SMEs secure bank loans and the influence of a firm’s size,age and the like.The findings generally support financial theories and previous studies about SMEs but also offer the basis for new arguments about financing SMEs in China.Research limitations/implications –The sample size is relatively small and statistical analysis is relatively straightforward.Practical implication –The present study will be of interest to policy makers developing new strategies and policies to support the financing of SMEs in China.Originality/value –The results from this study contribute to the understanding of current problems in financing Chinese small business enterprises.These include findings,which were not presented in other similar studies.Keywords China,Small tomedium-sized enterprises,Financing,Capital,BorrowingPaper type Research paperIntroductionA healthy and robust small and medium-sized enterprise (SME)sector is vital for sustainable competitive advantage and economic development in both developed and newly industrialized countries (NICs).This is especially the case for NICs in transition from a command to a market economy.Therefore,SMEs have been of primary interest to the policy makers in these countries.After a period of dominance by state-owned enterprises (SOEs)in China,private SMEs have made an outstanding contribution to China’s sustained and rapid economic development in recent years.According to China’s National Development and Reform Committee (NDRC,2006),SMEs represent 99.3per cent of the registered enterprises in the country and account for 55.6per cent of Chinese gross domestic product (GDP),62.3per cent of exports,46.2per cent of tax revenues and 75per cent of employment opportunities in cities and towns.The difficulty of financing SME growth is a universal problem.However,the issue is particularly poignant in China.Due to history,the command economy saw SOEs dominate the industrial landscape.These organisations were high up on thepolitical The current issue and full text archive of this journal is available at/0140-9174.htmMRN 31,12 960pecking order.Stakeholders of SMEs find they are discriminated against by a lack of access to external funding,uncertain property rights protection,the taxation regime and market opportunities.SME development in China today faces serious constraints, including scarcity of long-term financial resources(for example,long-term loans and venture capital)and a lack of managerial,financial,marketing and technical expertise. However,despite some improvement,change is still required in institutional services, enterprise development oriented toward industrial training,under-developed regulative bodies and law enforcement mechanism(Poutziouris et al.,2002).In response to the need to change and promote growth among SMEs in China,the government promulgated several laws including the Law of Small and Medium Sized Enterprises in June2002.Such laws were to provide clearer lines of legal responsibility in the sector.At the municipal level,entities like the Dalian Municipal Economic Commission view their role in formulating development planning for SMEs very seriously.The Commission promotes the establishment of service agencies for SMEs and seeks to improve the approval process for guaranteeing loans to SMEs(http:// /catalogdetail.jse).In this context,the credit guarantee agencies funded by the government were established to assist SMEs raise funds from financial institutions.However,questions remain unanswered concerning the actual success of the government’s initiative and the extent of the financial constraints that hinder SME expansion and growth.Against this background,SME financing remains one of the most under-researched areas in China.In addition,the murkiness of information,a lack of detailed micro-economic data on the small business sector and the funds SMEs raise in private equity and debt markets have made small business financing one of the most difficult fields in which to conduct empirical research(Berger and Udell,1998).Nevertheless,the increasingly important role of SMEs in the Chinese national economy also makes it one of the most interesting areas to study.This research seeks to contribute in this strategic area.The main objective of the study is to provide some preliminary descriptive evidence of SME financing.To achieve this end,a questionnaire survey was administered to a sample of60small businesses in China examining issues including the capital structure at start-up,the categories and extent of funds shortage,the financing pecking order in the growth period,the percentage of exercised bank loans and the important factors that determine the approval of such loans.Literature reviewA number of features distinguish small businesses from large firms in the context of financial management practices(Ang,1991).For example,small businesses are not publicly traded and rely mainly on private equity and debt markets to raise capital. These enterprises are normally owner–managed and the owner-managers generally possess general rather than specific expertise.Transaction costs in small businesses are usually higher than those costs in the large enterprises.Below is an identification of the relevant literature and previous studies pertinent to understand how small businesses are financed.Capital structure theories and financial growth circle modelCapital structure theory stems from the work of Modigliani and Miller(MM)(1958)on optimal capital structure,which has been extended and developed by a number of academic researchers.One of MM’s propositions suggests that the value of a companyBusiness financing in China 961is unchanged by the alternative mix of capital structure.Although a perfect market condition does not exist,the MM model is the starting point for the researching decisions about capital structure.Jensen and Meckling’s (1976)agency theory provides further insight into the financial management of capital structures (Gregory et al.,2005).According to Jensen and Meckling (1976),a firm must manage an agency relationship which is ‘‘a contract under which one or more persons (the principal[s])engage another person (the agent)to perform some service on their behalf which involves delegating some decision-making authority to the agent’’and consequently,pay agency costs that are the costs to keep an agency relationship such as those between owners and managers or between owner/managers and their debtors in an additional form (Cassar and Holmes,2003).Another important capital structure theory is Mayers’(1984)pecking order model,which suggests that firms have a particular preference order for their financing choices.In his opinion,due to the information asymmetry between the firm and potential financiers,managers know more about the firms’value and prospects than new investors (for example,new shareholders and lenders).Therefore,these new investors will expect a higher return on the capital they invest.It results when the net value of investment is positive.The firm will prefer inside finance to outside debt,short-term debt over long-term debt and outside debt over outside equity in order to keep the original ownership and control of the firm intact.Therefore,the best financing pecking order of a firm would be inside finance,issuing debt,then issuing equity (Cassar and Holmes,2003).Many researchers have examined the capital structure decision from the perspective of small firms (for example,Barton and Gordon,1987;Berger and Udell,1998;Cassar and Holmes,2003;Coleman,1988;Collins and Moore,1964;Dollinger,1995;Ferri,1981;Gaskill and Van Auken,1993;Gregory et al.,2005;Jones,1979;Kimki,1997;Poutziouris and Chittenden,1996;Titman and Wessels,1988;Van Auken and Neeley,1996;Welsch and White,1981;Wucinich,1979).This is because problems related to financing arising predominantly in small businesses and many failures in small and young firms are caused primarily by the inadequate financial resources available to fund the start-up and growth stages (Terpstra and Olson,1993).It is widely accepted by researchers that a capital structure decision between equity and debt in small businesses is partially different to large firms because of the transparency of information.Among the studies cited above,Berger and Udell’s (1998)financial growth model has attracted the attention of many researchers and has been accepted as a view of small-business capital structure (Gregory et al.,2005).Their model proposes that small businesses have a financial growth cycle in which financial needs and options change as the firm starts-up,grows,gains further experience and becomes more transparent with its information.Berger and Udell (1998)further suggest that a firm’s financial needs and options lie on a size/age/information continuum.When firms are in the smaller/younger/more opaque stage,they must rely on initial finance (for example,at start-up,the owner,family or friends provide funds),trade credit and/or finance from a guardian angel.In the growth stage,firms gain access to intermediated finance from both the equity and debt side (for example,venture capital and bank loans).As they continue to expand,firms may be able to gain access to public equity and debt markets.However,Berger and Udell (1998)also indicate that ‘‘the growth cycle paradigm is not intended to fit all small businesses and that firm size,age and information availability are far from perfectly correlated’’.MRN 31,12 962Other empirical studies on SME finance either fully or partially support or reject those capital structure theories and models.For example,Poutziouris et al.(1996), Dollinger(1995),Kimki(1997)and Barton and Gordon(1987)find that the life cycles of small entities influence their capital structure decisions.Jones(1979),Titman and Wessels(1988)and Homaifar et al.(1994)suggest that a positive correlation exists between firm size and capital structure.However,Norton(1990,1991)conjectures that these theories have very little relevance to capital structure decision-making in small firms.Kimki(1997)explores the relationship between capital structure and types of industry and his results demonstrate that a high debt ratio exists in firms in the machine,food,medicine and electronic power industries.Lending infrastructure,lending technologies and the difficulty of SME financing According to Cosh and Hughes(2000),the majority of SMEs have experienced rapid rates of growth and funding shortages during their embryonic years.The absence of adequate funding represents a major obstacle to a firm’s survival and chance of success during this start-up period(Hanson,1983;Westhead and Wright,2000).As a result,firms of this type become dependent on the availability of external sources of finance,especially external debt rather than external equity,reaffirming the pecking order hypothesis.The evidence suggests that the vast majority of small businesses identify commercial banks as their primary loan lenders,probably because commercial banks provide a wider range of credit,transaction,deposit and related financial services than do other financial institutions(Berger and Udell,1998;Riding et al.,1994).The access of SMEs to external finance has attracted much attention from academics and policy makers worldwide.The availability of credit for SMEs depends significantly on the nation’s financial structure and its accompanying lending infrastructure and technologies.Through these mechanisms,government policies and financial structures influence the level of credit available(Berger and Udell,2006). According to Berger and Udell(2006),the lending infrastructure ranges from the information,tax,legal,judicial and bankruptcy environments to the social and regulatory environments.They believe that‘‘all of these elements may affect SME credit availability by influencing the extent to which the different lending technologies may be legally and profitably employed’’.Lending technologies include information on which financial institutions rely upon to determine the supply of debt finance to firms while simultaneously addressing opacity problems.Current framework of lending technologies consists of two categories.One is transaction lending,which is based mainly on quantitative data(for example,asset-based lending and financial statement lending).The other is relationship lending that is largely based on qualitative information(that is, information gathered through continuous contact with the firm that wants the provision of financial services(Allen et al.,1991;Berger and Udell,1995;Cole,1998; Petersen and Rajan,1994).Other factors influencing a financial institution’s decision to grant(or not grant)a term loan include managerial strengths,income potential,strength of market,potential of a project and even geographical location(Haines et al.,1989).Financial theories have traditionally been developed to explain capital structure with empirical evidence,mainly based on large listed firms(Cassar and Holmes,2003). The literature has,therefore,attracted a number of studies focusing on gathering empirical data from small firms to explore if these arguments are also valid when applying them to institutional and large-scale settings.Binks et al.(1990)argue thatBusiness financing in China 963small businesses continually complain about a competitive disadvantage because of their inability to raise funds (that is,the so-called finance gap).This refers to a situation where a firm has profitable opportunities but insufficient funds,either from internal or external sources to exploit an opportunity.A finance gap arises because the demand from small firms exceeds the willingness of financial institutions to supply the finance at the current market conditions,a situation recognised decades ago in the report of the MacMillan Committee (1931).Today’s shortage of finance has been reported as a particular problem for SMEs in different stages of development and has attracted much attention from government,policy makers and academics (for example,ACOST,1990;Carter and Joues-Evans,2000;Robson,1984).The principal reason a finance gap exists in SME financing is that on the one hand,financial institutions use income-gearing approaches as basic criteria to evaluate potential loans by taking account of forecasted future income flows of enterprises.On the other hand,SMEs are not publicly priced and their labour force,suppliers and customers are generally kept private.Most SMEs do not have audited financial statements;consequently,it is difficult for these firms to convey quality and build up a reputation to overcome the information opacity surrounding their activities (Berger and Udell,1998).Small business financing in ChinaSince China’s Constitution acknowledged the private sector to be an integral part of the economy at the end of 1990s,the government has gradually lifted barriers on the development of SMEs to enable small businesses to develop quickly and become the growth engine of the Chinese economy.This is especially the case following China’s WTO membership in 2001(Chen,2006;Poutziouris et al.,2002).However,far from rewarding their contributions to the Chinese economy,SMEs are discriminated against in terms of their access to external funding because of the historical political pecking order of firms in China.The under developed financial market,which leads to the allocation of financial resources to the least efficient SOEs rather than most efficient private firms,also contributes to this level of discrimination (Podpiera,2006).For example,in June 2004,there were 3.3million registered private enterprises in China,which employed 47.1million people.SMEs generated more than half of Chinese GDP (compared with only 17per cent generated from SOEs)but received only 10per cent of all bank loans in 2004(compared with more than half received by SOEs)(PBOC,2004).The situation can be explained by governmental interference,the historical relationship between SOEs and the state-owned commercial banks (SOCBs)and China’s financial market environment.The Chinese banking system is made up of 5large SOCBs,3policy banks,12joint-stock commercial banks (JSCBs),124city commercial banks (CCBs),42urban credit cooperatives (UCCs),8,348rural credit cooperatives (RCCs),17rural commercial banks,and 113rural cooperative banks (CBRC,2007).While the four SOCBs account for 52.46per cent of total banking assets (Cousin,2007),the state holds between 95and 99per cent of all banking assets directly from SOCBs and indirectly through local governments and SOEs from the JSCBs and the CCBs (Beckier et al.,2005;OECD,2005).Apart from having a controlling equity stake,the state also has provided generous financial support to financial institutions.With this support,the state holds a very influential position.This allows the state to permeate strategic,operational and managerial decisions (Cousin,2007).For example,although inefficient SOEs are partly responsible for the large volumes of non-performing loans (NPLs)in China’s SOCBs,large SOEs still attract the majority ofMRN 31,12 964bank loans because the risk attached to these loans are thought of as being low–there is the high likelihood that failing SOEs will be rescued by the government if they are declared bankrupt(Cousin,2007).Additionally,larger SOEs and SOCBs have similar organisational structures and administrative styles,which were inherited from a time before the reforms that accompanied the open door policy.This shared interest creates an environment where lending by SOCBs is still partially determined by political reasons rather than by commercial motives(Cousin,2007;Park and Sehrt,2001). Moreover,the Chinese financial market is an under-developed debt and capital market with some peculiar features.Banks certainly face a number of challenges in China,such as insufficient capital, government intervention,poor risk management practices and high levels of liquidity caused by large numbers of NPLs.The immature financial system also places pressures on the banks.For example,banks require more tools to control the veracity and completeness of the information submitted to them by SME borrowers–there is a lack of credit registries for small businesses in China.From the side of the SMEs,the fact that most of them are still in the early stages of their development means they lack formal structure,good governance and collateral.The latter in particular poses an obstacle for the SMEs because many banks only accept very limited types of tangible fixed assets such as buildings or lease of land as collateral.These factors influence the access of SMEs to bank loans because these assets would be required to enhance the creditworthiness of SMEs.A lack of assets limits the capacity of SMEs to obtain loan guarantees from the banks(Zhang,2006).But such a loan guarantee system has not played the role as originally intended.In order to support SME development by improving their credit environment,from the beginning in1999,the Chinese Ministry of Finance in collaboration with other government departments set up a SME loan guarantee system.This process established more than200credit-guarantee institutions with a fund of10billion Yuan.However,today,the sector stands at a crossroad and it is expected that the industry will shrink in the future as it re-focuses its strategy(Cousin,2007).Wang(2004),for example,suggests that the current SME development boom might slow down without a long-term,systematic,unified and relatively independent SME development strategy and a system governed by effective policies(2004).Methodology and sample characteristicsThe approach to the research involved gathering data via a semi-structured questionnaire survey.The questionnaires were distributed and addressed to the owner or accountant of100SMEs in three cities of China in2006by mail and in person.The cities concerned were Beijing(the capital of China),Jinan(the capital city of Shandong province,which is one of middle-developed provinces in China)and Liaocheng(a small city in Shandong province).These cities are representative of large,medium and small-sized cities in China.Sixty questionnaires were completed and returned,which represent a response rate of60per cent.During the questionnaire design stage,a pre-test and a pilot study were conducted and the outcomes were considered carefully in order to improve the final version of the questionnaire.In this context,comments were collected from academics and practitioners concerning the questionnaire’s structure,content,wording and layout.All questions in the survey were close-ended to limit the respondents’’answers to the survey questions and encourage their focus on the issue at hand.Descriptive statisticalBusiness financing in China 965methods were employed to analyse and explain the data.The questions supporting this study were drawn from a large survey (available for the corresponding author).The characteristics of the sample are summarised in Table I.The data indicates that a large proportion of the respondents are from wholesale and retail companies.The SMEs are mainly family-owned small firms,which run businesses operating in local areas and employing fewer than 100employees.Most of the SMEs are at the start-up stage with an owner/manager who is on average between 40and 50years of age.These owner/managers tend to possess relatively low education qualifications.Survey results and discussionFinancial resources of start upOne question concerned the financial resources at start-up.These results,shown in Table II,indicate clearly that the key financial resources for the respondents at business start-up were the owner’s private savings,money borrowed from family and friends and bank loans.The findings are consistent with other studies in Western counties and in China.For example,Bank of England (2003)data suggest that 60per cent of business owners used their personal funds and finance from family members as the main sources of capital to start their business.In China,the results from a series of surveys by the China Industrial and Commercial Union and the Research Commission of Chinese Private Business in 1993,1995,1997,2000and 2002suggest that 65.5per cent of capital originated from self-accumulation,21per cent from bank loans and ruralTable I.Sample characteristicsPer cent (%)Per cent (%)Types of industryNumbers of employees Manufacture23.3<10051.7Construction13.3100-30025.0Wholesale and retail33.3301-5008.3Transportation11.7>50015.0Hotel and restaurant13.3100.0Others5.1100.0Types of tradingBusiness coverage Sole trader28.3Local 63.3Partnership10.0National 26.7Limited liability Co.61.7International 10.0100.0100.0Types of ownershipAge of owners Individually or family owned71.7<308.3Collectively owned8.330-3930.0State owned15.040-5051.7Share holding corporation5.0>5010.0100.0100.0Years in businessQualification of owners 0-3year31.7Up to high school 31.74-6year28.3Diploma 23.37-10year20.0First degree 30.0>11year20.0Master or over 15.0100.0100.0Note:Sample number ¼60MRN 31,12 966credit co-operatives and13.5per cent form funds borrowed from friends,relatives and other individuals when private firms were started(Li,1998;Wang,2004).Obviously,the proportion of SMEs using bank loans(56.7per cent)in our survey at start-up is higher than the figures reported by previous studies.Additional analysis from another question in the survey,which asked respondents to indicate if they have a bank loan,revealed that61.7per cent of the respondents had access to a bank loan. Therefore,the results might suggest that in recent years,the financial market in China has improved significantly.The Chinese banking sector is working reasonable well for the private firms and now small businesses are finding it easier to establish loans from banks and other financial institutions compared to the difficulties faced in recent years. Categories and extent of funding shortagesAnother question inquired about the types and extent of funding shortages faced by SMEs.The responses,summarised in Table III,suggest that SMEs face serious shortages in funding their day-to-day operational needs and expanding their business capacity. However,it is not surprising that the results indicate that the same owner/managers do not lack funds for the training and development of staff and expanding their market range. These positive result,however,are probably due to the small size of the firms at start-up.The problems of a lack of working and development capital among the operators of SMEs also exist in other countries and have attracted the attention of policy makers.In the UK,most SMEs encountered difficulties in accessing finance to support capital development and to provide working capital(Tucker and Lean,2001).Banks also favour short-term loans,which limits the potential of SMEs to undertake long-term strategic planning(Deakins and Hussain,1994).These barriers resulted in the UK government’s attempt to address the apparent failure of the market to supply adequateTable III.Extent of shortage of financial resourcesMean SD Rank Working capital 4.750.50841 Funds for purchasing new buildings and equipment 4.170.58492 Funds for technology improvement 2.950.53443 Funds for market expansion 2.470.59564 Funds for training staff 1.530.62355Notes:A5-point scale was used to collect data;score:1,not short at all;5,extremely short;3, mid point.Table II.Financial resources at start upNumber Per cent(%) Owner’s personal savings4473.3 Borrowing from friends and relatives3863.3 Bank loan3456.7 Funds from business partners1728.3 Funds from external parties1423.3 Funds from internal employees1118.3Note:Per cent¼number/60Business financing in China 967finance for small businesses,especially those in their start-up phase.Therefore,supporting the entrepreneurial activities of SMEs is now one of the UK government’s important strategies as it is in China.Preference of financing in growth periodThe pecking order hypothesis suggests that the owners of firms have a particular preference order for their choices concerning finance.They prefer to use internal rather than external finance and to use debt rather than equity.In fact,the literature on SMEs suggests that owner/managers are reluctant to give up their independence and control (Berggren et al.,2000;Berger and Udell,1998;Cosh and Hughes,1994;Cressay and Olofsson,1997;Jordan et al.,1998;Poutziouris et al.,1998).To test the pecking order theory,a question was designed to solicit responses about the financial preference of owner/managers during the growth period of their enterprises.These results are set out in Table IV .Considering the findings in Table II,at the start-up phase the owner/managers relied mainly on internal financing and assessed personal savings (73.3per cent)and borrowing from friends and relatives (63.3per cent).However,when businesses expand,they are keen on borrowing money from the debt market (80percent choose this as their first choice)rather than absorbing equity holders (only 1.7per cent preferred this option as their first choice).Therefore,the results confirm and support the pecking order hypothesis.Previous studies suggest that the independence motive is critical to understanding the observed choices about the capital structure of small businesses.In addition,the high demand for bank loans indicates that small businesses in China have a real need to access the outside debt market to fund rapid development.Importance of factors in helping secure bank loansConcerning what factors play important roles for SMEs in obtaining bank loans,one question was designed to identify those factors.The results are listed in Table V .Among 13factors,in rank order the following five factors emerged as being the most important:.secured property;.taxation submission;.national finance policies;.accounting documents (including profitability information);and .credit scoring.The results suggest that secured property is the most important factor in securing a bank loan.It supports the findings of other studies,which suggest that in mostTable IV .Preference of financialresources in growth period FirstSecond Third Total No.%No.%No.%No.%Bank loan4880.01220.00060100.0Internal financing1321.72846.71931.660100.0External equity fund 1 1.71931.64066.760100.0Note:Sample number (n ¼60)。
- 1、下载文档前请自行甄别文档内容的完整性,平台不提供额外的编辑、内容补充、找答案等附加服务。
- 2、"仅部分预览"的文档,不可在线预览部分如存在完整性等问题,可反馈申请退款(可完整预览的文档不适用该条件!)。
- 3、如文档侵犯您的权益,请联系客服反馈,我们会尽快为您处理(人工客服工作时间:9:00-18:30)。
Proceedings of the Third International Conference on Hybrid Intelligent Systems (HIS’03): Design and Application of Hybrid Intelligent Systems, A. Abraham, M. Koppen and K. Franke (Editors),Pages 692-701, December 2003, IOS Press.An Empirical Investigation of the Impact ofDiscretization on Common DataDistributionsMichael K. Ismail Vic CiesielskiDepartment of Computer ScienceRMIT University, MelbourneVIC 3001, AUSTRALIAAbstract.This study attempts to identify the merits of six of the most populardiscretization methods when confronted with a randomly generated datasetconsisting of attributes that conform to one of eight common statistical distributions.It is hoped that the analysis will enlighten as to a heuristic which identifies the mostappropriate discretization method to be applied, given some preliminary analysis orvisualization to determine the type of statistical distribution of the attribute to bediscretized. Further, the comparative effectiveness of discretization given each datadistribution is a primary focus. Analysis of the data was accomplished by inducing adecision tree classifier (C4.5) on the discretized data and an error measure was usedto determine the relative value of discretization. The experiments showed that themethod of discretization and the level of inherent error placed in the class attributehas a major impact on classification errors generated post-discretization. Moreimportantly, the general effectiveness of discretization varies significantlydepending on the shape of data distribution considered. Distributions that are highlyskewed or have high peaks tend to result in higher classification errors, and therelative superiority of supervised discretization over unsupervised discretization isdiminished significantly when applied to these data distributions.IntroductionDiscretization of continuous attributes not only broadens the scope of the number of datamining algorithms able to analyze data in discrete form, but can also dramatically increasethe speed at which these tasks can be performed [1]. There have been many studies thathave evaluated the relative effectiveness of the many discretization techniques along withsuggested optimizations [4,6,7]. However, these experiments have usually been carried outwith application to real-world datasets where no attempt has been made to identify the typeof probability density function of the continuous attribute.The effectiveness of various discretization methods as applied to a variety of data will be evaluated. All data has been artificially constructed and conform to common statisticaldistributions. Discretization and data mining operations will be performed using the WEKAdata mining software [11]. The comparative responsiveness of each distribution todiscretization is the primary focus of the experiments and will be achieved by the analysisof classification errors resulting from discretization. Thus, it is hoped that by datavisualization alone we can make inference as to the effectiveness of any subsequentdiscretization apriori and if applicable, isolate the most supportive discretization method toadopt. There are eight statistical distributions chosen that will be analyzed, along with sixdiscretization methods.1. Data Generation MethodologyEach attribute was formed with 10,000 instances as this provided accurate measures in terms of achieving the desired symmetry in distributions. Each class attribute was formed with an inbuilt error or class overlap (1%, 5%, 10%, 20%, 35%).For instance, if we have a numeric attribute, for example personal assets that contains 10,000 instances ranging from $50,000,000 to $50, for a 2-class label problem (sad, happy) we find the median value 5000th e.g. ($50,000). If we say that everyone below the median value (poor) is sad and everyone above the median value (rich) is happy, then anyone who is poor but happy and rich but sad contravenes our hypothesis. To illustrate this point further, consider the dataset of 10 instances in Table 1.Table 1. 0% error overlap dataset with 10 instancesValue 2 4 6 8 10 12 14 16 18 20Class small small small small small large large large large Large Intuitively, we can see that any sensible discretization method should recognize that a partition should be placed between values 10 and 12 as the class label changes from small to large. If all datasets were this simple our work would be done. However if we swap the two middle class labels (Table 2) there is some confusion as to where we should place partitions.Table 2. 20% error overlap dataset with 20 instancesAttribute 2 4 6 8 10 12 14 16 18 20Class small small small small large small large large large large Given that the middle 2 out of 10 values in the dataset are in dispute, we have used the phrase ‘error overlap’ to represent the level of uncertainty deliberately placed into the class labels when forming each class. Hence, the error overlap for Table 2 is 20% or 2/10. Figure 3 demonstrates as personal assets increase, at some point there is a change of mood from sad to happy, with the error overlap represented by the intersection of class 1 and class 2.Class 1 - sad Class 2 - happypersonal assetsFigure 1. 5% class error overlap (class 1 class 2) with 10,000 instancesTable 3 shows the number of errors built into the data (shaded rows) as the error overlap increases for a dataset of 10,000 instances.Table 3. Built in classification error rates2 class problem 1% 5% 10% 20% 35%Poor & sad 4950 4750 4500 4000 3250Poor & happy 50 250 500 1000 1750Rich & sad 50 250 500 1000 1750Rich & happy 4950 4750 4500 4000 3250where Poor personal assets < $50,000Rich personal assets > $50,000For 3 and 5 class label attributes a similar methodology was followed to build in an error rate into the data. For instance, 3 classes in the above case would result in class labels of: sad, content, happy and for a 5 class problem: very sad, sad, content, happy, very happy. 2. Data DistributionsFigure 2. Normal Figure 3. Uniform Figure 4. Leptokurtic Figure 5. PlatykurticFigure 6. Bimodal Figure 7. Skewed Figure 8. Exponential Figure 9. ZipfMany datasets conform to a Normal Distribution as depicted in Figure 2. They are characterized by a symmetrical bell shaped curve with a single peak at the median value which is also approximately equal the mean value. The normal probability distribution sets a benchmark for center, spread, skewness, and kurtosis that other shapes/distributions are measured. The probability density function used to generate a normal distribution is given in [10].Figure 3 shows a randomly generated Uniform Distribution. Uniform distributions are characterized by a roughly equal frequency count across the data range as every value has the same probability of occurrence.Figure 4 shows a Leptokurtic Distribution. This distribution is similar to a normal distribution however, the central area of the curve is more pronounced with a higher peak. Thus, more data values are concentrated close to the mean and median value. A co-efficient of kurtosis of magnitude greater than 3 formally recognizes a data’s distribution as being leptokurtic [10].The Platykurtic Distribution is the opposite of a leptokurtic distribution; it is characterized by a flatter than normal central peak and as such a more evenly symmetrical distribution of values across the data range as exhibited in Figure 5. Formally, if the coefficient of kurtosis is significantly less than three, it is regarded as platykurtic. Note, a uniform distribution is a special case of a platykurtic distribution.A Bimodal Distribution is a distribution that contains two distinct peaks or modes. Unlike the previous distributions, the data can become asymmetric. Two distinct peaks are prevalent in Figure 6, either can be regarded as the mode.In the real world, many datasets will not exhibit the symmetry of a normal distribution. Very often there will be a greater proportion of values either side of the mean or the distance between the mean and the extreme values either side of the mean differ. When this occurs, we are said to have skewed data. Formally, if the coefficient of skewness is significantly greater/less than zero, we have a skewed distribution [10]. Figure 7 depicts a positively Skewed Distribution characterized by a long right tail.Exponential Distributions are often used to model expectancy and lifetimes such as the life expectancy of humans, car engines, or light bulbs as depicted in Figure 8.A Zipf Distribution follows a straight line when frequency and rank are plotted on a double-logarithmic scale, highlighting the inverse correlation between ordered frequencies. An example of data that exhibits this pattern is the frequency of words in a book. Words such as ‘the’ and ’and’ have very high frequency counts in most long text files, whilst other words such as ‘alleviate’ occur rarely. As a Zipf distribution, all the words would be sorted into frequency order where each spike in the histogram represents a word. Figure 9 depicts an approximated Zipf distribution where there are many items with few occurrences and few items with many occurrences.4. Discretization Techniques4.1 Unsupervised DiscretizationThe simplest means of discretizing continuous features involves a class blind approach where only knowledge of the feature to be discretized is required. Here the user specifies the number of intervals or bins.4.1.1 Equal Interval Width/Uniform BinningThis method relies on sorting the data and dividing the data values into equally spaced bin ranges. A seed k supplied by the user determines how many bins are required. With this seed k, it is just a matter of finding the maximum and minimum values to derive the range and then partition the data into k bins.4.1.2 Equal Frequency/Histogram BinningPartitioning of data is based on allocating the same number of instances to each bin. Dividing the total number of instances n by k the number of bins supplied by the user does this.4.2 Supervised DiscretizationSupervised discretization on the other hand makes use of the instance/class labels during the discretization process to aid in the partitioning. Prior knowledge of the class label of each instance is incorporated at each iteration to refine partition breakpoint estimation of each bin.4.2.1 Holte’s 1R DiscretizerThis method uses an error-based approach using error counts to determine when to split intervals [4]. The attribute is sorted into ascending order and a greedy algorithm that divides the feature into bins where each contains only one instance of a particular class label is used. The danger inherent in such a technique is that each instance may end up belonging to a separate bin. To combat this problem, a minimum number of instances of a particular class for each bin as specified by the user (except for the upper most bin) is implemented. Hence any given bin can now contain a mixture of class labels andboundaries will not be continually divided leading to overfitting. Each bucket grows i.e. the partition shifts to the right until it has at least 6 instances of a class label, and continues until the instance to be considered is not part of the majority class label. Empirical analysis [4] suggests a minimum bin size of 6 performs the best.4.2.2 C4.5 DiscretizerC4.5 uses a bottom up approach that builds a complete tree and then prunes the number of intervals [8]. Information gain theory is used to determine at which threshold value the gain ratio is greatest in order to partition the data. A divide and conquer algorithm is then successively applied to determine whether to split each partition into smaller subsets at each iteration. Every non-leaf sub tree is examined from the bottom and if the predicted error is lower if a leaf replaces the sub tree, then a leaf replaces the sub tree.4.2.3 Fayyad and Irani’s Entropy Based MDL MethodThis method uses a top down approach whereby multiple ranges rather than binary ranges are created to form a tree via multi-way splits of the numeric attribute at the same node to produce discrete bins. Determining the exact cut off points for intervals is based on the Minimum Description Length Principle (MDLP) that biases a simpler theory that can explain the same body of data and favors a hypothesis which minimizes the probability of making a wrong decision assuming a uniform error cost. No pruning is applied to the grown tree.An information entropy/uncertainty minimization heuristic is used to select threshold boundaries by finding a single threshold that minimizes the entropy function over all possible thresholds [3]. This entropy function is then recursively applied to both of the partitions induced. Thresholds are placed half way between the two delimiting instances. At this point the MDL stopping criterion is applied to determine when to stop subdividing discrete intervals.4.2.4 Kononenko’s Entropy Based MDL MethodThis method is virtually identical to that of Fayyad and Irani except that it includes an adjustment for when multiple attributes are to be discretized. This algorithm provides a correction for the bias the entropy measure has towards an attribute with many values.5. Running Algorithms in WEKAThe dataset generated contained 8 attributes representing each data distribution. There are 15 class columns: 5 classes for the simplest 2 class problem with either a 1%, 5%, 10%, 20% or 35% error rate built into the class labels; and similarly 5 classes for a 3 class label and 5 class label problem. Each discretization method was tested with 1 attribute and 1 class column run together via the C4.5 classifier using 10-fold cross-validation. Each distribution was then analyzed by filtering out the appropriate columns with all 720 combinations run, (i.e. 6 discretization methods * 8 attributes * 15 classes).Equal width binning was run with the number of bins used set at the default setting of 10 bins. No attempt was made to optimize this value. The C4.5 algorithm was then applied to the discretized data to produce a tree. This classifier was chosen over a Bayesianclassifier as there are no normality constraints on the data required, especially relevant given the focus of the experiments.Equal frequency binning was run with the number of bins used set at the defaultsetting of 10 bins. The C4.5 algorithm was then applied to the discretized data to produce a tree. Holte’s 1R Discretizer was run using a default minimum bucket size set at the default setting of 6. C4.5 Discretizer was run using a confidence factor set to 0.25 as per the default value. Fayyad and Irani’s Entropy Based MDL Method was achieved using WEKA 3-2-3 with the use MDL parameter set to true. The C4.5 algorithm was used as the wrapper function. Kononenko’s Entropy Based MDL Method was achieved by setting the use MDL parameter to false. The C4.5 algorithm was used as the wrapper function.6. Analysis of ErrorsIn most practical situations, the best numerical prediction method is still the best no matter which error measure is used [11]. The root relative squared error was used as the basis for comparison. This measure gives extra emphasis to outliers and larger discrepancies are weighed more heavily than smaller ones. Further, it was able to detect subtle differences in the various error rates of each method, especially when the total error count or confusion matrix was the same. This measure is given by:r.r.s.e =(p 1 – a 1)2 + .. + (p n – a n )2 where p = predicted value, a = actual value, (a 1 – )2 + .. + (a n – )= 1/n i a i average value of a i .7. ResultsFigure 10. Normal dist. errors for 2-class labels Figure 11. Skewed dist. errors for 2-class labelsFigure 12. Unsupervised(Equal-width) vs. Supervised(Fayyad&Irani) average errors (Y-axis) for Exponentialand Uniform distributions plotted against number of class labels (X-axis) and error overlap (Z-axis)Figure 13. Average root relative squared error for all distributions and discretization methods7.1 Varying the Error OverlapFigure 10 plots the root relative squared error for each discretization method and error overlap for the 2-class label problem for a normally distributed continuous attribute. The first two unsupervised methods progressively deteriorate in predictive performance as the inherent error overlap is increased. On the other hand, the other methods have a stable performance as the error overlap is increased. Surprisingly, the MDL methods perform worse with an error overlap of 1% compared to when the error overlap is 35%. In contrast, Figure 11 shows a degenerative performance for the skewed distribution when the error overlap was increased, regardless of the discretization method used.7.2 Varying the Number of Class LabelsThe effect of increasing the number of class labels can be seen in Figure 11 where an example of the exponential and uniform distribution errors are highlighted when run with both an unsupervised (equal-width) and supervised method (Fayyad&Irani). When the number of class labels is increased from 2 to 3 and 5, the degenerative performance of the unsupervised methods becomes more pronounced. From Figure 12, the error rate rises sharply for both the exponential and uniform distributions. This pattern was replicated across the board for all distributions when unsupervised discretization was applied. The supervised methods provided mixed outcomes when the number of class labels increased. In Figure 12 we can see that the uniform distribution errors remained static for supervised discretization using Fayyad&Irani method even when the number of class labels increased. Only, the normal distribution followed this pattern (not shown). All other distributions followed a growth in the error rate similar to that shown for the supervised exponential errors in Figure 12.7.3 Comparison of Overall Error RatesFigure 13 shows a comparison of the performance of each discretization method as applied to each data distribution. Each bar represents the average root relative squared error bysumming across all overlaps and number of class labels and dividing by 15. The equal-interval binning technique appears to be more effective when the data distribution is flatter, as depicted by a uniform or platykurtic distribution. This concurs with the findings in [2] that this method of discretization is vulnerable to skewed data. Interestingly, the error rate of equal-interval binning converges to that of equal-frequency binning with flatter distributions as depicted in Figure 13. Equal interval binning often distributes instances unevenly across bins with some bins containing many instances and others possibly containing none. Figure 13 demonstrates the degenerative performance of equal-interval binning when the distribution being discretized is less even. It is thus no surprise that the leptokurtic and the zipf distribution are the worse performers applying this method, and the best performers being those distributions with a more even distribution, i.e. uniform and platykurtic distributions.Similarly, equal frequency binning can suffer from serious problems. Consider the event that we have 1000 instances and 10 intervals specified by the user. If there are 105 instances with a value between 10 and 20 corresponding to bin 2, then the remaining 5 instances are grouped into the next bin. This sort of occurrence undermines knowledge discovery in the sense that partitions then lose all semantic value. On the whole, the equal-frequency binning method shows relative indifference to the distribution of the data.The OneR discretizer works well with both a normal and uniform distribution. The OneR error rate is roughly 20% higher for all other distributions relative to the MDL methods. The problem with an error based method like OneR is that it may result in splitting intervals unnecessarily to minimize the error count and consequently blurs the understanding of classification results. Furthermore, multiple attributes that require discretization cannot be used with this algorithm.The C4.5 discretizer was the equal best performer for the normal and uniform distribution. This concurs with [5] who showed that discretization causes a small increase in error rate when the continuous feature is normally distributed. However, it was nearly 10% worse than the MDL entropy methods for the other six distributions. One factor that impacts on the overall tree generated is the confidence level. The default confidence parameter of 0.25 was used. Varying this value changes the level of pruning applied to the tree. The smaller this parameter the greater the level of pruning that occurs. It is a balancing act to prevent overfitting whilst trying to optimize this parameter in the context of knowledge discovery [5].The entropy based MDL methods combine both local and global information during learning, i.e. they use a wrapper function i.e. C4.5 in this case with the MDL criterion (global). The MDL pre-selects cut-points and the classifier decides which cut-point is the most appropriate in the current context. Using a global discretization as opposed to a local method (C4.5) makes the MDL methods less sensitive to variation from small fragmented data [2]. These methods were consistently high performers across all data distributions as depicted in Figure 13.7.4 Selection of the Number of BinsIn the experiments k bins set to the default value of ten was used. The choice of k has a major effect on error rates [6]. Identifying the best value of k to use is a trial and error method and cannot be applied universally to each attribute. In some cases, error rates may even compare favourably to supervised discretization, however, finding the optimum cut off thresholds by supervised discretization may involve 2an calculations where a is the number of attributes and n is the number of instances [7]. The unpredictability ofunsupervised methods as shown in the overall summary error rates for all distributions seems to make them a poor choice for discretization.Figure 14. Error rate for number of bins manually chosen for equal-width binning strategy for normaldistribution of 2 class problem with 35% error overlap.Figure 14 depicts the effect of the error rate on classification when the seed for an unsupervised method is varied. Here the seed represents a manually chosen number by the user that is the number of bins requested from the data. Figure 14 shows that the default value of 10 bins (used in all runs) is not the best selection of the number of bins in order to produce the smallest error rate in this instance but may be in others.7.5 Identifying a HeuristicInitial thoughts were that error rates were correlated positively with the level of kurtosis or skewness. The correlation coefficient for kurtosis against the root relative squared error was 0.45, indicating that there appears to be a significant relationship as shown in Figure 15. The relationship between skewness and the error rate as depicted in Figure 16 was less pronounced with the correlation coefficient at 0.22. Prudence is taken with influence regarding the size of the coefficients given the error measure used and the assumption of a linear relationship via a linear regression model. In general terms, we can deduce a positive relationship between the elevation (kurtosis) and symmetry (skewness) of the data and classification errors resulting from discretization. However, a word of caution is that discretization methods make a poor job of separating the two underlying populations in a variable that has a bimodal distribution [9].Figure 15. Kurtosis vs. average error rate : r2 = 0.45 Figure 16. Skewness vs. average error rate : r2 = 0.22 Via closer inspection of the shape of each distribution, it seems that distributions that have either:• a long thin tail (skewed, zipf and exponential distributions) or,• a high peak/concentration of data (leptokurtic, bimodal distribution)benefit the least from discretization. Similarly, these distributions gain the least from using a supervised method compared to an unsupervised method of discretization. For instance, the root relative squared error rate can be improved by 86% for a normal or uniform distribution, compared to 19% for a skewed distribution and 25% for a bimodal distribution (Fig.13).8. ConclusionFrom analysis the 720 runs it was found the distributions that benefit the most from discretization in ranked order are: (1) uniform, (2) normal, (3) platykurtic, (4) bimodal, (5) exponential, (6) zipf, (7) leptokurtic, (8) skewed.The higher ranked distributions, namely the uniform and normal distributions exhibit escalating error rates for the unsupervised discretization methods when the error overlap and/or the number of classes labels increases. These unsupervised methods were shown to be highly susceptible to an arbitrarily selected number of bins. In contrast, the error rates for the supervised discretization methods remained constant as both the error overlap and/or the number of class labels introduced increased.At the lower end of the ranked distributions, the classification error rates grew as the error overlap grew as expected, regardless of which discretization method was adopted. Unexpectedly, as more class labels were introduced, error rates increased at lower error overlap levels whilst remaining relatively static at the higher end of the error overlap level.In summary, we have identified a heuristic that is able to determine the relative effectiveness of discretization apriori by data visualization, and by establishing that a positive correlation between the level of kurtosis and skewness and the error rate from classification in the data distributions exists, with a caveat for bimodal distributions.In this work we examined classification errors based on a single discretized attribute. In future work we will look at combinations of discretized attributes.References1. Catlett, J. (1991b) “On Changing Continuous Attributes into Ordered Discrete Attributes”, InY.Kodrtoff, ed., EWSL-91. Lecture Notes in Artificial Intelligence 482, pp.164-178. Springer-Verlag, Berlin, Germany.2. Dougherty, J., Kohavi, R., Sahami, M. (1995) “Supervised and Unsupervised Discretization ofContinuous Features” Machine Learning: Proceedings of the 12th International Conference, Morgan Kaufmann, Los Altos, CA.3. Fayyad, U.M., Irani, K.B. (1993) “Multi-interval discretization of continuous valued attributes forclassification learning” In Proceedings of the 13th International Joint Conference on Artificial Intelligence, pp.1022-1027, Los Altos, CA: Morgan Kaufmann.4. Holte, R.C. (1993) “Very simple classification rules perform well on most commonly used datasets”5. Machine Learning 11, pp.63-90.6. Kohavi, R., Sahami, M. (1996) “Error-based and Entropy-based Discretization of ContinuousFeatures” In Proceedings of the 2nd International Conference on Knowledge Discovery and Data Mining, pp.114-119. Menlo Park:AAAI Press.7. Kononenko, I., Sikonja, M.R, (1995) “Discretization of continuous attributes using ReliefF”Proceedings of ERK'95 , Portoroz, Slovenia, 1995.8. Pazzani, M. (1995) “An iterative improvement approach for the discretization of numeric attributesin Bayesian classifiers” KDD-95 pp.228-2339. Quinlan, J.R. (1993) “C4.5 : Programs for Machine Learning”, Morgan Kaufmann, Los Altos, CA10. Scott, P.D., Williams, R.J., Ho, K.M. (1997) “Forming Categories in Exploratory Data Analysis andData Mining”, IDA 1997, pp.235-246.11. Smith, P.J. (1997) “Into Statistics” Springer-verlag, pp.205-206, 304-306, 323-329.12. Witten I., Frank, E. (2000) “Data Mining: Practical Machine Learning Tools and Techniques”Morgan Kaufmann, pp.80-82, 147-150, 238-246.。