Indirect Adaptive Robust Control of Electro-Hydraulic Systems Driven by Single-Rod Hydrauli
国际自动化与计算杂志.英文版.
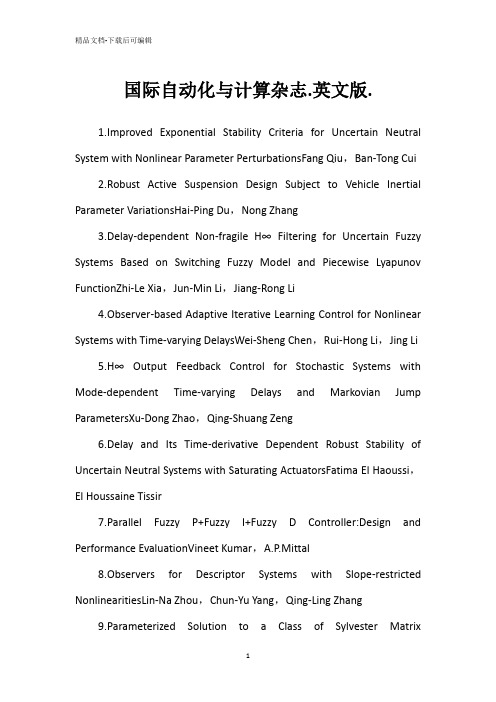
国际自动化与计算杂志.英文版.1.Improved Exponential Stability Criteria for Uncertain Neutral System with Nonlinear Parameter PerturbationsFang Qiu,Ban-Tong Cui2.Robust Active Suspension Design Subject to Vehicle Inertial Parameter VariationsHai-Ping Du,Nong Zhang3.Delay-dependent Non-fragile H∞ Filtering for Uncertain Fuzzy Systems Based on Switching Fuzzy Model and Piecewise Lyapunov FunctionZhi-Le Xia,Jun-Min Li,Jiang-Rong Li4.Observer-based Adaptive Iterative Learning Control for Nonlinear Systems with Time-varying DelaysWei-Sheng Chen,Rui-Hong Li,Jing Li5.H∞ Output Feedback Control for Stochastic Systems with Mode-dependent Time-varying Delays and Markovian Jump ParametersXu-Dong Zhao,Qing-Shuang Zeng6.Delay and Its Time-derivative Dependent Robust Stability of Uncertain Neutral Systems with Saturating ActuatorsFatima El Haoussi,El Houssaine Tissir7.Parallel Fuzzy P+Fuzzy I+Fuzzy D Controller:Design and Performance EvaluationVineet Kumar,A.P.Mittal8.Observers for Descriptor Systems with Slope-restricted NonlinearitiesLin-Na Zhou,Chun-Yu Yang,Qing-Ling Zhang9.Parameterized Solution to a Class of Sylvester MatrixEquationsYu-Peng Qiao,Hong-Sheng Qi,Dai-Zhan Cheng10.Indirect Adaptive Fuzzy and Impulsive Control of Nonlinear SystemsHai-Bo Jiang11.Robust Fuzzy Tracking Control for Nonlinear Networked Control Systems with Integral Quadratic ConstraintsZhi-Sheng Chen,Yong He,Min Wu12.A Power-and Coverage-aware Clustering Scheme for Wireless Sensor NetworksLiang Xue,Xin-Ping Guan,Zhi-Xin Liu,Qing-Chao Zheng13.Guaranteed Cost Active Fault-tolerant Control of Networked Control System with Packet Dropout and Transmission DelayXiao-Yuan Luo,Mei-Jie Shang,Cai-Lian Chen,Xin-Ping Guanparison of Two Novel MRAS Based Strategies for Identifying Parameters in Permanent Magnet Synchronous MotorsKan Liu,Qiao Zhang,Zi-Qiang Zhu,Jing Zhang,An-Wen Shen,Paul Stewart15.Modeling and Analysis of Scheduling for Distributed Real-time Embedded SystemsHai-Tao Zhang,Gui-Fang Wu16.Passive Steganalysis Based on Higher Order Image Statistics of Curvelet TransformS.Geetha,Siva S.Sivatha Sindhu,N.Kamaraj17.Movement Invariants-based Algorithm for Medical Image Tilt CorrectionMei-Sen Pan,Jing-Tian Tang,Xiao-Li Yang18.Target Tracking and Obstacle Avoidance for Multi-agent SystemsJing Yan,Xin-Ping Guan,Fu-Xiao Tan19.Automatic Generation of Optimally Rigid Formations Using Decentralized MethodsRui Ren,Yu-Yan Zhang,Xiao-Yuan Luo,Shao-Bao Li20.Semi-blind Adaptive Beamforming for High-throughput Quadrature Amplitude Modulation SystemsSheng Chen,Wang Yao,Lajos Hanzo21.Throughput Analysis of IEEE 802.11 Multirate WLANs with Collision Aware Rate Adaptation AlgorithmDhanasekaran Senthilkumar,A. Krishnan22.Innovative Product Design Based on Customer Requirement Weight Calculation ModelChen-Guang Guo,Yong-Xian Liu,Shou-Ming Hou,Wei Wang23.A Service Composition Approach Based on Sequence Mining for Migrating E-learning Legacy System to SOAZhuo Zhang,Dong-Dai Zhou,Hong-Ji Yang,Shao-Chun Zhong24.Modeling of Agile Intelligent Manufacturing-oriented Production Scheduling SystemZhong-Qi Sheng,Chang-Ping Tang,Ci-Xing Lv25.Estimation of Reliability and Cost Relationship for Architecture-based SoftwareHui Guan,Wei-Ru Chen,Ning Huang,Hong-Ji Yang1.A Computer-aided Design System for Framed-mould in Autoclave ProcessingTian-Guo Jin,Feng-Yang Bi2.Wear State Recognition of Drills Based on K-means Cluster and Radial Basis Function Neural NetworkXu Yang3.The Knee Joint Design and Control of Above-knee Intelligent Bionic Leg Based on Magneto-rheological DamperHua-Long Xie,Ze-Zhong Liang,Fei Li,Li-Xin Guo4.Modeling of Pneumatic Muscle with Shape Memory Alloy and Braided SleeveBin-Rui Wang,Ying-Lian Jin,Dong Wei5.Extended Object Model for Product Configuration DesignZhi-Wei Xu,Ze-Zhong Liang,Zhong-Qi Sheng6.Analysis of Sheet Metal Extrusion Process Using Finite Element MethodXin-Cun Zhuang,Hua Xiang,Zhen Zhao7.Implementation of Enterprises' Interoperation Based on OntologyXiao-Feng Di,Yu-Shun Fan8.Path Planning Approach in Unknown EnvironmentTing-Kai Wang,Quan Dang,Pei-Yuan Pan9.Sliding Mode Variable Structure Control for Visual Servoing SystemFei Li,Hua-Long Xie10.Correlation of Direct Piezoelectric Effect on EAPap under Ambient FactorsLi-Jie Zhao,Chang-Ping Tang,Peng Gong11.XML-based Data Processing in Network Supported Collaborative DesignQi Wang,Zhong-Wei Ren,Zhong-Feng Guo12.Production Management Modelling Based on MASLi He,Zheng-Hao Wang,Ke-Long Zhang13.Experimental Tests of Autonomous Ground Vehicles with PreviewCunjia Liu,Wen-Hua Chen,John Andrews14.Modelling and Remote Control of an ExcavatorYang Liu,Mohammad Shahidul Hasan,Hong-Nian Yu15.TOPSIS with Belief Structure for Group Belief Multiple Criteria Decision MakingJiang Jiang,Ying-Wu Chen,Da-Wei Tang,Yu-Wang Chen16.Video Analysis Based on Volumetric Event DetectionJing Wang,Zhi-Jie Xu17.Improving Decision Tree Performance by Exception HandlingAppavu Alias Balamurugan Subramanian,S.Pramala,B.Rajalakshmi,Ramasamy Rajaram18.Robustness Analysis of Discrete-time Indirect Model Reference Adaptive Control with Normalized Adaptive LawsQing-Zheng Gao,Xue-Jun Xie19.A Novel Lifecycle Model for Web-based Application Development in Small and Medium EnterprisesWei Huang,Ru Li,Carsten Maple,Hong-Ji Yang,David Foskett,Vince Cleaver20.Design of a Two-dimensional Recursive Filter Using the Bees AlgorithmD. T. Pham,Ebubekir Ko(c)21.Designing Genetic Regulatory Networks Using Fuzzy Petri Nets ApproachRaed I. Hamed,Syed I. Ahson,Rafat Parveen1.State of the Art and Emerging Trends in Operations and Maintenance of Offshore Oil and Gas Production Facilities: Some Experiences and ObservationsJayantha P.Liyanage2.Statistical Safety Analysis of Maintenance Management Process of Excavator UnitsLjubisa Papic,Milorad Pantelic,Joseph Aronov,Ajit Kumar Verma3.Improving Energy and Power Efficiency Using NComputing and Approaches for Predicting Reliability of Complex Computing SystemsHoang Pham,Hoang Pham Jr.4.Running Temperature and Mechanical Stability of Grease as Maintenance Parameters of Railway BearingsJan Lundberg,Aditya Parida,Peter S(o)derholm5.Subsea Maintenance Service Delivery: Mapping Factors Influencing Scheduled Service DurationEfosa Emmanuel Uyiomendo,Tore Markeset6.A Systemic Approach to Integrated E-maintenance of Large Engineering PlantsAjit Kumar Verma,A.Srividya,P.G.Ramesh7.Authentication and Access Control in RFID Based Logistics-customs Clearance Service PlatformHui-Fang Deng,Wen Deng,Han Li,Hong-Ji Yang8.Evolutionary Trajectory Planning for an Industrial RobotR.Saravanan,S.Ramabalan,C.Balamurugan,A.Subash9.Improved Exponential Stability Criteria for Recurrent Neural Networks with Time-varying Discrete and Distributed DelaysYuan-Yuan Wu,Tao Li,Yu-Qiang Wu10.An Improved Approach to Delay-dependent Robust Stabilization for Uncertain Singular Time-delay SystemsXin Sun,Qing-Ling Zhang,Chun-Yu Yang,Zhan Su,Yong-Yun Shao11.Robust Stability of Nonlinear Plants with a Non-symmetric Prandtl-Ishlinskii Hysteresis ModelChang-An Jiang,Ming-Cong Deng,Akira Inoue12.Stability Analysis of Discrete-time Systems with Additive Time-varying DelaysXian-Ming Tang,Jin-Shou Yu13.Delay-dependent Stability Analysis for Markovian Jump Systems with Interval Time-varying-delaysXu-Dong Zhao,Qing-Shuang Zeng14.H∞ Synchronization of Chaotic Systems via Delayed Feedback ControlLi Sheng,Hui-Zhong Yang15.Adaptive Fuzzy Observer Backstepping Control for a Class of Uncertain Nonlinear Systems with Unknown Time-delayShao-Cheng Tong,Ning Sheng16.Simulation-based Optimal Design of α-β-γ-δ FilterChun-Mu Wu,Paul P.Lin,Zhen-Yu Han,Shu-Rong Li17.Independent Cycle Time Assignment for Min-max SystemsWen-De Chen,Yue-Gang Tao,Hong-Nian Yu1.An Assessment Tool for Land Reuse with Artificial Intelligence MethodDieter D. Genske,Dongbin Huang,Ariane Ruff2.Interpolation of Images Using Discrete Wavelet Transform to Simulate Image Resizing as in Human VisionRohini S. Asamwar,Kishor M. Bhurchandi,Abhay S. Gandhi3.Watermarking of Digital Images in Frequency DomainSami E. I. Baba,Lala Z. Krikor,Thawar Arif,Zyad Shaaban4.An Effective Image Retrieval Mechanism Using Family-based Spatial Consistency Filtration with Object RegionJing Sun,Ying-Jie Xing5.Robust Object Tracking under Appearance Change ConditionsQi-Cong Wang,Yuan-Hao Gong,Chen-Hui Yang,Cui-Hua Li6.A Visual Attention Model for Robot Object TrackingJin-Kui Chu,Rong-Hua Li,Qing-Ying Li,Hong-Qing Wang7.SVM-based Identification and Un-calibrated Visual Servoing for Micro-manipulationXin-Han Huang,Xiang-Jin Zeng,Min Wang8.Action Control of Soccer Robots Based on Simulated Human IntelligenceTie-Jun Li,Gui-Qiang Chen,Gui-Fang Shao9.Emotional Gait Generation for a Humanoid RobotLun Xie,Zhi-Liang Wang,Wei Wang,Guo-Chen Yu10.Cultural Algorithm for Minimization of Binary Decision Diagram and Its Application in Crosstalk Fault DetectionZhong-Liang Pan,Ling Chen,Guang-Zhao Zhang11.A Novel Fuzzy Direct Torque Control System for Three-level Inverter-fed Induction MachineShu-Xi Liu,Ming-Yu Wang,Yu-Guang Chen,Shan Li12.Statistic Learning-based Defect Detection for Twill FabricsLi-Wei Han,De Xu13.Nonsaturation Throughput Enhancement of IEEE 802.11b Distributed Coordination Function for Heterogeneous Traffic under Noisy EnvironmentDhanasekaran Senthilkumar,A. Krishnan14.Structure and Dynamics of Artificial Regulatory Networks Evolved by Segmental Duplication and Divergence ModelXiang-Hong Lin,Tian-Wen Zhang15.Random Fuzzy Chance-constrained Programming Based on Adaptive Chaos Quantum Honey Bee Algorithm and Robustness AnalysisHan Xue,Xun Li,Hong-Xu Ma16.A Bit-level Text Compression Scheme Based on the ACW AlgorithmHussein A1-Bahadili,Shakir M. Hussain17.A Note on an Economic Lot-sizing Problem with Perishable Inventory and Economies of Scale Costs:Approximation Solutions and Worst Case AnalysisQing-Guo Bai,Yu-Zhong Zhang,Guang-Long Dong1.Virtual Reality: A State-of-the-Art SurveyNing-Ning Zhou,Yu-Long Deng2.Real-time Virtual Environment Signal Extraction and DenoisingUsing Programmable Graphics HardwareYang Su,Zhi-Jie Xu,Xiang-Qian Jiang3.Effective Virtual Reality Based Building Navigation Using Dynamic Loading and Path OptimizationQing-Jin Peng,Xiu-Mei Kang,Ting-Ting Zhao4.The Skin Deformation of a 3D Virtual HumanXiao-Jing Zhou,Zheng-Xu Zhao5.Technology for Simulating Crowd Evacuation BehaviorsWen-Hu Qin,Guo-Hui Su,Xiao-Na Li6.Research on Modelling Digital Paper-cut PreservationXiao-Fen Wang,Ying-Rui Liu,Wen-Sheng Zhang7.On Problems of Multicomponent System Maintenance ModellingTomasz Nowakowski,Sylwia Werbinka8.Soft Sensing Modelling Based on Optimal Selection of Secondary Variables and Its ApplicationQi Li,Cheng Shao9.Adaptive Fuzzy Dynamic Surface Control for Uncertain Nonlinear SystemsXiao-Yuan Luo,Zhi-Hao Zhu,Xin-Ping Guan10.Output Feedback for Stochastic Nonlinear Systems with Unmeasurable Inverse DynamicsXin Yu,Na Duan11.Kalman Filtering with Partial Markovian Packet LossesBao-Feng Wang,Ge Guo12.A Modified Projection Method for Linear FeasibilityProblemsYi-Ju Wang,Hong-Yu Zhang13.A Neuro-genetic Based Short-term Forecasting Framework for Network Intrusion Prediction SystemSiva S. Sivatha Sindhu,S. Geetha,M. Marikannan,A. Kannan14.New Delay-dependent Global Asymptotic Stability Condition for Hopfield Neural Networks with Time-varying DelaysGuang-Deng Zong,Jia Liu hHTTp://15.Crosscumulants Based Approaches for the Structure Identification of Volterra ModelsHouda Mathlouthi,Kamel Abederrahim,Faouzi Msahli,Gerard Favier1.Coalition Formation in Weighted Simple-majority Games under Proportional Payoff Allocation RulesZhi-Gang Cao,Xiao-Guang Yang2.Stability Analysis for Recurrent Neural Networks with Time-varying DelayYuan-Yuan Wu,Yu-Qiang Wu3.A New Type of Solution Method for the Generalized Linear Complementarity Problem over a Polyhedral ConeHong-Chun Sun,Yan-Liang Dong4.An Improved Control Algorithm for High-order Nonlinear Systems with Unmodelled DynamicsNa Duan,Fu-Nian Hu,Xin Yu5.Controller Design of High Order Nonholonomic System with Nonlinear DriftsXiu-Yun Zheng,Yu-Qiang Wu6.Directional Filter for SAR Images Based on NonsubsampledContourlet Transform and Immune Clonal SelectionXiao-Hui Yang,Li-Cheng Jiao,Deng-Feng Li7.Text Extraction and Enhancement of Binary Images Using Cellular AutomataG. Sahoo,Tapas Kumar,B.L. Rains,C.M. Bhatia8.GH2 Control for Uncertain Discrete-time-delay Fuzzy Systems Based on a Switching Fuzzy Model and Piecewise Lyapunov FunctionZhi-Le Xia,Jun-Min Li9.A New Energy Optimal Control Scheme for a Separately Excited DC Motor Based Incremental Motion DriveMilan A.Sheta,Vivek Agarwal,Paluri S.V.Nataraj10.Nonlinear Backstepping Ship Course ControllerAnna Witkowska,Roman Smierzchalski11.A New Method of Embedded Fourth Order with Four Stages to Study Raster CNN SimulationR. Ponalagusamy,S. Senthilkumar12.A Minimum-energy Path-preserving Topology Control Algorithm for Wireless Sensor NetworksJin-Zhao Lin,Xian Zhou,Yun Li13.Synchronization and Exponential Estimates of Complex Networks with Mixed Time-varying Coupling DelaysYang Dai,YunZe Cai,Xiao-Ming Xu14.Step-coordination Algorithm of Traffic Control Based on Multi-agent SystemHai-Tao Zhang,Fang Yu,Wen Li15.A Research of the Employment Problem on Common Job-seekersand GraduatesBai-Da Qu。
Adaptive Trajectory Tracking Control of Skid-Steered Mobile Robots
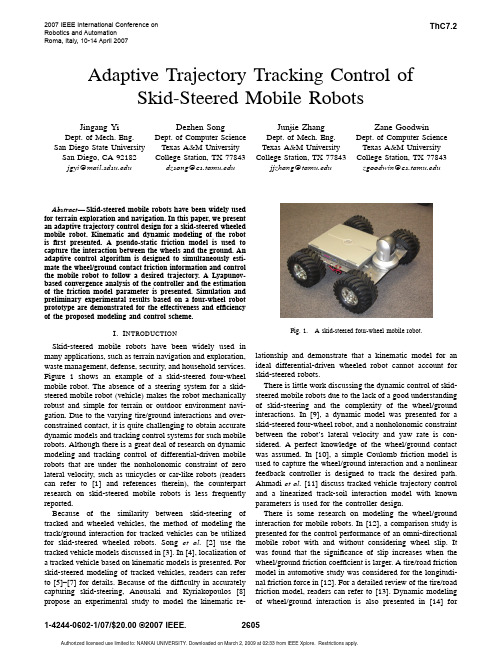
1-4244-0602-1/07/$20.00 ©2007 IEEE.
2605
Authorized licensed use limited to: NANKAI UNIVERSITY. Downloaded on March 2, 2009 at 02:33 from IEEE Xplore. Restrictions apply.
I. I NTRODUCTION Skid-steered mobile robots have been widely used in many applications, such as terrain navigation and exploration, waste management, defense, security, and household services. Figure 1 shows an example of a skid-steered four-wheel mobile robot. The absence of a steering system for a skidsteered mobile robot (vehicle) makes the robot mechanically robust and simple for terrain or outdoor environment navigation. Due to the varying tire/ground interactions and overconstrained contact, it is quite challenging to obtain accurate dynamic models and tracking control systems for such mobile robots. Although there is a great deal of research on dynamic modeling and tracking control of differential-driven mobile robots that are under the nonholonomic constraint of zero lateral velocity, such as unicycles or car-like robots (readers can refer to [1] and references therein), the counterpart research on skid-steered mobile robots is less frequently reported. Because of the similarity between skid-steering of tracked and wheeled vehicles, the method of modeling the track/ground interaction for tracked vehicles can be utilized for skid-steered wheeled robots. Song et al. [2] use the tracked vehicle models discussed in [3]. In [4], localization of a tracked vehicle based on kinematic models is presented. For skid-steered modeling of tracked vehicles, readers can refer to [5]–[7] for details. Because of the difficulty in accurately capturing skid-steering, Anousaki and Kyriakopoulos [8] propose an experimental study to model the kinematic re-
基于不确定逼近的机械手间接自适应鲁棒预测控制

LI Gui.qiu 。CHEN Zhi.wang (1.Department ofInformation Engineering,Changzhou Institute of Mechatronic Technology,Changzhou Jiangsu 213164,China; 2.Key Laboratory o f Industrial Computer Control Engineering of Hebei Province,Yanshan University,Qinhuangdao Hebei 066004,China)
[2]Indirect Robust Adaptive Fault-Tolerant Control for Attitude
![[2]Indirect Robust Adaptive Fault-Tolerant Control for Attitude](https://img.taocdn.com/s3/m/2f40a2c50508763230121207.png)
Indirect Robust Adaptive Fault-Tolerant Control for AttitudeTracking of SpacecraftWenchuan Cai ∗North Carolina A&T State University,Greensboro,North Carolina 27411X.H.Liao †Volvo North American,Inc.,Greensboro,North Carolina 27455and Y.D.Song ‡North Carolina A&T State University,Greensboro,North Carolina 27411DOI:10.2514/1.31158Reliable and cost-effective control of spacecraft should account for modeling uncertainties,unexpected disturbances,subsystem failures,and limited resources simultaneously.This paper presents an indirect (nonregressor-based)approach to attitude tracking control of spacecraft.It is shown that the control algorithms developed are not only robust against external disturbances and adaptive to unknown and time-varying mass/inertia properties,but also able to accommodate actuator failures under limited thrusts.All are achieved with inexpensive online computations (a feature of practical importance in reducing the usage of onboard resources in terms of computing power and memory size).Furthermore,this method is user/designer friendly in that it does not involve a time-consuming design procedure and demands little redesigning or reprogramming during vehicle operation.The bene fits of the proposed control method are analytically authenticated and also validated via simulation study.I.IntroductionADVANCED space missions demand the development of effective spacecraft control systems to ensure rapid,accurate,and global response to various attitude maneuvering commands.Such a response should be achieved globally in the presence of modeling uncertainties,external disturbances,subsystem failures,and/or limited resources (energy,memory space,computing power,etc.).A signi ficant challenge arises when those issues are treated simultaneously.Although there are rich results on attitude control of spacecraft in the literature,such as nonlinear feedback control [1,2],variable structure control [3],optimal control [4,5],adaptive control,and robust control [6–11],very few have explicitly dealt with these issues concurrently.In this paper,we present a control scheme to achieve high precision attitude tracking of spacecraft with explicit consideration of uncertainties,disturbances,actuator failures,and limited resources (with particular attention to limited thrusts and cost-effective online computation).The main contributions of this work relative to others are as follows.Indirect (nonregressor-based)robust adaptive control algorithms are developed to achieve robustness against modeling uncertainties and unexpected disturbances.The proposed control scheme ensures stable attitude tracking with guaranteed performance (not just stabilization or rest-to-rest regulation).The method is also adaptive to unknown and time-varying mass/inertia property arising from varying mass distribution due to fuel usage and articulation.Unlike most other four-parameter quaternion-based methods,which demand somewhat heavy online computations [2,4,5,10–12],achieve angular velocity tracking only [13,14],or involve restrictive control parameters [8,15],the proposed method ensures both velocity and attitude tracking withsimple design procedures and inexpensive online computations,a feature of practical importance for real-time implementation especially when onboard memory space and computing power are limited.Furthermore,the proposed control scheme is fault tolerant to thruster failures under limited thrusts.The rest of the paper is organized as follows.In Sec.II,the idea of using indirect core information of the system uncertainties is described and the attitude tracking control problem of spacecraft is formulated using the unit quaternion to represent the attitude orientation.In Sec.III,we present the attitude tracking control algorithms and show their effectiveness in dealing with modeling uncertainties,external disturbances,actuator failures,and thrust saturation.Numerical simulations on various thruster failure situations are presented in Sec.IV to demonstrate the performance of the proposed control method.Finally,we conclude the paper in Sec.V.II.Attitude Dynamics and Problem FormulationThe spacecraft is modeled as a rigid body with actuators to provide torques along three mutually perpendicular axes that de fine a body-fixed frame B .The equations of motion in terms of kinematics and dynamics are given by (see [16],Chapter 4):J _!! J ! T d (1)_q 12 q q 0I !(2)_q 0 12q T !(3)where !2R 3is the angular velocity of the spacecraft with respect toan inertial frame I and expressed in body frame B ;I 2R 3 3is the identity matrix; q;q 0 2R 3 R denotes the unit quaternion representing the attitude orientation of the spacecraft in the body frame B with respect to the inertial frame I ,satisfying q T q q 20 1;J 2R 3 3is the inertia matrix of the spacecraft expressed in B ; 2R 3and T d 2R 3denote the control torques and the external disturbances respectively;and the operator ! denotes a skew-symmetric matrix acting on the vector ! !1!2!3 T and has the formReceived 20March 2007;revision received 16March 2008;accepted for publication 29March 2008.Copyright ©2008by Yongduan Song.Published by the American Institute of Aeronautics and Astronautics,Inc.,with permission.Copies of this paper may be made for personal or internal use,on condition that the copier pay the $10.00per-copy fee to the Copyright Clearance Center,Inc.,222Rosewood Drive,Danvers,MA 01923;include the code 0731-5090/08$10.00in correspondence with the CCC.∗Research Assistant,Center for Cooperative Systems.†System Design Engineer.‡Professor,Department of Electrical and Computer Engineering;also National Institute of Aerospace,100Exploration Way,Hampton,VA 23666.J OURNAL OF G UIDANCE ,C ONTROL ,AND D YNAMICS Vol.31,No.5,September –October 20081456D o w n l o a d e d b y X I 'A N J I A O T O N G U N I VE R S I T Y o n N o v e m b e r 17, 2013 | h t t p ://a r c .a i a a .o r g | D O I : 10.2514/1.31158! 0!3!2!30 !1 !2!12435(4)Remark 1:Note that during operation the mass properties of the spacecraft may be uncertain or may change due to onboard payload motion,rotation of solar arrays,or fuel consumptions,making J time varying and even uncertain;thus,control design should not use such J directly.On the other hand,although unknown and time varying,J remains positive de finite and bounded during the entire operation of the vehicle.It is therefore reasonable to assume that there exists a constant (unknown)c J 0such that k J kc J <1.Also,it is practical to assume that kd Jd t k c f <1for some unknown constant cf 0.To address the attitude tracking issue,we de fine the attitude tracking error q e ;q e 0 2R 3 R as the relative orientation between the body frame B and the desired frame D with orientation q d ;q d 0 2R 3 R ,which is computed asq e q d 0q q 0q d q q d(5)q e 0 q d 0q 0 q T d q(6)where q T d q d q 2d 0 1and q T e q e q 2e 0 1.Assume that !d ;q d 2R 3 R 3(desired angular velocity and attitude)are bounded suchthat k !d k c 1<1.k _!d k c 2<1for some unknown constants c 1 0and c 2 0.The rotation matrix is given by C q 2e 0 q T e q e I 2q e q T e 2q e 0q e (note that k C k 1and _C ! e C ).De fine the relative angular velocity !e 2R 3of B with respect to D as!e ! C!d(7)From Eqs.(1–7),it can be derived that [8,16]J _!e ! J ! T d J ! e C!d C _!d (8)_qe 1 q eq e 0I !e(9)_q e 0 1q T e !e(10)To develop the control scheme,we de fine the filtered error variables !e q e >0(11)where is a free design parameter chosen by the designer/user.Then,considering Eqs.(7)and (8),we haveJ _sL q e 0:5d Jd ts (12)withL ! J ! J ! C!d C!d C _!d T d 2J q e q e 0I !e q e 0:5d Jd ts (13)The reason for subtracting q e 0:5d Jd tfrom Eq.(12)and adding it to Eq.(13)is to facilitate the stability analysis,as will become clear later.Note that L is the lumped term containing two parts:1)system nonlinearities (depending on desired attitude trajectory and physical parameters,the moment inertia in particular),and 2)external disturbances (changing with operating conditions).One of the major challenges for attitude tracking control design stems from such an uncertain term in the dynamic equation.Different means of treating L lead to different control schemes.Model-based nonlinear control assumes that precise L is available for direct cancellation.Regressor-based adaptive and robust control is based on precise analytic structure of L to derive online updating algorithms for unknown but constant parameters in the system.In general,this method demands heavy computations and time-consuming design procedures,yet cannot deal with time-varying parameters [5–8].We explore an indirect (nonregressor-based)approach to dealing with the effect of such L .This is done by not focusing on L itself,but on its bound,which allows the core information on L to be extracted and used for control design.A similar idea was used in Singh [17]for attitude tracking control without considering singularities,thrusters failures,or thrust limits.In our design,we use this nonregressor-based method to develop robust adaptive control algorithms to cope with the effect of uncertainties and disturbances and to accommodate actuation failures and thrust limits.To this end,we first examine the environmental disturbances due to gravitation,solar radiation,magnetic forces (all could be assumed bounded),and aerodynamic drags (proportional to the square of angular velocity)[16,18].With all those disturbances considered,it is reasonable to assume that the external disturbances T d satisfy k T d k c g c d k !k 2with c d 0and c g 0unknown but constant.Also note that k q eq e 0I k 1and k C k 1.Then under the assumption that !d ,_!d ,q d ,and _q d are bounded for all t 0,it is straightforward to show that k qe q e 0I !e k k q e q e 0I ! C!d k k !k c w for anunknown constant c w 0.Finally,note that k d Js k c f k ! C!d q e k c f k !k c 0for some constant c 0 0.Conse-quently,it can concluded that,although L contains nonlinear,uncertain,and time-varying terms,there always exist some unknown constants b 0 0,b 1 0,b 2 0,and b 0such thatk L k b 0 b 1k !k b 3k !k 2 b(14) 1 k !k k !k 2(15)It is important to note that Eq.(14)holds for any type of rigid spacecraft and Eq.(15)is independent of any physical parameters or operating conditions of spacecraft under consideration.In other words,both Eqs.(14)and (15)are true regardless of external disturbances,uncertain and time-varying moment inertia,or type of spacecraft.This fact is used for our control design,which leads to a highly robust,adaptive,and fault-tolerant control scheme that does not need to be redesigned or reprogrammed even if the operating conditions or physical parameters of the spacecraft change.Furthermore,using the indirect nonregressor-based method leads to control algorithms that are much simpler in structure and demand much less online computations.More about this is discussed in the next section.The control objective is to develop a robust,adaptive,and fault-tolerant attitude tracking control scheme such that the following goals are achieved in the presence of external disturbances,moment inertia uncertainties,possible actuation failures,and thrust limits:R1)The closed-loop system is globally stable in that all the internal signal variables are bounded and continuous.R2)The attitude orientation and velocity tracking errors converge to a small set containing the origin,that is,k q e t k " 1,k !e t k "2t T .R3)The tracking performance indexI q limt !11t Z t0k s !e ;q e k 2d is bounded.III.Fault-Tolerant Robust Adaptive AttitudeTracking ControlWe first consider the case in which the vehicle is endowed with only three thrusters and each thruster experiences fading actuation (partial power loss)but is still active.The results are extended to the case in which some of the thrusters have completely failed and there exists actuation limit on each thruster.A.Fault-Tolerant Attitude Tracking Control with Fading ActuatorsWhen the vehicle has three actuators and each of them partially loses its actuation effectiveness,the attitude dynamics are governedCAI,LIAO,AND SONG 1457D o w n l o a d e d b y X I 'A N J I A O T O N G U N I VE R S I T Y o n N o v e m b e r 17, 2013 | h t t p ://a r c .a i a a .o r g | D O I : 10.2514/1.31158byJ _!! J ! T d (16)where 2R 3 3is the actuation effectiveness matrix of the form1000 2 000 3 2435(17)with 0 i 1being the “actuator health indicator ”for the i thactuator.The case in which i 1implies that the actuator is healthy; i 0is the case in which the i th actuator is turned off or totally failed;and 0< i <1corresponds to the case in which the i th actuator partially loses its actuating power (fading actuation).When the three actuators encounter partial power loss (fading actuation),the actuation effectiveness matrix becomes uncertain or even time varying but remains positive de finite.In such case,we have the following result.Theorem 1:Consider a spacecraft involving fading actuation in that the attitude dynamics are governed by Eq.(16)with 0< i <1(i 1,2,3).If the following control scheme is implemented,k 0 t s;t^b k s k "_^b1^b 2k s k 2k s k ";"1(18)where k 0>0, >0, 1>0,and 2>0are chosen by the designer.Then the control objectives as stated in R1–R3are achieved.Proof :Let min min f 1; 2; 3g ,which is positive as long as the thrust power is not completely lost.Consider the Lyapunov function candidateV 12s T J s12 2 minb min ^b 2 h q T e q e 1 q e 0 2i (19)In view of Eq.(12),it follows that_V0:5s T d J d t s T s T L 0:5d J d t s q e1 2b min ^b _^b !T e q e (20)Note that the last term in Eq.(20)was obtained by usingq T e q e q 2e 0 1,_q e 0 12q T e !e,and the de finition of s as in Eq.(11).Further simpli fication of Eq.(20)leads to_V s T L 12b min ^b _^b 2q T e q e With the control algorithms as given in Eq.(18),it can be shown that _V s T k 0 s s T L 1 2b min ^b _^b 2q T e q ek 0 min s T s 2q T e q e min k s k 2k s k b1 2b min ^b _^b k 0 min s T s 2q T e q e min ^b k s k 2 k s k b 12 b min ^b_^b k 0 min s T s 2q T e q e b min ^bk s k 2"b1 2b min ^b _^b k 0 min s T s 2q T e q e b 12b min ^b^b (21)wherek s k 1(8" 0),1,(8 0),and the updatingscheme for ^bas given in Eq.(18)were employed.Because 12 b min ^b^b 12 2 minb min ^b 2 12 2 min b 2 2min ^b 212 2 min b min ^b 2 1b 22 2 min and1 2 b min ^b ^b 1 min 2b ^b min ^b21 min2 ^b b 2 min 2 1b 24 2 min 1b 24 2 minIt is readily obtained from Eq.(19)that _V k 0 min s T s 2q T e q e 12 2 minb min ^b21b 22 2 minb 0V "0(22a)_V k 0 min s T s 2q T e q e "1(22b)_V k 0 min s T s 2q T e q e "2(22c)with0 min f k 0 min = max J ; 1; g >0"0 1 q 0 21b 22min b <1"11b 22 2 minb <1;"21b 24 2 minb <"1<1Note that Eq.(22a)implies that V 2L 1.Consequently,we haves 2L 1,q e 2L 1,and ^b2L 1,which ensure that 2L 1,_^b 2L 1, 2L 1,and F c 2L 1on the time interval [0,T ]and the solution can be extended past t T .The same arguments hold for any finite T and the solution exists for all t 2 0;1 [15,19].Moreover,it is seenfrom Eqs.(22b)and (22c)that _V<0when s;q e are outside of the setB 1≜(s;q e :k s k"10min r ;k q e k "1p )or the setB 2≜( s;q e :k s k"2k 0 min r ;k q e k "2p)Because "1>"2,set B 1encloses set B 2completely;thus,the systemstates s;q e may move in or out of B 2but,once inside the set B 1, s;q e cannot go out of it because they will be attracted back to B 2.That is,the states are con fined in the set B 1.Furthermore,because of Eq.(11),!e is bounded as well.Its bound can be determined asB 3≜f !e :k !e k"2p gThis can be shown by using !e 0:5d J d t ,instead of q e 0:5d J d t,in Eqs.(12)and (13).With the same Lyapunov function candidate as given in Eq.(19),it follows that1458CAI,LIAO,AND SONGD o w n l o a d e d b y X I 'A N J I A O T O N G U N I VE R S I T Y o n N o v e m b e r 17, 2013 | h t t p ://a r c .a i a a .o r g | D O I : 10.2514/1.31158_V0:5s T d J d t s T s T L ! 0:5d J d t s !e1 2 b min ^b _^b !T e q e s T s T L ! 1 2b min ^b _^b !T e !e where L ! takes the same form as in Eq.(13)with q e 0:5d Jbeing replaced by !e 0:5d Jd t.Note that such L ! still obeys the relation (14)and (15).Thus,it is straightforward to show that _V k 0 min s T s !T e !e "2,that is.,!e is bounded byB 3≜f !e :k !e k"2p gFinally,it can be shown from Eq.(22c)thatlimt !11t Zt0k s k 2d t limt !1V 0 k 0 min t"2k 0 min1b 24 2 2min k 0bk 0 min <1implying that larger k 0and 2(faster updating rate)and smaller and1lead to better performance.Thus,the result as stated in Theorem 1is established.Remark 2:Note that when all the actuators are healthy,the actuation effectiveness matrix I and the control scheme remains the same as in Eq.(18),implying that the proposed control as given in Eq.(18)is able to achieve attitude tracking regardless of the thruster health condition as long as no thruster is completely failed.Remark 3:It is worth mentioning that,although the parameter min is used in stability analysis,an analytical estimate of this parameter is not needed because the proposed control algorithms do not involve such a parameter.As can be seen from Eq.(18),the control scheme only involves simple functions and variables.All can be computed inexpensively;less computing power is needed compared to most existing methods.If a vehicle with only three actuators suffers from a failure of one or more thrusters ( i 0),the vehicle becomes underactuated.Under certain conditions,it is still possible to achieve attitude tracking along certain directions [20,21].It is noted that in this case arbitrary attitude tracking along all three directions in the presence of modeling uncertainties and disturbances as well as thrust faults becomes physically challenging.We address this issue next by considering the case in which the vehicle is equipped with more than three thrusters.B.Fault-Tolerant Attitude Tracking Control Under Engine FailuresWhen the vehicle has more than three thrusters,some of which might experience losing power partially or totally,its attitude dynamics are described byJ _!! J ! D F T d (23)where F 2R n n >3 denotes the propulsion force vector produced by the n thrusters,D 2R 3 n is the thruster distribution matrix (for a given vehicle,D is available and can be made full-row rank by properly placing the thrusters at certain locations and directions on the vehicle),and the matrix diag 1;...; n 2R n n is a matrix characterizing the health condition of the engines. is an identity matrix only if all engines are healthy.Otherwise, is a diagonal matrix with uncertain and time-varying diagonal elements.The case of i 0indicates that the engine has either totally failed or been shut down purposely and,in either case,we want to keep the vehicle under control and achieve stable and high precise attitude tracking.It is interesting that this can be achieved with a slight modi fication to the control scheme presented earlier,as follows:F k 0 t D T s; t^b k s k "_^b 1^b 2k s k 2 k s k ";"1(24)where all the control variables and parameters are de fined as before.The stability and control performance can be analyzed by using a similar Lyapunov function candidate:V 12s T J : s12 2 minb min ^b 2 h q T e q e 1 q e 0 2i (25)where min >0is some unknown constant less than the minimum eigenvalue of D D T .Following the same lines as in the proof of Theorem 1,it can be shown that_Vs T k 0 D D T s s T L 1 2b min ^b _^b 2q T e q e k 0 min s T s 2q Te q e1min 2b min ^b2 1b 2min 2band the following result can be established.Theorem 2:Consider the spacecraft with the attitude dynamics as in Eq.(23).Assume that there are n thrusters (n >3)properly mounted along the vehicle and the remaining active thrusters (including the fading ones)are able to produce a combined force suf ficient enough to allow the vehicle to perform given maneuvers.If the control scheme (24)is applied,stable attitude tracking is ensured when all thrusters of the vehicle suffer from fading actuation (0< i <1).Furthermore,the control scheme is still able to achieve stable attitude tracking even if some of the thrusters completely fail to work as long as the number of failed thrusters is no more than n 3such that D D T remains positive de finite.Remark 4:Because is not used in the control scheme,there is no need to include a health monitoring unit to identify or estimate which thruster is unhealthy.Knowledge of the degree of failure for each thruster is not even needed.The thruster fault accommodation/compensation is done automatically and adaptively by the proposed control algorithms.This feature is necessary to build affordable and effective fault-tolerant flight control schemes.Remark 5:Because the thruster distribution matrix D is made full-row rank (by proper placement of the thrusters on the vehicle),the stability is ensured as long as D D T is positive de finite.For instance,consider a vehicle with six thrusters that are distributed in such a way thatD 1000:20:10:30100:60:50:300100:20:42435and the actuation effectiveness matrix diag 0:5;0:7;0:7;0;0;0 ,implying that three of the thrusters suffer from fading actuation and the other three have totally failed.In such a case, min D D T 0:5and the control scheme is still able to ensure stable attitude tracking.Of course,the implicit assumption is that the remaining active thrusters are able to produce a suf ficient actuating torque vector for the vehicle to perform the given maneuvers.C.Fault-Tolerant Attitude Tracking Control Under Thrust Failures and Thrust LimitsIt is of theoretical and practical importance to consider the factors of uncertainties,disturbances,actuation failures,and thrust limitssimultaneously.Let j F i j F i max(i 1;2;...;n )and F imax >0denote the maximum allowable thrust force of each thruster.For the system to admit a feasible attitude tracking control solution under such a severe situation,it is necessary to assume that the functionalCAI,LIAO,AND SONG 1459D o w n l o a d e d b y X I 'A N J I A O T O N G U N I VE R S I T Y o n N o v e m b e r 17, 2013 | h t t p ://a r c .a i a a .o r g | D O I : 10.2514/1.31158(not necessarily healthy)thrusters are able to produce a combined force suf ficient enough to allow the vehicle to perform a given maneuver in the sense that there exists a constant f 0>0so thatmin F maxk D kb f 0(26)where min >0is de fined as before andF max min f F 1max ;F 2max ;...;F nmax g .A similar assumption was proposed in [13,14,18,22]without considering thrust faults.The control scheme ensuring attitude tracking of spacecraft under the conditions as mentioned is given byF F maxD Tk D ksat k 0 t s (27)withsat k 0 t ss if k s k F max = k 0 k 0 t s maxif k s k F max = k 0t^bk s k ";_^b 1^b 2k s k 2 k s k ";"1where 1 k !k k !k 2and k 0>0, >0, 1>0,and 2>0are chosen by the designer.Theorem 3:Consider the spacecraft equipped with n thrusters(n >3)under thrust limits j F i j F imax(i 1;2;...;n ).Assume the number of the failed thrusters is no more than n 3such that D D T is positive de finite and that the remaining active thrusters (including the fading ones)are able to produce a combined force suf ficient enough to allow the vehicle to perform given maneuvers in that Eq.(26)holds.If the control scheme (27)is applied,then the control objectives as stated in R1–R3are achieved.To show the stability,two cases need to be addressed.Case 1:k s k F max = k 0 ,F F max D T s,which satis fies the force constraint of j F i j F max F imax ,considering the Lyapunov function candidate asV 1s T J s h q T e q e 1 q e 0 2iit follows that_V s T D F L 2q T e q es TD D T F max s L 2q T e q ek s k min F max k D k b q T e q e k s k f 0 2k q e k 2<0Case 2:k s k F max = k 0 ,F D Tk 0 s ,considering the Lyapunov function candidate as in Eq.(25),it follows that_Vs T k 0 D D Tk D ks s T L 1 2 b min ^b _^b 2q T e q e k 0 min k D k s T s 2q T e q e12 min 2b min ^b2 1b 22 min 2bThe result thus is established using the same argument as in the Proof of Theorem 2.Note that sat is continuous everywhere including at the point k s k F max = k 0 ,the control is bounded and smootheverywhere.Also,it can be veri fied that j F i j F max F imax8s .Remark 6:It is seen that control scheme (27)not only meets the thrust limit,but also effectively accommodates modeling uncertainties,external disturbances,and thrust faults.It has a simple structure in which the inertia matrix (practically time varying and uncertain)or its estimation is not directly involved,thus simplifying the design process and online computation signi ficantly.The control scheme can be easily set up and implemented with much less demand on onboard computing power and memory space compared to most existing methods.IV.Simulation StudyTo verify the effectiveness of the proposed control scheme,simulations on a vehicle with six thrusters under various conditions are conducted.The six thrusters are assumed to be distributed symmetrically on three axes of the body frame B of the spacecraft,and the propulsion force is perpendicular to the corresponding axis such that the distribution matrix can be simply determined by the distance l i (i 1;...;6)between the center of mass of the vehicle and the position of the thruster,as illustrated in Fig.1.In this simulation,l 1 l 2 0:8 m ,l 3 l 4 l 5 l 6 0:7 m ,so that the distribution matrix isD 0:8 0:80000000:7 0:70000000:7 0:72435This simulation is carried out under the condition that the momentinertia matrix J J 0 J u t is unknown and time varying (due to,for instance,both fuel burning and payload release)as re flected in J u t and depicted in Fig.2(noting the “jump ”variation of the elements)and the normal part J 0isJ 0 2000:901700:90152435taken from [13,14,22](J 0is usually nondiagonal unless the axis of the body frame and the principal axis coincide).The vehicle is to perform the maneuver that changes its attitude from the initial attitudeq 0 0:1;0:15; 0:2 Tand q 0 01 q T qp 1460CAI,LIAO,AND SONGD o w n l o a d e d b y X I 'A N J I A O T O N G U N I VE R S I T Y o n N o v e m b e r 17, 2013 | h t t p ://a r c .a i a a .o r g | D O I : 10.2514/1.31158to a new attitude along the desired trajectoryq d t3p 3sin 0:1t ; 6p 6sin 0:1t 12sin 0:1t T andq d 0 t1 q T d q dq The disturbance torque simulated is of the formT d k !k 2 0:5 sin 0:8t cos 0:5t cos 0:3t T .We simulate three different cases:1)healthy thrusters,2)thrusters with fading and failed actuation,and 3)thrusters with limited thrusts and failed actuation.Our earlier theoretical analysis declares that controller (24)can deal with cases 1and 2and controller (27)can handle case 3effectively;we now verify that claim.Note that,in both controllers,one only needs to simply select the control parameters k 0>0, 2>0, >0, 1>0,and >0.In the simulation,they are chosen quite arbitrarily as k 0 20, 2 100, 2, 1 0:01,and 0:1and remain unchanged for all the simulation cases.Also note that there is no need to carry out the time-consuming and sometimes painful task of analytical derivation (in contrast with most other control methods)to set up the controller.A.Healthy ActuatorsWith all thrusters functioning healthily,control scheme (24)is applied.The angular velocity and attitude tracking errors are shown in Fig.3.The three control torques produced by the six thrusters are presented in Fig.4.The control performance index and the adaptiveparameter ^bt are depicted in Figs.5and 6,respectively.One can observe high control precision and good tracking process.The control action is bounded and smooth.B.Fading Actuation and Failed ActuationThis represents a severe case in which not only do some thrusterslose partial power with randomly varying heath levels,but some thrusters have also totally failed or are shut down purposely,as illustrated in Fig.7in which the health level of each thruster is generated by the following function i 0:7 0:15rand t i 0:1sin 0:5t i =3 ;i 1;...;6(28)which swings between 0.95and 0.45,where t i mod t t i ; T ,with t i 0:4 i 1 s and T 2:4s denoting the time delay and generation interval,respectively.rand 2 1;1 is a random number generator,which generates a random number if t i 0and holds its previous value if t i ≠0.Among the six thrusters,thruster 3only supplies 20%of the actuation power at the time instant t 8s and,after,thrusters 4and 5have failed or are shut down after t 10and 12s,respectively.The control (24)with the samecontrolFig.2Uncertain moment ofinertia.Fig.3Angular velocity (rad =s )and attitude trackingerrors.Fig.4Three actuation torques (N m).Fig.5Bounded control performance index.Fig.6Adaptive parameter ^bt .CAI,LIAO,AND SONG 1461D o w n l o a d e d b y X I 'A N J I A O T O N G U N I VE R S I T Y o n N o v e m b e r 17, 2013 | h t t p ://a r c .a i a a .o r g | D O I : 10.2514/1.31158。
Robust Control and Estimation
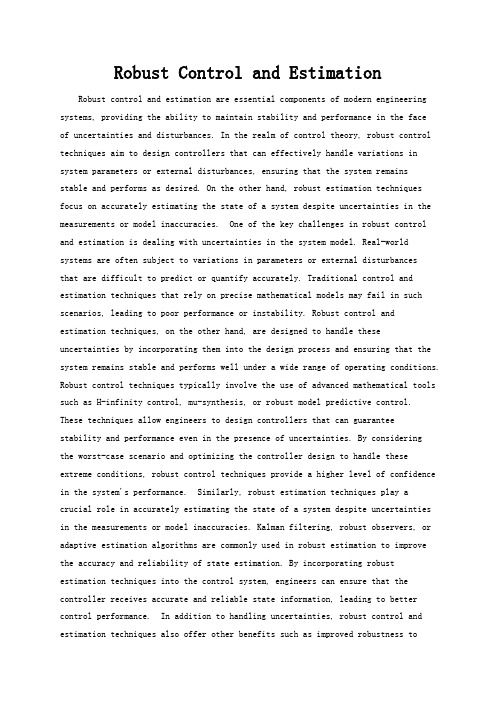
Robust Control and Estimation Robust control and estimation are essential components of modern engineering systems, providing the ability to maintain stability and performance in the faceof uncertainties and disturbances. In the realm of control theory, robust control techniques aim to design controllers that can effectively handle variations in system parameters or external disturbances, ensuring that the system remainsstable and performs as desired. On the other hand, robust estimation techniques focus on accurately estimating the state of a system despite uncertainties in the measurements or model inaccuracies. One of the key challenges in robust controland estimation is dealing with uncertainties in the system model. Real-world systems are often subject to variations in parameters or external disturbancesthat are difficult to predict or quantify accurately. Traditional control and estimation techniques that rely on precise mathematical models may fail in such scenarios, leading to poor performance or instability. Robust control andestimation techniques, on the other hand, are designed to handle theseuncertainties by incorporating them into the design process and ensuring that the system remains stable and performs well under a wide range of operating conditions. Robust control techniques typically involve the use of advanced mathematical tools such as H-infinity control, mu-synthesis, or robust model predictive control.These techniques allow engineers to design controllers that can guaranteestability and performance even in the presence of uncertainties. By consideringthe worst-case scenario and optimizing the controller design to handle these extreme conditions, robust control techniques provide a higher level of confidencein the system's performance. Similarly, robust estimation techniques play acrucial role in accurately estimating the state of a system despite uncertaintiesin the measurements or model inaccuracies. Kalman filtering, robust observers, or adaptive estimation algorithms are commonly used in robust estimation to improvethe accuracy and reliability of state estimation. By incorporating robustestimation techniques into the control system, engineers can ensure that the controller receives accurate and reliable state information, leading to better control performance. In addition to handling uncertainties, robust control and estimation techniques also offer other benefits such as improved robustness tosensor noise, modeling errors, or external disturbances. By designing controllers and estimators that are robust to these factors, engineers can enhance the overall performance and reliability of the system. Moreover, robust control and estimation techniques can also simplify the tuning process for controllers, as they are designed to be more forgiving of variations in system parameters. Overall, robust control and estimation play a critical role in ensuring the stability, performance, and reliability of modern engineering systems. By incorporating robust techniques into the design process, engineers can create systems that are more resilient to uncertainties and disturbances, leading to improved overall performance and reliability. As technology continues to advance and systems become more complex, the importance of robust control and estimation techniques will only continue to grow, making them essential tools for engineers in various fields.。
基于动态逆方法的飞行控制系统设计与仿真

5.章卫国.杨向中模糊控制理论与应用 1999
6.申玉安.申学仁.李云保自动飞行控制系统 2003
7.章卫国.肖顺达一种近似解耦方法在飞行控制系统中的应用 1998(01)
8.张明廉飞行控制系统 1996
9.郭锁凤.申功璋.吴成富先进飞行控制系统 2003
10.郭锁凤.朱恩动态逆飞控系统中处理非定常气动力的方法 1998(05)
7.魏中成飞机总体设计中的飞行控制律模型设计与应用方法研究[学位论文]硕士 2007
引用本文格式:杜金刚基于动态逆方法的飞行控制系统设计与仿真[学位论文]硕士 2006
本章应用Simulink仿真软件,将本论文撰写过程中所编写的仿真程序,汇集在一起,综台成为一个基于动态逆方法的飞行控制仿真软件包。以利于仿真验证和相关内容是查询学习。
6.1主界面
基于动态逆控制方法的飞行控制仿真主界面见图6.1
图6·1基于动态逆方法的飞行控制仿真主界面
本论文内容所涉及的仿真程序分为两个部分,一部分为基于动态逆方法带推
24.Consolini L.Tosques M Local path following problem for time-varying nonlinear control affine systems 2003
25.Tomas-Rodriguez M.Banks S P The dynamical inverse problem for a nonlinear Schrodinger equation using boundary control 2003
20.肖顺达推力矢量飞机是未来战斗机的发展方向
21.夏小华.高为炳非线性系统控制及解耦 1993
H-无穷 控制
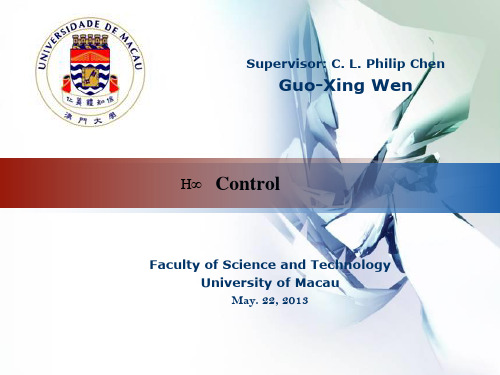
Robust Adaptive Neural Control for a Class of Perturbed Strict Feedback Nonlinear Systems
Step i:
Robust Adaptive Neural Control for a Class of Perturbed Strict Feedback Nonlinear Systems
H-infinity control question: Designing a desired controller so that the closed-loop system is stability and the transfer matrix can be minimized
Fundamental knowledge
The practical control law:
H-infinity tracking design in indirect adaptive fuzzy control
Finally, we have
H-infinity tracking design in indirect adaptive fuzzy control
Robust Adaptive Neural Control for a Class of Perturbed Strict Feedback Nonlinear Systems
Step n:
THE END
Q&A
23
Backstepping design: step 1:
Robust Adaptive Neural Control for a Class of Perturbed Strict Feedback Nonlinear Systems
正弦信号跟踪控制算法
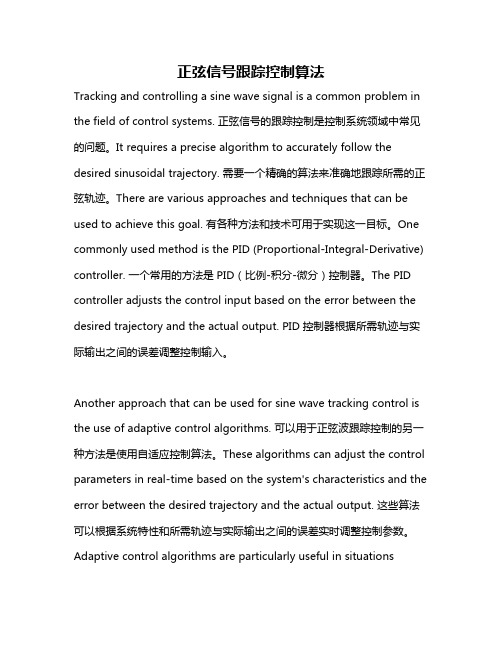
正弦信号跟踪控制算法Tracking and controlling a sine wave signal is a common problem in the field of control systems. 正弦信号的跟踪控制是控制系统领域中常见的问题。
It requires a precise algorithm to accurately follow the desired sinusoidal trajectory. 需要一个精确的算法来准确地跟踪所需的正弦轨迹。
There are various approaches and techniques that can be used to achieve this goal. 有各种方法和技术可用于实现这一目标。
One commonly used method is the PID (Proportional-Integral-Derivative) controller. 一个常用的方法是PID(比例-积分-微分)控制器。
The PID controller adjusts the control input based on the error between the desired trajectory and the actual output. PID控制器根据所需轨迹与实际输出之间的误差调整控制输入。
Another approach that can be used for sine wave tracking control is the use of adaptive control algorithms. 可以用于正弦波跟踪控制的另一种方法是使用自适应控制算法。
These algorithms can adjust the control parameters in real-time based on the system's characteristics and the error between the desired trajectory and the actual output. 这些算法可以根据系统特性和所需轨迹与实际输出之间的误差实时调整控制参数。
具有磁滞输入非线性系统的鲁棒自适应控制_英文_
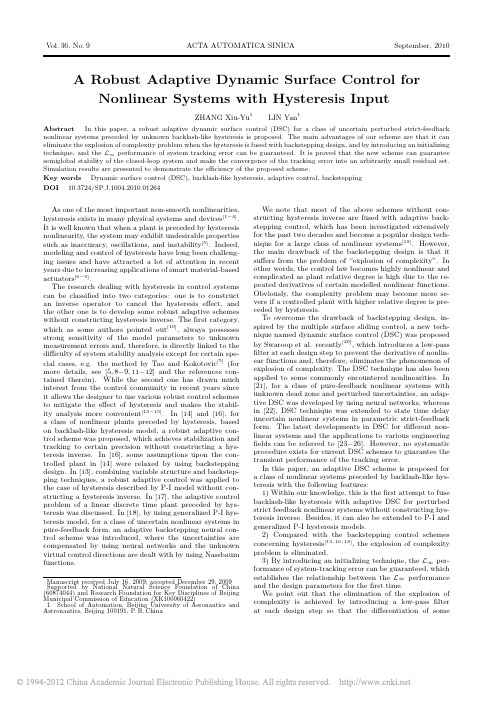
We note that most of the above schemes without constructing hysteresis inverse are fused with adaptive backstepping control, which has been investigated extensively for the past two decades and become a popular design technique for a large class of nonlinear systems[19] . However, the main drawback of the backstepping design is that it suffers from the problem of “explosion of complexity”. In other words, the control law becomes highly nonlinear and complicated as plant relative degree is high due to the repeated derivatives of certain modelled nonlinear functions. Obviously, the complexity problem may become more severe if a controlled plant with higher relative degree is preceded by hysteresis. To overcome the drawback of backstepping design, inspired by the multiple surface sliding control, a new technique named dynamic surface control (DSC) was proposed by Swaroop et al. recently[20] , which introduces a low-pass filter at each design step to prevent the derivative of nonlinear functions and, therefore, eliminates the phenomenon of explosion of complexity. The DSC technique has also been applied to some commonly encountered nonlinearities. In [21], for a class of pure-feedback nonlinear systems with unknown dead zone and perturbed uncertainties, an adaptive DSC was developed by using neural networks, whereas in [22], DSC technique was extended to state time delay uncertain nonlinear systems in parametric strict-feedback form. The latest developments in DSC for different nonlinear systems and the applications to various engineering fields can be referred to [23−26]. However, no systematic procedure exists for current DSC schemes to guarantee the transient performance of the tracking error. In this paper, an adaptive DSC scheme is proposed for a class of nonlinear systems preceded by backlash-like hysteresis with the following features: 1) Within our knowledge, this is the first attempt to fuse backlash-like hysteresis with adaptive DSC for perturbed strict feedback nonlinear systems without constructing hysteresis inverse. Besides, it can also be extended to P-I and generalized P-I hysteresis models. 2) Compared with the backstepping control schemes concerning hysteresis[13, 16, 18] , the explosion of complexity problem is eliminated. 3) By introducing an initializing technique, the L∞ performance of system-tracking error can be guaranteed, which establishes the relationship between the L∞ performance and the design parameters for the first time. We point out that the elimination of the explosion of complexity is achieved by introducing a low-pass filter at each design step so that the differentiation of some
基于应答器位置测量的AUV非线性模型预测对接控制
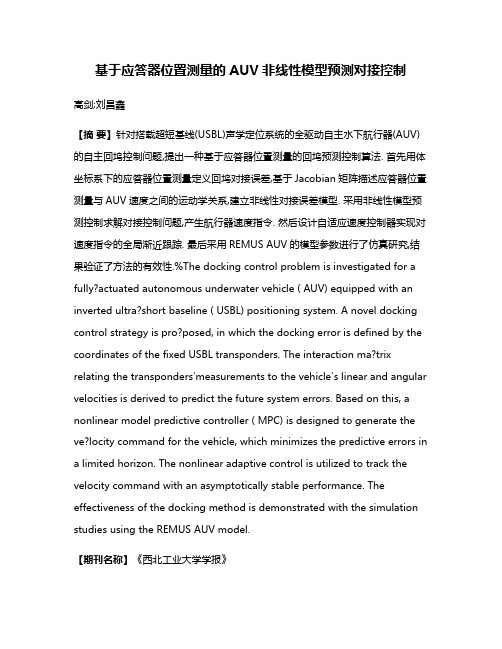
基于应答器位置测量的AUV非线性模型预测对接控制高剑;刘昌鑫【摘要】针对搭载超短基线(USBL)声学定位系统的全驱动自主水下航行器(AUV)的自主回坞控制问题,提出一种基于应答器位置测量的回坞预测控制算法. 首先用体坐标系下的应答器位置测量定义回坞对接误差,基于Jacobian矩阵描述应答器位置测量与AUV速度之间的运动学关系,建立非线性对接误差模型. 采用非线性模型预测控制求解对接控制问题,产生航行器速度指令. 然后设计自适应速度控制器实现对速度指令的全局渐近跟踪. 最后采用REMUS AUV的模型参数进行了仿真研究,结果验证了方法的有效性.%The docking control problem is investigated for a fully?actuated autonomous underwater vehicle ( AUV) equipped with an inverted ultra?short baseline ( USBL) positioning system. A novel docking control strategy is pro?posed, in which the docking error is defined by the coordinates of the fixed USBL transponders. The interaction ma?trix relating the transponders′measurements to the vehicle′s linear and angular velocities is derived to predict the future system errors. Based on this, a nonlinear model predictive controller ( MPC) is designed to generate the ve?locity command for the vehicle, which minimizes the predictive errors in a limited horizon. The nonlinear adaptive control is utilized to track the velocity command with an asymptotically stable performance. The effectiveness of the docking method is demonstrated with the simulation studies using the REMUS AUV model.【期刊名称】《西北工业大学学报》【年(卷),期】2015(033)005【总页数】7页(P860-866)【关键词】自主水下航行器;对接;逆USBL定位系统;模型预测控制;自适应控制系统【作者】高剑;刘昌鑫【作者单位】西北工业大学航海学院,陕西西安 710072;西北工业大学航海学院,陕西西安 710072【正文语种】中文【中图分类】TP24自主水下航行器(autonomous underwater vehicle, AUV)是人类探索海洋的重要工具,AUV与水下回收平台的对接问题是迫切需要研究解决的关键技术之一。
自动化专业毕业设计外文翻译--输入力矩受限的机器人鲁棒自适应控制
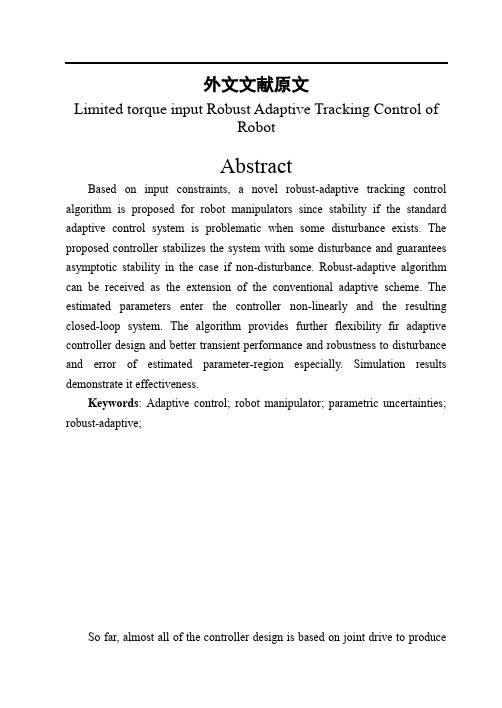
外文文献原文Limited torque input Robust Adaptive Tracking Control ofRobotAbstractBased on input constraints, a novel robust-adaptive tracking control algorithm is proposed for robot manipulators since stability if the standard adaptive control system is problematic when some disturbance exists. The proposed controller stabilizes the system with some disturbance and guarantees asymptotic stability in the case if non-disturbance. Robust-adaptive algorithm can be received as the extension of the conventional adaptive scheme. The estimated parameters enter the controller non-linearly and the resulting closed-loop system. The algorithm provides further flexibility fir adaptive controller design and better transient performance and robustness to disturbance and error of estimated parameter-region especially. Simulation results demonstrate it effectiveness.Keywords: Adaptive control; robot manipulator; parametric uncertainties; robust-adaptive;So far, almost all of the controller design is based on joint drive to produceany torque on the basis of; and is subject to the physical conditions, the output of the drive torque is limited, so the controller may lead to the control failure or deterioration of the quality control.Therefore the controller design must take into account the limited joint drive dynamic capability.For example, the operation of the industry to help the robot, some parameters are uncertain or unknown, adaptive control is based on the estimated parameters to deal with such issues one of the main control strategy, using the robot dynamic equations of linear parametric nature, through an integral operator estimates the robot parameters. As integral part of the role in the continued interference conditions, stability control system is not easy, so appropriate to limit or adjust the integral part of the role of the adaptive system to achieve an effective means of stabilization. Son ah estimated parameters can limit the extent required, thereby increasing the robustness of adaptive control system. However, this algorithm has six switch component, a little complicated, but really is the parameter is not specified range, it cannot give the system control quality and robustness of information.This paper presents a simple robust adaptive control algorithm, when the estimated parameters field contains the parameters of the true value, the closed-loop system to achieve asymptotically stable tracking; when there is interference or the estimated parameters with the true value of free parameter that is when the error system is stable.1. MANIPULATOR DYNAMIC MODEL AND CHARACTERISTIC MODELConsider a robotic manipulator with n degrees of freedom. The continuous Lagrange dynamic model is given by()(,)()M q q C q q q G q u ++= Where q ∈R n and q ∈R nare the vector of generalized joint coordinates and velocity coordinates, respectively. The inertia matrix M(q)-M T (q)> 0 ,and there exist two constant positive scalars M min and M max such that minM ≤M ≤max M , nu R ∈is the vector of commanded generalized force, and (,)C q qq andG(q) are the terms due to Carioles, Centripetal and gravity forces. In actual application, the uncertain parameters and un-modeled dynamics usually exist in the established dynamic model in (1).When the sample time sT is small enough, at instant t=k s T q and q can be approximated by()(1)s q k q k q T --≈ and 2(1)2()(1)s q k q k q k qT +-+-≈ . Respectively Using the above relationships thediscrete-time representation of (1) becomes ()()()()()()()()()()12211S M q k q k f k q k f k q k G k u k T '∙+--+=(2a) Premultiplying(2a) by ()()21sT mM q k -results in()()()()()()()()1211q k f k q k f k q k G k k u k β'+---+- where()()()()()()112,s f k I T M q k C q k qk -=- , ()()()()()()12,s f k I T M q k C q k qk -=-+ ()()()()()()()()2121s s G k T M q k G q k k T Mq k β--'==and I denotes the unitary diagonal matrix with an appropriate dimension.If the designed (),,u t q q (),,u t q q is continuous in t ,q and q , then the solution (q,q ) of (1) will be continuously differentiable. Let()()()1,,W q qM q C q q -= and (),ij w q q be ij-th element of matrix (),ij W q q ; We define()()()()11111SF k f k f k T =--and then ΔF1(k) can be expressed as()()()()()()()1,1,1F k W q k qk W q k q k =--- For the ij-th element Δf1,ij(k) of matrix ΔF1(k)we can get()()()()()()()()1,,1,1ij ij ij f k w q k qk w q k q k =---- =()()()()()()()1111,|1ij q q k T qq k w q qq k q k q ςς=-=-∂---∂()()()()()()()1111,|1ij q q k T qq k w q qq k q k q ςς=-=-∂---∂ =()()()()()11211,|1ij s q q k T qq k w q q T q k q ςςς=-=-∂--∂ ()()()()()11311,|1ij s q q k T qq k w q qT q k q ςςς=-=-∂--∂with 0≤1ς≤1,and 2ς,3ς≈1 for a small sample time Ts . From (3), it can beseen that ΔF1(k)→0 as Ts converges to zero in a compact set of (),q q(),q q.Similar properties can also be achieved for the coefficient matrixes f2(k),()G k '()G k ' and β (k) .In a compact set of (),q q, the following properties can be deduced from (3)and the expressions of the coefficient matrixes of (2b):Property 1: If the sample time Ts is small enough,then all coefficient matrixes of (2b) are slowly time varying;Property 2: f1(k)→2I,f2(k)→ −I and f1(k)+f2(k)→I, as the sample time Ts converges to zero. Then we can define the discrete equation (2b) with Properties 1 and 2 as the robotic manipulator characteristic model.2. MULTI-VARIABLE GSA CONTROLLER WITH NN COMPENSATIONDiscrete equation (2b) can be expressed as follows:()()()()1T q k k k e k θφ+=+()()()()1T q k k k e k θφ+=+ (4) Where()()()()()12,,,Tk f k f k k G k θβ'=⎡⎤⎣⎦,()()()(),1,,1TT T T Tk q k q k u k φ⎡⎤=--⎣⎦,e(k) denotes the vector of white noise with zero mean. In the case of ()G k ' ≡0, ()k θand ()k φ can be reduced to()()()()12,,Tk f k f k k θβ=⎡⎤⎣⎦()()()(),1,,TT T Tk q k q k u k φ⎡⎤=-⎣⎦Then the elements of q(k +1) can be expressed as()()()()1i i j q k k k e k φθ+=+ (5)wherei = 1,…,n, i q (k+1)) is the element i of q(k +1), ()i e k is the element i of e(k) and ()i k θ is the column i of the matrix()()()()()()()()()()()11111T T TP k k k P k p k k p k k k P k k φφλλφφ-⎡⎤--=∙--⎢⎥+-⎢⎥⎣⎦()k Θ . When the coefficient matrixes are unknown, it can be estimated by()ˆk Θ=π (q(k),q(k−1),...,u(k−1),...) , (6a) where ()ˆk Θis the estimated coefficie nt matrix of Θ(k) at the instant t=kTs , and ()π∙ denotes an estimation operator. Considering the coefficient matrixesof the characteristic model being slowly time-varying, we can obtain the selected estimation operator by the weighted least squares method (WLS)[13], namely()()()()()()()()()()()()1ˆ111i i i i TP k k k k q k k k k k P k k φθθφθλφφ-+=+∙+-+-, ()()()()()()()()()()()11111T T TP k k k P k p k k p k k k P k k φφλλφφ-⎡⎤--=∙--⎢⎥+-⎣⎦with λ(k+1)=μ0λ(k)+(1−μ0), 0<μ0 ≤1, and ()ˆik θ the column i of the matrix ()ˆk Θ. Given a desired smooth trajectory ()d q t , the adaptive control controller is designed as follows()()()()T G c u k u k u k u k =++ (7)with the feed forward control law designedas()()()()()()()()()()112ˆˆˆ11T d d du k k k I q k f k q k f k q k βε-=+∙+---(7a) and the multi-variable GSAC feedback law as()()()()()()()()()()11122ˆˆˆˆ1Gdu k k k I L fk q k L f k q k G k βε-'=+∙--+ (7b)where ()()()d qk q k q k =- is the tracking error andε(k) >0 is a small scalar that avoids the estimated matrix ()ˆk β being singular. The term of ()cu k will be designed later; L1 and L2 are golden-section coefficients, that is,210.6182L =≈130.3822L =≈, 210.6182L =≈,which satisfy the relationship L1+L2=1 and 212L L = Substituting (7) into (2), we can get()()()()()()()()22112111s c M q k q k L f qk L f q k k T u k +---=- (8)()()()()()()()()()()()()()()()()()()()()()()()()()()()()()()()()()11121222122122ˆˆˆ11d s c k M q k M k q k M q k f M k f k L q k L q k M q k s k M q k L f k s k M q k L I f k f k q k k T u k M q k L f k s k =-+--++=----+-=- ()()()()()()()()()()()()()()()2212ˆˆˆˆ11d M q k f M k f k L q k L q k M q k G k M k G q k ---+-+-and ()()()()12ˆˆsM k T k k I βε-=+.Defining the tracking filtered error s(k +1) as and using the relationships L1+L2=1 and 212L L =,212L L = (8) can be expressed as()()()()()()()()()()()()()222121M q k s k M q k L f k s k M q k L I f k f k qk +=---- ()()21s c k T u k +- =()()()()22M q k L f k s k - (9)()()()()()()()()2212ˆˆˆs c M q k L I f k f k q k k T u k +--+- Which()()()()()()()()1212k k M q k L I f k f k qk =--- ()()()()()()212ˆˆˆM q k L I f k f k q k +-- Assuming ,()()()ˆM k M q k =,()()()()()11ˆˆM k f k M q k f k = and ()()()()()22ˆˆM k f k M q k f k =,if ()c u k is selected as ()()()()()()()2212ˆˆˆc s u k T M q k L I f k f k q k -=-- then Δ(k) = 0 , and then (9) can be written as()()()221s k L f k s k +=-()()()221s k L f k s k +=- (10)Since in Property 2 ()2f k I →-()2f k I →-as Ts → 0 in a compact set of (),q q(),q q , a small sample time Ts can be selected such that the inequality ()221L f k < can be satisfied. Therefore, the tracking filtered error s(k)asymptotically converges to zero in this case. The convergence of s(k) to zero in turn guarantees the convergence of q(k) to zero. Because of the dynamics of the estimator and the time-varying coefficients of the characteristic model, it is almost impossible to satisfy the above assumptions. Therefore, we can design a suitable compensation control law ()c u k to avoid possibly the case that the control performance is deteriorated or that the close loop system is even unstable due to the estimation errors. Hence ()c u k is designed as()()()()()()()()()()()2212ˆˆˆc s i i i u k T M k L I f k f k q k k k Ty k k ψδ-=--+=+()()()111s k q k L q k +=+-()()()()()()()()2212ˆˆˆc s u k T M k L I f k f k q k k -=--+ (11) where ()ˆkis the estimate of Δ(k) . Assuming Δ(k) is smooth enough and bounded, it then can be approximated by the linearly parameterized NN to any required degree of accuracy [6,14]. Then the element Δi(k) of Δ(k) can be expressed as()()()i i i k Ty k k ψδ=+ (12)where i= 1,…n, T n i R ψψ∈ is the column i of the optimal NN weightmatrix,1T n ψψψψ⎡⎤=⎣⎦…,.Activation functions ()()(),,Tn y k y k y k ψ⎡⎤=⎣⎦… represent the basis function vector, which can be selected as any one of Gaussian radial basis, B-spine basis, Wavelet basis, and etc. [14], and δi (k) denotes the element I of the NN reconstruction error vectork δ(k), namely()()()1,T n k k k δδδ=⎡⎤⎣⎦…, .Using compensation control law ()c u k , (9) can be written as ()()()()()()()221M q k s k M q k L f k s k +=-()()11ˆˆ,Tn n y k k ψψψψψψδ⎡⎤+--+⎣⎦… (13) Where ()ˆi k ψis the estimate of i ψ, and ()max sup i kk ψδδ=<∞ An estimate ()ˆi k ψis now obtained by minimizing the cost function ()()()()1112T J s k M q k s k =++ (14)After substituting (13) into (14), the gradient of the cost function in (14) is derived as()()1ˆT Jy k s k ψ∂=-+∂ (15) According to the gradient descent method the NN weight adaptation law can be designed as()()()()ˆˆ11T k k y k s k ψψα+=++ (16) with α > 0 . Then the compensation control law ()c u k in (11) can bewritten as()()()()()()()()2122211ˆˆTc s su k M k L I f k f k qk k y k T T ψ=--+ (17) In view of the case ()()12ˆˆ0I fk f k --≈ the term ()cu k can be simplified as()()()21ˆTc su k k y k T ψ=4. SIMULATION RESULTSConsider a planar, two-link, articulated manipulator as in [3] (as presented in Fig. 1), whose dynamics can be written explicitly as()1112111212212222210M M qq u hq h q q M M q q u hq --+⎛⎫⎛⎫⎛⎫⎛⎫⎛⎫+= ⎪ ⎪⎪ ⎪ ⎪⎝⎭⎝⎭⎝⎭⎝⎭⎝⎭Where122123242cos sin M M a a q a q ==++ 11132322cos 2sin M a a q a q =++ 222M a =3242sin cos h a q a q =-With 22211111c e e ce e a I m l I m l m l =++++,22e e ce a I m l =+31cos e ce e a m l l δ=,41sin e ce e a m l l δ=,111,1,2,30,e e m l m δ==== 110.12,0.5,0.25,c e I I I ===and 0.6ce l =. In the simulation, the sample time Ts = 2ms, the initial values and the parameters of the estimator and the controller are selected as P(0) =1×310I,λ(0) = 0.96 , μ0 = 0.98 , the anti -singularity factors(k) can be designed as ε(k) =5×610-exp(−kTs).According to the Property 2, the initial estimate values of the characteristicmodel coefficient matrixes are chosen as ()()12ˆˆ02,0f I f I ==-A basis set of activation function y(k) can be selected as in the Random Vector Function Link net [16], namely,()()()T y k V X k σ= (19) with V a randomly selected matrix and X(k) the NN input vector. ()σ∙can be chosen as the h yperbolic tangent function, and X(k) can be taken as()()()()(),1,,,1TT T T Td d X k q k q k q k q k ⎡⎤=-⎣⎦.The adaptation gain for the NN weight tuning is taken as α = 0.005 , and the initial values of the weights are set to zeros.The desired trajectory is chosen as()()()301cos 2,451cos 2T d q t t t ππ⎡⎤=--⎣⎦(20)外文翻译输入力矩受限的机器人鲁棒自适应控制摘要在输入力矩受限的情况下,提出一种全的简单鲁棒自适应控制算法。
ACS控制器在超精密定位系统中的应用
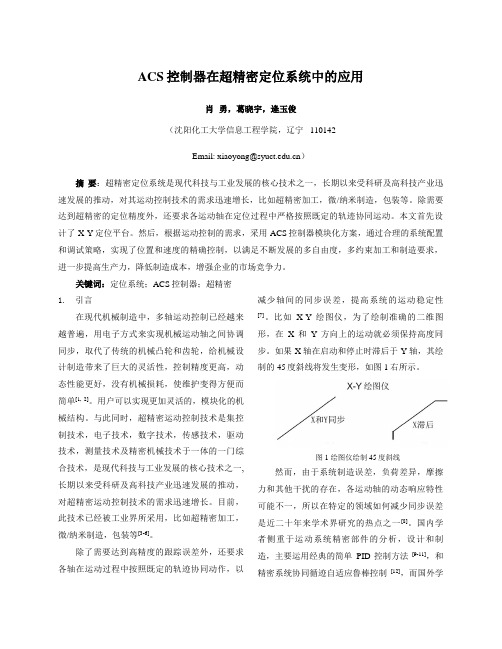
ACS控制器在超精密定位系统中的应用肖勇,葛晓宇,逄玉俊(沈阳化工大学信息工程学院,辽宁 110142Email: xiaoyong@)摘要:超精密定位系统是现代科技与工业发展的核心技术之一,长期以来受科研及高科技产业迅速发展的推动,对其运动控制技术的需求迅速增长,比如超精密加工,微/纳米制造,包装等。
除需要达到超精密的定位精度外,还要求各运动轴在定位过程中严格按照既定的轨迹协同运动。
本文首先设计了X-Y定位平台。
然后,根据运动控制的需求,采用ACS控制器模块化方案,通过合理的系统配置和调试策略,实现了位置和速度的精确控制,以满足不断发展的多自由度,多约束加工和制造要求,进一步提高生产力,降低制造成本,增强企业的市场竞争力。
关键词:定位系统;ACS控制器;超精密1.引言在现代机械制造中,多轴运动控制已经越来越普遍,用电子方式来实现机械运动轴之间协调同步,取代了传统的机械凸轮和齿轮,给机械设计制造带来了巨大的灵活性,控制精度更高,动态性能更好,没有机械损耗,使维护变得方便而简单[1, 2]。
用户可以实现更加灵活的,模块化的机械结构。
与此同时,超精密运动控制技术是集控制技术,电子技术,数字技术,传感技术,驱动技术,测量技术及精密机械技术于一体的一门综合技术,是现代科技与工业发展的核心技术之一,长期以来受科研及高科技产业迅速发展的推动,对超精密运动控制技术的需求迅速增长。
目前,此技术已经被工业界所采用,比如超精密加工,微/纳米制造,包装等[3-6]。
除了需要达到高精度的跟踪误差外,还要求各轴在运动过程中按照既定的轨迹协同动作,以减少轴间的同步误差,提高系统的运动稳定性[7]。
比如X-Y绘图仪,为了绘制准确的二维图形,在X和Y方向上的运动就必须保持高度同步。
如果X轴在启动和停止时滞后于Y轴,其绘制的45度斜线将发生变形,如图1右所示。
图1 绘图仪绘制45度斜线然而,由于系统制造误差,负荷差异,摩擦力和其他干扰的存在,各运动轴的动态响应特性可能不一,所以在特定的领域如何减少同步误差是近二十年来学术界研究的热点之一[8]。
Observer based adaptive robust control of a class of nonlinear systems with dynamic uncerta
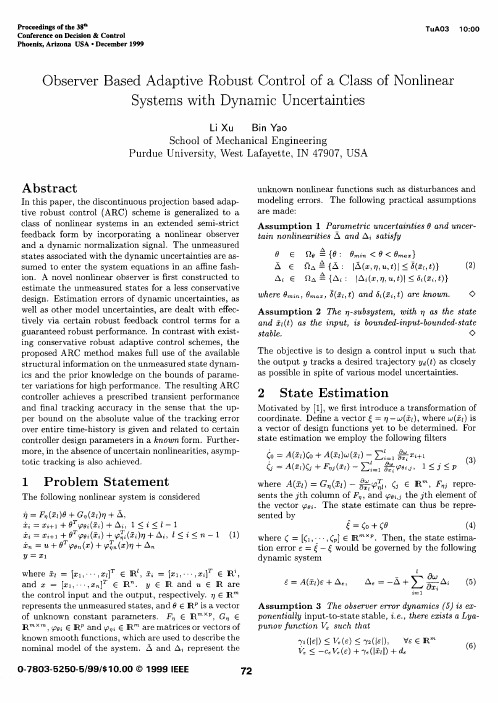
(2)
0
where &in,
b(?i, t) and & (zc~,t) are known.
Assumption 2 The q-subsystem, with 11 as the state and %:l(t) as the input, is bounded-input-bounded-state
i. ii.
A(Q’i.92
– @’@t
– @~C + Ai)
Z%atsz ~ o constant
(16)
The control law and parameter update law are summarized in the following. The detailed design procedure and the proof of theorem can be obtained from the authors. step 1< i <1 – 1. Let z, = z~ – a~_,, so(t) = yd(t), AI(z,t)= Al(z,t), @I(zI) WI(Z1),and recursively de= fine the following functions
Proceedings of the 38” Conference on Decision & Control Phoenix, Arizona USA December 1999
l
TuAO3
IO:00
Observer Based Adaptive Robust Control of a Class of Nonlinear Systems with Dynamic Uncertainties
Adaptive Robust Precision Motion Control of Linear Motors With Negligible Electrical Dynami

Adaptive Robust Precision Motion Control of Linear Motors With Negligible Electrical Dynamics:Theory and ExperimentsLi Xu and Bin Yao,Member,IEEEAbstract—Linear motors offer several advantages over their rotary counterparts in many applications requiring linear motion by eliminating mechanical transmission mechanisms.However, these advantages are obtained at the expense of added difficulties in controlling such a system.This paper studies the high perfor-mance robust motion control of an epoxy core linear motor,which has negligible electrical dynamics due to the fast response of the electrical subsystem.A discontinuous projection based adaptive robust controller(ARC)is first constructed.The controller theoretically guarantees a prescribed transient performance and final tracking accuracy in general,while achieving asymptotic tracking in the presence of parametric uncertainties.A desired compensation ARC scheme is then presented,in which the regressor is calculated using reference trajectory information only.The resulting controller has several implementation advan-tages such as less on-line computation time,reduced effect of measurement noise,a separation of robust control design from parameter adaptation,and a faster adaptation rate.Both schemes are implemented and compared on an epoxy core linear motor. Extensive comparative experimental results are presented to illustrate the effectiveness and the achievable control performance of the two ARC designs.Index Terms—Adaptive control,linear motors,motion control, precision manufacturing,robust control.I.I NTRODUCTIONM ODERN mechanical systems,such as semiconductor manufacturing equipment,often require high-speed/ high-accuracy linear motions.These linear motions are usually realized using rotary motors with mechanical transmission mechanisms such as reduction gears and lead screw.Such mechanical transmissions not only significantly reduce linear motion speed and dynamic response,but also introduce back-lash,large frictional and inertial loads,and structural flexibility. As an alternative,direct drive linear motors,which eliminate the use of mechanical transmissions,show promise for widespread use in high-speed/high-accuracy positioning systems[1]–[3]. Significant effort has been devoted to solving the difficul-ties in controlling linear motors[1],[2],[4],[5],[3],[6].EarlyManuscript received February2,2000;revised August3,2001.Recom-mended by Technical Editor K.Ohnishi.This work was supported in part by the National Science Foundation under the CAREER Grant CMS-9734345and in part by the Purdue Research Foundation.This work was presented in part at the2000American Control Conference,Chicago IL,June2000.The authors are with the School of Mechanical Engineering,Purdue Univer-sity,West Lafayette,IN47907USA(e-mail:byao@). Publisher Item Identifier S1083-4435(01)10732-5.work includestheII.P ROBLEM F ORMULATION AND D YNAMIC M ODELS The linear motor considered here is a current-controlled three-phase epoxy core motor driving a linear positioning stage supported by recirculating bearings.To fulfill the high performance requirements,the model is obtained to include most nonlinear effects like friction and ripple forces.In the derivation of the model,the current dynamics is neglected in comparison to the mechanical dynamics due to the much faster electric response.The mathematical model of the system can be described by the following equations:1represents the position of the inertiaload,is the normalized lumped effect of uncertainnonlinearities such asfrictionis an equivalent viscous friction coefficient of thesystem,are empirical parameters usedto describe the Stribeck effect.In practice,due to the inaccuracyof the positioning stage and ball bearings,the friction force mayalso depend onpositionis the position output,and rep-resents the lumped disturbance.Let be the reference motion trajectory.The objective isto synthesize a controlinputtracksas closely as possible in spite of various model uncertainties.III.A DAPTIVE R OBUST C ONTROL OF L INEAR M OTORSA.Design Models and AssumptionsIt is seen that the friction model(3)is discontinuousat.Thus,one cannot use this model for friction compensationsince there is no way that the motor can generate a discontin-uous motor force to accomplish the task.To bypass this tech-1Note that all terms are written in terms of the unit of input voltage.nical difficulty,a simple continuous friction model will be usedto approximate the actual discontinuous friction model(3)formodel compensation;the model used in the paper is givenby,,and the nominal value ofthe lumpeddisturbanceasas(6)(7)where(8)(9)where,,andfor two vectors is performed in terms of the correspondingelements of thevectors.and the estimation error(i.e.,).In view of(8),the following adaptation law withdiscontinuous projection modification can beused(10)where is a diagonalmatrix,is defined in[17],[18]asandandotherwise.(11)It can be shown[10]that for any adaptationfunction(13)where is the output trackingerror,is the desired trajectory to be trackedbyis small or converges to zero exponentially,thenthe output trackingerroras smallas possible.Differentiating (13)and noting (7),oneobtains(14)where(15)whereis the adjustable model compensation needed for achieving perfect tracking,and(17)whereis used to stabilize the nominal system,and is a robust feedback term used to attenuate the effect of model uncertainties as follows.Noting Assumption 1and P1of (12),there existsasuch that the following two conditions aresatisfied:is a design parameter which can be arbitrarily small.Es-sentially,i of (18)showsthatis synthesized to dominate the model uncertainties coming from both parametric uncertainties and uncertain nonlinearities ,and ii of (18)is to make surethatis dissipating in nature so that it does not interfere with the functionality of the adaptive controlpart .Theorem 1:If the adaptation function in (10)is chosenas.B)If after a finite time ,there exist parametric uncer-tainties only(i.e.,,),then,in addition to results in A),zero final tracking error is also achieved,i.e.,be any smooth functionsatisfyingsatisfying (18)is givenbycan be worked outin the same way as in[10]–[12].],can be generated using a filter.Forexample,can begenerated by the following third order stablesystem,IV .D ESIRED C OMPENSATION ARC (DCARC)In the ARC design presented in Section III,theregressor(19)depends onstatehas to becalculated on-line based on the actual measurement of the ve-locity .Thus,the effect of measurement noise may be severe,and a slow adaptation rate may have to be used,which in turn reduces the effect of parameter adaptation.Second,despite thatthe intention ofintroducingis for model compensation,be-causeofdepends on the actual feedback of the state also.Although theoretically the effect of this added implicit feedback loop has been considered in the robust control law design as seen from condition i)of (18),practically,there still exists cer-tain interactions between the modelcompensation and the ro-bustcontrolsubstituted by the desiredregressor(25)where,oneobtains(26)Comparing (26)with (16),it can be seen that two additional terms (underbraced)appear,which may demand a strengthenedrobust controlfunction(27)wherebeing a nonlinear gain large enough such that thematrixis required to satisfy the following constrains similar to(18):can be obtained using the techniques inRemark1.For example,similar to(23),this function is chosenas(33)Theorem2:If the DCARC law(25)is applied,thenA)In general,all signals are bounded.Furthermore,thepositive definitefunction(34)is bounded aboveby.B)If after a finite time,there exist parametric uncer-tainties only(i.e.,,),then,in addition toresults in A),zero final tracking error is also achieved,i.e.,Remark5:It is noted that the condition on the selection ofrobust feedbackgain is much less restrictive than those in[14],[13].This is achieved by judiciously selecting a p.d.func-tion given by(34)instead of the general formulations in[14],[13].represent the samplinginstance,th diagonalelementof bethegiven by(25)is(37)Since depends on the reference trajectory only and itsderivative can be pre-computed,one can integrate(37)by parts toobtainV.C OMPARATIVE E XPERIMENTSA.Experiment SetupThe experimental set-up shown in Fig.1consists of fourmajor components:aprecision–-axis on topof-axis is used.Standard least-square identificationis performed to obtain the parameters oftheFig.1.Experimental setup.nominal valuesof(V/m/s(V/m/sand.B.Performance IndexAs in [14],[8],the following performance indexes will be used to measure the quality of each control algorithm:I1),where,the maximal absolute value of the trackingerror,is used as an index of measure of transient performance ;I3).,the maximal absolute value of the tracking error during the last two seconds,is used as an index of measure of final tracking accuracy ;I4),the normalized control vari-ations,is used to measure the degree of control chattering ,where-axis.The controlsystem is implemented using a dSPACE DS1103controller board.The controller executes programs at a sampling fre-quencykHz,which results in a velocity measurement resolution of 0.0025m/s.The robust controltermsis defined in (22).Then,satisfies (17)and (18).Similarly,choose a feedbackgain large enough suchthat(40)where(41)As before,the following variables are available (ei-ther measured or computed)for controlimplementation:,and .Suppose that the parametersof (14)are known,the control objective can be achieved with the following PID control law with modelcompensation(45)whereTABLE IP ERFORMANCE I NDEXES FOR S INUSOIDAL TRAJECTORYthree closed-loop polesat.The continuousfunction,.DRC :Deterministic Robust Control—The same control law as the above ARC but without using parameter adaptation,i.e.,lettingand:Comparative experiments are run for tracking asinusoidal trajectory.The desiredtrajectory,,and.Set 2:To test the performance robustness of the algorithmsto parameter variations,the 20lb.payload is removed,which is equivalentto.Set 3:A large step disturbance (a simulated 0.5V electricalsignal)is addedarounds and removedaround s to test the performance robustness of eachcontroller to disturbance.The experimental results in terms of performance indexes are given in Table I.As seen from the table,in terms ofperformanceindexesand ,PID performs poorly for all three sets,but with a slightly lesser degree of control input chattering.One may argue that the performance of PID control can be further improved by increasing the feedback gains.However,in practice,feedback gains have upperlimitsFig.2.Tracking errors for sinusoidal trajectory with load.because the bandwidth of every physical system is finite.To verify this claim,the closed-loop poles of PID controller areplaced at300,which is translated into PID gainsof,can be used for ARC,which reduces the effect of param-eter adaptation.When we tried to increase the adaptation rate for ARC further,the system is subjected to quite severe control chattering due to the measurement noises (especially velocity feedback).Comparatively,due to the use of desired compen-sation structure and the free-velocity-feedback implementation of parameter adaptation law presented in Remark 6,DCARC is not so sensitive to velocity measurement noise.In return,a larger adaptation rate can be used for DCARC and the param-eter adaptation algorithm of DCARC is able to pick up the ac-tual value of the inertial load more quickly,which can be seen from the parameter estimates shown in Figs.3and 4for ARC and DCARC,respectively.The control inputs of the three con-trollers are given in Fig.5.As expected,all controllers use al-most the same amount of control effort,and ARC has a larger degree of control input chattering than DCARC.For Set 2,the tracking errors are given in Fig.6.It shows that both ARC and DCARC achieve good tracking performance in spite of the change of inertia load.Again,DCARC has the bestperformance in termsof,,and .The tracking errors for Set 3are given in Fig.7.As seen from the figures,the added large disturbance does not affect the per-formance of ARC and DCARC much,except for the spike when the sudden change of the disturbance occurs.This result illus-trates the performance robustness of ARC and DCARC designs.Again,DCARC performs best in termsof,,and .Case 2—High-Acceleration/High-Speed Point-to-Point Mo-tion Trajectory (Without Load):A fast point-to-point desiredFig.3.Parameter estimation ofARC.Fig.4.Parameter estimation ofDCARC.Fig.5.Control inputs for sinusoidal trajectory withload.Fig.6.Tracking errors for sinusoidal trajectory without load.motion trajectory with high-acceleration/deceleration,which runs back and forth several times,is shown in Fig.8.Thetrajectory has a maximum velocityofm/s anda Fig.7.Tracking errors for sinusoidal trajectory withdisturbance.Fig.8.Point-to-point motiontrajectory.Fig.9.Tracking errors for point-to-point motion trajectory.maximum accelerationof .The tracking errors of PID,DRC and DCARC are shown in Fig.9.As seen,the proposed DCARC has a much better performance than PID and DRC.Furthermore,during the zero velocity portion of motion,the tracking error iswithinis and/or the larger the feedbackgainFig.10.Tracking errors of DCARC controllers for sinusoidal trajectory with disturbance.previous experiments,a constant lumped robust feedback gain(for DCARC)has been used to have a simple implementation.However,such a simplification has the limita-tion that the lumped robust feedback gain cannot be too large since otherwise the resulting control input may chatter severely due to the amplification of measurement noises.To bypass this implementation problem,in the following,a nonlinear lumped robust feedback gain will be ly,during the nominal tracking period when the tracking error is small,the same level of lumped robust feedback gain as in previous experiments will be used to minimize the effect of measurement noise to keep the control input chattering at a reasonable level.During the tran-sient period when the tracking error is large,a larger lumped robust feedback gain will be used to provide a stronger instan-taneous feedback for a better transient tracking performance.Since the proposed ARC strategy is able to use fast adaptation and the transient period is quite short as seen from previous ex-periments,the increase of control chattering due to the increased feedback gain in the transient may not be noticeable.To illus-trate this nonlinear robust feedback gain design concept,in the following,the DCARC controller is used as an example to see the benefit of using such a nonlinear gain.Choose a nonlineargain large enough suchthat(46)whereand,,wheneversand s).At the same time,the degree of con-trol chattering is kept almost at the same level.The tracking er-rors of tracking the point-to-point motion trajectory is shownin Fig.11.Again,the DCARC with the nonlinear robustgainFig.11.Tracking errors of DCARC controllers for point-to-point motion.achieves a better tracing performance than the DCARC with a constant feedback gain.VI.C ONCLUSIONSIn this paper,an ARC controller and a desired compensation ARC controller have been developed for high performance ro-bust motion control of linear motors with negligible electrical dynamics.The proposed controllers take into account the ef-fect of model uncertainties coming from the inertia load,fric-tion force,force ripple and external disturbances.Theoretically,the resulting controllers guarantee a prescribed transient perfor-mance and final tracking accuracy in general while achieving asymptotic tracking in the presence of parametric uncertain-ties.Furthermore,it is shown that the desired compensation ARC scheme,in which the regressor is calculated using refer-ence trajectory information only,offers several implementation advantages such as less on-line computation time,reduced ef-fect of measurement noise,a separation of robust control de-sign from parameter adaptation,and a faster adaptation rate in parative experimental results are obtained for the motion control of an epoxy core linear motor.Exper-imental results illustrate the high-performance of the proposed ARC strategies and show the advantages and drawbacks of each method.A PPENDIX IProof of Theorem 1:From (16)and (17),the derivativeof(48)which leads to (21)and thus proves the results in A)of Theorem1.Now consider the situation in B)of Theorem 1,i.e.,,.Choose a p.d.function(50)Therefore,is uniformly continuous.By Barbalat’s lemma,A PPENDIX IIProof of Theorem2:Along the trajectory of(26),the time derivative of(51)andgiven by(29)is p.d.,then,thederivative of(55)which leads to(35)and the results in A)of Theorem2is proved. Now consider the situation in B)of Theorem2,i.e.,,.Choose a p.d.function(57)where.Therefore,andR EFERENCES[1] D.M.Alter and T.C.Tsao,“Control of linear motors for machine toolfeed drives:Design and implementation of h。
未知环境下柔性二连杆机械臂的自适应阻抗控制

(3)分析了外扰力幅值变化下两自由度轧机辊系的分岔特性,得到了轧机工作辊及支承辊出现周期运动㊁倍周期运动以及混沌运动的条件,发现外扰幅值的变化会使轧机辊系出现不同的运动状态,为进一步抑制轧机振动提供了理论参考㊂参考文献:[1] S u n J i a n l i a n g,P e n g Y a n,L i u H o n g m i n.N o n l i n e a rV i b r a t i o na n dS t a b i l i t y o fM o v i n g S t r i p w i t hT i m e‐d e p e n d e n tT e n s i o n i nR o l l i n g P r o c e s s[J].J o u r n a l o fI r o na n d S t e e l R e s e a r c h(I n t e r n a t i o n a l),2010,17(6):11‐15.[2] S h i P e i m i n g,L i J i z h a o,J i a n g J i n s h u i,e t a l.N o n l i n-e a r D y n a m i c s of T o r s i o n a l V i b r a t i o n f o r R o l l i n gM i l l’sM a i nD r i v eS y s t e mu n d e rP a r a m e t r i cE x c i t a-t i o n[J].J o u r n a l o f I r o na n dS t e e lR e s e a r c h(I n t e r-n a t i o n a l),2013,20(1):7‐12.[3] S w i a t o n i o w s k iA,B a rA.P a r a m e t r i c a lE x c i t e m e n tV i b r a t i o ni n T a n d e m M i l l s M a t h e m a t i c a l M o d e la n d I t sA n a l y s i s[J].J o u r n a l o fM a t e r i a l sP r o c e s s i n gT e c h n o l o g y,2009,134(2):214‐224. [4] 孟令启,徐如松,王建勋,等.中厚板轧机非线性参激的振动[J].重庆大学学报,2008,31(4):393‐397.M e n g L i n g q i,X u R u s o n g,W a n g J i a n x u n,e ta l.P a r a m e t r i c a l l y E x c i t e dN o n l i n e a rV i b r a t i o n so fM e-d i u m a n d He a v y P l a t e M i l l i n g[J].J o u r n a l o fC h o n g q i n g U n i v e r s i t y,2008,31(4):393‐397.[5] 侯东晓,刘彬,时培明.分段非线性轧机辊系系统的分岔行为研究[J].振动与冲击,2010,29(12): 132‐135.H o uD o n g x i a o,L i uB i n,S h i P e i m i n g.R e s e a r c ho nS e g m e n t e d N o n l i n e a r B i f u r c a t i o n C h a r a c t e r i s t i c o fR o l l i n g M i l l[J].J o u r n a lo f V i b r a t i o na n dS h o c k, 2010,29(12):132‐135.[6] 刘浩然,侯东晓,时培明,等.轧机辊系滞后非线性垂直振动系统的振动特性[J].机械工程学报, 2011,47(13):65‐71.L i uH a o r a n,H o uD o n g x i a o,S h i P e i m i n g,e t a l.V i-b r a t i o nC h a r ac t e r i s t i c s o fH y s t e r e t i cN o n l i n e a rV e r-t i c a lV i b r a t i o nS y s t e m o fR o l l i n g M i l lR o l l e r[J].J o u r n a l o fM e c h a n i c a lE n g i n e e r i n g,2011,47(13): 65‐71.[7] 白金兰,王军生,王国栋,等.提高冷轧过程控制轧制力模型的设定精度[J].钢铁研究学报,2006(3): 21‐25.B a i J i n l a n,W a n g J u n s h e n g,W a n g G u o d o n g,e t a l.I m p r o v e m e n t i nS e t t i n g A c c u r a c y o fR o l l i n g F o r c eM o d e l d u r i n g P r o c e s sC o n t r o l o fC o l dR o l l i n g[J].J o u r n a l o f I r o na n dS t e e lR e s e a r c h,2006(3):21‐25.[8] 孙一康.带钢冷连轧计算机控制[M].北京:冶金工业出版社,2002.[9] 杨志芬.带钢冷轧机轧制力模型研究及应用[D].秦皇岛:燕山大学,2011.[10] T a m i y aT,F u r u iK,L i d a H,e ta l.A n a l y s i so fC h a t t e r i n g P h e n o m e n o ni nC o l d R o l l i n g[C]//I n-t e r n a t i o n a lC o n f e r e n c eo nS t e e lR o l l i n g,V o l.I I,S c i e n c e a n d T e c h n o l o g y o fF l a tR o l l e dP r o d u c t s.T o k y o,1980:1191‐1202.(编辑 陈 勇)作者简介:刘 彬,男,1953年生㊂燕山大学电气工程学院教授㊁博士㊂主要研究方向为机械非线性振动动力学及测量技术㊂赵红旭,男,1989年生㊂燕山大学电气工程学院硕士研究生㊂朱 月,男,1988年生㊂燕山大学电气工程学院硕士研究生㊂刘浩然,男,1980年生㊂燕山大学电气工程学院副教授㊁博士㊂侯东晓(通信作者),男,1982年生㊂东北大学秦皇岛分校控制工程学院讲师㊁博士㊂(上接第2319页)[14] 詹沙磊,刘南.基于模糊目标规划的应急物流多目标随机规划模型[J].中国机械工程,2011,22(23):2858‐2862.Z h a nS h a l e i,L i uN a n.A M u l t i‐o b j e c t i v eS t o c h a s-t i cP r o g r a mm i n g M o d e lf o rE m e r g e n c y L o g i s t i c sB a s e do nF u z z y G o a l P r o g r a mm i n g[J].C h i n aM e-c h a n i c a l E n g i n e e r i n g,2011,22(23):2858‐2862.[15] 闻博,李宏光.含分段线性隶属函数的模糊规划建模方法[J].化工学报,2010,61(8):2149‐2153.W e nB o,L i H o n g g u a n g.M o d e l i n g M e t h o d o l o g yf o r F u z z y P r og r a mm i n g w i th Pi e c e w i s e L i n e a rM e m b e r s h i p F u n c t i o n s[J].J o u r n a lo f C h e m i c a lI n d u s t r y a n d E n g i n e e r i n g,2010,61(8):2149‐2153.[16] M a n u e lD M,D a v i dP,P a n d i a nV.V e n d o rS e l e c-t i o nP r o b l e mb y U s i n g a n I n t e r a c t i v eF u z z y M u l t i‐o b j e c t i v eA p p r o a c h w i t h M o d i f i e dS‐c u r v e M e m-b e r s h i p F u nc t i o n s[J].C o m p u t e r sa nd M a t he m a t-i c sw i t hA p p l i c a t i o n s,2010,60(4):1038‐1048.(编辑 王艳丽)作者简介:彭安华,男,1973年生㊂中国矿业大学机电工程学院博士研究生,淮海工学院工程训练中心副教授㊂主要研究方向为模糊度准则决策及其在先进制造技术中的应用㊂获中国专利3项㊂发表论文近50篇㊂肖兴明,男,1962年生㊂中国矿业大学机电工程学院教授㊁博士研究生导师㊂岳 睿,男,1966年生㊂中国矿业大学机电工程学院博士研究生㊂㊃0532㊃中国机械工程第25卷第17期2014年9月上半月Copyright©博看网. All Rights Reserved.未知环境下柔性二连杆机械臂的自适应阻抗控制金英连 王斌锐 方水光中国计量学院,杭州,310018摘要:针对机器人与环境接触作业的需求,基于假设模态法建立了刚柔耦合动力学模型,并考虑环境参数不精确干扰,设计了自适应阻抗控制器,分析了其稳定条件㊂在MA T L A B /S i m u l i n k 中搭建了可对柔性机械臂进行自适应阻抗控制的仿真平台,计算了平面内二连杆柔性机械臂在含有三角形凹陷环境表面的接触运动和柔性变形模态㊂对比分析了刚性和柔性机械臂位控和力控效果及自适应项对控制响应的影响㊂基于S t a u b l i 机器人展开了实验㊂结果表明:柔性变形会使得机械臂的位控和力控效果变差;自适应阻抗控制会改善控制响应,对环境不确定具有鲁棒性㊂设计的自适应阻抗控制可实现柔性机械臂在不规则表面的稳定接触和运动㊂关键词:机械臂;刚柔耦合;动力学仿真;自适应阻抗控制;模态中图分类号:T P 242 D O I :10.3969/j.i s s n .1004-132X.2014.17.015A d a p t i v e I m p e n d e n c eC o n t r o l o fT w o ‐l i n k s F l e x i b l eM a n i pu l a t o r u n d e rU n k n o w nE n v i r o n m e n t s J i nY i n g l i a n W a n g B i n r u i F a n g S h u i g u a n gC h i n a J i l i a n g U n i v e r s i t y ,H a n gz h o u ,310018A b s t r a c t :F o r t h e o p e r a t i o n c o n t a c t i n g w i t ho b j e c t s ,a r i g i d ‐f l e x i b l e c o u p l i n g d yn a m i c sm o d e l w a s e s t a b l i s h e db a s e do n t h e a s s u m e dm o d e a p p r o a c h f o r t w o ‐l i n k f l e x i b l em a n i p u l a t o r s .A na d a p t i v e i m -p e n d e n c e s c h e m ew a s d e s i g n e d t o c o m p e n s a t e t h e e n v i r o n m e n t u n c e r t a i n t i e s a n d t h e s t a b i l i t y co n d i t i o n w a s a n a l y z e d .T h ec o n t r o l s i m u l a t i o n p l a t f o r m w a se s t a b l i s h e du s i n g MA T L A B /S i m u l i n k .T h et i pm o t i o na n dd e f o r m a t i o nm o d ew e r e c o m p u t e du n d e rm a n i p u l a t o r s l i d i n g o n t h e s u r f a c ew i t h t h e t r i a n -g u l a r c r a c k .P e r f o r m a n c e s o f p o s i t i o na n d f o r c e c o n t r o lw e r e c o m p a r e db e t w e e n t h e f l e x i b l e a n d r i gi d m a n i p u l a t o r ,a n d p e r f o r m a n c e s o f a d a p t i v e i m p e d a n c e c o n t r o l w e r e a n a l y z e d .T h e p h y s i c a l e x pe r i m e n t w a s c o n d u c t e do nS t a u b l i r o b o t .T h e r e s u l t s s h o wt h ef l e x i b l e d e f o r m a t i o nd e c r e a s e s t h e p o s i t i o n a n df o r c e c o n t r o l p r e c i s i o n ,a n d t h e a d a p t i v e i m p e d a n c e p r o p o s e d c a n i n c r e a s e t h e r e s p o n s e t i m e .A d a pt i v e i m p e d a n c e i s r o b u s t t o t h e e n v i r o n m e n t p o s i t i o nu n c e r t a i n t i e s a n d c a n r e a l i z e t h e f l e x i b l em a n i p u l a t o r s t a b l y c o n t a c t i n g a n dm o v i n g on t h en o t ‐f l a t s u r f a c e .K e y w o r d s :m a n i p u l a t o r ;r i g i d ‐f l e x i b l e c o u p l i n g ;d y n a m i c s s i m u l a t i o n ;a d a p t i v e i m p e n d e n c e c o n -t r o l;m o d e 收稿日期:2013 04 22基金项目:国家自然科学基金资助项目(50905170);浙江省自然科学基金资助项目(L Q 13E 050004,L Y 14F 030021);质检公益性行业科研专项(201210076)0 引言由于操作对象的特殊性,所以要求与环境接触的作业机器人需具有一定的柔顺性㊂此种机器人的设计较多采用轻质材料以减小惯性或在关节处添加弹簧阻尼调节器㊂机器人可通过力矩传感器检测关节力矩,当力矩过大时启动急停措施[1]㊂急停虽能降低误操作损害,但当机器人和环境障碍物发生接触碰撞时,机械臂的惯性还是会对机械臂和接触物体造成一定程度的破坏㊂阻抗控制方法是实现机器人主动柔顺控制最为有效的方法之一[2],该方法是由H o g a n [3]在1987年提出的㊂后期许多学者在此基础上基于积分流形[4]㊁奇异摄动法[5]发展了众多的阻抗控制方法㊂阻抗控制方法相比力位混合控制方法具有鲁棒性好㊁动作规划较少等优点[6]㊂阻抗控制可分为基于动力学模型的方法[7]和基于位置的方法[8]㊂基于动力学模型的方法需知机器人的精确模型,而不需要检测力的变化,但机器人的精确模型通常难以得到;基于位置的阻抗控制方法需知环境位置的精确信息,而由于测量误差,环境位置往往不能精确得到㊂考虑到机器人模型的不精确,自适应方法被应用到阻抗控制中[9]㊂机器人自适应阻抗控制多采用刚体模型进行研究㊂文献[9]虽考虑了关节柔性,但忽略了机器人的材质柔性影响㊂而实际情况是,机器人材质柔性在一些精度较高的场合不能被忽略㊂本文在综合考虑机械臂材质柔性㊁环境未知和动力学模型不精确等情况下,设计了自适应阻抗算法㊂本文首先建立柔性机器人动力学模型,并针对接触运动设计了自适应阻抗控制算法,分析了稳定条件;而后利用S i m u l i n k 搭建了不规则环境下可对柔性机械臂进行自适应阻抗控制的仿真平台;通㊃1532㊃未知环境下柔性二连杆机械臂的自适应阻抗控制金英连 王斌锐 方水光Copyright ©博看网. All Rights Reserved.过仿真对比分析了三角形凹陷对柔性机器人一阶模态的影响,刚性和柔性机器人在自适应阻抗控制下位控和力控效果,以及自适应项对机器人位控和力控效果的影响㊂最后给出了实验结果㊂1 柔性机器人自适应阻抗控制器设计1.1 机械臂的阻抗控制阻抗控制的实质是调节机械臂末端接触力与末端位置两者之间的关系㊂借鉴文献[10],C a r -t e s i a n 坐标系下的机器人目标阻抗模型为M d ~X ¨+C d~X ㊃+K d ~X =F e(1)~X=X d -X 式中,~X 为机械臂末端位置误差;X d ㊁X 分别为期望位置(当目标为与环境发生接触时,环境位置就是期望位置)和实际位置;F e 为作用在机械臂末端的接触力;M d ㊁C d ㊁K d 分别为机械臂的目标惯性矩阵㊁阻尼矩阵㊁刚度矩阵㊂式(1)表明阻抗控制需已知环境位置,而实际中往往很难得到精确的环境位置;为实现高精度的位置控制,要求机械臂自身的阻抗参数比较大,而这样会导致机械臂与障碍物接触力过大[11]㊂根据接触环境设定新的阻抗参数,既可以实现精确的位置控制,又可以减小机械臂和接触物的损伤㊂1.2 自适应阻抗控制器设计考虑柔性处理后机器人运动更符合实际情况,但柔性机器人动力学方程具有非线性㊁多变量㊁强耦合等特点,不利于控制[12],所以采用模态假设法对动力学方程进行解耦,建立解耦后柔性连杆机械臂动力学方程如下:M (1×1)11M (1×2)12M (1×2)13M (2×1)21M (2×2)22M (2×2)éëêêùûúú23θ¨(1×1)q ¨(2×1)1q ¨(2×1)éëêêêêùûúúúú2+2θ㊃0(1×1)0(1×2)0(1×2)0(2×1)C (2×2)1C (2×2)éëêêùûúú2θ㊃(1×1)q ㊃(2×1)1q ㊃(2×1)éëêêêêùûúúúú2+0(1×1)0(1×2)0(1×2)0(2×1)K (2×2)1K (2×2)éëêêùûúú2θ(1×1)q (2×1)1q (2×1)éëêêêùûúúú2=τ(1×1)0(2×1)0(2×1éëêêêùûúúú)(2)其中,τ为连杆所受到的外加转动力矩,θ为连杆的广义转角,q 1=[q 11 q12]T为连杆的径向模态(取前两阶模态),q 2=[q 21 q22]T为轴向模态,q 1㊁q 2为时间t 的函数,M (I)i j (i =1,2,j =1,2,3)㊁C (J )i (i =1,2)㊁K (K )i(i =1,2)分别为广义质量矩阵㊁阻尼矩阵㊁刚度矩阵中的元素,上标表示维数㊂式(2)可简写成矩阵形式M p ¨+C p ㊃+K p =τ(3)式中,p =(θ,q 1,q 2)T为广义坐标;M ㊁C ㊁K ㊁τ分别为广义质量矩阵㊁阻尼矩阵㊁刚度矩阵和关节总力矩矩阵㊂柔性机器人关节所受总力矩为τ=τt -τe -τf(4)τe =J TF e(5)式中,τt 为关节力矩;τf 为摩擦力矩;τe 为环境接触力矩;J 为J a c o b i a n 矩阵㊂考虑发生接触时,用实际环境位置X e 代替式(1)中的X d ,且在式(1)右端减去末端期望接触力F d ,可得柔顺接触阻抗模型M d E ¨+C dE ㊃+K d E =F e -F d (6)E =X e -X将环境建模为弹性体,则F e =-K e E式中,K e 为环境刚度矩阵㊂由求解微分方程(式(6))可得,当控制接触力达到F e =F d 时,为满足理想稳定状态,力控方向上刚度应满足K d =0㊂当忽略摩擦力时,接触力方向与接触面法线方向相同㊂假设机械臂末端只在x 方向受力,并用矩阵小写符号表示矩阵对应x 方向的元素,对于机器人自由空间和接触空间,式(6)可分别写成m d e ¨+c d e ㊃+k d e =-f d (自由空间)(7)m d e ¨+c d e ㊃=f e -f d (接触空间)(8)由于环境未知,实际环境位置x e 很难预先得到精确值,往往只能获得估计值㊂用估计的环境位置x 'e 代替实际环境位置x e ,定义e '=x 'e-x ,则m d e ¨'+c d e ㊃'+k d e '=-f d (自由空间)(9)m d e ¨'+c d e ㊃'=f e -f d (接触空间)(10)为保证在有位置估计误差下实现稳定的接触力,在式(10)中增加自适应调整项Ω(t),可得m d e ¨'+c d (e ㊃'+Ω(t ))=f e -f d(11)为保证式(11)中的误差收敛,自适应调整项需根据接触力误差进行调节㊂本文采用自学习方法,设计Ω(t)为Ω(t )=Ω(t -λ)+η[fd (t -λ)-fe (t -λ)](12)式中,λ为控制器采样周期;η为学习率㊂将式(12)代入式(11),求L a pl a c e 变换,可建立位置误差和接触力误差的传递函数㊂根据二阶时滞系统稳定条件及其T a y l o r 级数展开,可得λ≪1 0<η<λc dλ+m d 式(3)中的实际质量矩阵㊁阻尼矩阵㊁刚度矩阵中的参数很难精确获得,即动力学模型不精确,模型参数需采用估计值,则式(3)变为㊃2532㊃中国机械工程第25卷第17期2014年9月上半月Copyright ©博看网. All Rights Reserved.^M p¨+H =τ(13)H =(M -^M )p ¨+C p ㊃+K p =τ-^M p¨(14)式中,^M 为M 矩阵的估计值㊂进一步取H 的估计值为^H =H (t -λ)=τ(t -λ)-^M p¨(t -λ)并将其代入到式(13)中,可得^M p¨+^H =τ(15)将式(15)代入式(9)和式(11),并拓展到整个C a r t e s i a n 空间,可推导出柔性机器人自适应阻抗控制算法为τ=^M J -1(U -J ㊃p ㊃)+τ(t -λ)-^M p¨(t -λ)(16)U =X ¨'e +M -1d (F d +C dE ㊃'+K d E ') 自由空间X ¨'e +M -1d [F d -F e +C d (E ㊃'+Ω)]{接触空间(17)2 仿真与实验2.1 仿真平台对由两相同的匀质细长连杆(为凸显柔性)组成的机械臂进行水平面运动仿真,并忽略摩擦力㊂机械臂物理参数如表1所示㊂机械臂运动情况如图1所示㊂实际环境位置在x 方向0.8m 处,估计环境位置为x 方向0.83m 处,大于实际环境位置,从而保证机械臂表1 匀质连杆的物理参数参数数值参数数值长度(m )1.0横截面积(m2)3.7×10-4质量(k g )1.0弹性模量(G P a)71密度(k g/m 3)2.7×103惯性矩(m 4)1.0×10-8末端与环境接触[13]㊂为验证自适应阻抗控制算法对未知环境位置的自适应能力,实际环境具有三角形凹陷㊂控制目标为机械臂从自由空间开始运动,到达约束空间后,与环境发生接触碰撞,且一直保持接触滑动㊂图1 机器人运动示意连杆1与x 正向的夹角为θ1,连杆2相对于连杆1的夹角为θ2,逆时针为正㊂利用MA T -L A B /S i m u l i n k 搭建柔性机器人自适应阻抗控制仿真平台,如图2所示㊂图2 柔性机械臂自适应阻抗控制仿真采用MA T L A B 中提供的基于R u n ge ‐K u t t a 算法的变步长o d e 45微分方程求解函数,仿真时长为3s ㊂机械臂初始位置分别为θ1=π2,θ2=-π3㊂x 方向的环境刚度k e =4000N /m ,y 方向环境刚度为0,x 方向的期望接触力f d =10N ,忽略摩擦力㊂仿真中,只考虑径向模态q 1,忽略轴向模态q 2,并设定连杆平面内转动的动力学和目标阻抗参数为^M =0.1000.éëêêùûúú1 M d =I C d =2000éëêêùûúú050K d =00éëêêùûúú0625 λ=0.0005 η=0.01C -1d 2.2 柔性和刚性机械臂自适应阻抗控制对比自适应阻抗控制下,机械臂从自由空间开始运动,在0.1s 左右,x ≥x e (实际应用中,由于x e 未知,可用力传感器来检测机器人末端是否与环境接触),机械臂与环境接触,到达约束空间,开始自适应阻抗控制;在0.6s 左右,进入三角形凹陷区;在1.4s 左右,到达三角形的顶点;在2.2s 左右,离开三角形凹陷区㊂仿真表明机械臂末端与环境始终保持接触状态㊂柔性机械臂末端径向振动一阶模态如图3所示㊂由图3可见,柔性机械臂的一阶振动模态坐标最大可达0.081m ,因此忽略机械臂的柔性将㊃3532㊃未知环境下柔性二连杆机械臂的自适应阻抗控制金英连 王斌锐 方水光Copyright ©博看网. All Rights Reserved.会带来较大的误差㊂机械臂从自由空间运动到约束空间后,由于接触作用,柔性变形大幅减弱到-0.02~0.02m 内,且一阶模态坐标趋于零,柔性对机械臂运动影响降低;机械臂末端在遇到环境三角形凹陷时,一阶模态有小幅变化㊂图3 机械臂一阶模态坐标柔性和刚性机械臂位控和力控效果对比如图4所示㊂由图4b 可见,柔性机械臂与环境的接触碰撞力峰值小于刚性机械臂与环境的接触碰撞力峰值;但由于柔性机械臂存在横向弹性变形,柔性机械臂的位置和接触力仿真曲线均在刚性机械臂曲线上下振动㊂在0.32s 时,刚性机械臂末端接触力已经能稳定在期望的接触力(10N )附近;而柔性机械臂末端接触力达到稳定在期望接触力附近所需时间延长㊂刚性机械臂接触力经过三角形凹陷后,逐渐趋于期望的稳定状态;柔性机械臂接触力则存在振动㊂由于柔性变形,柔性机械臂末端的位移和接触力有振荡,但其均值与刚性机械臂一致㊂(a)末端位控效果(b)末端力控效果图4 机械臂末端力控和位控效果2.3 自适应项对位控和力控的影响本文进一步对比分析了有无自适应项情况下,柔性机械臂阻抗控制的力控和位控效果㊂为便于比较,对柔性机械臂末端的位移和接触力作均值滤波处理㊂对比结果如图5所示㊂(a)位控效果(b)力控效果图5 阻抗控制和自适应阻抗控制的位控与力控效果由图5a 可见,两种控制对理想平面环境的位控效果相差不大;但对于环境的三角形凹陷,自适应阻抗控制算法下的位置波形相位超前于阻抗控制算法下的位置波形相位,所以自适应阻抗控制对环境位置变形的响应快于阻抗控制对环境位置变形的响应㊂由图5b 可见,阻抗控制下,机械臂在遇到三角形凹陷的第一个折点后力稳定在6.25N 左右,误差为37.5%;遇到第二个折点后力维持在17.28N 左右,误差为72.8%;自适应阻抗控制下,机械臂在遇到三角形凹陷的折点后,每次都能较快地稳定在期望力10N 左右㊂说明自适应阻抗控制较单纯的阻抗控制其力控响应快,但折点处接触力超调较大㊂2.4 实验采用S t a u b l iR X 90工业机器人进行了实验研究,实验环境和力控效果如图6所示㊂由图6可见,本文设计的自适应阻抗控制在实物实验中也能将接触力控制在理想值附近㊂实际得到的接触力有波动,波动大小与实际存在的摩擦力㊁细长铝杆的柔性变形以及压力传感器的测量误差有关㊂3 结论(1)设计的自适应阻抗控制算法可实现机械臂在不规则环境表面的稳定接触和运动㊂(2)柔性变形对精确的位置控制不可被忽略,且会导致柔性机械臂末端接触力波动比刚性机械臂剧烈,使得柔性机械臂的位控和力控效果变差㊂㊃4532㊃中国机械工程第25卷第17期2014年9月上半月Copyright ©博看网. All Rights Reserved.。
ardc控制算法
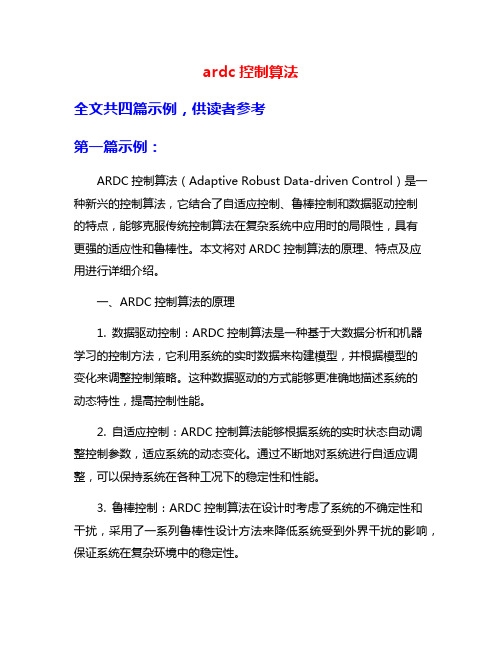
ardc控制算法全文共四篇示例,供读者参考第一篇示例:ARDC控制算法(Adaptive Robust Data-driven Control)是一种新兴的控制算法,它结合了自适应控制、鲁棒控制和数据驱动控制的特点,能够克服传统控制算法在复杂系统中应用时的局限性,具有更强的适应性和鲁棒性。
本文将对ARDC控制算法的原理、特点及应用进行详细介绍。
一、ARDC控制算法的原理1. 数据驱动控制:ARDC控制算法是一种基于大数据分析和机器学习的控制方法,它利用系统的实时数据来构建模型,并根据模型的变化来调整控制策略。
这种数据驱动的方式能够更准确地描述系统的动态特性,提高控制性能。
2. 自适应控制:ARDC控制算法能够根据系统的实时状态自动调整控制参数,适应系统的动态变化。
通过不断地对系统进行自适应调整,可以保持系统在各种工况下的稳定性和性能。
3. 鲁棒控制:ARDC控制算法在设计时考虑了系统的不确定性和干扰,采用了一系列鲁棒性设计方法来降低系统受到外界干扰的影响,保证系统在复杂环境中的稳定性。
3. 易实现:ARDC控制算法结合了数据驱动技术和传统控制理论,使得控制器的设计和实现更加简单和直观。
只需通过系统的实时数据来进行控制,无需对系统进行复杂的数学建模。
4. 广泛应用:ARDC控制算法适用于各种复杂系统的控制,包括机械系统、电气系统、化工系统等。
在实际工程中已经得到了广泛的应用和验证。
1. 工业过程控制:ARDC控制算法可以应用于各种工业过程控制系统中,能够实现对生产过程的精确控制和优化,提高生产效率和质量。
ARDC控制算法是一种具有很高潜力的控制算法,它结合了自适应、鲁棒和数据驱动的特点,能够适应各种复杂系统的控制需求,具有很广泛的应用前景。
希望通过本文的介绍,读者能够对ARDC控制算法有更深入的了解,同时也能够在实际工程中应用和推广这种新型的控制算法。
【写完文】看完本文,相信读者对ARDC控制算法应该有了一定的了解,尽管这个算法非常新颖,但在未来的控制领域中可能会有非常广泛的应用,进一步的研究和探索将使得这个算法能够更好地应用到未来的控制系统中,实现更加精准和高效的控制。
Robust Control
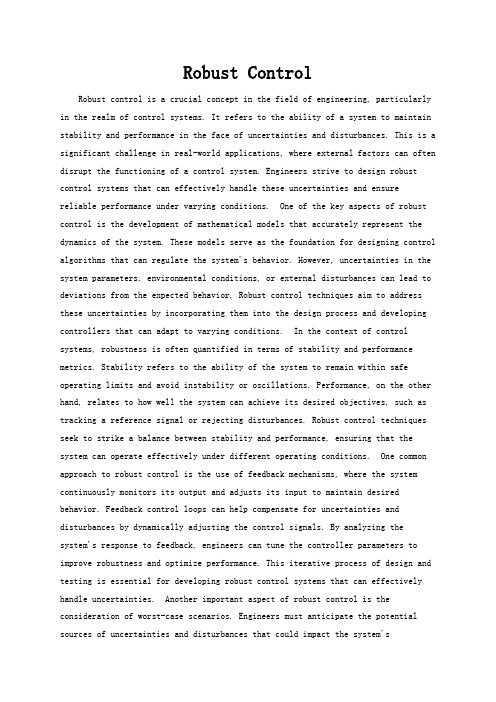
Robust ControlRobust control is a crucial concept in the field of engineering, particularly in the realm of control systems. It refers to the ability of a system to maintain stability and performance in the face of uncertainties and disturbances. This is a significant challenge in real-world applications, where external factors can often disrupt the functioning of a control system. Engineers strive to design robust control systems that can effectively handle these uncertainties and ensurereliable performance under varying conditions. One of the key aspects of robust control is the development of mathematical models that accurately represent the dynamics of the system. These models serve as the foundation for designing control algorithms that can regulate the system's behavior. However, uncertainties in the system parameters, environmental conditions, or external disturbances can lead to deviations from the expected behavior. Robust control techniques aim to address these uncertainties by incorporating them into the design process and developing controllers that can adapt to varying conditions. In the context of control systems, robustness is often quantified in terms of stability and performance metrics. Stability refers to the ability of the system to remain within safe operating limits and avoid instability or oscillations. Performance, on the other hand, relates to how well the system can achieve its desired objectives, such as tracking a reference signal or rejecting disturbances. Robust control techniques seek to strike a balance between stability and performance, ensuring that the system can operate effectively under different operating conditions. One common approach to robust control is the use of feedback mechanisms, where the system continuously monitors its output and adjusts its input to maintain desired behavior. Feedback control loops can help compensate for uncertainties and disturbances by dynamically adjusting the control signals. By analyzing the system's response to feedback, engineers can tune the controller parameters to improve robustness and optimize performance. This iterative process of design and testing is essential for developing robust control systems that can effectively handle uncertainties. Another important aspect of robust control is the consideration of worst-case scenarios. Engineers must anticipate the potential sources of uncertainties and disturbances that could impact the system'sperformance and design controllers that can mitigate these effects. By analyzing the system's response to extreme conditions, engineers can ensure that the control system remains stable and reliable under challenging circumstances. This proactive approach to robust control can help prevent catastrophic failures and ensure the system's safety and reliability. In conclusion, robust control is a critical aspect of engineering design, particularly in the development of control systems. By incorporating uncertainties and disturbances into the design process, engineers can create robust control systems that can effectively handle varying conditions and maintain stability and performance. Through the use of feedback mechanisms, worst-case analysis, and iterative design processes, engineers can optimize the robustness of control systems and ensure their reliable operation in real-world applications. Embracing the challenges of uncertainty and complexity, engineers can develop innovative solutions that push the boundaries of control theory and enhance the performance of dynamic systems.。
直线电机定位力波动的辨识及迭代补偿方法
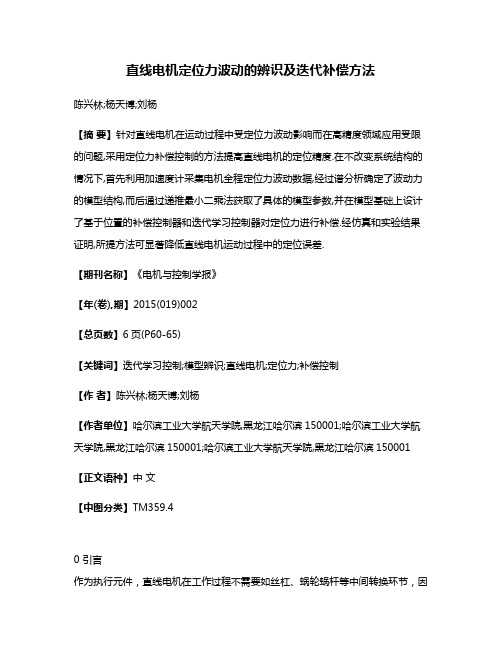
直线电机定位力波动的辨识及迭代补偿方法陈兴林;杨天博;刘杨【摘要】针对直线电机在运动过程中受定位力波动影响而在高精度领域应用受限的问题,采用定位力补偿控制的方法提高直线电机的定位精度.在不改变系统结构的情况下,首先利用加速度计采集电机全程定位力波动数据,经过谱分析确定了波动力的模型结构,而后通过递推最小二乘法获取了具体的模型参数,并在模型基础上设计了基于位置的补偿控制器和迭代学习控制器对定位力进行补偿.经仿真和实验结果证明,所提方法可显著降低直线电机运动过程中的定位误差.【期刊名称】《电机与控制学报》【年(卷),期】2015(019)002【总页数】6页(P60-65)【关键词】迭代学习控制;模型辨识;直线电机;定位力;补偿控制【作者】陈兴林;杨天博;刘杨【作者单位】哈尔滨工业大学航天学院,黑龙江哈尔滨150001;哈尔滨工业大学航天学院,黑龙江哈尔滨150001;哈尔滨工业大学航天学院,黑龙江哈尔滨150001【正文语种】中文【中图分类】TM359.40 引言作为执行元件,直线电机在工作过程不需要如丝杠、蜗轮蜗杆等中间转换环节,因此克服了传统伺服系统中由机械转换机构带来的效率低、体积大、精度低等缺陷。
据此明显的优势,直线电机广泛应用于精密运动系统。
然而,直线电机在运行过程中也受到各种干扰的作用:除电流纹波扰动之外,由于直线电机本身的机械结构限制导致磁场畸变,还会受到诸如齿槽推力波动、边端效应、磁阻推力波动、端部效应等。
为了实现更高精度的直线电机伺服系统,必须对这些干扰进行抑制。
其中,齿槽力波动和边端效应由于只与电机初级和次级的相对位置有关,通常合称为直线电机的定位力。
定位力通常呈周期性变化,在单边平板铁芯式直线电机的运动过程中影响尤为严重[1-2]。
以某光刻机系统为例,由直线电机定位力为主的扰动造成的轨迹误差可达40 μm以上[3]。
因此在高精度运动系统中,直线电机定位力的补偿控制方法对于提高其运动性能不容忽视。
小型四旋翼飞行器姿态的自抗扰控制
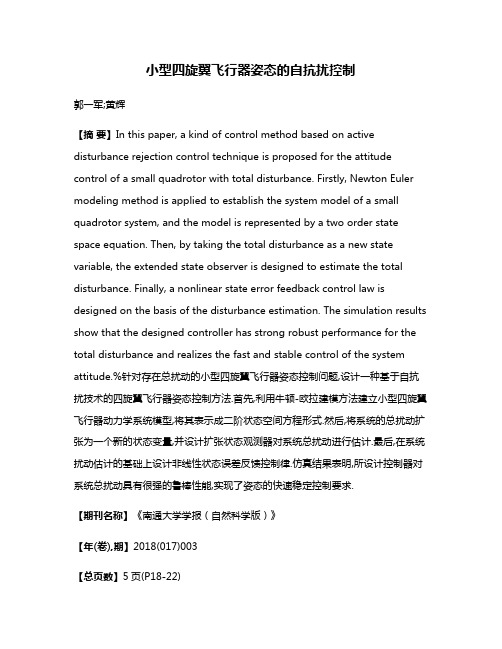
小型四旋翼飞行器姿态的自抗扰控制郭一军;黄辉【摘要】In this paper, a kind of control method based on active disturbance rejection control technique is proposed for the attitude control of a small quadrotor with total disturbance. Firstly, Newton Euler modeling method is applied to establish the system model of a small quadrotor system, and the model is represented by a two order state space equation. Then, by taking the total disturbance as a new state variable, the extended state observer is designed to estimate the total disturbance. Finally, a nonlinear state error feedback control law is designed on the basis of the disturbance estimation. The simulation results show that the designed controller has strong robust performance for the total disturbance and realizes the fast and stable control of the system attitude.%针对存在总扰动的小型四旋翼飞行器姿态控制问题,设计一种基于自抗扰技术的四旋翼飞行器姿态控制方法.首先,利用牛顿-欧拉建模方法建立小型四旋翼飞行器动力学系统模型,将其表示成二阶状态空间方程形式.然后,将系统的总扰动扩张为一个新的状态变量,并设计扩张状态观测器对系统总扰动进行估计.最后,在系统扰动估计的基础上设计非线性状态误差反馈控制律.仿真结果表明,所设计控制器对系统总扰动具有很强的鲁棒性能,实现了姿态的快速稳定控制要求.【期刊名称】《南通大学学报(自然科学版)》【年(卷),期】2018(017)003【总页数】5页(P18-22)【关键词】四旋翼飞行器;姿态控制;自抗扰控制;扩张状态观测器【作者】郭一军;黄辉【作者单位】黄山学院机电工程学院, 安徽黄山 245041;黄山学院机电工程学院, 安徽黄山 245041【正文语种】中文【中图分类】TP241小型四旋翼飞行器近年来发展迅速,由于其具有体积小、质量轻、可垂直起降、机动性强等特点,被广泛地应用于军事和民用领域[1].但由于小型四旋翼模型存在非线性、状态变量间耦合等问题,另外小型四旋翼系统在空中作业时易受到环境不确定因素的干扰,因此对于小型四旋翼飞行器姿态控制系统须满足较强的抗干扰性能要求.为了提高四旋翼姿态控制系统的抗扰性,及获得良好的控制效果,一些非线性控制方法在四旋翼系统中获得了广泛应用.文献[2]为了解决四旋翼无人机姿态控制中存在的问题,提出一种基于反步滑模自抗扰算法的姿态控制器;文献[3]将四旋翼飞行器模型中存在的耦合项和其他未知干扰当作总和扰动,运用扩张状态观测器对其进行估计并以此为基础设计系统的PD控制器,可以克服系统扰动带来的消极影响;文献[4]为抑制四旋翼飞行器系统中的常值干扰及幅值有界且能量有界的干扰,设计了一种非线性PID姿态控制器;文献[5]针对四旋翼姿态易受内部参数摄动与外界环境干扰等不确定性因素的影响,设计了一种鲁棒自适应姿态控制器,提高了系统的抗扰动性能.为提高四旋翼飞行器姿态控制系统对系统扰动的适应能力和姿态控制精度,本文基于自抗扰控制方法设计了四旋翼飞行器姿态控制器.1 四旋翼飞行器姿态控制系统建模在忽略轴承间的摩擦、大气对螺旋桨的干扰以及陀螺效益等因素影响的情况下,基于牛顿-欧拉建模方法可以得到四旋翼飞行器的动力学系统模型[6-7].式中:x,y,z为惯性坐标;φ,θ,ψ分别为滚转角、俯仰角和偏航角;M为四旋翼飞行器质量;L为旋翼中心到机体坐标原点的距离;kx,ky,kz,kφ,kθ,kψ为各通道阻力系数;dx,dy,dz,dφ,dθ,dψ表示各通道的干扰信号;ix,ix,iz为转动惯量;g为重力加速度;u1,u2,u3,u4为控制信号.由式(1)可得四旋翼飞行器姿态控制系统方程为令则四旋翼飞行器姿态控制模型的状态空间表达式可进一步表示为式中,u=[u2 u3 u4]T为系统控制输入向量;Y=[y1 y1 y2]T为系统输出向量;F=[f1 f2 f3]T为系统总扰动向量,B为系统输入矩阵.F,B有如下形式:2 自抗扰控制器设计四旋翼飞行器姿态控制系统存在模型参数不确定性和外部扰动.为提升四旋翼姿态控制系统的姿态跟踪效果,本文采用自抗扰技术设计四旋翼姿态跟踪控制器.自抗扰控制是一种对模型依赖程度较低的控制算法,它对于系统中的干扰具有较好的抑制作用能力,非常适合于直升机这类动态特性复杂且存在各种不确定性的非线性系统[8].本文针对四旋翼飞行器姿态控制设计自抗扰控制器.2.1 跟踪微分器设计系统跟踪微分器一方面可为系统的期望输入信号安排过渡过程,另一方面也可获得高信噪比的期望输入信号的微分信号.式中:v0φ,v0θ,v0ψ分别为四旋翼飞行器滚转角、俯仰角和偏航角的期望值;v1φ,v1θ,v1ψ分别为对应期望值的跟踪信号;v2φ,v2θ,v2ψ分别为对应期望值的微分信号;r0为跟踪微分器的快速因子;h0为跟踪微分器的滤波因子;fhan(·)为最速跟踪控制综合函数[9-11].2.2 非线性扩张状态观测器设计令状态向量 X3=F,且X˙3=h(t),则式(3)可被增广为式中,X3为系统的总扰动向量.非线性扩张状态观测器可对系统的总扰动进行估计.系统(7)非线性扩张状态观测器可设计为式中,eo1,eo2=[eo21 eo22 eo23]T=Z2-X2,eo3=[eo31 eo32 eo33]T=Z3-X3为系统状态和总扰动估计误差向量;Z1=[z1φ z1θ z1ψ]T,Z2=[z2φ z2θz2ψ]T,Z3=[z3φ z3θ z3ψ]T为系统非线性扩张状态观测器的状态向量;K1=diag{k11 k12 k13},K2=diag{k21 k22 k23},K3=diag{k31 k32 k33}为非线性扩张状态观测器的增益矩阵;α1=0.5;α2=0.25;σ > 0 为待整定参数;非线性函数 fal(·)的具体形式参见文献[12-15].2.3 非线性状态误差反馈控制律设计式中,e1φ,e2φ分别为滚转角控制误差及其控制误差的导数;e1θ,e2θ分别为俯仰角控制误差及其控制误差的导数;e1ψ,e2ψ分别为偏航角控制误差及其控制误差的导数;r,h分别为速度因子和精度因子;c为相应的控制参数.3 仿真分析设置四旋翼飞行器系统各仿真参数为:通道阻力系数kφ =0.03 Ns/rad,kθ=0.03 Ns/rad,kψ =0.03 Ns/rad;旋转中心到螺旋桨中心的距离 L=0.25 m;四旋翼飞行器质量M=0.95 kg;转动惯量ix=0.016 5 kg·m2,iy=0.016 7 kg·m2,iz=0.030 2 kg·m2.滚转角、俯仰角和偏航角的初始值为[0.1 0.4 0]T,设定滚转角、俯仰角和偏航角的期望值为[0.5 0.3 0.6]T .假设通道的干扰信号为跟踪微分器参数设置为 r0=500,h0=0.01;扩张状态观测器参数设置为K1=diag{30 30 30},K2=diag{800 800 800},K3=diag{2 000 2 000 2 000},α1=0.5,α2=0.25;非线性状态误差反馈控制律参数设置为c=0.5,r=10 000,h=0.005.仿真结果如图1~3所示,图1为姿态角跟踪曲线;图2为系统扰动观测误差曲线;图3为控制信号曲线.图1 姿态角跟踪曲线图图2 系统扰动观测误差曲线图图3 控制信号曲线图从图1可以看出,系统姿态角可在较短的时间内完成对期望值的跟踪,超调量小且跟踪误差几乎为零.说明所设计控制器对于系统的扰动具有很强的鲁棒性,系统扰动对跟踪控制性能几乎没有影响.由图2可知,扩张状态观测器对系统扰动进行估计时,在估计的初始阶段估计误差相对较大,但很快估计误差就可控制在一个较小的范围内.从图3可以看出,当系统的姿态角误差消除后控制信号的变化很平稳. 为进一步验证所设计的控制器的鲁棒性能,在不改变控制器相关参数的情况下,假设通道干扰信号的幅度增加为原来的一倍,即干扰信号变化为仿真结果如图4、图5所示.由图可见,在控制器参数未发生改变的情况下,尽管系统的扰动发生了改变,但姿态角跟踪曲线基本没有改变,且扩张状态观测器还是可以实现对系统总扰动的有效估计.说明本文所设计控制器具有很强的鲁棒性能. 图4 姿态角跟踪曲线图图5 系统扰动观测误差曲线图4 结论本文针对存在系统扰动的四旋翼飞行器姿态控制问题,基于自抗扰控制方法设计一种姿态跟踪自抗扰控制器,给出了控制器设计的具体步骤并进行了仿真验证.仿真结果表明,自抗扰姿态跟踪控制器具有很强的鲁棒性,可以有效抑制系统扰动对姿态跟踪控制性能的不利影响,实现姿态角的精确跟踪控制.参考文献:【相关文献】[1]陈增强,王辰璐,李毅,等.基于积分滑模的四旋翼飞行器控制系统设计[J].系统仿真学报,2015,27(9):2181-2186.[2]窦景欣,孔祥希,闻邦椿.四旋翼姿态的反步滑模自抗扰控制及稳定性[J].东北大学学报(自然科学版),2016,37 (10):1415-1420.[3]檀姗姗,杨洪玖,路继勇,等.基于扩张状态观测器的飞行器姿态控制研究[J].燕山大学学报,2017,41(6):521-527.[4]宿敬亚,樊鹏辉,蔡开元.四旋翼飞行器的非线性PID姿态控制[J].北京航空航天大学学报,2011,37(9):1054-1058.[5]甄红涛,齐晓慧,夏明旗,等.四旋翼无人机鲁棒自适应姿态控制[J].控制工程,2013,20(5):915-919.[6]窦景欣,孔祥希,闻邦椿.四旋翼无人机模糊自抗扰姿态控制及稳定性分析[J].中国惯性技术学报,2015,23(6):824-830.[7]ZHAO B,XIAN B,ZHANG Y,et al.Nonlinear robust adaptive tracking control of a quadrotor UAV via immersion and invariance methodology[J].IEEE Transactions on Industrial Electronics,2015,62(5):2891-2902.[8]方勇纯,申辉,孙秀云,等.无人直升机航向自抗扰控制[J].控制理论与应用,2014,31(2):238-243.[9]韩京清.自抗扰控制技术:估计补偿不确定因素的控制技术[M].北京:国防工业出版社,2008.[10]李大字,李憧,靳其兵.不确定系统的滑模与自抗扰控制方法[J].上海交通大学学报,2016,50(6):917-922.[11]HAN J Q.From PID to active disturbance rejection control[J].IEEE Transactions on Industrial Electronics,2009,56(3):900-906.[12]刘子建,吴敏,王春生,等.三相电压型PWM整流器自抗扰控制[J].信息与控制,2011,40(4):452-458.[13]赵林峰,徐磊,陈无畏.基于自抗扰控制的自动泊车路径跟踪[J].中国机械工程,2017,28(8):966-973.[14]王高林,王博文,张国强,等.无齿轮永磁曳引机无称重传感器自抗扰控制策略[J].电工技术学报,2016,31(Sup 2):203-209.[15]黄庆,黄守道,匡江传,等.基于模糊自抗扰的PMSM无速度传感器控制[J].湖南大学学报(自然科学版),2012,39(7):37-43.。
- 1、下载文档前请自行甄别文档内容的完整性,平台不提供额外的编辑、内容补充、找答案等附加服务。
- 2、"仅部分预览"的文档,不可在线预览部分如存在完整性等问题,可反馈申请退款(可完整预览的文档不适用该条件!)。
- 3、如文档侵犯您的权益,请联系客服反馈,我们会尽快为您处理(人工客服工作时间:9:00-18:30)。
Indirect Adaptive Robust Control of Electro-Hydraulic Systems Driven by Single-Rod Hydraulic Actuator ∗
∗ The
work is supported in part through the NSF grant CMS-0220179.
0-7803-7759-1/03/$17.00 © 2003 IEEE
296
y2
l2
l3
xst
o2
x2
y3
z1
q3
nificantly. Furthermore, the moment of inertial of external payload J1 is unknown. The cylinder dynamics can be written as V1 (q) ˙ ∂x ˜1 P1 = −A1 q ˙ + Q1 + Q βe ∂q V2 (q) ˙ ∂x ˜2 P2 = A2 q ˙ − Q2 − Q βe ∂q
liver high performance in spite of both parameter uncertainties and uncertain nonlinearities, are essential for successful operations of high-performance electro-hydraulic system. In [2] and [3], the adaptive robust control (ARC) technique proposed by Yao and Tomizuka in [4] and [5], was applied to precision motion control of electro-hydraulic systems driven by single-rod actuators. The underline parameter estimation law in ARC controller are based on the direct adaptive control design such as the tuning function based adaptive backstepping, in which the adaptive control law and parameter adaptation law are synthesized simultaneously to meet the sole objective of reducing the output tracking error. Such a design normally leads to a controller whose dynamic order is as low as the number of unknown parameters to be adapted, and achieves excellent output tracking performance even in the presence of model uncertainties and external disturbances as done in [2] and [3]. However, the direct ARC (DARC) approach also has the drawback that the design of adaptive control law and the parameter estimation law cannot be separated and the choice of the parameter estimation algorithm is limited to the gradient type with certain actual tracking errors as driving signals. It is well known that the gradient type of parameter estimation law may not have as good convergence properties as other types of estimation laws (e.g., the least square method). Furthermore, although the desired trajectory might be persistently exciting and of large signal, the actual tracking errors in implementation are normally very small, and thus the parameter adaptation is prone to be corrupted by other factors such as the sampling delay and noise that have been neglected when synthesizing the parameter adaptation law. As a result, in implementation, the parameter estimates in the DARC are normally not accurate enough to be used for secondary purposes such as prognostics and machine component health monitoring, even when the desired trajectory is persistently exciting enough. The paper focuses on the of precision motion control of electro-hydraulic systems driven by single-rod actuators while accurate parameter estimates are needed for secondary purposes. An IARC design will be presented to overcome the poor parameter estimation problem of the DARC designs
Song Liu School of Mechanical Engineering Purdue University W. Lafayette, IN47907, USA Email: liu1@ Bin Yao School of Mechanical Engineering Purdue University W. Lafayette, IN47907, USA Email: byao@
Abstract This paper presents an indirect adaptive robust control (IARC) of electro-hydraulic systems driven by singlerod hydraulic actuators. Unlike the tracking-performanceoriented direct adaptive robust control (DARC) algorithm, in addition to good output tracking performance, the IARC also focuses on accurate parameter estimates for secondary purposes such as machine health monitoring and prognostics. Accurate parameter estimates are obtained through parameter estimation algorithms based on the plant dynamics rather than the tracking error dynamics, while robust stability and performance are achieved through adaptive robust control. Comparative experimental results show that the proposed IARC achieves good tracking performance and accurate parameter estimation. INTRODUCTION Electro-hydraulic systems have been widely used in industry due to their small size-to-power ratio and the ability to apply very large force and torque. However, precision motion control of hydraulic systems is far from trivial. The dynamics of hydraulic systems are highly nonlinear [1]. Furthermore, the system may be subjected to non-smooth and discontinuous nonlinearities due to control input saturation, directional change of valve opening, friction, and valve overlap. Aside from the nonlinear nature of hydraulic dynamics, electro-hydraulic systems also have large extent of model uncertainties, which can be classified into two categories: parametric uncertainties and uncertain nonlinearities. Examples of parametric uncertainties include the large change in load seen by the actuator and large variations in the hydraulic parameters (e.g., bulk modulus) due to the change of temperature, component wear, etc. Uncertain nonlinearities, such as the external disturbances, leakage, and friction, cannot be modelled exactly and the nonlinear functions that describing them are usually unknown. These model uncertainties may cause the controlled system, designed on the nominal model, to be unstable or have a much degraded performance. Nonlinear robust control techniques, which can de-