美赛论文模板
美国大学生数学建模竞赛优秀论文
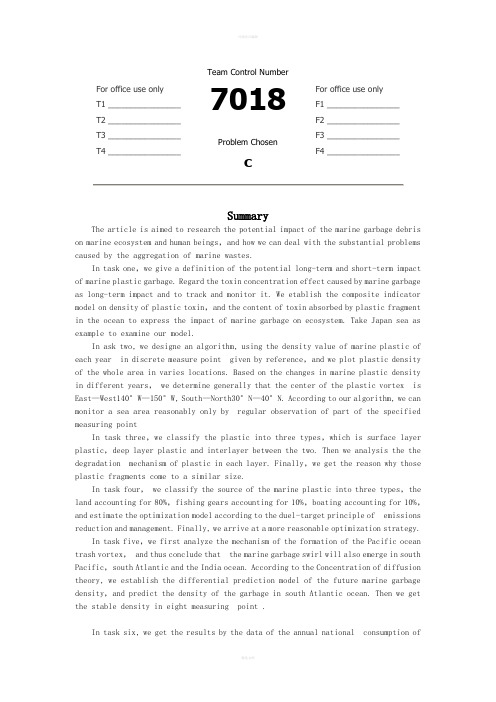
For office use onlyT1________________ T2________________ T3________________ T4________________Team Control Number7018Problem ChosencFor office use onlyF1________________F2________________F3________________F4________________ SummaryThe article is aimed to research the potential impact of the marine garbage debris on marine ecosystem and human beings,and how we can deal with the substantial problems caused by the aggregation of marine wastes.In task one,we give a definition of the potential long-term and short-term impact of marine plastic garbage. Regard the toxin concentration effect caused by marine garbage as long-term impact and to track and monitor it. We etablish the composite indicator model on density of plastic toxin,and the content of toxin absorbed by plastic fragment in the ocean to express the impact of marine garbage on ecosystem. Take Japan sea as example to examine our model.In ask two, we designe an algorithm, using the density value of marine plastic of each year in discrete measure point given by reference,and we plot plastic density of the whole area in varies locations. Based on the changes in marine plastic density in different years, we determine generally that the center of the plastic vortex is East—West140°W—150°W, South—North30°N—40°N. According to our algorithm, we can monitor a sea area reasonably only by regular observation of part of the specified measuring pointIn task three,we classify the plastic into three types,which is surface layer plastic,deep layer plastic and interlayer between the two. Then we analysis the the degradation mechanism of plastic in each layer. Finally,we get the reason why those plastic fragments come to a similar size.In task four, we classify the source of the marine plastic into three types,the land accounting for 80%,fishing gears accounting for 10%,boating accounting for 10%,and estimate the optimization model according to the duel-target principle of emissions reduction and management. Finally, we arrive at a more reasonable optimization strategy.In task five,we first analyze the mechanism of the formation of the Pacific ocean trash vortex, and thus conclude that the marine garbage swirl will also emerge in south Pacific,south Atlantic and the India ocean. According to the Concentration of diffusion theory, we establish the differential prediction model of the future marine garbage density,and predict the density of the garbage in south Atlantic ocean. Then we get the stable density in eight measuring point .In task six, we get the results by the data of the annual national consumption ofpolypropylene plastic packaging and the data fitting method, and predict the environmental benefit generated by the prohibition of polypropylene take-away food packaging in the next decade. By means of this model and our prediction,each nation will reduce releasing 1.31 million tons of plastic garbage in next decade.Finally, we submit a report to expediction leader,summarize our work and make some feasible suggestions to the policy- makers.Task 1:Definition:●Potential short-term effects of the plastic: the hazardeffects will be shown in the short term.●Potential long-term effects of the plastic: thepotential effects, of which hazards are great, willappear after a long time.The short- and long-term effects of the plastic on the ocean environment:In our definition, the short-term and long-term effects of the plastic on the ocean environment are as follows.Short-term effects:1)The plastic is eaten by marine animals or birds.2) Animals are wrapped by plastics, such as fishing nets, which hurt or even kill them.3)Deaden the way of the passing vessels.Long-term effects:1)Enrichment of toxins through the food chain: the waste plastic in the ocean has no natural degradation in theshort-term, which will first be broken down into tinyfragments through the role of light, waves,micro-organisms, while the molecular structure has notchanged. These "plastic sands", easy to be eaten byplankton, fish and other, are Seemingly very similar tomarine life’s food,causing the enrichment and delivery of toxins.2)Accelerate the greenhouse effect: after a long-term accumulation and pollution of plastics, the waterbecame turbid, which will seriously affect the marineplants (such as phytoplankton and algae) inphotosynthesis. A large number of plankton’s deathswould also lower the ability of the ocean to absorbcarbon dioxide, intensifying the greenhouse effect tosome extent.To monitor the impact of plastic rubbish on the marine ecosystem:According to the relevant literature, we know that plastic resin pellets accumulate toxic chemicals , such as PCBs、DDE , and nonylphenols , and may serve as a transport medium and soure of toxins to marine organisms that ingest them[]2. As it is difficult for the plastic garbage in the ocean to complete degradation in the short term, the plastic resin pellets in the water will increase over time and thus absorb more toxins, resulting in the enrichment of toxins and causing serious impact on the marine ecosystem.Therefore, we track the monitoring of the concentration of PCBs, DDE, and nonylphenols containing in the plastic resin pellets in the sea water, as an indicator to compare the extent of pollution in different regions of the sea, thus reflecting the impact of plastic rubbish on ecosystem.To establish pollution index evaluation model: For purposes of comparison, we unify the concentration indexes of PCBs, DDE, and nonylphenols in a comprehensive index.Preparations:1)Data Standardization2)Determination of the index weightBecause Japan has done researches on the contents of PCBs,DDE, and nonylphenols in the plastic resin pellets, we illustrate the survey conducted in Japanese waters by the University of Tokyo between 1997 and 1998.To standardize the concentration indexes of PCBs, DDE,and nonylphenols. We assume Kasai Sesside Park, KeihinCanal, Kugenuma Beach, Shioda Beach in the survey arethe first, second, third, fourth region; PCBs, DDE, andnonylphenols are the first, second, third indicators.Then to establish the standardized model:j j jij ij V V V V V min max min --= (1,2,3,4;1,2,3i j ==)wherej V max is the maximum of the measurement of j indicator in the four regions.j V min is the minimum of the measurement of j indicatorstandardized value of j indicator in i region.According to the literature [2], Japanese observationaldata is shown in Table 1.Table 1. PCBs, DDE, and, nonylphenols Contents in Marine PolypropyleneTable 1 Using the established standardized model to standardize, we have Table 2.In Table 2,the three indicators of Shioda Beach area are all 0, because the contents of PCBs, DDE, and nonylphenols in Polypropylene Plastic Resin Pellets in this area are the least, while 0 only relatively represents the smallest. Similarly, 1 indicates that in some area the value of a indicator is the largest.To determine the index weight of PCBs, DDE, and nonylphenolsWe use Analytic Hierarchy Process (AHP) to determine the weight of the three indicators in the general pollution indicator. AHP is an effective method which transforms semi-qualitative and semi-quantitative problems into quantitative calculation. It uses ideas of analysis and synthesis in decision-making, ideally suited for multi-index comprehensive evaluation.Hierarchy are shown in figure 1.Fig.1 Hierarchy of index factorsThen we determine the weight of each concentrationindicator in the generall pollution indicator, and the process are described as follows:To analyze the role of each concentration indicator, we haveestablished a matrix P to study the relative proportion.⎥⎥⎥⎦⎤⎢⎢⎢⎣⎡=111323123211312P P P P P P P Where mn P represents the relative importance of theconcentration indicators m B and n B . Usually we use 1,2,…,9 and their reciprocals to represent different importance. The greater the number is, the more important it is. Similarly, the relative importance of m B and n B is mn P /1(3,2,1,=n m ).Suppose the maximum eigenvalue of P is m ax λ, then theconsistency index is1max --=n nCI λThe average consistency index is RI , then the consistencyratio isRICI CR = For the matrix P of 3≥n , if 1.0<CR the consistency isthougt to be better, of which eigenvector can be used as the weight vector.We get the comparison matrix accoding to the harmful levelsof PCBs, DDE, and nonylphenols and the requirments ofEPA on the maximum concentration of the three toxins inseawater as follows:⎥⎥⎥⎦⎤⎢⎢⎢⎣⎡=165416131431P We get the maximum eigenvalue of P by MATLAB calculation0012.3max =λand the corresponding eigenvector of it is()2393.02975.09243.0,,=W1.0042.012.1047.0<===RI CI CR Therefore,we determine the degree of inconsistency formatrix P within the permissible range. With the eigenvectors of p as weights vector, we get thefinal weight vector by normalization ()1638.02036.06326.0',,=W . Defining the overall target of pollution for the No i oceanis i Q , among other things the standardized value of threeindicators for the No i ocean is ()321,,i i i i V V V V = and the weightvector is 'W ,Then we form the model for the overall target of marine pollution assessment, (3,2,1=i )By the model above, we obtained the Value of the totalpollution index for four regions in Japanese ocean in Table 3T B W Q '=In Table3, the value of the total pollution index is the hightest that means the concentration of toxins in Polypropylene Plastic Resin Pellets is the hightest, whereas the value of the total pollution index in Shioda Beach is the lowest(we point up 0 is only a relative value that’s not in the name of free of plastics pollution)Getting through the assessment method above, we can monitor the concentration of PCBs, DDE and nonylphenols in the plastic debris for the sake of reflecting the influence to ocean ecosystem.The highter the the concentration of toxins,the bigger influence of the marine organism which lead to the inrichment of food chain is more and more dramatic.Above all, the variation of toxins’ concentration simultaneously reflects the distribution and time-varying of marine litter. We can predict the future development of marine litter by regularly monitoring the content of these substances, to provide data for the sea expedition of the detection of marine litter and reference for government departments to make the policies for ocean governance.Task 2:In the North Pacific, the clockwise flow formed a never-ending maelstrom which rotates the plastic garbage. Over the years, the subtropical eddy current in North Pacific gathered together the garbage from the coast or the fleet, entrapped them in the whirlpool, and brought them to the center under the action of the centripetal force, forming an area of 3.43 million square kilometers (more than one-third of Europe) .As time goes by, the garbage in the whirlpool has the trend of increasing year by year in terms of breadth, density, and distribution. In order to clearly describe the variability of the increases over time and space, according to “Count Densities of Plastic Debris from Ocean Surface Samples North Pacific Gyre 1999—2008”, we analyze the data, exclude them with a great dispersion, and retain them with concentrated distribution, while the longitude values of the garbage locations in sampled regions of years serve as the x-coordinate value of a three-dimensional coordinates, latitude values as the y-coordinate value, the Plastic Count per cubic Meter of water of the position as the z-coordinate value. Further, we establish an irregular grid in the yx plane according to obtained data, and draw a grid line through all the data points. Using the inverse distance squared method with a factor, which can not only estimate the Plastic Count per cubic Meter of water of any position, but also calculate the trends of the Plastic Counts per cubic Meter of water between two original data points, we can obtain the unknown grid points approximately. When the data of all the irregular grid points are known (or approximately known, or obtained from the original data), we can draw the three-dimensional image with the Matlab software, which can fully reflect the variability of the increases in the garbage density over time and space.Preparations:First, to determine the coordinates of each year’s sampled garbage.The distribution range of garbage is about the East - West 120W-170W, South - North 18N-41N shown in the “Count Densities of Plastic Debris from Ocean Surface Samples North Pacific Gyre 1999--2008”, we divide a square in the picture into 100 grids in Figure (1) as follows:According to the position of the grid where the measuring point’s center is, we can identify the latitude and longitude for each point, which respectively serve as the x- and y- coordinate value of the three-dimensional coordinates.To determine the Plastic Count per cubic Meter of water. As the “Plastic Count per cubic Meter of water” provided by “Count Densities of P lastic Debris from Ocean Surface Samples North Pacific Gyre 1999--2008”are 5 density interval, to identify the exact values of the garbage density of one year’s different measuring points, we assume that the density is a random variable which obeys uniform distribution in each interval.Uniform distribution can be described as below:()⎪⎩⎪⎨⎧-=01a b x f ()others b a x ,∈We use the uniform function in Matlab to generatecontinuous uniformly distributed random numbers in each interval, which approximately serve as the exact values of the garbage density andz-coordinate values of the three-dimensional coordinates of the year’s measuring points.Assumptions(1)The data we get is accurate and reasonable.(2)Plastic Count per cubic Meter of waterIn the oceanarea isa continuous change.(3)Density of the plastic in the gyre is a variable by region.Density of the plastic in the gyre and its surrounding area is interdependent , However, this dependence decreases with increasing distance . For our discussion issue, Each data point influences the point of each unknown around and the point of each unknown around is influenced by a given data point. The nearer a given data point from the unknown point, the larger the role.Establishing the modelFor the method described by the previous,we serve the distributions of garbage density in the “Count Pensities of Plastic Debris from Ocean Surface Samples North Pacific Gyre 1999--2008”as coordinates ()z y,, As Table 1:x,Through analysis and comparison, We excluded a number of data which has very large dispersion and retained the data that is under the more concentrated the distribution which, can be seen on Table 2.In this way, this is conducive for us to get more accurate density distribution map.Then we have a segmentation that is according to the arrangement of the composition of X direction and Y direction from small to large by using x co-ordinate value and y co-ordinate value of known data points n, in order to form a non-equidistant Segmentation which has n nodes. For the Segmentation we get above,we only know the density of the plastic known n nodes, therefore, we must find other density of the plastic garbage of n nodes.We only do the sampling survey of garbage density of the north pacificvortex,so only understand logically each known data point has a certain extent effect on the unknown node and the close-known points of density of the plastic garbage has high-impact than distant known point.In this respect,we use the weighted average format, that means using the adverse which with distance squared to express more important effects in close known points. There're two known points Q1 and Q2 in a line ,that is to say we have already known the plastic litter density in Q1 and Q2, then speculate the plastic litter density's affects between Q1、Q2 and the point G which in the connection of Q1 and Q2. It can be shown by a weighted average algorithm22212221111121GQ GQ GQ Z GQ Z Z Q Q G +*+*=in this formula GQ expresses the distance between the pointG and Q.We know that only use a weighted average close to the unknown point can not reflect the trend of the known points, we assume that any two given point of plastic garbage between the changes in the density of plastic impact the plastic garbage density of the unknown point and reflecting the density of plastic garbage changes in linear trend. So in the weighted average formula what is in order to presume an unknown point of plastic garbage density, we introduce the trend items. And because the greater impact at close range point, and thus the density of plastic wastes trends close points stronger. For the one-dimensional case, the calculation formula G Z in the previous example modify in the following format:2212122212212122211111112121Q Q GQ GQ GQ Q Q GQ Z GQ Z GQ Z Z Q Q Q Q G ++++*+*+*=Among them, 21Q Q known as the separation distance of the known point, 21Q Q Z is the density of plastic garbage which is the plastic waste density of 1Q and 2Q for the linear trend of point G . For the two-dimensional area, point G is not on the line 21Q Q , so we make a vertical from the point G and cross the line connect the point 1Q and 2Q , and get point P , the impact of point P to 1Q and 2Q just like one-dimensional, and the one-dimensional closer of G to P , the distant of G to P become farther, the smaller of the impact, so the weighting factor should also reflect the GP in inversely proportional to a certain way, then we adopt following format:221212222122121222211111112121Q Q GQ GP GQ GQ Q Q GQ GP Z GQ Z GQ Z Z P Q Q Q Q G ++++++*+*+*=Taken together, we speculated following roles:(1) Each known point data are influence the density of plastic garbage of each unknown point in the inversely proportional to the square of the distance;(2) the change of density of plastic garbage between any two known points data, for each unknown point are affected, and the influence to each particular point of their plastic garbage diffuse the straight line along the two known particular point; (3) the change of the density of plastic garbage between any two known data points impact a specific unknown points of the density of plastic litter depends on the three distances: a. the vertical distance to a straight line which is a specific point link to a known point;b. the distance between the latest known point to a specific unknown point;c. the separation distance between two known data points.If we mark 1Q ,2Q ,…,N Q as the location of known data points,G as an unknown node, ijG P is the intersection of the connection of i Q ,j Q and the vertical line from G to i Q ,j Q()G Q Q Z j i ,,is the density trend of i Q ,j Q in the of plasticgarbage points and prescribe ()G Q Q Z j i ,,is the testing point i Q ’ s density of plastic garbage ,so there are calculation formula:()()∑∑∑∑==-==++++*=Ni N ij ji i ijGji i ijG N i Nj j i G Q Q GQ GPQ Q GQ GP G Q Q Z Z 11222222111,,Here we plug each year’s observational data in schedule 1 into our model, and draw the three-dimensional images of the spatial distribution of the marine garbage ’s density with Matlab in Figure (2) as follows:199920002002200520062007-2008(1)It’s observed and analyzed that, from 1999 to 2008, the density of plastic garbage is increasing year by year and significantly in the region of East – West 140W-150W, south - north 30N-40N. Therefore, we can make sure that this region is probably the center of the marine litter whirlpool. Gathering process should be such that the dispersed garbage floating in the ocean move with the ocean currents and gradually close to the whirlpool region. At the beginning, the area close to the vortex will have obviously increasable about plastic litter density, because of this centripetal they keeping move to the center of the vortex ,then with the time accumulates ,the garbage density in the center of the vortex become much bigger and bigger , at last it becomes the Pacific rubbish island we have seen today.It can be seen that through our algorithm, as long as the reference to be able to detect the density in an area which has a number of discrete measuring points,Through tracking these density changes ,we Will be able to value out all the waters of the density measurement through our models to determine,This will reduce the workload of the marine expedition team monitoring marine pollution significantly, and also saving costs .Task 3:The degradation mechanism of marine plasticsWe know that light, mechanical force, heat, oxygen, water, microbes, chemicals, etc. can result in the degradation of plastics . In mechanism ,Factors result in the degradation can be summarized as optical ,biological,and chemical。
美赛论文(最终版)

For office use onlyT1________________ T2________________ T3________________ T4________________Team Control Number 46639Problem ChosenCFor office use onlyF1________________F2________________F3________________F4________________2016 MCM/ICM Summary SheetAn Optimal Investment Strategy ModelSummaryWe develop an optimal investment strategy model that appears to hold promise for providing insight into not only how to sort the schools according to investment priority, but also identify optimal investment amount of a specific school. This model considers a large number of parameters thought to be important to investment in the given College Scorecard Data Set.In order to develop the required model, two sub-models are constructed as follows: 1.For Analytic Hierarchy Process (AHP) Model, we identify the prioritizedcandidate list of schools by synthesizing the elements which have an influence on investment. First we define the specific value of any two elements’ effect on investment. And then the weight of each element’s influence on investment can be identified. Ultimately, we take the relevant parameters into the calculated weight, and then we get any school’s recommended value of investment.2.For Return On Investment M odel, it’s constructed on the basis of AHP Model.Let us suppose that all the investment is used to help the students to pay tuition fee.Then we can see optimal investment as that we help more students to the universities of higher return rate. However, because of dropout rate, there will be an optimization investment amount in each university. Therefore, we can change the problem into a nonlinear programming problem. We identify the optimal investment amount by maximizing return-on-investment.Specific attention is given to the stability and error analysis of our model. The influence of the model is discussed when several fundamental parameters vary. We attempt to use our model to prioritize the schools and identify investment amount of the candidate schools, and then an optimal investment strategy is generated. Ultimately, to demonstrate how our model works, we apply it to the given College Scorecard Data Set. For various situations, we propose an optimal solution. And we also analyze the strengths and weaknesses of our model. We believe that we can make our model more precise if more information are provided.Contents1.Introduction 21.1Restatement of the Problem (2)1.2Our Approach (2)2.Assumptions 23.Notations 34.The Optimal Investment Model 44.1Analytic Hierarchy Process Model (4)4.1.1Constructing the Hierarchy (4)4.1.2Constructing the Judgement Matrix (5)4.1.3Hierarchical Ranking (7)4.2Return On Investment Model (8)4.2.1Overview of the investment strategy (8)4.2.2Analysis of net income and investment cost (9)4.2.3Calculate Return On Investment (11)4.2.4Maximize the Total Net Income (11)5.Test the Model125.1Error Analysis (12)5.2Stability Analysis (13)6.Results136.1Results of Analytic Hierarchy Process (13)6.2Results of Return On Investment Model (14)7.Strengths and Weaknesses157.1Strengths (15)7.2Weaknesses (16)References16 Appendix A Letter to the Chief Financial Officer, Mr. Alpha Chiang.171.Introduction1.1Restatement of the ProblemIn order to help improve educational performance of undergraduates attending colleges and universities in the US, the Goodgrant Foundation intends to donate a total of $100,000,000 to an appropriate group of schools per year, for five years, starting July 2016. We are to develop a model to determine an optimal investment strategy that identifies the school, the investment amount per school, the return on that investment, and the time duration that the organization’s money should be provided to have the highest likelihood of producing a strong positive effect on student performance. Considering that they don’t want to duplicate the investments and focus of other large grant organizations, we interpret optimal investment as a strategy that maximizes the ROI on the premise that we help more students attend better colleges. So the problems to be solved are as follows:1.How to prioritize the schools by optimization level.2.How to measure ROI of a school.3.How to measure investment amount of a specific school.1.2Our ApproachWe offer a model of optimal investment which takes a great many factors in the College Scorecard Data Set into account. To begin with, we make a 1 to N optimized and prioritized candidate list of school we are recommending for investment by the AHP model. For the sake that we invest more students to better school, several factors are considered in the AHP model, such as SAT score, ACT score, etc. And then, we set investment amount of each university in the order of the list according to the standard of maximized ROI. The implement details of the model will be described in section 4.2.AssumptionsWe make the following basic assumptions in order to simplify the problem. And each of our assumptions is justified.1.Investment amount is mainly used for tuition and fees. Considering that theincome of an undergraduate is usually much higher than a high school students, we believe that it’s necessary to help more poor students have a chance to go to college.2.Bank rates will not change during the investment period. The variation ofthe bank rates have a little influence on the income we consider. So we make this assumption just to simplify the model.3.The employment rates and dropout rates will not change, and they aredifferent for different schools4.For return on investment, we only consider monetary income, regardlessof the intangible income.3.NotationsWe use a list of symbols for simplification of expression.4.The Optimal Investment ModelIn this section, we first prioritize schools by the AHP model (Section 4.1), and then calculate ROI value of the schools (Section 4.2). Ultimately, we identify investment amount of every candidate schools according to ROI (Section 4.3).4.1Analytic Hierarchy Process ModelIn order to prioritize schools, we must consider each necessary factor in the College Scorecard Data Set. For each factor, we calculate its weight value. And then, we can identify the investment necessity of each school. So, the model can be developed in 3 steps as follows:4.1.1Constructing the HierarchyWe consider 19 elements to measure priority of candidate schools, which can be seen in Fig 1. The hierarchy could be diagrammed as follows:Fig.1AHP for the investment decisionThe goal is red, the criteria are green and the alternatives are blue. All the alternatives are shown below the lowest level of each criterion. Later in the process, each alternatives will be rated with respect to the criterion directly above it.As they build their hierarchy, we should investigate the values or measurements of the different elements that make it up. If there are published fiscal policy, for example, or school policy, they should be gathered as part of the process. This information will be needed later, when the criteria and alternatives are evaluated.Note that the structure of the investment hierarchy might be different for other foundations. It would definitely be different for a foundation who doesn't care how much his score is, knows he will never dropout, and is intensely interested in math, history, and the numerous aspects of study[1].4.1.2Constructing the Judgement MatrixHierarchy reflects the relationship among elements to consider, but elements in the Criteria Layer don’t always weigh equal during aim measure. In deciders’ mind, each element accounts for a particular proportion.To incorporate their judgments about the various elements in the hierarchy, decision makers compare the elements “two by two”. The fundamental scale for pairwise comparison are shown in Fig 2.Fig 2Right now, let's see which items are compared. Our example will begin with the six criteria in the second row of the hierarchy in Fig 1, though we could begin elsewhere if we want. The criteria will be compared as to how important they are to the decisionmakers, with respect to the goal. Each pair of items in this row will be compared.Fig 3 Investment Judgement MatrixIn the next row, there is a group of 19 alternatives under the criterion. In the subgroup, each pair of alternatives will be compared regarding their importance with respect to the criterion. (As always, their importance is judged by the decision makers.) In the subgroup, there is only one pair of alternatives. They are compared as to how important they are with respect to the criterion.Things change a bit when we get to the alternatives row. Here, the factor in each group of alternatives are compared pair-by-pair with respect to the covering criterion of the group, which is the node directly above them in the hierarchy. What we are doing here is evaluating the models under consideration with respect to score, then with respect to Income, then expenditure, dropout rate, debt and graduation rate.The foundation can evaluate alternatives against their covering criteria in any order they choose. In this case, they choose the order of decreasing priority of the covering criteria.Fig 4 Score Judgement MatrixFig 5 Expenditure Judgement MatrixFig 6 Income Judgement MatrixFig 7 Dropout Judgement MatrixFig 8 Debt Judgement MatrixFig 9 Graduation Matrix4.1.3 Hierarchical RankingWhen the pairwise comparisons are as numerous as those in our example, specialized AHP software can help in making them quickly and efficiently. We will assume that the foundation has access to such software, and that it allows the opinions of various foundations to be combined into an overall opinion for the group.The AHP software uses mathematical calculations to convert these judgments to priorities for each of the six criteria. The details of the calculations are beyond the scope of this article, but are readily available elsewhere[2][3][4][5]. The software also calculates a consistency ratio that expresses the internal consistency of the judgments that have been entered. In this case the judgments showed acceptable consistency, and the software used the foundation’s inputs to assign these new priorities to the criteria:Fig 10.AHP hierarchy for the foundation investing decision.In the end, the AHP software arranges and totals the global priorities for each of the alternatives. Their grand total is 1.000, which is identical to the priority of the goal. Each alternative has a global priority corresponding to its "fit" to all the foundation's judgments about all those aspects of factor. Here is a summary of the global priorities of the alternatives:Fig 114.2 ROI Model4.2.1 Overview of the investment strategyConsider a foundation making investment on a set of N geographically dispersed colleges and university in the United States, D = {1, 2, 3……N }. Then we can select top N schools from the candidate list which has been sorted through analytic hierarchy process. The total investment amount is M per year which is donated by the Goodgrant Foundation. The investment amount is j m for each school j D ∈, satisfying the following balance constraint:j j D mM ∈=∑ (1)W e can’t invest too much or too little money to one school because we want to help more students go to college, and the student should have more choices. Then the investment amount for each school must have a lower limit lu and upper limit bu as follows:j lu m bu ≤≤ (2)The tuition and fees is j p , and the time duration is {1,2,3,4}j t ∈. To simplify ourmodel, we assume that our investment amount is only used for freshmen every year. Because a freshmen oriented investment can get more benefits compared with others. For each school j D ∈, the number of the undergraduate students who will be invested is j n , which can be calculated by the following formula :,jj j j m n j D p t =∈⨯ (3)Figure12The foundation can use the ROI model to identify j m and j t so that it canmaximize the total net income. Figure1 has shown the overview of our investment model. We will then illustrate the principle and solution of this model by a kind of nonlinear programming method.4.2.2 Analysis of net income and investment costIn our return on investment model, we first focus on analysis of net income and investment cost. Obviously, the future earnings of undergraduate students are not only due to the investment itself. There are many meaning factors such as the effort, the money from their parents, the training from their companies. In order to simplify the model, we assume that the investment cost is the most important element and we don’t consider other possible influence factors. Then we can conclude that the total cost of the investment is j m for each school j D ∈.Figure 13For a single student, the meaning of the investment benefits is the expected earnings in the future. Assuming that the student is not going to college or university after graduating from high school and is directly going to work. Then his wage base is 0b as a high school graduate. If he works as a college graduate, then his wage base is 0a . Then we can give the future proceeds of life which is represented symbolically by T and we use r to represent the bank rates which will change over time. We assume that the bank rates will not change during the investment period. Here, we use bank rates in 2016 to represent the r . The future proceeds of life of a single undergraduate student will be different due to individual differences such as age, physical condition environment, etc. If we consider these differences, the calculation process will be complicated. For simplicity’s sake, we uniform the future proceeds of life T for 20 years. Then we will give two economics formulas to calculate the total expected income in the next T years for graduates and high school graduates:40(1)Tk k a u r +==+∑(4) 40(1)T kk b h r +==+∑(5) The total expected income of a graduate is u , and the total expected income of a highschool graduate is h .Then, we continue to analyze the net income. The net income can be calculated by the following formula:os NetIncome TotalIncome C t =- (6) For each school j D ∈, the net income is j P , the total income is j Q , and the cost is j m . Then we will get the following equation through formula (6):j j j P Q m =- (7)Therefore, the key of the problem is how to calculate j Q . In order to calculate j Q, weneed to estimate the number of future employment j ne . The total number of the invested is j n , which has been calculated above. Considering the dropout rates j α and the employment rates j β for each school j , we can calculate the number of future employment j ne through the following formula:(4)(1)jt j j j j n e n βα-=⨯⨯- (8)That way, we can calculate j Q by the following formula:()j j Q ne u h =⨯- (9)Finally, we take Eq. (2) (3) (4) (7) (8) into Eq. (6), and we will obtain Eq. (9) as follows:4(4)00400(1)()(1)(1)j TT t j j j j j k kk k j jm a b P m p t r r βα+-+===⨯⨯-⨯--⨯++∑∑ (10) We next reformulate the above equation of j P for concise presentation:(4)(1)j t j jj j j jc m P m t λα-⨯⨯=⨯-- (11)where jj j p βλ= and 400400(1)(1)TT k kk k a b c r r ++===-++∑∑ .4.2.3 Calculate Return On InvestmentROI is short of return on investment which can be determined by net income andinvestment cost [7]. It conveys the meaning of the financial assessment. For each schoolj D ∈ , the net income is j P , and the investment cost equals to j m . Then the j ROIcan be calculated by the following formula:100%j j jP ROI m =⨯ (12)We substitute Eq. (10) into Eq. (11), and we will get a new formula as follows:(4)((1)1)100%j t j j j jc ROI t λα-⨯=⨯--⨯ (13)4.2.4 Maximize the Total Net IncomeGiven the net income of each school, we formulate the portfolio problem that maximize the total net income, S=Max(4)((1))j t j jj j j j Dj Djc m P m t λα-∈∈⨯⨯=⨯--∑∑ (14)S. T.jj DmM ∈=∑,{1,2,3,4}t = ,j lu m bu ≤≤ ,Considering the constraint jj DmM ∈=∑, we can further simplify the model,S is equivalent to S’=Max(4)((1))j t j jj j j Dj Djc m P t λα-∈∈⨯⨯=⨯-∑∑ (15)S. T.jj DmM ∈=∑,{1,2,3,4t = ,j l u m b u ≤≤. By solving the nonlinear programming problem S’, we can get the sameanswer as problem S.5. Testing the Model 5.1 Error AnalysisSince the advent of analytic hierarchy process, people pay more attention to it due to the specific applicability, convenience, practicability and systematization of the method. Analytic hierarchy process has not reached the ideal situation whether in theory or application level because the results depend largely on the preference and subjective judgment. In this part, we will analyze the human error problem in analytic hierarchy process.Human error is mainly caused by human factors. The human error mainly reflects on the structure of the judgment matrix. The causes of the error are the following points:1. The number of times that human judge the factors’ importance is excessive.2. The calibration method is not perfect.Then we will give some methods to reduce errors:1. Reduce times of human judgment. One person repeatedly gave the samejudgment between two factors. Or many persons gave the same judgment between two factors one time. Finally, we take the average as result.2. Break the original calibration method. If we have defined the ranking vector111121(,...)n a a a a =between the factor 1A with others. Then we can get all theother ranking vector. For example : 12122111(,1...)na a a a a =.5.2 Stability AnalysisIt is necessary to analyze the stability of ranking result [6], because the strong subjectivefactors. If the ranking result changed a little while the judgment changed a lot, we can conclude that the method is effective and the result is acceptable, and vice versa. We assume that the weight of other factors will change if the weight of one factor changed from i ξ to i η:[8](1)(,1,2...,)(1)i j j i i j n i j ηξηξ-⨯==≠- (16)And it is simple to verify the equation:11nii η==∑ (17)And the new ranking vector ω will be:A ωη=⨯ (18)By this method, the Relative importance between other factors remain the same while one of the factor has changed.6. Results6.1 Results of Analytic Hierarchy ProcessWe can ranking colleges through the analytic hierarchy process, and we can get the top N = 20 schools as follows6.2 Results of Return On Investment ModelBased on the results above, we next use ROI model to distribute investment amountj m and time duration j t for each school j D ∈ by solving the following problem:Max (4)((1))j t j jj j j Dj Djc m P t λα-∈∈⨯⨯=⨯-∑∑S. T.jj DmM ∈=∑,{1,2,3,4t = , j l u m b u≤≤ . In order to solve the problem above, we collected the data from different sources. Inthe end, we solve the model with Lingo software. The program code is as follows:model: sets:roi/1..20/:a,b,p,m,t;endsets data:a = 0.9642 0.9250 0.9484 0.9422 0.9402 0.9498 0.90490.9263 0.9769 0.9553 0.9351 0.9123 0.9410 0.98610.9790 0.9640 0.8644 0.9598 0.9659 0.9720;b = 0.8024 0.7339 0.8737 0.8308 0.8681 0.7998 0.74920.6050 0.8342 0.8217 0.8940 0.8873 0.8495 0.87520.8333 0.8604 0.8176 0.8916 0.7527 0.8659;p = 3.3484 3.7971 3.3070 3.3386 3.3371 3.4956 3.22204.0306 2.8544 3.1503 3.2986 3.3087 3.3419 2.78452.9597 2.92713.3742 2.7801 2.5667 2.8058;c = 49.5528;enddatamax=@sum(roi(I):m(I)/t(I)/p(I)*((1-b(I))^4)*c*(1-a(I)+0.05)^(4-t(I)));@for(roi:@gin(t));@for(roi(I):@bnd(1,t(I),4));@for(roi(I):@bnd(0,m(I),100));@sum(roi(I):m(I))=1000;ENDFinally, we can get the investment amount and time duration distribution as follows:7.Strengths and Weaknesses7.1Strengths1.Fixing the bank rates during the investment period may run out, but it will haveonly marginal influences.2.For return on investment, we only consider monetary income, regardless of the3.intangible income. But the quantization of these intangible income is very importantand difficult. It needs to do too much complicated technical analysis and to quantify 4.too many variables. Considering that the investment persists for a short time, thiskind of random error is acceptable.5.Due to our investment which is freshmen oriented, other students may feel unfair.It is likely to produce adverse reaction to our investment strategy.6.The cost estimation is not impeccable. We only consider the investment amount andignore other non-monetary investment.5. AHP needs higher requirements for personnel quality.7.2Weaknesses1.Our investment strategy is distinct and clear, and it is convenient to implement.2.Our model not only identifies the investment amount for each school, but alsoidentifies the time duration that the organization’s money should be provide d.3.Data processing is convenient, because the most data we use is constant, average ormedian.4.Data sources are reliable. Our investment strategy is based on some meaningful anddefendable subset of two data sets.5.AHP is more simple, effective and universal.References[1] Saaty, Thomas L. (2008). Decision Making for Leaders: The Analytic Hierarchy Process for Decisions in a Complex World. Pittsburgh, Pennsylvania: RWS Publications. ISBN 0-9620317-8-X.[2] Bhushan, Navneet, Kanwal Rai (January 2004). Strategic Decision Making: Applying the Analytic Hierarchy Process. London: Springer-Verlag. ISBN 1-8523375-6-7.[3] Saaty, Thomas L. (2001). Fundamentals of Decision Making and Priority Theory. Pittsburgh, Pennsylvania: RWS Publications. ISBN 0-9620317-6-3.[4] Trick, Michael A. (1996-11-23). "Analytic Hierarchy Process". Class Notes. Carnegie Mellon University Tepper School of Business. Retrieved 2008-03-02.[5] Meixner, Oliver; Reiner Haas (2002). Computergestützte Entscheidungs-findung: Expert Choice und AHP – innovative Werkzeuge zur Lösung komplexer Probleme (in German). Frankfurt/Wien: Redline Wirtschaft bei Ueberreuter. ISBN 3-8323-0909-8.[6] Hazelkorn, E. The Impact of League Tables and Ranking System on Higher Education Decision Making [J]. Higher Education Management and Policy, 2007, 19(2), 87-110.[7] Leslie: Trainer Assessment: A Guide to Measuring the Performance of Trainers and Facilitors, Second Edition, Gower Publishing Limited, 2002.[8] Aguaron J, Moreno-Jimenea J M. Local stability intervals in the analytic hierarchy process. European Journal of Operational Research. 2000Letter to the Chief Financial Officer, Mr. Alpha Chiang. February 1th, 2016.I am writing this letter to introduce our optimal investment strategy. Before I describe our model, I want to discuss our proposed concept of a return-on-investment (ROI). And then I will describe the optimal investment model by construct two sub-model, namely AHP model and ROI model. Finally, the major results of the model simulation will be showed up to you.Considering that the Goodgrant Foundation aims to help improve educational performance of undergraduates attending colleges and universities in the US, we interpret return-on-investment as the increased income of undergraduates. Because the income of an undergraduate is generally much higher than a high school graduate, we suggest all the investment be used to pay for the tuition and fees. In that case, if we take both the income of undergraduates’ income and dropout rate into account, we can get the return-in-investment value.Our model begins with the production of an optimized and prioritized candidate list of schools you are recommending for investment. This sorted list of school is constructed through the use of specification that you would be fully qualified to provided, such as the score of school, the income of graduate student, the dropout rate, etc. With this information, a precise investment list of schools will be produced for donation select.Furthermore, we developed the second sub-model, ROI model, which identifies the investment amount of each school per year. If we invest more money in a school, more students will have a chance to go to college. However, there is an optimal investment amount of specific school because of the existence of dropout. So, we can identify every candidate school’s in vestment amount by solve a nonlinear programming problem. Ultimately, the result of the model simulation show that Washington University, New York University and Boston College are three schools that worth investing most. And detailed simulation can be seen in our MCM Contest article.We hope that this model is sufficient in meeting your needs in any further donation and future philanthropic educational investments within the United States.。
美赛论文模版
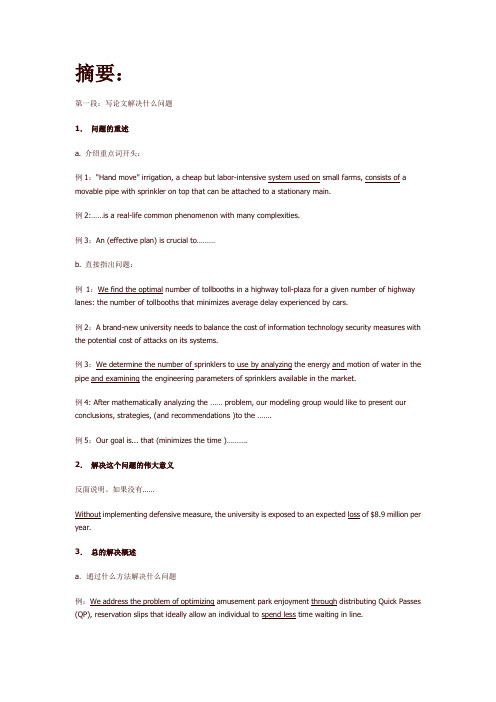
摘要:第一段:写论文解决什么问题1.问题的重述a. 介绍重点词开头:例1:“Hand move” irrigation, a cheap but labor-intensive system used on small farms, consists of a movable pipe with sprinkler on top that can be attached to a stationary main.例2:……is a real-life common phenomenon with many complexi t ies.例3:An (effective plan) is crucial to………b. 直接指出问题:例1:We find the optimal number of tollbooths in a highway toll-plaza for a given number of highway lanes: the number of tollbooths that minimizes average delay experienced by cars.例2:A brand-new university needs to balance the cost of information technology security measures wi t h the potential cost of attacks on its systems.例3:We determine the number of sprinklers to use by analyzing the energy and motion of water in the pipe and examining the engineering parameters of sprinklers available in the market.例4: After mathematically analyzing the …… problem, our modeling group would like to present our conclusions, strategies, (and recommendations )to the …….例5:Our goal is... that (mini mizes the time )……….2.解决这个问题的伟大意义反面说明。
美赛论文模板(超实用)

TitileSummaryDuring cell division, mitotic spindles are assembled by microtubule-based motor proteins1, 2. The bipolar organization of spindles is essential for proper segregation of chromosomes, and requires plus-end-directed homotetrameric motor proteins of the widely conserved kinesin-5 (BimC) family3. Hypotheses for bipolar spindle formation include the 'push−pull mitotic muscle' model, in which kinesin-5 and opposing motor proteins act between overlapping microtubules2, 4, 5. However, the precise roles of kinesin-5 during this process are unknown. Here we show that the vertebrate kinesin-5 Eg5 drives the sliding of microtubules depending on their relative orientation. We found in controlled in vitro assays that Eg5 has the remarkable capability of simultaneously moving at 20 nm s-1 towards the plus-ends of each of the two microtubules it crosslinks. For anti-parallel microtubules, this results in relative sliding at 40 nm s-1, comparable to spindle pole separation rates in vivo6. Furthermore, we found that Eg5 can tether microtubule plus-ends, suggesting an additional microtubule-binding mode for Eg5. Our results demonstrate how members of the kinesin-5 family are likely to function in mitosis, pushing apart interpolar microtubules as well as recruiting microtubules into bundles that are subsequently polarized by relative sliding. We anticipate our assay to be a starting point for more sophisticated in vitro models of mitotic spindles. For example, the individual and combined action of multiple mitotic motors could be tested, including minus-end-directed motors opposing Eg5 motility. Furthermore, Eg5 inhibition is a major target of anti-cancer drug development, and a well-defined and quantitative assay for motor function will be relevant for such developmentsContentTitile (1)Summary (1)1Introduction (1)1.1Restatement of the Problem (1)1.2Background (1)1.1.1Common Solving Technique (1)1.1.2Previous Works (1)1.3Example (1)2Analysis of the Problem (1)2.1Outline of the Approach (1)2.2Basic Assumptions (2)2.3Definitions and Key Terms (2)3Calculating and Simplifying the Model (2)4The Model Results (3)5Validating the Model (3)6Strengths and Weaknesses (3)6.1Strengths (3)6.2Weaknesses (3)7Food for Thought (3)8Conclusion (3)References (4)Appendices (4)Appendix A Source Code (4)Appendix B (4)1Introduction1.1Restatement of the Problem …1.2Background…1.1.1Common Solving Technique…1.1.2Previous Works…1.3Example…2Analysis of the Problem …2.1Outline of the Approach…2.2Basic Assumptions●●●●●2.3Definitions and Key Terms●●●●Table 1.…Symbol Meaning Unit3Calculating and Simplifying the Model …4The Model Results……5Validating the Model…6Strengths and Weaknesses6.1S trengths●●●●6.2W eaknesses●●●●7Food for Thought…8Conclusion….References…AppendicesAppendix A Source CodeHere are the simulation programmes we used in our model as follow. Input matlab source:……….Appendix B…….Input C++ source:…………..…………..。
美赛论文模板
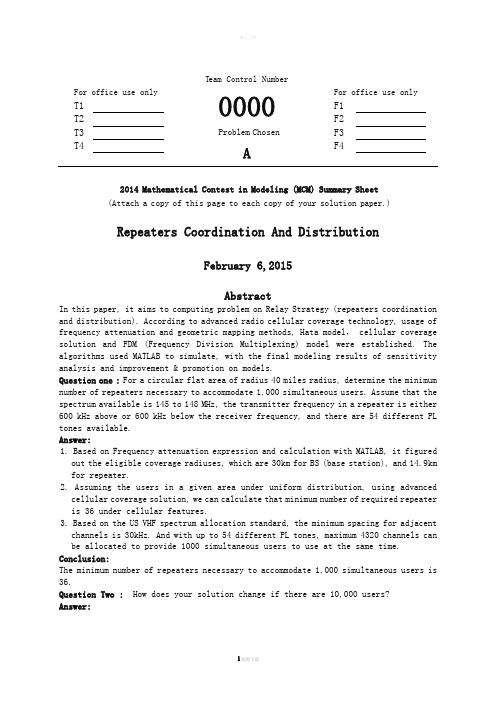
T eam Control NumberFor office use only0000For office use onlyT1 F1T2 F2T3 Problem Chosen F3T4 A F42014 Mathematical Contest in Modeling (MCM) Summary Sheet(Attach a copy of this page to each copy of your solution paper.)Repeaters Coordination And DistributionFebruary 6,2015AbstractIn this paper, it aims to computing problem on Relay Strategy (repeaters coordination and distribution). According to advanced radio cellular coverage technology, usage of frequency attenuation and geometric mapping methods, Hata model, cellular coverage solution and FDM (Frequency Division Multiplexing) model were established. The algorithms used MATLAB to simulate, with the final modeling results of sensitivity analysis and improvement & promotion on models.Question one : For a circular flat area of radius 40 miles radius, determine the minimum number of repeaters necessary to accommodate 1,000 simultaneous users. Assume that the spectrum available is 145 to 148 MHz, the transmitter frequency in a repeater is either 600 kHz above or 600 kHz below the receiver frequency, and there are 54 different PL tones available.Answer:1. Based on Frequency attenuation expression and calculation with MATLAB, it figuredout the eligible coverage radiuses, which are 30km for BS (base station), and 14.9km for repeater.2. Assuming the users in a given area under uniform distribution, using advancedcellular coverage solution, we can calculate that minimum number of required repeater is 36 under cellular features.3. Based on the US VHF spectrum allocation standard, the minimum spacing for adjacentchannels is 30kHz. And with up to 54 different PL tones, maximum 4320 channels can be allocated to provide 1000 simultaneous users to use at the same time. Conclusion:The minimum number of repeaters necessary to accommodate 1,000 simultaneous users is 36.Question Two : How does your solution change if there are 10,000 users?Answer:1. Since the given spectrum is in a fixed range, even if 54 different PL tones can not be allocated enough channels for 10,000 simultaneous users. So the number of repeaters will be increased, meanwhile, the given area will be divided into different parts.2. On the assumption that uniform distribution of the population in the given area, it will be divided into 3 sub-regions equally by analyzing the binding domain, frequency spectrum and PL tones three independent factors. And then the number of repeaters within each sub-region will be classified discussion.3. The FDM (Frequency Division Multiplexing) model is established here to improve channel efficiency to accommodate up to 10,000 simultaneous users Conclusion:The minimum number of repeaters necessary to accommodate 10,000 simultaneous users is 126.Question Three : Discuss the case where there might be defects in line-of-sight propagation caused by mountainous areas. Answer:Basically, under the same condition for question 1&2, the mountainous area will be analyzed as following:1. The function for relationship between radio attenuation x caused by obstacles and the eligible coverage radius d for repeater is 2249.354371.4110x d -=, which is to analyze the impact on the number of repeaters under full signal coverage. 2. For the mountain barrier, based on the different situation of mountains, the addition of repeaters on the suitable location will be discussed to achieve full coverage. This paper describes model established by using of cellular coverage technology and frequency attenuation expression, to achieve simple, fast, accurate algorithm. And also illustrated the effect takes the entire article. In the end, the sensitivity analysis and error calculation are applied for modeling, making the model practically.Key words: Cellular Coverage technology, frequency attenuation expression, channel allocation, MatlabRepeaters coordination and distributionContent1 Restatement of the Problem (1)1.1 Introduction (1)1.2 The Problem (1)2 Simplifying Assumption (1)3 Phrase explain (1)4 Model (2)4.1 Model I (2)4.1.1 Analysis of the Problem (2)4.1.2 Model Design (2)5 Sensitivity analysis (2)6 Model extension (2)7 Evaluating our model (2)7.1 The strengths of model (2)7.2 The weaknesses of model (2)References (3)1 Restatement of the Problem1.1 IntroductionThe VHF radio spectrum involves line-of-sight transmission and reception. This limitation can be overcome by “repeaters,” which pick up weak signals, amplify them, and retransmit them on a different frequency. Thus, using a repeater, low-power users (such as mobile stations) can communicate with one another in situations where direct user-to-user contact would not be possible. However, repeaters can interfere with one another unless they are far enough apart or transmit on sufficiently separated frequencies.1.2 The ProblemYour job is to:◆Design a scheme that determines the minimum number of repeaters necessaryto accommodate 1,000 simultaneous users in a circular flat area of radius40 miles radius.And assume that the spectrum available is 145 to 148 MHz,the transmitter frequency in a repeater is either 600 kHz above or 600 kHz below the receiver frequency, and there are 54 different PL tones available.◆Change your scheme to accommodate 1,0000 simultaneous users base on yourmodel.◆Discuss the case where there might be defects in line-of-sight propagationcaused by mountainous areas.2 Simplifying Assumption3 Phrase explain4 Model4.1 Model I4.1.1 Analysis of the Problem4.1.2 Model Design5 Sensitivity analysisSymbol◆N: the number of total repeaters in the circle area ◆Q: the number of the users in the circle area◆k: the number of the red circle in figure 2最前面最好有一个Symbol List6 Model extension7 Evaluating our model7.1 The strengths of model7.2 The weaknesses of modelReferences参考文献不要引用非常差的期刊的论文,要引用比较厉害的英文期刊,证明你有足够的阅读文献量。
【完整解析】美赛-数学建模-写作模版(各部分)

Summary:clearly describe your approach to the problem and,most prominently,your most important conclusions.●Restatement and clarification of the problem:State in your own words what you aregoing to do.●Explain assumptions and rationale(principle)/justification:Emphasize the assumptionsthat bear on the problem.Clearly list all variables used in your model.●Include your model design and justification for type model used or developed.●Describe model testing and sensitivity analysis,including error analysis,etc.●Discuss the strengths and weaknesses of your model or approach摘要第一段:写论文解决什么问题.1.问题的重述a.介绍重点词开头:例1:“Hand move”irrigation,a cheap but labor-intensive system used on small farms,consists of a movable pipe with sprinkler on top that can be attached to a stationary main.例2:……is a real-life common phenomenon with many complexities.例3:An(effective plan)is crucial to………b.直接指出问题:例1:We find the optimal number of tollbooths in a highway toll-plaza for a given number of highway lanes:the number of tollbooths that minimizes average delay experienced by cars.我们找到了在给定XX的情况下最佳的……例2:A brand-new university needs to balance the cost of information technology security measures with the potential cost of attacks on its systems.XX需要具有B性能的C例3:We determine the number of sprinklers to use by analyzing the energy and motion of water in the pipe and examining the engineering parameters of sprinklers available in the market.我们通过分析参数B确定A,并且检验了现实情况C例4:After mathematically analyzing the……problem,our modeling group would like to present our conclusions,strategies,(and recommendations)to the…….在数学分析B后,我们的模型组将呈现了我们的结论和建议We begin by considering only the rigid recoil effects of the bat–ball col-LisionOur main goal is to understand the sweet spot.A secondary goal is tounderstand the differences between the sweet spots of different bat types.Because the collision happens on such a short time-scale(around1ms),we treat the bat as a free body.That is to say,we are not concerned with the batter’s hands exerting force on the bat that may be transferred to the ball....Our paper is organized as follows....例5:Our goal is...that(minimizes the time)……….2.解决这个问题的伟大意义反面说明。
美国大学生数学建模竞赛 二等奖论文

The P roblem of R epeater C oordination SummaryThis paper mainly focuses on exploring an optimization scheme to serve all the users in a certain area with the least repeaters.The model is optimized better through changing the power of a repeater and distributing PL tones,frequency pairs ing symmetry principle of Graph Theory and maximum coverage principle,we get the most reasonable scheme.This scheme can help us solve the problem that where we should put the repeaters in general cases.It can be suitable for the problem of irrigation,the location of lights in a square and so on.We construct two mathematical models(a basic model and an improve model)to get the scheme based on the relationship between variables.In the basic model,we set a function model to solve the problem under a condition that assumed.There are two variables:‘p’(standing for the power of the signals that a repeater transmits)and‘μ’(standing for the density of users of the area)in the function model.Assume‘p’fixed in the basic one.And in this situation,we change the function model to a geometric one to solve this problem.Based on the basic model,considering the two variables in the improve model is more reasonable to most situations.Then the conclusion can be drawn through calculation and MATLAB programming.We analysis and discuss what we can do if we build repeaters in mountainous areas further.Finally,we discuss strengths and weaknesses of our models and make necessary recommendations.Key words:repeater maximum coverage density PL tones MATLABContents1.Introduction (3)2.The Description of the Problem (3)2.1What problems we are confronting (3)2.2What we do to solve these problems (3)3.Models (4)3.1Basic model (4)3.1.1Terms,Definitions,and Symbols (4)3.1.2Assumptions (4)3.1.3The Foundation of Model (4)3.1.4Solution and Result (5)3.1.5Analysis of the Result (8)3.1.6Strength and Weakness (8)3.1.7Some Improvement (9)3.2Improve Model (9)3.2.1Extra Symbols (10)Assumptions (10)3.2.2AdditionalAdditionalAssumptions3.2.3The Foundation of Model (10)3.2.4Solution and Result (10)3.2.5Analysis of the Result (13)3.2.6Strength and Weakness (14)4.Conclusions (14)4.1Conclusions of the problem (14)4.2Methods used in our models (14)4.3Application of our models (14)5.Future Work (14)6.References (17)7.Appendix (17)Ⅰ.IntroductionIn order to indicate the origin of the repeater coordination problem,the following background is worth mentioning.With the development of technology and society,communications technology has become much more important,more and more people are involved in this.In order to ensure the quality of the signals of communication,we need to build repeaters which pick up weak signals,amplify them,and retransmit them on a different frequency.But the price of a repeater is very high.And the unnecessary repeaters will cause not only the waste of money and resources,but also the difficulty of maintenance.So there comes a problem that how to reduce the number of unnecessary repeaters in a region.We try to explore an optimized model in this paper.Ⅱ.The Description of the Problem2.1What problems we are confrontingThe signals transmit in the way of line-of-sight as a result of reducing the loss of the energy. As a result of the obstacles they meet and the natural attenuation itself,the signals will become unavailable.So a repeater which just picks up weak signals,amplifies them,and retransmits them on a different frequency is needed.However,repeaters can interfere with one another unless they are far enough apart or transmit on sufficiently separated frequencies.In addition to geographical separation,the“continuous tone-coded squelch system”(CTCSS),sometimes nicknamed“private line”(PL),technology can be used to mitigate interference.This system associates to each repeater a separate PL tone that is transmitted by all users who wish to communicate through that repeater. The PL tone is like a kind of password.Then determine a user according to the so called password and the specific frequency,in other words a user corresponds a PL tone(password)and a specific frequency.Defects in line-of-sight propagation caused by mountainous areas can also influence the radius.2.2What we do to solve these problemsConsidering the problem we are confronting,the spectrum available is145to148MHz,the transmitter frequency in a repeater is either600kHz above or600kHz below the receiver frequency.That is only5users can communicate with others without interferences when there’s noPL.The situation will be much better once we have PL.However the number of users that a repeater can serve is limited.In addition,in a flat area ,the obstacles such as mountains ,buildings don’t need to be taken into account.Taking the natural attenuation itself is reasonable.Now the most important is the radius that the signals transmit.Reducing the radius is a good way once there are more users.With MATLAB and the method of the coverage in Graph Theory,we solve this problem as follows in this paper.Ⅲ.Models3.1Basic model3.1.1Terms,Definitions,and Symbols3.1.2Assumptions●A user corresponds a PLz tone (password)and a specific frequency.●The users in the area are fixed and they are uniform distribution.●The area that a repeater covers is a regular hexagon.The repeater is in the center of the regular hexagon.●In a flat area ,the obstacles such as mountains ,buildings don’t need to be taken into account.We just take the natural attenuation itself into account.●The power of a repeater is fixed.3.1.3The Foundation of ModelAs the number of PLz tones (password)and frequencies is fixed,and a user corresponds a PLz tone (password)and a specific frequency,we can draw the conclusion that a repeater can serve the limited number of users.Thus it is clear that the number of repeaters we need relates to the density symboldescriptionLfsdfminrpμloss of transmission the distance of transmission operating frequency the number of repeaters that we need the power of the signals that a repeater transmits the density of users of the areaof users of the area.The radius of the area that a repeater covers is also related to the ratio of d and the radius of the circular area.And d is related to the power of a repeater.So we get the model of function()min ,r f p µ=If we ignore the density of users,we can get a Geometric model as follows:In a plane which is extended by regular hexagons whose side length are determined,we move a circle until it covers the least regular hexagons.3.1.4Solution and ResultCalculating the relationship between the radius of the circle and the side length of the regular hexagon.[]()()32.4420lg ()20lg Lfs dB d km f MHz =++In the above formula the unit of ’’is .Lfs dB The unit of ’’is .d Km The unit of ‘‘is .f MHz We can conclude that the loss of transmission of radio is decided by operating frequency and the distance of transmission.When or is as times as its former data,will increase f d 2[]Lfs .6dB Then we will solve the problem by using the formula mentioned above.We have already known the operating frequency is to .According to the 145MHz 148MHz actual situation and some authority material ,we assume a system whose transmit power is and receiver sensitivity is .Thus we can conclude that ()1010dBm mW +106.85dBm −=.Substituting and to the above formula,we can get the Lfs 106.85dBm −145MHz 148MHz average distance of transmission .()6.4d km =4mile We can learn the radius of the circle is 40mile .So we can conclude the relationship between the circle and the side length of regular hexagon isR=10d.1)The solution of the modelIn order to cover a certain plane with the least regular hexagons,we connect each regular hexagon as the honeycomb.We use A(standing for a figure)covers B(standing for another figure), only when As don’t overlap each other,the number of As we use is the smallest.Figure1According to the Principle of maximum flow of Graph Theory,the better of the symmetry ofthe honeycomb,the bigger area that it covers(Fig1).When the geometric centers of the circle andthe honeycomb which can extend are at one point,extend the honeycomb.Then we can get Fig2,Fig4:Figure2Fig3demos the evenly distribution of users.Figure4Now prove the circle covers the least regular hexagons.Look at Fig5.If we move the circle slightly as the picture,you can see three more regularhexagons are needed.Figure 52)ResultsThe average distance of transmission of the signals that a repeater transmit is 4miles.1000users can be satisfied with 37repeaters founded.3.1.5Analysis of the Result1)The largest number of users that a repeater can serveA user corresponds a PL and a specific frequency.There are 5wave bands and 54different PL tones available.If we call a code include a PL and a specific frequency,there are 54*5=270codes.However each code in two adjacent regular hexagons shouldn’t be the same in case of interfering with each other.In order to have more code available ,we can distribute every 3adjacent regular hexagons 90codes each.And that’s the most optimized,because once any of the three regular hexagons have more codes,it will interfere another one in other regular hexagon.2)Identify the rationality of the basic modelNow we considering the influence of the density of users,according to 1),90*37=3330>1000,so here the number of users have no influence on our model.Our model is rationality.3.1.6Strength and Weakness●Strength:In this paper,we use the model of honeycomb-hexagon structure can maximize the use of resources,avoiding some unnecessary interference effectively.It is much more intuitive once we change the function model to the geometric model.●Weakness:Since each hexagon get too close to another one.Once there are somebuildingsor terrain fluctuations between two repeaters,it can lead to the phenomenon that certain areas will have no signals.In addition,users are distributed evenly is not reasonable.The users are moving,for example some people may get a party.3.1.7Some ImprovementAs we all know,the absolute evenly distribution is not exist.So it is necessary to say something about the normal distribution model.The maximum accommodate number of a repeater is 5*54=270.As for the first model,it is impossible that 270users are communicating in a same repeater.Look at Fig 6.If there are N people in the area 1,the maximum number of the area 2to area 7is 3*(270-N).As 37*90=3330is much larger than 1000,our solution is still reasonable to this model.Figure 63.2Improve Model3.2.1Extra SymbolsSigns and definitions indicated above are still valid.Here are some extra signs and definitions.symboldescription Ra the radius of the circular flat area the side length of a regular hexagon3.2.2Additional AdditionalAssumptionsAssumptions ●The radius that of a repeater covers is adjustable here.●In some limited situations,curved shape is equal to straight line.●Assumptions concerning the anterior process are the same as the Basic Model3.2.3The Foundation of ModelThe same as the Basic Model except that:We only consider one variable(p)in the function model of the basic model ;In this model,we consider two varibles(p and μ)of the function model.3.2.4Solution and Result1)SolutionIf there are 10,000users,the number of regular hexagons that we need is at least ,thus according to the the Principle of maximum flow of Graph Theory,the 10000111.1190=result that we draw needed to be extended further.When the side length of the figure is equal to 7Figure 7regular hexagons,there are 127regular hexagons (Fig 7).Assuming the side length of a regular hexagon is ,then the area of a regular hexagon is a .The area of regular hexagons is equal to a circlewhose radiusis 22a =1000090R.Then according to the formula below:.221000090a R π=We can get.9.5858R a =Mapping with MATLAB as below (Fig 8):Figure 82)Improve the model appropriatelyEnlarge two part of the figure above,we can get two figures below (Fig 9and Fig 10):Figure 9AREAFigure 10Look at the figure above,approximatingAREA a rectangle,then obtaining its area to getthe number of users..The length of the rectangle is approximately equal to the side length of the regular hexagon ,athe width of the rectangle is ,thus the area of AREA is ,then R −*R a ⎛⎞−⎜⎟⎜⎟⎝⎠we can get the number of users in AREA is(),2**10000 2.06R a R π⎡⎤⎛⎞−⎢⎥⎜⎟⎢⎥⎝⎠=⎢⎥⎢⎥⎢⎥⎣⎦9.5858R a =As 2.06<<10,000,2.06can be ignored ,so there is no need to set up a repeater in.There are 6such areas(92,98,104,110,116,122)that can be ignored.At last,the number of repeaters we should set up is,1276121−=2)Get the side length of the regular hexagon of the improved modelThus we can getmile=km 40 4.1729.5858a == 1.6* 6.675a =3)Calculate the power of a repeaterAccording to the formula[]()()32.4420lg ()20lg Lfs dB d km f MHz =++We get32.4420lg 6.67520lg14592.156Los =++=32.4420lg 6.67520lg14892.334Los =++=So we get106.85-92.156=14.694106.85-92.334=14.516As the result in the basic model,we can get the conclusion the power of a repeater is from 14.694mW to 14.516mW.3.2.5Analysis of the ResultAs 10,000users are much more than 1000users,the distribution of the users is more close toevenly distribution.Thus the model is more reasonable than the basic one.More repeaters are built,the utilization of the outside regular hexagon are higher than the former one.3.2.6Strength and Weakness●Strength:The model is more reasonable than the basic one.●Weakness:Repeaters don’t cover all the area,some places may not receive signals.And thefoundation of this model is based on the evenly distribution of the users in the area,if the situation couldn’t be satisfied,the interference of signals will come out.Ⅳ.Conclusions4.1Conclusions of the problem●Generally speaking,the radius of the area that a repeater covers is4miles in our basic model.●Using the model of honeycomb-hexagon structure can maximize the use of resources,avoiding some unnecessary interference effectively.●The minimum number of repeaters necessary to accommodate1,000simultaneous users is37.The minimum number of repeaters necessary to accommodate10,000simultaneoususers is121.●A repeater's coverage radius relates to external environment such as the density of users andobstacles,and it is also determined by the power of the repeater.4.2Methods used in our models●Analysis the problem with MATLAB●the method of the coverage in Graph Theory4.3Application of our models●Choose the ideal address where we set repeater of the mobile phones.●How to irrigate reasonably in agriculture.●How to distribute the lights and the speakers in squares more reasonably.Ⅴ.Future WorkHow we will do if the area is mountainous?5.1The best position of a repeater is the top of the mountain.As the signals are line-of-sight transmission and reception.We must find a place where the signals can transmit from the repeater to users directly.So the top of the mountain is a good place.5.2In mountainous areas,we must increase the number of repeaters.There are three reasons for this problem.One reason is that there will be more obstacles in the mountainous areas. The signals will be attenuated much more quickly than they transmit in flat area.Another reason is that the signals are line-of-sight transmission and reception,we need more repeaters to satisfy this condition.Then look at Fig11and Fig12,and you will know the third reason.It can be clearly seen that hypotenuse is larger than right-angleFig11edge(R>r).Thus the radius will become smaller.In this case more repeaters are needed.Fig125.3In mountainous areas,people may mainly settle in the flat area,so the distribution of users isn’t uniform.5.4There are different altitudes in the mountainous areas.So in order to increase the rate of resources utilization,we can set up the repeaters in different altitudes.5.5However,if there are more repeaters,and some of them are on mountains,more money will be munication companies will need a lot of money to build them,repair them when they don’t work well and so on.As a result,the communication costs will be high.What’s worse,there are places where there are many mountains but few persons. Communication companies reluctant to build repeaters there.But unexpected things often happen in these places.When people are in trouble,they couldn’t communicate well with the outside.So in my opinion,the government should take some measures to solve this problem.5.6Another new method is described as follows(Fig13):since the repeater on high mountains can beFig13Seen easily by people,so the tower which used to transmit and receive signals can be shorter.That is to say,the tower on flat areas can be a little taller..Ⅵ.References[1]YU Fei,YANG Lv-xi,"Effective cooperative scheme based on relay selection",SoutheastUniversity,Nanjing,210096,China[2]YANG Ming,ZHAO Xiao-bo,DI Wei-guo,NAN Bing-xin,"Call Admission Control Policy based on Microcellular",College of Electical and Electronic Engineering,Shijiazhuang Railway Institute,Shijiazhuang Heibei050043,China[3]TIAN Zhisheng,"Analysis of Mechanism of CTCSS Modulation",Shenzhen HYT Co,Shenzhen,518057,China[4]SHANGGUAN Shi-qing,XIN Hao-ran,"Mathematical Modeling in Bass Station Site Selectionwith Lingo Software",China University of Mining And Technology SRES,Xuzhou;Shandong Finance Institute,Jinan Shandon,250014[5]Leif J.Harcke,Kenneth S.Dueker,and David B.Leeson,"Frequency Coordination in the AmateurRadio Emergency ServiceⅦ.AppendixWe use MATLAB to get these pictures,the code is as follows:1-clc;clear all;2-r=1;3-rc=0.7;4-figure;5-axis square6-hold on;7-A=pi/3*[0:6];8-aa=linspace(0,pi*2,80);9-plot(r*exp(i*A),'k','linewidth',2);10-g1=fill(real(r*exp(i*A)),imag(r*exp(i*A)),'k');11-set(g1,'FaceColor',[1,0.5,0])12-g2=fill(real(rc*exp(i*aa)),imag(rc*exp(i*aa)),'k');13-set(g2,'FaceColor',[1,0.5,0],'edgecolor',[1,0.5,0],'EraseMode','x0r')14-text(0,0,'1','fontsize',10);15-Z=0;16-At=pi/6;17-RA=-pi/2;18-N=1;At=-pi/2-pi/3*[0:6];19-for k=1:2;20-Z=Z+sqrt(3)*r*exp(i*pi/6);21-for pp=1:6;22-for p=1:k;23-N=N+1;24-zp=Z+r*exp(i*A);25-zr=Z+rc*exp(i*aa);26-g1=fill(real(zp),imag(zp),'k');27-set(g1,'FaceColor',[1,0.5,0],'edgecolor',[1,0,0]);28-g2=fill(real(zr),imag(zr),'k');29-set(g2,'FaceColor',[1,0.5,0],'edgecolor',[1,0.5,0],'EraseMode',xor';30-text(real(Z),imag(Z),num2str(N),'fontsize',10);31-Z=Z+sqrt(3)*r*exp(i*At(pp));32-end33-end34-end35-ezplot('x^2+y^2=25',[-5,5]);%This is the circular flat area of radius40miles radius 36-xlim([-6,6]*r)37-ylim([-6.1,6.1]*r)38-axis off;Then change number19”for k=1:2;”to“for k=1:3;”,then we get another picture:Change the original programme number19“for k=1:2;”to“for k=1:4;”,then we get another picture:Change the original programme number19“for k=1:2;”to“for k=1:1;”,and delete number 35“ezplot('x^2+y^2=25',[-5,5]);”then we get another picture:Change the original programme number19“for k=1:2;”to“for k=1:6;”,and change number 35“ezplot('x^2+y^2=25',[-5,5]);”to”ezplot('x^2+y^2=6*sqrt(3)*10000/360/pi',[-20,20]);”Change number36,37“36-xlim([-6,6]*r)37-ylim([-6.1,6.1]*r)”To“xlim([-20,20]*r)ylim([-20.1,20.1]*r)”,then we getTeam#8921Page21of21Delete“36-xlim([-6,6]*r)37-ylim([-6.1,6.1]*r)”We get。
美赛论文优秀模版可编辑

2015Mathematical Contest in Modeling (MCM/ICM) Summary SheetIn order to evaluate the performanee of a coach, we describe metrics in five aspects: historicalrecord, game gold content, playoff performanee, honors and contribution to the sports. Moreover, each aspect is subdivided into several sec on dary metrics. Take playoff performa nce as example, we collect postseas on result (Sweet Sixtee n, Final Four, etc.) per year from NCAA official website, Wikimedia and so on.First, ****grade.To eval*** , in turn, are John Wooden, Mike Krzyzewski, Adolph Rupp, Dean Smith and Bob Kni ght.Time line horizon does make a difference. According to turning points in NCAA history, we divide theprevious century into six periods with different time weights which lead to the cha nge of ranking.We con duct sen sitivity an alysis on FSE to find best membership fun cti on and calculati on rule. Sen sitivity an alysis on aggregation weight is also performed. It proves AM performs better than single model. As a creative use,top 3 presidents (U.S.) are picked out: Abraham Lincoln, George Washi ngton, Fran kli n D. RooseveltAt last, the stre ngth and weak ness of our mode are discussed, non-tech ni cal expla nati on is prese nted and the future work is poin ted as well.Key words: Ebola virus diseaseEpidemiology West Africa; ******ContentsI. I ntroduct ion (2)1.1 ............................................................................................................................................................................... 2 For office use only Team Con trol Number For office use only T1 _____________________ 11111 T2 ______________________ F1 _____________________ F2 _____________________ T3 ____________________ T4 ____________________Problem Chose nABCD F3 _____________________ F4 _____________________1.2 (2)1.3 (2)1.4 (2)1.5 (2)1.6 (2)II.The Descripti on of the Problem (2)2.1How do we approximate the whole course of pay ing toll? (2)2.2How do we defi ne the optimal configuration? (3)2.3The local optimizati on and the overall optimization (3)2.4The differe nces in weights and sizes of vehicles (3)2.5What if there is no data available? (3)III....................................................................................................................................................................................... M odels (3)3.1Basic Model (3)3.1.1Terms, Definitions and Symbols (3)3.1.2Assumpti ons (3)3.1.3The Foun datio n of Model (4)3.1.4Soluti on and Result (4)3.1.5An alysis of the Result (4)3.1.6Stren gth and Weakness (4)3.2Improved Model (4)3.2.1Extra Symbols (5)3.2.2Additi onal Assumpti ons (5)3.2.3The Foun datio n of Model (5)3.2.4Soluti on and Result (5)3.2.5An alysis of the Result (6)3.2.6Stren gth and Weakness (6)IV.Con clusi ons (6)4.1Con clusi ons of the problem (6)4.2Methods used in our models (6)4.3Applicati ons of our models (6)V.Future Work (6)5.1Ano ther model (6)5.1.1The limitations of queuing theory (6)5.1.2 (6)5.1.3 (7)5.1.4 (7)5.2Ano ther layout of toll plaza (7)5.3The n ewly- adopted chargi ng methods (7)VI.References (8)VII.Appe ndix (8)I.I ntroductionIn order to indicate the origin of the toll way problems, the following background is worth men ti oning.1.11.21.31.41.51.6II.The Description of the Problem2.1How d o we approximate the whole course of pay ing toll?2.2How d o we defi ne the optimal con figurati on?1)From the perspective of motorist:2)From the perspective of the toll plaza:3)Compromise:2.3The l ocal optimization and the overall optimizationVirtually:2.4The differe nces in weights and sizes of vehicl es2.5What if there is no data availabl e?III.Models3.1Basic Model3.1.1Terms, Definitions and SymbolsThe sig ns and defi niti ons are mostly gen erated fronqueu ing theory.3.1.2Assumpti ons3.1.3The Foun dation of Model1)The utility functionThe cost of toll plaza:The loss of motorist:The weight of each aspect:Compromise:2)The integer programmingAccord ing to queu ing theory, we can calculate the statistical properties as follows.3)The overall optimization and the local optimizationThe overall optimization:The local optimization:The optimal number of tollbooths:3.1.4Solution and Result1)The solution of the integer programming:2)Results:3.1.5An alysis of the ResultLocal optimization and overalloptimization:Sensitivity: The result is quite sen sitive to the cha nge of thehree parametersTrend:Comparison:3.1.6Stre ngth and Weak nessStrength: In despite of this, the model has provedhat . Moreover, we have draw n some usefulcon clusi on sabout . The model is fit for, such asWeakness:This model just applies to . As we havestated, . That' s just whatwe should do in the improved model.3.2 Improved Model3.2.1Extra SymbolsSigns and defi niti ons in dicated above are still valid. Here are some extra sig ns and defi niti ons.3.2.2Additi onal Assumpti onsAssumpti ons concerning the an terior process are the same as the Basic Model.3.2.3The Foun dation of Model1) How do we determine the optimal number?As we have con cluded from the Basic Model,3.2.4Solution and Result1)Simulation algorithmBased on the analysis above, we design our simulation arithmetic as follows.Step1:Step2:Step3:Step4:Step5:Step6:Step7:Step8:Step9:2)Flow chartThe figure below is the flow chart of the simulatio n.3)Solution3.2.5 Analysis of the Result3.2.6 Stre ngth and Weak nessStrength: The Improved Model aims to make up forthe n eglect of . The result seems to declare that this model is more reas on able tha n the Basic Model and much more effective tha n the existi ng desig n.Weakness: . Thus the model is still an approximate on a large scale. This hasdoomed to limit the applications of it.IV. Conclusions4.1 Con clusi ons of the probl em4.2 Methods used in our mod els4.3 Applicati ons of our mod elsV. Future Work5.1 Ano ther model5.1.1 The limitations of queuing theory5.1.25.1.35.1.41)2)3)4)5.2 Ano ther layout of toll plaza5.3 The newly- ad opted charging methodsVI. References[1][2][3][4]VII. Appe ndix。
美赛(mcm)论文LaTeX模板
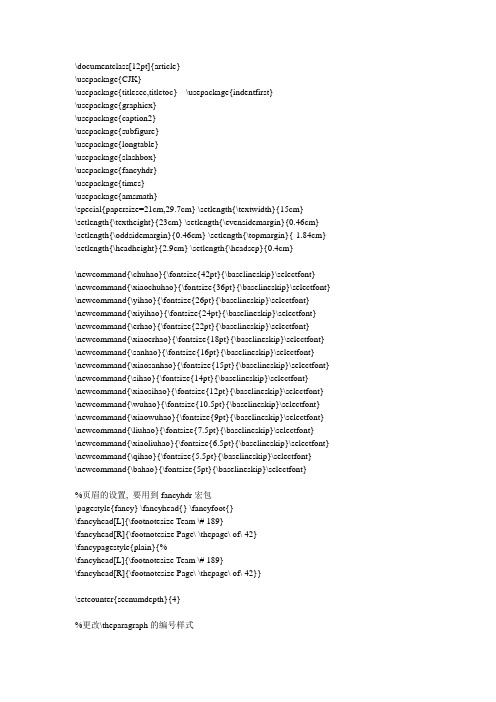
\documentclass[12pt]{article}\usepackage{CJK}\usepackage{titlesec,titletoc} \usepackage{indentfirst}\usepackage{graphicx}\usepackage{caption2}\usepackage{subfigure}\usepackage{longtable}\usepackage{slashbox}\usepackage{fancyhdr}\usepackage{times}\usepackage{amsmath}\special{papersize=21cm,29.7cm} \setlength{\textwidth}{15cm}\setlength{\textheight}{23cm} \setlength{\evensidemargin}{0.46cm}\setlength{\oddsidemargin}{0.46cm} \setlength{\topmargin}{-1.84cm} \setlength{\headheight}{2.9cm} \setlength{\headsep}{0.4cm}\newcommand{\chuhao}{\fontsize{42pt}{\baselineskip}\selectfont}\newcommand{\xiaochuhao}{\fontsize{36pt}{\baselineskip}\selectfont} \newcommand{\yihao}{\fontsize{26pt}{\baselineskip}\selectfont}\newcommand{\xiyihao}{\fontsize{24pt}{\baselineskip}\selectfont}\newcommand{\erhao}{\fontsize{22pt}{\baselineskip}\selectfont}\newcommand{\xiaoerhao}{\fontsize{18pt}{\baselineskip}\selectfont} \newcommand{\sanhao}{\fontsize{16pt}{\baselineskip}\selectfont}\newcommand{\xiaosanhao}{\fontsize{15pt}{\baselineskip}\selectfont} \newcommand{\sihao}{\fontsize{14pt}{\baselineskip}\selectfont}\newcommand{\xiaosihao}{\fontsize{12pt}{\baselineskip}\selectfont} \newcommand{\wuhao}{\fontsize{10.5pt}{\baselineskip}\selectfont} \newcommand{\xiaowuhao}{\fontsize{9pt}{\baselineskip}\selectfont} \newcommand{\liuhao}{\fontsize{7.5pt}{\baselineskip}\selectfont}\newcommand{\xiaoliuhao}{\fontsize{6.5pt}{\baselineskip}\selectfont} \newcommand{\qihao}{\fontsize{5.5pt}{\baselineskip}\selectfont}\newcommand{\bahao}{\fontsize{5pt}{\baselineskip}\selectfont}%页眉的设置, 要用到fancyhdr宏包\pagestyle{fancy} \fancyhead{} \fancyfoot{}\fancyhead[L]{\footnotesize Team \# 189}\fancyhead[R]{\footnotesize Page\ \thepage\ of\ 42}\fancypagestyle{plain}{%\fancyhead[L]{\footnotesize Team \# 189}\fancyhead[R]{\footnotesize Page\ \thepage\ of\ 42}}\setcounter{secnumdepth}{4}%更改\theparagraph的编号样式\makeatletter\renewcommand{\theparagraph}{\@arabic\c@paragraph}\makeatother%章节格式的设置\titleformat{\section}{\erhao\bf}{}{0em}{}[]\titleformat{\subsection}{\xiaoerhao\bf}{}{0em}{}[]\titleformat{\subsubsection}{\sanhao\bf}{}{0em}{}[]\titleformat{\paragraph}[hang]{\vspace*{0.5ex}\sihao\bf}{\hspace*{1em}\theparagraph)}{0.5em }{}[\vspace*{-0.5ex}]%更改插图的标题\renewcommand{\figurename}{\wuhao\bf\sf Figure}\renewcommand{\captionlabeldelim}{\ }%更改表格的标题\renewcommand{\tablename}{\wuhao\bf\sf Table}%更改图形或表格与其标题的间距\setlength{\abovecaptionskip}{10pt}\setlength{\belowcaptionskip}{10pt}%定义产生不浮动图形和表格的标题的命令\figcaption和\tabcaption\makeatletter\newcommand\figcaption{\def\@captype{figure}\caption}\newcommand\tabcaption{\def\@captype{table}\caption}\makeatother%自定义的可以调整粗细的水平线命令, 用于绘制表格, 调用格式\myhline{0.5mm}.\makeatletter\def\myhline#1{%\noalign{\ifnum0=`}\fi\hrule \@height #1 \futurelet\reserved@a\@xhline}\makeatother%第一层列表序号为带圈的阿拉伯数字\renewcommand{\labelenumi}{\textcircled{\arabic{enumi}}}%更改脚注设置\renewcommand{\thefootnote}{\fnsymbol{footnote}}\begin{document}\begin{CJK*}{GBK}{song}\CJKtilde\title{\bf\yihao Aviation Baggage Screening\\{\&} Flight Schedule}\author{}\date{}\maketitle\section{Introduction}Following the terrorist attacks on September 11, 2001, there isintense interest in improving the security screening process forairline passengers and their baggage. Airlines and airports areconsidered high-threat targets for terrorism, so aviation securityis crucial to the safety of the air-travelling public. Bombs andexplosives have been known to be introduced to aircraft by holdbaggage and cargo, carried on by passengers, and hidden withinaircraft supplies.At present To Screen or Not to Screen, that is a Hobson's choice.US Current laws mandate 100{\%} screening of all checked bags at the 429passenger airports throughout the nation by explosive detection systems(EDS) by the end of the Dec 31 2003. However, because the manufacturers arenot able to produce the expected number of EDS required to meet the federalmandate, so it is significant to determine the correct number of devicesdeploy at each airport, and to take advantage of them effectively.The Transportation Security Administration (TSA) needs a complicatedanalysis on how to allocate limited device and how to best use them.Our paper contains the mathematical models to determine the number of EDSsand flight schedules for all airports in Midwest Region. We also discuss theETD devices as the additional security measures and the future developmentof the security systems.\section{Assumption and Hypothesis}\begin{itemize}\item The passengers who will get on the same airplane will arrive uniformly, namely the distribution is flat.\item The detection systems, both EDS and ETD, operate all the time during peak hour, except downtime.\item The airline checks the passengers randomly, according to its claim.\item The passengers, who are just landing and leave out, do not have to be checked through EDS or ETD.\item According to the literature, the aircraft loads approximately equal among the sets of departing flight during the peak hour.\item The landing flight did not affect the departure of the plane.\item Once a passenger arrives, he can go to EDS to be checked, except he has to wait in line.\item Once passengers finish screening, they can broad on the plane in no time.\item During peak hours, a set of flights departs at the same time every the same minutes.\item All the runways are used as much as possible during peak hours.\item The maximum number of the baggage is two, which a passenger can carry on plane. []\item The detection machine examines the bags at the same speed.\item EDS cannot make mistakes that it detect a normal object as an explosive.\end{itemize}\section{Variable and Definition}\begin{longtable}{p{100pt}p{280pt}}\caption{Variables}\\ %第一页表头的标题\endfirsthead %第一页的标题结束\caption{(continued)}\\ %第二页的标题\endhead %第二页的标题结束\hline\hline\textbf{Symbol}&\textbf{Description}\\\hline$n_{ij}$&The airplane number of the $i^{\mathrm{th}}$ type in the $j^{\mathrm{th}}$ flight set\\\hline${NP}_i\:(i=1,2,\ldots)$&The number of passengers on each airplanes of the same type.\\\hline$\xi_{ij}\:(i,j = 1,2,\cdots)$&The number of baggage on each airplane of the $j^{\mathrm{th}}$ flights\\\hline$a$&The maximal number of airplanes type\\\hline$B_j^{set}$&The total baggage number of each set of flight\\\hline${NF}_i$&Number of airplanes of each type\\\hline$\bar{\rho}$&The mean value of passengers' baggage coming per minute in every flight set\\ \hline$N_{set}$&The number of flight sets\\\hline$B_{total}$&The total number of checked baggage during the peak hour\\\hline$H_{peak}$&The length of the peak hour\\\hline$T_{set}$&The time length during which each flight set's passengers wait to be checked\\\hline$\Delta t$&The time interval between two consecutive flight set\\\hline$N_{EDS}$&The number of all the EDSs\\\hline$N_{shadow}$&The number of flight sets whose passengers will be mixed up before being checked\\\hline$v_{EDS}$&The number of baggage checking by one EDS per minute\\\hline$\rho_j$&The number of passengers' baggage coming per minute in one flight set\\\hline$N_{runway}$&The number of an airport's runway\\\hline\\*[-2.2ex]${\bar{B}}^{set}$&The mean value of checked baggage number of every flight set\\\hline$M$&The security cost\\\hline\hline\label{tab1}\end{longtable}\subsubsection{Definition:}\begin{description}\item[Flight set] A group of flights take off at the same time\item[The length of peak hour] The time between the first set of flight and the last set\end{description}\section{Basic Model}During a peak hour, many planes and many passengers would departfrom airports. Therefore, It is difficult to arrange for thepassengers to enter airports. If there are not enough EDSs forpassengers' baggage to check, it will take too long time for themto enter. That would result in the delay of airplanes. On thecontrary, if there are too many EDSs, it will be a waste. It isour task to find a suitable number of EDSs for airport. In orderto reach this objective, we use the linear programming method tosolve it.\subsection{Base analysis}The airplanes are occupied at least partly. The passengers'baggage would be checked by EDSs before they get on the airplanes.We have assumed that every passenger carry two baggages. Thisassumption would simplify the problem. According to the data fromthe problem sheet, we can obtain the useful information thatairlines claim 20{\%} of the passengers do not check any luggage,20{\%} check one bag, and the remaining passengers check two bags.Therefore, we can gain the total number of passengers' baggagethat should be carried on one plane: $\xi_{ij}$. Moreover, we canget the equation that calculate $\xi_{ij}$:\[\xi_{ij}={NP}_i\times 20\%+{NP}_i\times 60\%\times 2\]We define the matrix below as airplane baggage number matrix:\[\overset{\rightharpoonup}{\xi}_j=\left[\xi_{1j}\quad\xi_{2j}\quad\cdots\quad\xi_{ij}\quad\cdots\ right]\]We define the matrix below as flight schedule matrix:\[\left[\begin{array}{llcl}n_{11}&n_{12}&\cdots&n_{1N_{set}}\\n_{21}&n_{22}&\cdots&n_{2N_{set}}\\\multicolumn{4}{c}\dotfill\\n_{a1}&n_{a2}&\cdots&n_{aN_{set}}\end{array}\right]\]In this matrix, $n_{ij}$ is the airplane number of the$i^{\mathrm{th}}$ type in the $j^{\mathrm{th}}$ flight set whichwill take off. Apparently, this value is an integer.We define the matrix below as flight set baggage number matrix:\[\left[B_1^{set}\quad B_2^{set}\quad\cdots\quad B_j^{set}\quad\cdots\quad B_a^{set}\right] \]It is clear that they meet the relation below:\begin{equation}\begin{array}{cl}&\left[\xi_{1j}\quad\xi_{2j}\quad\cdots\quad\xi_{ij}\quad\cdots\right]\cdot\left[\begin{array}{llcl}n_{11}&n_{12}&\cdots&n_{1N_{set}}\\n_{21}&n_{22}&\cdots&n_{2N_{set}}\\\multicolumn{4}{c}\dotfill\\n_{a1}&n_{a2}&\cdots&n_{aN_{set}}\end{array}\right]\\=&\left[B_1^{set}\quad B_2^{set}\quad\cdots\quad B_j^{set}\quad\cdots\quadB_a^{set}\right]\end{array}\label{Flight:baggage}\end{equation}Then, we know:\[B_j^{set}=\sum\limits_{i=1}^a\xi_{ij}\times n_{ij}\]There are some constraints to the equation (\ref{Flight:baggage}).First, for each set of flight, the total number of airplanesshould be less than the number of runways. Second, the totalairplane number of the same type listed in the equation(\ref{Flight:baggage}) from every set of flight should be equal tothe actual airplane number of the same type during the peak hour.We can express them like these:\[\sum\limits_{i=1}^a n_{ij}\le N_{runway}\quad\quad\sum\limits_{j=1}^b n_{ij}={NF}_i \]We should resolve the number of flight sets. According to our assumptions,during the peak hour, the airlines should make the best use of the runways.Then get the number of flight sets approximately based on the number of allthe airplanes during the peak hour and that of the runways. We use anequation below to express this relation:\begin{equation}N_{set}=\left\lceil\frac{\sum\limits_{j=1}^{N_{set}}\sum\limits_{i=1}^an_{ij}}{N_{runway}}\right\rceil\label{sets:number}\end{equation}The checked baggage numbers of each flight set are equal to eachother according to our assumption. We make it based on literature.It can also simplify our model. We define $\bar{B}^{set}$ as themean value of checked baggage number of every flight set.Moreover, We define $\bar{\rho}$ as the mean value of checkedbaggage number of every flight set per minute:\[\bar{B}^{set}=\frac{B_{total}}{N_{set}}\]\[\bar{\rho}=\frac{\bar{B}^{set}}{T_{set}}=\frac{B_{total}}{T_{set}N_{set}}=\frac{B_{total}\Delta t}{T_{set}H_{peak}}\]The course of passengers' arrival and entering airport isimportant for us to decide the number of EDSs and to make the flights schedule. Therefore, we should analyze this process carefully. Passengers will arrive between forty-five minutes andtwo hours prior to the departure time, and the passengers who will get on the same airplane will arrive uniformly. Then we can getthe flow density of all checked baggage at any time during passengers' entering. This value is the sum of numbers of passengers' checked baggage coming per minute. To calculate this value, firstly, we should obtain flow density of each flight set's checked baggage. We define $\rho_j $, namely the number of checked baggage per minute of one flight set:\[\rho_j=\frac{B_j^{set}}{T_{set}}\]Secondly, we draw graphic to help us to understand. We use rectangle to express the time length for all the passengers of one flight set to come and check bags. In the graphic, the black partis the period for them to come. During the white part, no passengers for this flight set come. According to the problem sheet, the former is 75 minute, and the latter is 45 minute. The length of rectangle is 120 minute. $T_{set}$ is the period during which all passengers of one flight set wait to be checked. Sincewe have assumed that each time interval between two consecutive flight set is same value, we define $\Delta t$ as it. Observe the section that value we want to solve is $\sum\limits_j\rho_j$. Moreover, we can get another important equation from the graphic below:\begin{equation}N_{set}=\frac{H_{peak}}{\Delta t}\label{PeakHour}\end{equation}\begin{figure}[hbtp]\centering\includegraphics[width=298.2pt,totalheight=141.6pt]{fig01.eps} \caption{}\label{fig1}\end{figure}Each EDS has certain capacity. If the number of EDSs is $N_{EDS}$and one EDS can check certain number of baggage per minute (Thatis checking velocity, marked by $v_{EDS}$), the total checkingcapacity is $N_{EDS}\cdot\frac{v_{EDS}}{60}$. $v_{EDS}$ is between160 and 210.Now we can easily decide in what condition the passengers can be checkedwithout delay:\[\sum\limits_j\rho_j\le v_{EDS}\]The passengers have to queue before being checked:$\sum\limits_j\rho_j>v_{EDS}$Well then, how can we decide how many $\rho_j$? It depends on howmany flight sets whose passengers will be mixed up before beingchecked. We note it as $N_{shadow} $. Return to the Figure\ref{fig1}, we can know:\[N_{shadow}=\left\lfloor\frac{T_{set}}{\Delta t}\right\rfloor\]\begin{figure}%[htbp]\centering\includegraphics[width=240pt,totalheight=131.4pt]{fig02.eps}\caption{}\label{fig2}\end{figure}From Figure \ref{fig1} and Figure \ref{fig2}, we can get theresult as follows:\begin{enumerate}\item If $N_{shadow}\le N_{set}$, namely $H_{peak}>T_{set}$, then $\sum\limits_{j=1}^{N_{shadow}}\rho _j\le N_{EDS}\frac{v_{EDS}}{60}$\renewcommand{\theequation}{\arabic{equation}a}That is:\begin{equation}N_{EDS}\ge\frac{60}{v_{EDS}}\sum\limits_{j=1}^{N_{shadow}}\rho_j\approx\frac{60}{v_{ EDS}}N_{shadow}\bar{\rho}=\frac{60B_{total}\Deltat}{v_{EDS}T_{set}H_{peak}}N_{shadow}\label{EDS:number:a}\end{equation}\item If $N_{shadow}>N_{set}$, namely $H_{peak}\le T_{set}$, then $\sum\limits_{j=1}^{N_{set}}\rho_j\le N_{EDS}\frac{v_{EDS}}{60}$\setcounter{equation}{3}\renewcommand{\theequation}{\arabic{equation}b}That is:\begin{equation}N_{EDS}\ge\frac{60}{v_{EDS}}\sum\limits_{j=1}^{N_{set}}\rho_j\approx\frac{60}{v_{EDS} }N_{set}\bar{\rho}=\frac{60B_{total}\Delta t}{v_{EDS}T_{set}H_{peak}}N_{set}\label{EDS:number:b}\end{equation}\end{enumerate}\subsection{The number of EDSs}Then we begin to resolve the number of EDSs assisted by the linearprogramming method.EDS is operational about 92{\%} of the time. That is to say, whenever it isduring a peak hour, there are some EDSs stopping working. Then the workingefficiency of all the EDSs is less than the level we have expected.Therefore, the airline has to add more EDSs to do the work, which can bedone with less EDSs without downtime.We use binomial distribution to solve this problem. $N$ is the number ofactual EDSs with downtime and $k$ is the number of EDSs without downtime. Ifprobability is $P$, we can get the equation below:\[\left(\begin{array}{c}N\\k\end{array}\right)\cdot98\%^k\cdot(1-98\%)^{N-k}=P\]We can obtain $N$ when we give $P$ a certain value. In this paper,$P$ is 95{\%}. The $N_{EDS}$ is the actual number we obtainthrough the equation above.Now we have assumed that passengers can be checked unless be delayed by thepeople before him once he arrives at airport. Apparently, if the time lengthbetween two sets of flight is short, the density of passengers will begreat. It will bring great stress to security check and may even make somepassengers miss their flight. To resolve this question, the airline has toinstall more EDSs to meet the demand. However, this measure will cost muchmore money. Consequently, we have to set a suitable time interval betweentwo set of flight.Based on the base analysis above. We can use the equation(\ref{sets:number}) to decide the number of flight sets $N_{set}$assuming we know the number of runways of a certain airport. Thenbased on the equation (\ref{PeakHour}), we can decide the peakhour length $H_{peak}$ when we assume a time interval between two consecutive flight sets. Then we use \textcircled{1} and\textcircled{2} to decide which to choose between equation(\ref{EDS:number:a}) and equation (\ref{EDS:number:b}). In consequence, we can obtain the minimum of EDSs number.If we choose different numbers of runways and the time intervalsbetween two flight sets, we can get different EDSs numbers. Inthis paper that followed, we gain a table of some value of$N_{runway}$ and $\Delta t$ with the corresponding EDSs numbers. Moreover, we draw some figure to reflect their relation.For a certain airport, its number of runway is known. Givencertain time interval ($\Delta t$), we can get the length of thepeak hour ($H_{peak}$). When the $N_{runway}$ is few enough,perhaps $H_{peak}$ is too long to be adopted. However, for acertain airline, they can decide the time interval of their ownpeak hour. In this given time interval, they could find theminimum of $N_{runway}$ through the Figure \ref{fig3}. We draw a sketch map to describe our steps.\begin{figure}[hbtp]\centering\includegraphics[width=352.8pt,totalheight=214.2pt]{fig03.eps}\caption{}\label{fig3}\end{figure}\subsection{The Flight Schedule }According to the base analysis, we can know that the flightschedule matrix and $\Delta t$ is one form of flight timetable. In``The number of EDSs'', we can get suitable $\Delta t$. Then weshould resolve the flight schedule matrix.Because we have assumed that the checked baggage numbers of each flight set are equal to each other. It can be described as follows:\[\left\{\begin{array}{l}\rho_j\approx\bar{\rho}\\B_j^{set}\approx\bar{B}^{set}\end{array}\right.\begin{array}{*{20}c}\hfill&{j=1,2,\cdots,N_{set}}\hfill\end{array}\]The flight schedule matrix subject to this group:\[\left\{\begin{array}{ll}\sum\limits_{j=1}^{N_{set}}n_{ij}={NF}_i&i=1,2,\cdots\\\sum\limits_{i=1}^a n_{ij}\le N_{runway}&j=1,2,\cdots,N_{set}\\n_{ij}\ge0,&\mathrm{and}\:n_{ij}\:\mathrm{is}\:\mathrm{a}\:\mathrm{Integer}\end{array}\right.\]In order to make the best use of runway, we should make$\sum\limits_{i=1}^a n_{ij}$ as great as we can unless it exceed$N_{runway}$.Then we can see that how to resolve the flight schedule matrix is a problemof divide among a group of integers. This group is all the numbers of eachflight passengers' baggage in one flight set. We program for this problemusing MA TLAB and we get at least one solution in the end. However, thematrix elements we have obtained are not integer, we have to adjust them tobe integers manually.\subsection{Results and Interpretation for Airport A and B}The number of passengers in a certain flight (${NP}_i$), the timelength of security checking ($T_{set}$), the checking velocity ofEDS ($v_{EDS}$), and the number of baggage carried by onepassenger are random.\subsubsection{Data Assumption:}\begin{itemize}\item $T_{set}$ is 110 minutes, which is reasonable for airline.\item To simplify the problem, we assume that every passenger carry 2 baggage. If some of thepassengers carry one baggage, the solution based on 2 baggages per passenger meets therequirement.\item The number of runways in airport A and airport B is 5.\end{itemize}\subsubsection{Airport A:}Once the number of runway and the number of the flights aredecided, the flight schedule matrix is decided, too. We producethis matrix using MATLAB. This matrix companied by $\Delta t$ isthe flight schedule for airport A. $\Delta t$ will be calculatedin (\ref{Flight:baggage}), (\ref{sets:number}) and(\ref{PeakHour}).We calculate $N_{EDS}$ and make the flight timetable in threeconditions. The three conditions and the solution are listed asfollowed:\paragraph{Every flight are fully occupied}The checking speed of EDS is 160 bags/hour.\begin{table}[htbp]\centering\caption{}\begin{tabular}{*{11}c}\myhline{0.4mm}$\mathbf{\Deltat(\min)}$&\textbf{2}&\textbf{4}&\textbf{6}&\textbf{8}&\textbf{10}&\textbf{12}&\textbf{14} &\textbf{16}&\textbf{18}&\textbf{20}\\\myhline{0.4mm}$N_{EDS}(\ge)$&31&31&31&31&31&29&24&22&20&17\\\hline$H_{peak}(\min)$&20&40&60&80&100&120&140&160&180&200\\\myhline{0.4mm}\end{tabular}\label{tab2}\end{table}We assume that the suitable value of $H_{peak}$ is 120 minutes.Then the suitable value of $\Delta t$ is about 12 minutes, and$N_{EDS}$ is 29 judged from Figure \ref{fig4}. Certainly, we canwork $\Delta t$ and $N_{EDS}$ out through equation.\begin{figure}[htbp]\centering\includegraphics[width=294.6pt,totalheight=253.2pt]{fig04.eps}\caption{}\label{fig4}\end{figure}\paragraph{Every flight is occupied by the minimal number of passengers onstatistics in the long run.}The checking speed of EDS is 210 bags/hour.\begin{table}[htbp]\centering\caption{}\begin{tabular}{*{11}c}\myhline{0.4mm}$\mathbf{\Deltat(\min)}$&\textbf{2}&\textbf{4}&\textbf{6}&\textbf{8}&\textbf{10}&\textbf{12}&\textbf{14} &\textbf{16}&\textbf{18}&\textbf{20}\\\myhline{0.4mm}$N_{EDS}(\ge)$&15&15&15&15&15&14&13&12&10&7\\\hline$H_{peak}(\min)$&20&40&60&80&100&120&140&160&180&200\\\myhline{0.4mm}\end{tabular}\label{tab3}\end{table}We assume that the suitable value of $H_{peak}$ is 120 minutes.Then the suitable value of $\Delta t$ is about 12 minutes, and$N_{EDS}$ is 14 judging from Figure \ref{fig5}. Certainly, we canwork $\Delta t$ and $N_{EDS}$ out through equation.\begin{figure}[htbp]\centering\includegraphics[width=294.6pt,totalheight=253.2pt]{fig05.eps}\caption{}\label{fig5}\end{figure}\paragraph{${NP}_i$ and $v_{EDS}$ are random value produced by MATLAB.}\begin{table}[htbp]\centering\caption{}\begin{tabular}{*{11}c}\myhline{0.4mm}$\mathbf{\Deltat(\min)}$&\textbf{2}&\textbf{4}&\textbf{6}&\textbf{8}&\textbf{10}&\textbf{12}&\textbf{14} &\textbf{16}&\textbf{18}&\textbf{20}\\\myhline{0.4mm}$N_{EDS}(\ge)$&15&22&21&21&15&17&21&16&13&14\\\hline$H_{peak}(\min)$&20&40&60&80&100&120&140&160&180&200\\\myhline{0.4mm}\end{tabular}\label{tab4}\end{table}We assume that the suitable value of $H_{peak}$ is 120 minutes.Then the suitable value of $\Delta t$ is about 12 minutes, and$N_{EDS}$ is 17 judging from Figure \ref{fig6}. Certainly, we canwork $\Delta t$ and $N_{EDS}$ out through equation.\begin{figure}[htbp]\centering\includegraphics[width=294.6pt,totalheight=249.6pt]{fig06.eps}\caption{}\label{fig6}\end{figure}\subsubsection{Interpretation:}By analyzing the results above, we can conclude that when$N_{EDS}$ is 29, and $\Delta t$ is 12, the flight schedule willmeet requirement at any time. The flight schedule is:\\[\intextsep]\begin{minipage}{\textwidth}\centering\tabcaption{}\begin{tabular}{c|*{8}c|c|c}\myhline{0.4mm}\backslashbox{\textbf{Set}}{\textbf{Type}}&\textbf{1}&\textbf{2}&\textbf{3}&\textbf{4}&\te xtbf{5}&\textbf{6}&\textbf{7}&\textbf{8}&\textbf{Numbers of Bags}&\textbf{Numbers of Flights}\\\myhline{0.4mm}1&2&0&0&0&2&1&0&0&766&5\\\hline2&2&0&2&0&2&0&0&0&732&4\\\hline3&0&1&1&1&2&0&0&0&762&4\\\hline4&0&1&0&0&2&1&0&0&735&4\\\hline5&0&1&0&0&2&1&0&0&735&5\\\hline6&2&0&0&0&1&0&0&1&785&5\\\hline7&2&0&0&0&2&0&1&0&795&5\\\hline8&0&1&0&0&2&1&0&0&735&4\\\hline9&2&0&0&0&2&1&0&0&766&5\\\hline10&0&0&0&2&2&0&0&0&758&5\\\hlineTotal&10&4&3&3&19&5&1&1&7569&46\\\myhline{0.4mm}\end{tabular}\label{tab5}\end{minipage}\\[\intextsep]We have produced random value for ${NP}_i$ and $v_{EDS}$. On thiscondition, the number of EDSs is 17, which is less than 29 that wedecide for the airport A. That is to say our solution can meet thereal requirement.\subsubsection{Airport B:}\paragraph{The passenger load is 100{\%}}The checking speed of EDS is 160 bags/hour.\begin{table}[htbp]\centering\caption{}\begin{tabular}{*{11}c}\myhline{0.4mm}$\mathbf{\Deltat(\min)}$&\textbf{2}&\textbf{4}&\textbf{6}&\textbf{8}&\textbf{10}&\textbf{12}&\textbf{14} &\textbf{16}&\textbf{18}&\textbf{20}\\\myhline{0.4mm}$N_{EDS}(\ge)$&33&33&33&33&33&30&27&23&21&19\\\hline$H_{peak}(\min)$&20&40&60&80&100&120&140&160&180&200\\\myhline{0.4mm}\end{tabular}\label{tab6}\end{table}We assume that the suitable value of $H_{peak}$ is 120 minutes.Then the suitable value of $\Delta t$ is about 12 minutes, and。
数学建模美赛写作模版(包含摘要、格式、总结、表格、公式、图表、假设)
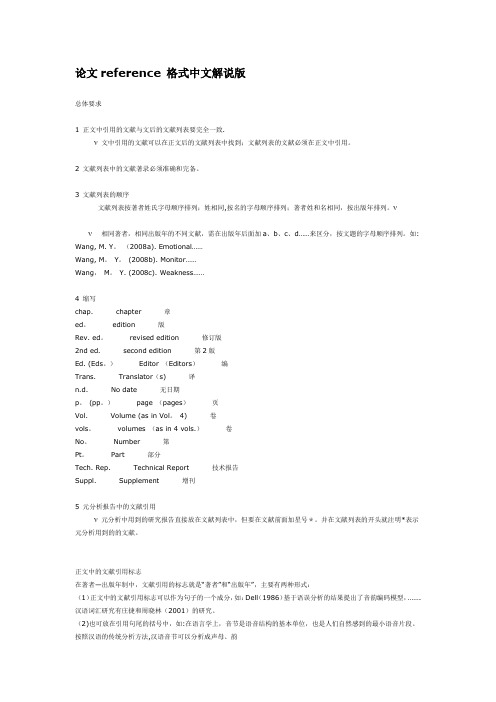
论文reference 格式中文解说版总体要求1 正文中引用的文献与文后的文献列表要完全一致.ν文中引用的文献可以在正文后的文献列表中找到;文献列表的文献必须在正文中引用。
2 文献列表中的文献著录必须准确和完备。
3 文献列表的顺序文献列表按著者姓氏字母顺序排列;姓相同,按名的字母顺序排列;著者姓和名相同,按出版年排列。
νν相同著者,相同出版年的不同文献,需在出版年后面加a、b、c、d……来区分,按文题的字母顺序排列。
如: Wang, M. Y。
(2008a). Emotional……Wang, M。
Y。
(2008b). Monitor……Wang,M。
Y. (2008c). Weakness……4 缩写chap. chapter 章ed。
edition 版Rev. ed。
revised edition 修订版2nd ed. second edition 第2版Ed. (Eds。
)Editor (Editors)编Trans. Translator(s) 译n.d. No date 无日期p。
(pp。
)page (pages)页Vol. Volume (as in Vol。
4) 卷vols。
volumes (as in 4 vols.)卷No。
Number 第Pt。
Part 部分Tech. Rep. Technical Report 技术报告Suppl. Supplement 增刊5 元分析报告中的文献引用ν元分析中用到的研究报告直接放在文献列表中,但要在文献前面加星号*。
并在文献列表的开头就注明*表示元分析用到的的文献。
正文中的文献引用标志在著者—出版年制中,文献引用的标志就是“著者”和“出版年”,主要有两种形式:(1)正文中的文献引用标志可以作为句子的一个成分,如:Dell(1986)基于语误分析的结果提出了音韵编码模型,…….汉语词汇研究有庄捷和周晓林(2001)的研究。
(2)也可放在引用句尾的括号中,如:在语言学上,音节是语音结构的基本单位,也是人们自然感到的最小语音片段。
美赛论文格式及注意事项
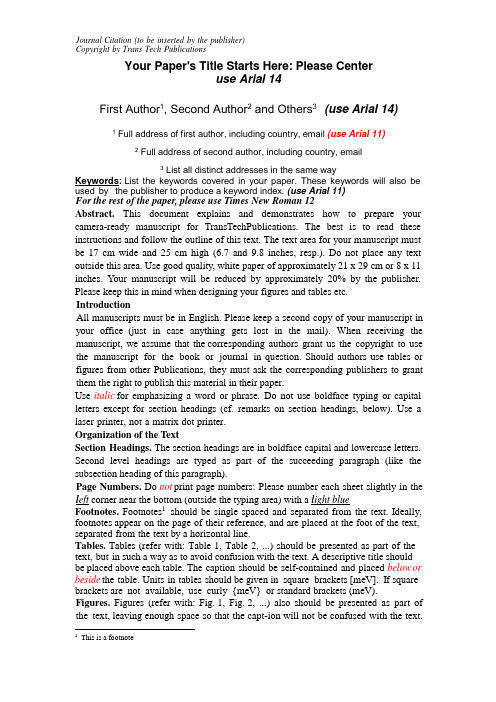
Your Paper's Title Starts Here: Please Centeruse Arial 14First Author1, Second Author2 and Others3 (use Arial 14)1 Full address of first author, including country, email (use Arial 11)2 Full address of second author, including country, email3 List all distinct addresses in the same wayKeywords: List the keywords covered in your paper. These keywords will also be(use Arial 11)For the rest of the paper, please use Times New Roman 12Abstract. This document explains and demonstrates how to prepare your camera-ready manuscript for TransTechPublications. The best is to read these instructions and follow the outline of this text. The text area for your manuscript must be 17 cm wide and 25 cm high (6.7 and 9.8 inches, resp.). Do not place any text outside this area. Use good quality, white paper of approximately 21 x 29 cm or 8 x 11 inches. Y our manuscript will be reduced by approximately 20% by the publisher. Please keep this in mind when designing your figures and tables etc.IntroductionAll manuscripts must be in English. Please keep a second copy of your manuscript in your office (just in case anything gets lost in the mail). When receiving the manuscript, we assume that the corresponding authors grant us the copyright to use the manuscript for the book or journal in question. Should authors use tables or figures from other Publications, they must ask the corresponding publishers to grant them the right to publish this material in their paper.Use italic for emphasizing a word or phrase. Do not use boldface typing or capital letters except for section headings (cf. remarks on section headings, below). Use a laser printer, not a matrix dot printer.Organization of the TextSection Headings. The section headings are in boldface capital and lowercase letters. Second level headings are typed as part of the succeeding paragraph (like the subsection heading of this paragraph).Page Numbers. Do not print page numbers: Please number each sheet slightly in the corner near the bottom (outside the typing area) with aFootnotes. Footnotes1should be single spaced and separated from the text. Ideally, footnotes appear on the page of their reference, and are placed at the foot of the text, separated from the text by a horizontal line.Tables. Tables (refer with: Table 1, Table 2, ...) should be presented as part of the text, but in such a way as to avoid confusion with the text. A descriptive title should be placed above each table. The caption should be self-contained and placed below or beside the table. Units in tables should be given in square brackets [meV]. If square brackets are not available, use curly {meV} or standard brackets (meV). Figures. Figures (refer with: Fig. 1, Fig. 2, ...) also should be presented as part o f the text, leaving enough space so that the capt-ion will not be confused with the text. 1This is a footnoteThe caption should be self-contained and placed below or beside the figure. Generally, only original drawings or photographic reproductions are acceptable. Only very good photocopies are acceptable. Utmost care must be taken to insert the figures incorrect alignment with the text. Half-tone pictures should be in the form of glossy prints. If possible, please include your figures as graphic images in the electronic version. If TTP is required to scan and insert images, please keep the following points in mind:(a) the allotted space (for inserting illustrations) must exactly match the space made available in the camera-ready version, so that the electronic version is identical to the hard copy with regard to page and line breaks.(b) the required positioning of any high-quality separate illustration must be clearly indicated on its reverse side. The size of the illustrations must exactly match the space left in the camera-ready manuscript.Equations. Equations (refer with: Eq. 1, Eq. 2, ...) should be indented 5 mm (0.2").There should be one line of space above the equation and one line of space below itbefore the text continues. The equations have to be numbered sequentially, and the number put in parentheses at the right-hand edge of the text. Equations should bepunctuated as if they were an ordinary part of the text. Punctuation appears after theequation but before the equation number, e.g.c2 = a2 + b2. (1)Literature ReferencesReferences are cited in the text just by square brackets [1]. (If square brackets are not available, slashes may be used instead, e.g. /2/.) Two or more references at a time may be put in one set of brackets [3,4]. The references are to be numbered in the order in which they are cited in the text and are to be listed at the end of the contribution under a heading References, see our example below.SummaryOn your floppy disk, please indicate the format and word processor used. Please also provide your phone number, fax number and e-mail address for rapid communication with the publisher (will not be published). Please always send your disk along with a hard copy that must match the disk's content exactly. If you follow the foregoing, your paper will conform to the requirements of the publisher and facilitate a problem-free publication process.References[1] Dj.M. Maric, P.F. Meier and S.K. Estreicher: Mater. Sci. Forum Vol. 83-87 (1992), p. 119[2] M.A. Green: High Efficiency Silicon Solar Cells (Trans Tech Publications, Switzerland 1987).。
美赛论文LaTeX模板
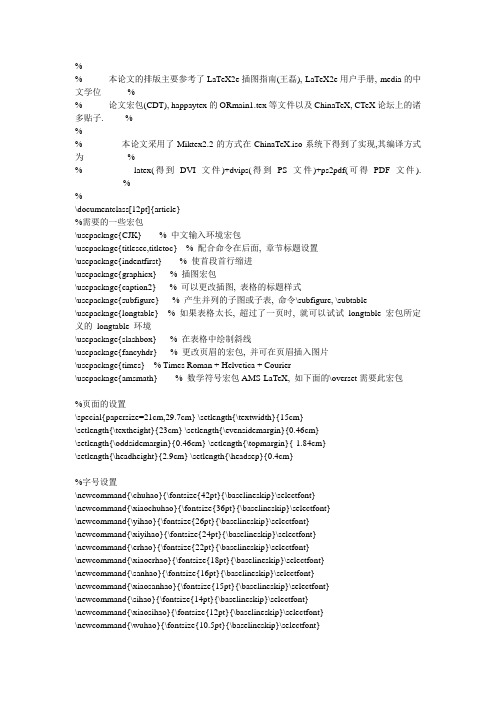
%% 本论文的排版主要参考了LaTeX2e插图指南(王磊), LaTeX2e用户手册, media的中文学位%% 论文宏包(CDT), happaytex的ORmain1.tex等文件以及ChinaTeX, CTeX论坛上的诸多贴子. %%% 本论文采用了Miktex2.2的方式在ChinaTeX.iso系统下得到了实现,其编译方式为%% latex(得到DVI文件)+dvips(得到PS文件)+ps2pdf(可得PDF文件).%%\documentclass[12pt]{article}%需要的一些宏包\usepackage{CJK} % 中文输入环境宏包\usepackage{titlesec,titletoc} % 配合命令在后面, 章节标题设置\usepackage{indentfirst} % 使首段首行缩进\usepackage{graphicx} % 插图宏包\usepackage{caption2} % 可以更改插图, 表格的标题样式\usepackage{subfigure} % 产生并列的子图或子表, 命令\subfigure, \subtable\usepackage{longtable} % 如果表格太长, 超过了一页时, 就可以试试longtable 宏包所定义的longtable 环境\usepackage{slashbox} % 在表格中绘制斜线\usepackage{fancyhdr} % 更改页眉的宏包, 并可在页眉插入图片\usepackage{times} % Times Roman + Helvetica + Courier\usepackage{amsmath} % 数学符号宏包AMS-LaTeX, 如下面的\overset需要此宏包%页面的设置\special{papersize=21cm,29.7cm} \setlength{\textwidth}{15cm}\setlength{\textheight}{23cm} \setlength{\evensidemargin}{0.46cm}\setlength{\oddsidemargin}{0.46cm} \setlength{\topmargin}{-1.84cm}\setlength{\headheight}{2.9cm} \setlength{\headsep}{0.4cm}%字号设置\newcommand{\chuhao}{\fontsize{42pt}{\baselineskip}\selectfont}\newcommand{\xiaochuhao}{\fontsize{36pt}{\baselineskip}\selectfont}\newcommand{\yihao}{\fontsize{26pt}{\baselineskip}\selectfont}\newcommand{\xiyihao}{\fontsize{24pt}{\baselineskip}\selectfont}\newcommand{\erhao}{\fontsize{22pt}{\baselineskip}\selectfont}\newcommand{\xiaoerhao}{\fontsize{18pt}{\baselineskip}\selectfont}\newcommand{\sanhao}{\fontsize{16pt}{\baselineskip}\selectfont}\newcommand{\xiaosanhao}{\fontsize{15pt}{\baselineskip}\selectfont}\newcommand{\sihao}{\fontsize{14pt}{\baselineskip}\selectfont}\newcommand{\xiaosihao}{\fontsize{12pt}{\baselineskip}\selectfont}\newcommand{\wuhao}{\fontsize{10.5pt}{\baselineskip}\selectfont}\newcommand{\xiaowuhao}{\fontsize{9pt}{\baselineskip}\selectfont}\newcommand{\liuhao}{\fontsize{7.5pt}{\baselineskip}\selectfont}\newcommand{\xiaoliuhao}{\fontsize{6.5pt}{\baselineskip}\selectfont}\newcommand{\qihao}{\fontsize{5.5pt}{\baselineskip}\selectfont}\newcommand{\bahao}{\fontsize{5pt}{\baselineskip}\selectfont}%页眉的设置, 要用到fancyhdr宏包\pagestyle{fancy} \fancyhead{} \fancyfoot{}\fancyhead[L]{\footnotesize Team \# 189}\fancyhead[R]{\footnotesize Page\ \thepage\ of\ 42}\fancypagestyle{plain}{%\fancyhead[L]{\footnotesize Team \# 189}\fancyhead[R]{\footnotesize Page\ \thepage\ of\ 42}}\setcounter{secnumdepth}{4}%更改\theparagraph的编号样式\makeatletter\renewcommand{\theparagraph}{\@arabic\c@paragraph}\makeatother%章节格式的设置\titleformat{\section}{\erhao\bf}{}{0em}{}[]\titleformat{\subsection}{\xiaoerhao\bf}{}{0em}{}[]\titleformat{\subsubsection}{\sanhao\bf}{}{0em}{}[]\titleformat{\paragraph}[hang]{\vspace*{0.5ex}\sihao\bf}{\hspace*{1em}\theparagraph)}{0.5em }{}[\vspace*{-0.5ex}]%更改插图的标题\renewcommand{\figurename}{\wuhao\bf\sf Figure}\renewcommand{\captionlabeldelim}{\ }%更改表格的标题\renewcommand{\tablename}{\wuhao\bf\sf Table}%更改图形或表格与其标题的间距\setlength{\abovecaptionskip}{10pt}\setlength{\belowcaptionskip}{10pt}%定义产生不浮动图形和表格的标题的命令\figcaption和\tabcaption\makeatletter\newcommand\figcaption{\def\@captype{figure}\caption}\newcommand\tabcaption{\def\@captype{table}\caption}\makeatother%自定义的可以调整粗细的水平线命令, 用于绘制表格, 调用格式\myhline{0.5mm}. \makeatletter\def\myhline#1{%\noalign{\ifnum0=`}\fi\hrule \@height #1 \futurelet\reserved@a\@xhline}\makeatother%第一层列表序号为带圈的阿拉伯数字\renewcommand{\labelenumi}{\textcircled{\arabic{enumi}}}%更改脚注设置\renewcommand{\thefootnote}{\fnsymbol{footnote}}\begin{document}\begin{CJK*}{GBK}{song}\CJKtilde\title{\bf\yihao Aviation Baggage Screening\\{\&} Flight Schedule}\author{}\date{}\maketitle\section{Introduction}Following the terrorist attacks on September 11, 2001, there isintense interest in improving the security screening process forairline passengers and their baggage. Airlines and airports areconsidered high-threat targets for terrorism, so aviation securityis crucial to the safety of the air-travelling public. Bombs andexplosives have been known to be introduced to aircraft by holdbaggage and cargo, carried on by passengers, and hidden withinaircraft supplies.At present To Screen or Not to Screen, that is a Hobson's choice.US Current laws mandate 100{\%} screening of all checked bags at the 429 passenger airports throughout the nation by explosive detection systems(EDS) by the end of the Dec 31 2003. However, because the manufacturers arenot able to produce the expected number of EDS required to meet the federal mandate, so it is significant to determine the correct number of devicesdeploy at each airport, and to take advantage of them effectively.The Transportation Security Administration (TSA) needs a complicatedanalysis on how to allocate limited device and how to best use them.Our paper contains the mathematical models to determine the number of EDSsand flight schedules for all airports in Midwest Region. We also discuss theETD devices as the additional security measures and the future developmentof the security systems.\section{Assumption and Hypothesis}\begin{itemize}\item The passengers who will get on the same airplane will arrive uniformly, namely the distribution is flat.\item The detection systems, both EDS and ETD, operate all the time during peak hour, except downtime.\item The airline checks the passengers randomly, according to its claim.\item The passengers, who are just landing and leave out, do not have to be checked through EDS or ETD.\item According to the literature, the aircraft loads approximately equal among the sets of departing flight during the peak hour.\item The landing flight did not affect the departure of the plane.\item Once a passenger arrives, he can go to EDS to be checked, except he has to wait in line.\item Once passengers finish screening, they can broad on the plane in no time.\item During peak hours, a set of flights departs at the same time every the same minutes.\item All the runways are used as much as possible during peak hours.\item The maximum number of the baggage is two, which a passenger can carry on plane. []\item The detection machine examines the bags at the same speed.\item EDS cannot make mistakes that it detect a normal object as an explosive.\end{itemize}\section{Variable and Definition}\begin{longtable}{p{100pt}p{280pt}}\caption{Variables}\\ %第一页表头的标题\endfirsthead %第一页的标题结束\caption{(continued)}\\ %第二页的标题\endhead %第二页的标题结束\hline\hline\textbf{Symbol}&\textbf{Description}\\\hline$n_{ij}$&The airplane number of the $i^{\mathrm{th}}$ type in the $j^{\mathrm{th}}$ flight set\\\hline${NP}_i\:(i=1,2,\ldots)$&The number of passengers on each airplanes of the same type.\\\hline$\xi_{ij}\:(i,j = 1,2,\cdots)$&The number of baggage on each airplane of the $j^{\mathrm{th}}$ flights\\\hline$a$&The maximal number of airplanes type\\\hline$B_j^{set}$&The total baggage number of each set of flight\\\hline${NF}_i$&Number of airplanes of each type\\\hline$\bar{\rho}$&The mean value of passengers' baggage coming per minute in every flight set\\ \hline$N_{set}$&The number of flight sets\\\hline$B_{total}$&The total number of checked baggage during the peak hour\\\hline$H_{peak}$&The length of the peak hour\\\hline$T_{set}$&The time length during which each flight set's passengers wait to be checked\\\hline$\Delta t$&The time interval between two consecutive flight set\\\hline$N_{EDS}$&The number of all the EDSs\\\hline$N_{shadow}$&The number of flight sets whose passengers will be mixed up before being checked\\\hline$v_{EDS}$&The number of baggage checking by one EDS per minute\\\hline$\rho_j$&The number of passengers' baggage coming per minute in one flight set\\\hline$N_{runway}$&The number of an airport's runway\\\hline\\*[-2.2ex]${\bar{B}}^{set}$&The mean value of checked baggage number of every flight set\\\hline$M$&The security cost\\\hline\hline\label{tab1}\end{longtable}\subsubsection{Definition:}\begin{description}\item[Flight set] A group of flights take off at the same time\item[The length of peak hour] The time between the first set of flight and the last set\end{description}\section{Basic Model}During a peak hour, many planes and many passengers would departfrom airports. Therefore, It is difficult to arrange for thepassengers to enter airports. If there are not enough EDSs forpassengers' baggage to check, it will take too long time for themto enter. That would result in the delay of airplanes. On thecontrary, if there are too many EDSs, it will be a waste. It isour task to find a suitable number of EDSs for airport. In orderto reach this objective, we use the linear programming method tosolve it.\subsection{Base analysis}The airplanes are occupied at least partly. The passengers'baggage would be checked by EDSs before they get on the airplanes.We have assumed that every passenger carry two baggages. Thisassumption would simplify the problem. According to the data fromthe problem sheet, we can obtain the useful information thatairlines claim 20{\%} of the passengers do not check any luggage,20{\%} check one bag, and the remaining passengers check two bags.Therefore, we can gain the total number of passengers' baggagethat should be carried on one plane: $\xi_{ij}$. Moreover, we canget the equation that calculate $\xi_{ij}$:\[\xi_{ij}={NP}_i\times 20\%+{NP}_i\times 60\%\times 2\]We define the matrix below as airplane baggage number matrix:\[\overset{\rightharpoonup}{\xi}_j=\left[\xi_{1j}\quad\xi_{2j}\quad\cdots\quad\xi_{ij}\quad\cdots\ right]\]We define the matrix below as flight schedule matrix:\[\left[\begin{array}{llcl}n_{11}&n_{12}&\cdots&n_{1N_{set}}\\n_{21}&n_{22}&\cdots&n_{2N_{set}}\\\multicolumn{4}{c}\dotfill\\n_{a1}&n_{a2}&\cdots&n_{aN_{set}}\end{array}\right]\]In this matrix, $n_{ij}$ is the airplane number of the$i^{\mathrm{th}}$ type in the $j^{\mathrm{th}}$ flight set whichwill take off. Apparently, this value is an integer.We define the matrix below as flight set baggage number matrix:\[\left[B_1^{set}\quad B_2^{set}\quad\cdots\quad B_j^{set}\quad\cdots\quad B_a^{set}\right] \]It is clear that they meet the relation below:\begin{equation}\begin{array}{cl}&\left[\xi_{1j}\quad\xi_{2j}\quad\cdots\quad\xi_{ij}\quad\cdots\right]\cdot\left[\begin{array}{llcl}n_{11}&n_{12}&\cdots&n_{1N_{set}}\\n_{21}&n_{22}&\cdots&n_{2N_{set}}\\\multicolumn{4}{c}\dotfill\\n_{a1}&n_{a2}&\cdots&n_{aN_{set}}\end{array}\right]\\=&\left[B_1^{set}\quad B_2^{set}\quad\cdots\quad B_j^{set}\quad\cdots\quad B_a^{set}\right]\end{array}\label{Flight:baggage}\end{equation}Then, we know:\[B_j^{set}=\sum\limits_{i=1}^a\xi_{ij}\times n_{ij}\]There are some constraints to the equation (\ref{Flight:baggage}).First, for each set of flight, the total number of airplanesshould be less than the number of runways. Second, the totalairplane number of the same type listed in the equation(\ref{Flight:baggage}) from every set of flight should be equal tothe actual airplane number of the same type during the peak hour.We can express them like these:\[\sum\limits_{i=1}^a n_{ij}\le N_{runway}\quad\quad\sum\limits_{j=1}^b n_{ij}={NF}_i \]We should resolve the number of flight sets. According to our assumptions,during the peak hour, the airlines should make the best use of the runways.Then get the number of flight sets approximately based on the number of allthe airplanes during the peak hour and that of the runways. We use anequation below to express this relation:\begin{equation}N_{set}=\left\lceil\frac{\sum\limits_{j=1}^{N_{set}}\sum\limits_{i=1}^an_{ij}}{N_{runway}}\right\rceil\label{sets:number}\end{equation}The checked baggage numbers of each flight set are equal to eachother according to our assumption. We make it based on literature.It can also simplify our model. We define $\bar{B}^{set}$ as themean value of checked baggage number of every flight set.Moreover, We define $\bar{\rho}$ as the mean value of checkedbaggage number of every flight set per minute:\[\bar{B}^{set}=\frac{B_{total}}{N_{set}}\]\[\bar{\rho}=\frac{\bar{B}^{set}}{T_{set}}=\frac{B_{total}}{T_{set}N_{set}}=\frac{B_{total}\ Delta t}{T_{set}H_{peak}}\]The course of passengers' arrival and entering airport isimportant for us to decide the number of EDSs and to make theflights schedule. Therefore, we should analyze this processcarefully. Passengers will arrive between forty-five minutes andtwo hours prior to the departure time, and the passengers who willget on the same airplane will arrive uniformly. Then we can getthe flow density of all checked baggage at any time duringpassengers' entering. This value is the sum of numbers ofpassengers' checked baggage coming per minute. To calculate thisvalue, firstly, we should obtain flow density of each flight set'schecked baggage. We define $\rho_j $, namely the number of checkedbaggage per minute of one flight set:\[\rho_j=\frac{B_j^{set}}{T_{set}}\]Secondly, we draw graphic to help us to understand. We userectangle to express the time length for all the passengers of oneflight set to come and check bags. In the graphic, the black partis the period for them to come. During the white part, nopassengers for this flight set come. According to the problemsheet, the former is 75 minute, and the latter is 45 minute. Thelength of rectangle is 120 minute. $T_{set}$ is the period duringwhich all passengers of one flight set wait to be checked. Sincewe have assumed that each time interval between two consecutiveflight set is same value, we define $\Delta t$ as it. Observe thesection that value we want to solve is $\sum\limits_j\rho_j$. Moreover, we can get another important equation from the graphic below:\begin{equation}N_{set}=\frac{H_{peak}}{\Delta t}\label{PeakHour}\end{equation}\begin{figure}[hbtp]\centering\includegraphics[width=298.2pt,totalheight=141.6pt]{fig01.eps}\caption{}\label{fig1}\end{figure}Each EDS has certain capacity. If the number of EDSs is $N_{EDS}$ and one EDS can check certain number of baggage per minute (Thatis checking velocity, marked by $v_{EDS}$), the total checking capacity is $N_{EDS}\cdot\frac{v_{EDS}}{60}$. $v_{EDS}$ is between 160 and 210.Now we can easily decide in what condition the passengers can be checked without delay:\[\sum\limits_j\rho_j\le v_{EDS}\]The passengers have to queue before being checked:$\sum\limits_j\rho_j>v_{EDS}$Well then, how can we decide how many $\rho_j$? It depends on how many flight sets whose passengers will be mixed up before being checked. We note it as $N_{shadow} $. Return to the Figure\ref{fig1}, we can know:\[N_{shadow}=\left\lfloor\frac{T_{set}}{\Delta t}\right\rfloor\]\begin{figure}%[htbp]\centering\includegraphics[width=240pt,totalheight=131.4pt]{fig02.eps}\caption{}\label{fig2}\end{figure}From Figure \ref{fig1} and Figure \ref{fig2}, we can get theresult as follows:\begin{enumerate}\item If $N_{shadow}\le N_{set}$, namely $H_{peak}>T_{set}$, then $\sum\limits_{j=1}^{N_{shadow}}\rho _j\le N_{EDS}\frac{v_{EDS}}{60}$\renewcommand{\theequation}{\arabic{equation}a}That is:\begin{equation}N_{EDS}\ge\frac{60}{v_{EDS}}\sum\limits_{j=1}^{N_{shadow}}\rho_j\approx\frac{60}{v_{ EDS}}N_{shadow}\bar{\rho}=\frac{60B_{total}\Deltat}{v_{EDS}T_{set}H_{peak}}N_{shadow}\label{EDS:number:a}\end{equation}\item If $N_{shadow}>N_{set}$, namely $H_{peak}\le T_{set}$, then $\sum\limits_{j=1}^{N_{set}}\rho_j\le N_{EDS}\frac{v_{EDS}}{60}$\setcounter{equation}{3}\renewcommand{\theequation}{\arabic{equation}b}That is:\begin{equation}N_{EDS}\ge\frac{60}{v_{EDS}}\sum\limits_{j=1}^{N_{set}}\rho_j\approx\frac{60}{v_{EDS} }N_{set}\bar{\rho}=\frac{60B_{total}\Delta t}{v_{EDS}T_{set}H_{peak}}N_{set}\label{EDS:number:b}\end{equation}\end{enumerate}\subsection{The number of EDSs}Then we begin to resolve the number of EDSs assisted by the linearprogramming method.EDS is operational about 92{\%} of the time. That is to say, whenever it isduring a peak hour, there are some EDSs stopping working. Then the workingefficiency of all the EDSs is less than the level we have expected.Therefore, the airline has to add more EDSs to do the work, which can bedone with less EDSs without downtime.We use binomial distribution to solve this problem. $N$ is the number ofactual EDSs with downtime and $k$ is the number of EDSs without downtime. Ifprobability is $P$, we can get the equation below:\[\left(\begin{array}{c}N\\k\end{array}\right)\cdot98\%^k\cdot(1-98\%)^{N-k}=P\]We can obtain $N$ when we give $P$ a certain value. In this paper,$P$ is 95{\%}. The $N_{EDS}$ is the actual number we obtainthrough the equation above.Now we have assumed that passengers can be checked unless be delayed by the people before him once he arrives at airport. Apparently, if the time length between two sets of flight is short, the density of passengers will begreat. It will bring great stress to security check and may even make some passengers miss their flight. To resolve this question, the airline has toinstall more EDSs to meet the demand. However, this measure will cost much more money. Consequently, we have to set a suitable time interval between two set of flight.Based on the base analysis above. We can use the equation(\ref{sets:number}) to decide the number of flight sets $N_{set}$assuming we know the number of runways of a certain airport. Thenbased on the equation (\ref{PeakHour}), we can decide the peakhour length $H_{peak}$ when we assume a time interval between two consecutive flight sets. Then we use \textcircled{1} and\textcircled{2} to decide which to choose between equation(\ref{EDS:number:a}) and equation (\ref{EDS:number:b}). In consequence, we can obtain the minimum of EDSs number.If we choose different numbers of runways and the time intervalsbetween two flight sets, we can get different EDSs numbers. Inthis paper that followed, we gain a table of some value of$N_{runway}$ and $\Delta t$ with the corresponding EDSs numbers. Moreover, we draw some figure to reflect their relation.For a certain airport, its number of runway is known. Givencertain time interval ($\Delta t$), we can get the length of thepeak hour ($H_{peak}$). When the $N_{runway}$ is few enough,perhaps $H_{peak}$ is too long to be adopted. However, for acertain airline, they can decide the time interval of their ownpeak hour. In this given time interval, they could find theminimum of $N_{runway}$ through the Figure \ref{fig3}. We draw asketch map to describe our steps.\begin{figure}[hbtp]\centering\includegraphics[width=352.8pt,totalheight=214.2pt]{fig03.eps}\caption{}\label{fig3}\end{figure}\subsection{The Flight Schedule }According to the base analysis, we can know that the flightschedule matrix and $\Delta t$ is one form of flight timetable. In``The number of EDSs'', we can get suitable $\Delta t$. Then weshould resolve the flight schedule matrix.Because we have assumed that the checked baggage numbers of each flight setare equal to each other. It can be described as follows:\[\left\{\begin{array}{l}\rho_j\approx\bar{\rho}\\B_j^{set}\approx\bar{B}^{set}\end{array}\right.\begin{array}{*{20}c}\hfill&{j=1,2,\cdots,N_{set}}\hfill\end{array}\]The flight schedule matrix subject to this group:\[\left\{\begin{array}{ll}\sum\limits_{j=1}^{N_{set}}n_{ij}={NF}_i&i=1,2,\cdots\\\sum\limits_{i=1}^a n_{ij}\le N_{runway}&j=1,2,\cdots,N_{set}\\n_{ij}\ge0,&\mathrm{and}\:n_{ij}\:\mathrm{is}\:\mathrm{a}\:\mathrm{Integer} \end{array}\right.\]In order to make the best use of runway, we should make$\sum\limits_{i=1}^a n_{ij}$ as great as we can unless it exceed$N_{runway}$.Then we can see that how to resolve the flight schedule matrix is a problemof divide among a group of integers. This group is all the numbers of eachflight passengers' baggage in one flight set. We program for this problemusing MA TLAB and we get at least one solution in the end. However, thematrix elements we have obtained are not integer, we have to adjust them tobe integers manually.\subsection{Results and Interpretation for Airport A and B}The number of passengers in a certain flight (${NP}_i$), the timelength of security checking ($T_{set}$), the checking velocity ofEDS ($v_{EDS}$), and the number of baggage carried by onepassenger are random.\subsubsection{Data Assumption:}\begin{itemize}\item $T_{set}$ is 110 minutes, which is reasonable for airline.\item To simplify the problem, we assume that every passenger carry 2 baggage. If some of thepassengers carry one baggage, the solution based on 2 baggages per passenger meets therequirement.\item The number of runways in airport A and airport B is 5.\end{itemize}\subsubsection{Airport A:}Once the number of runway and the number of the flights aredecided, the flight schedule matrix is decided, too. We producethis matrix using MATLAB. This matrix companied by $\Delta t$ isthe flight schedule for airport A. $\Delta t$ will be calculatedin (\ref{Flight:baggage}), (\ref{sets:number}) and(\ref{PeakHour}).We calculate $N_{EDS}$ and make the flight timetable in threeconditions. The three conditions and the solution are listed asfollowed:\paragraph{Every flight are fully occupied}The checking speed of EDS is 160 bags/hour.\begin{table}[htbp]\centering\caption{}\begin{tabular}{*{11}c}\myhline{0.4mm}$\mathbf{\Deltat(\min)}$&\textbf{2}&\textbf{4}&\textbf{6}&\textbf{8}&\textbf{10}&\textbf{12}&\textbf{14} &\textbf{16}&\textbf{18}&\textbf{20}\\\myhline{0.4mm}$N_{EDS}(\ge)$&31&31&31&31&31&29&24&22&20&17\\\hline$H_{peak}(\min)$&20&40&60&80&100&120&140&160&180&200\\\myhline{0.4mm}\end{tabular}\label{tab2}\end{table}We assume that the suitable value of $H_{peak}$ is 120 minutes.Then the suitable value of $\Delta t$ is about 12 minutes, and$N_{EDS}$ is 29 judged from Figure \ref{fig4}. Certainly, we canwork $\Delta t$ and $N_{EDS}$ out through equation.\begin{figure}[htbp]\centering\includegraphics[width=294.6pt,totalheight=253.2pt]{fig04.eps}\caption{}\label{fig4}\end{figure}\paragraph{Every flight is occupied by the minimal number of passengers onstatistics in the long run.}The checking speed of EDS is 210 bags/hour.\begin{table}[htbp]\centering\caption{}\begin{tabular}{*{11}c}\myhline{0.4mm}$\mathbf{\Deltat(\min)}$&\textbf{2}&\textbf{4}&\textbf{6}&\textbf{8}&\textbf{10}&\textbf{12}&\textbf{14} &\textbf{16}&\textbf{18}&\textbf{20}\\\myhline{0.4mm}$N_{EDS}(\ge)$&15&15&15&15&15&14&13&12&10&7\\\hline$H_{peak}(\min)$&20&40&60&80&100&120&140&160&180&200\\\myhline{0.4mm}\end{tabular}\label{tab3}\end{table}We assume that the suitable value of $H_{peak}$ is 120 minutes.Then the suitable value of $\Delta t$ is about 12 minutes, and$N_{EDS}$ is 14 judging from Figure \ref{fig5}. Certainly, we canwork $\Delta t$ and $N_{EDS}$ out through equation.\begin{figure}[htbp]\centering\includegraphics[width=294.6pt,totalheight=253.2pt]{fig05.eps}\caption{}\label{fig5}\end{figure}\paragraph{${NP}_i$ and $v_{EDS}$ are random value produced by MATLAB.}\begin{table}[htbp]\centering\caption{}\begin{tabular}{*{11}c}\myhline{0.4mm}$\mathbf{\Deltat(\min)}$&\textbf{2}&\textbf{4}&\textbf{6}&\textbf{8}&\textbf{10}&\textbf{12}&\textbf{14} &\textbf{16}&\textbf{18}&\textbf{20}\\\myhline{0.4mm}$N_{EDS}(\ge)$&15&22&21&21&15&17&21&16&13&14\\\hline$H_{peak}(\min)$&20&40&60&80&100&120&140&160&180&200\\\myhline{0.4mm}\end{tabular}\label{tab4}\end{table}We assume that the suitable value of $H_{peak}$ is 120 minutes.Then the suitable value of $\Delta t$ is about 12 minutes, and$N_{EDS}$ is 17 judging from Figure \ref{fig6}. Certainly, we canwork $\Delta t$ and $N_{EDS}$ out through equation.\begin{figure}[htbp]\centering\includegraphics[width=294.6pt,totalheight=249.6pt]{fig06.eps}\caption{}\label{fig6}\end{figure}\subsubsection{Interpretation:}By analyzing the results above, we can conclude that when$N_{EDS}$ is 29, and $\Delta t$ is 12, the flight schedule willmeet requirement at any time. The flight schedule is:\\[\intextsep]\begin{minipage}{\textwidth}\centering\tabcaption{}\begin{tabular}{c|*{8}c|c|c}\myhline{0.4mm}\backslashbox{\textbf{Set}}{\textbf{Type}}&\textbf{1}&\textbf{2}&\textbf{3}&\textbf{4}&\te xtbf{5}&\textbf{6}&\textbf{7}&\textbf{8}&\textbf{Numbers of Bags}&\textbf{Numbers of Flights}\\\myhline{0.4mm}1&2&0&0&0&2&1&0&0&766&5\\\hline2&2&0&2&0&2&0&0&0&732&4\\\hline3&0&1&1&1&2&0&0&0&762&4\\\hline4&0&1&0&0&2&1&0&0&735&4\\\hline5&0&1&0&0&2&1&0&0&735&5\\\hline6&2&0&0&0&1&0&0&1&785&5\\\hline7&2&0&0&0&2&0&1&0&795&5\\\hline8&0&1&0&0&2&1&0&0&735&4\\\hline9&2&0&0&0&2&1&0&0&766&5\\\hline10&0&0&0&2&2&0&0&0&758&5\\\hlineTotal&10&4&3&3&19&5&1&1&7569&46\\\myhline{0.4mm}\end{tabular}\label{tab5}\end{minipage}\\[\intextsep]We have produced random value for ${NP}_i$ and $v_{EDS}$. On thiscondition, the number of EDSs is 17, which is less than 29 that wedecide for the airport A. That is to say our solution can meet thereal requirement.\subsubsection{Airport B:}\paragraph{The passenger load is 100{\%}}The checking speed of EDS is 160 bags/hour.\begin{table}[htbp]\centering。
美赛写作模板及参赛经验分享
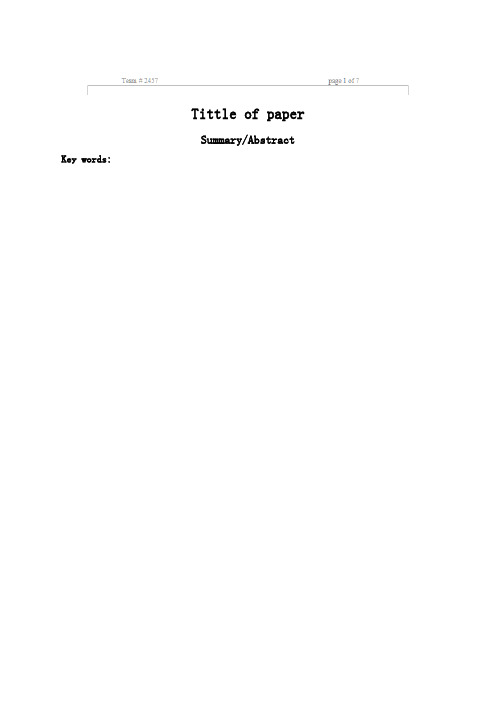
Tittle of paperSummary/Abstract Key words:I.Introduction(引言)Organ transplantation is a preferable treatment for the most serious forms of end-stage diseases. In recent years, advances in medical science and technology have made solid organ transplantation an increasingly successful and common medical procedure, a literal ''second chance at life". Not only does it offer the best hope for complete rehabilitation, but it has also proved to be the most cost-effective of all treatment options, including dialysis. Consequently, more and more people are benefiting from organ transplants and their survival rates are steadily improving. The surgical techniques involved have been mastered for half a century and are now considered as routine. The two main sources of kidneys for transplantation are deceased-donor kidneys and live-donations from family and friends. However, unfortunately, there is a considerable shortage of donor organs, compared to demands. As a matter of fact, efficient matching and allocation of organs donated has become an exigent problem.The United Network for Organ Sharing (UNOS), as the operator of the Organ Procurement and Transplantation Network (OPTN), is responsible for transplant organ distribution in the United States. UNOS oversees the allocation of many different types of transplants,including liver, kidney, pancreas, heart, lung, and cornea.Focusing on kidney transplantation, based on UNOS Kidney Allocation Model, we develop a mathematical model for US transplant networks. First, incoming organs are matched with waiting candidates by medical institutions considering the factors as ABO blood compatibility, the degree of recipient major HLA mismatch in order to obtain a matching degree applied on the allocation part. After that, from the patients’perspective, on the basis of linear regression, priority weight is defined by pondering age, disease severity, time on waiting, PRA level, and region. Applying this mechanism of ranking, we realize MWBM (Maximum Weight Bipartite-graph Matching) and SMGS (Stable Matching based on Gale-Shapley algorithm). MWBM focuses on the optimal assignment of donors following the algorithm of bipartite-graph maximum weight matching; SMGS emphasizes the process of exchanges in order to obtain the stable exchanges between donors and candidates on the waiting list.II.T he Description of Problem(问题重述)III.Basic Assumptions●The level of mismatch is only relative to the number of antigens.●The data and information are accurately registered according tothe medical measures●The data and information are refreshed in time according to thestatus of the patients●No differences in the quality of the donor kidneys●The quality of the donor kidney is constantIV.D efinitions and Notations●Kidney transplantation: A kidney transplant is a surgical procedure to implant a healthykidney into a patient with kidney failure.●Prioritization●MD: Matching Degree●PW: Prioritization weight●MWSM: Maximum Weight Bipartite Matching●SMGS: Stable Matching based on Gale-Shapley algorithm或V.ModelsThrough the investigation of US transplantation network, we draw a general picture of the mechanism. With reference to some resources available on the website of UNOS, a flow chart (Figure 1) is developed showing the procedure of the network.Currently, the initial waiting list is composed of patients whoare waiting for a kidney or combined kidney-pancreas transplant. For the first time, the patients are requested to show the correct and scientific information to the US kidney transplant network which is needed for donor-recipient matching, the ranking of patients on the waiting list, and determining the outcome of those transplanted. The patients’waiting lists are composed of initial patients, historical patients and unsuccessful recipient after transplantation. Historical patients refer to registered patients whose status have changed and have an influence on the procedure.A patient is taken off the waiting list when a graft is offered and accepted by that patient or the patient is dead while waiting for a transplant. Unsuccessful recipients refer to the patients who have a bad result of transplantation calling for transplantation again, as it is so-called relistFigure 1. A schematic depicting the steps occurring in thetransplantation networks......Table 1.Survival rate involving HLA mismatchVI.C onclusions.Our model for the optimal allocation of the donor organs is established by three modules, procurement of MD and PW, optimal assignment by MWBM model and Stable Matching of Gale-Shapley algorithm. The model has offered a convincing procedure of the allocation with the ……VII.Strengths and weaknesses(模型优缺点)Strengths……WeaknessesVIII.References注意文献的积累,不要等到文章写完再去重新寻找文献。
美赛论文模版(非常实用)

Title Abstract:Key words:Contents1. Introduction (3)1.1 Why does toll way collects toll? (3)1.2 Toll modes (3)1.3 Toll collection methods (3)1.4 Annoyance in toll plazas (3)1.5 The origin of the toll way problem (3)1.6 Queuing theory (4)2. The Description of Problem (5)2.1 How do we approximate the whole course of paying toll? (5)2.2 How do we define the optimal configuration? (5)2.2.1 From the perspective of motorist (5)2.2.2 From the perspective of the toll plaza (6)2.2.3 Compromise (6)2.3 Overall optimization and local optimization (6)2.4 The differences in weights and sizes of vehicles (7)2.5 What if there is no data available? (7)3. Models (7)3.1 Basic Model (7)3.1.1 Symbols and Definitions (7)3.1.2 Assumptions (8)3.1.3 The Foundation of Model (9)3.1.4 Solution and Result (11)3.1.5 Analysis of the Result (11)3.1.6 Strength and Weakness (13)3.2 Improved Model (14)3.2.1 Extra Symbols (14)3.2.2 Additional Assumptions (14)3.2.3 The Foundation of Model (14)3.2.4 Solution and Result (15)3.2.5 Analysis of the Result (18)3.2.6 Strength and Weakness (19)4. Conclusions (19)4.1 Conclusions of the problem (19)4.2 Methods used in our models (19)4.3 Application of our models (19)5. Future Work (19)5.1 Another model (19)5.2 Another layout of toll plaza (23)5.3 The newly- adopted charging methods (23)6.References (23)7.Appendix (23)Programs and codes (24)I. IntroductionIn order to indicate the origin of the toll way problems, the following background is worth mentioning.1.11.21.31.41.51.6II. The Description of the Problem2.1 How do we approximate the whole course of paying toll?●●●●2.2 How do we define the optimal configuration?1) From the perspective of motorist:2) From the perspective of the toll plaza:3) Compromise:2.3 The local optimization and the overall optimization●●●Virtually:2.4 The differences in weights and sizes of vehicles2.5 What if there is no data available?III. Models3.1 Basic Model3.1.1 Terms, Definitions and SymbolsThe signs and definitions are mostly generated from queuing theory.●●●●●3.1.2 Assumptions●●●●●3.1.3 The Foundation of Model1) The utility function●The cost of toll plaza:●The loss of motorist:●The weight of each aspect:●Compromise:2) The integer programmingAccording to queuing theory, we can calculate the statistical properties as follows.3)The overall optimization and the local optimization●The overall optimization:●The local optimization:●The optimal number of tollbooths:3.1.4 Solution and Result1) The solution of the integer programming:2) Results:3.1.5 Analysis of the Result●Local optimization and overall optimization:●Sensitivity: The result is quite sensitive to the change of the threeparameters●Trend:●Comparison:3.1.6 Strength and Weakness●Strength: In despite of this, the model has proved that . Moreover, wehave drawn some useful conclusions about . T he model is fit for, such as●Weakness: This model just applies to . As we have stated, .That’sjust what we should do in the improved model.3.2 Improved Model3.2.1 Extra SymbolsSigns and definitions indicated above are still valid. Here are some extra signs and definitions.●●●●3.2.2 Additional Assumptions●●●Assumptions concerning the anterior process are the same as the Basic Model.3.2.3 The Foundation of Model1) How do we determine the optimal number?As we have concluded from the Basic Model,3.2.4 Solution and Result1) Simulation algorithmBased on the analysis above, we design our simulation arithmetic as follows.●Step1:●Step2:●Step3:●Step4:●Step5:●Step6:●Step7:●Step8:●Step9:2) Flow chartThe figure below is the flow chart of the simulation.3) Solution3.2.5 Analysis of the Result3.2.6 Strength and Weakness●Strength: The Improved Model aims to make up for the neglect of .The result seems to declare that this model is more reasonable than the Basic Model and much more effective than the existing design.●Weakness: . Thus the model is still an approximate on a large scale. Thishas doomed to limit the applications of it.IV. Conclusions4.1 Conclusions of the problem●●●4.2 Methods used in our models●●●4.3 Applications of our models●●●V. Future Work5.1 Another model5.1.1The limitations of queuing theory 5.1.25.1.35.1.41)●●●●2)●●●3)●●●4)5.2 Another layout of toll plaza5.3 The newly- adopted charging methodsVI. References[1][2][3][4]VII. Appendix。
美赛论文LaTeX模板
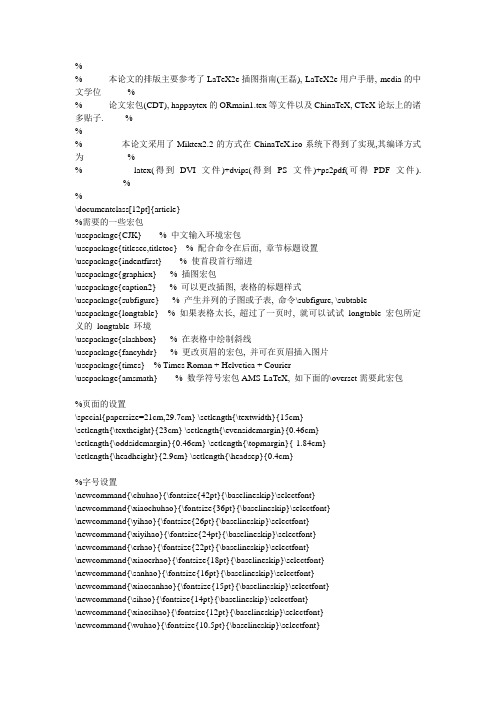
%% 本论文的排版主要参考了LaTeX2e插图指南(王磊), LaTeX2e用户手册, media的中文学位%% 论文宏包(CDT), happaytex的ORmain1.tex等文件以及ChinaTeX, CTeX论坛上的诸多贴子. %%% 本论文采用了Miktex2.2的方式在ChinaTeX.iso系统下得到了实现,其编译方式为%% latex(得到DVI文件)+dvips(得到PS文件)+ps2pdf(可得PDF文件).%%\documentclass[12pt]{article}%需要的一些宏包\usepackage{CJK} % 中文输入环境宏包\usepackage{titlesec,titletoc} % 配合命令在后面, 章节标题设置\usepackage{indentfirst} % 使首段首行缩进\usepackage{graphicx} % 插图宏包\usepackage{caption2} % 可以更改插图, 表格的标题样式\usepackage{subfigure} % 产生并列的子图或子表, 命令\subfigure, \subtable\usepackage{longtable} % 如果表格太长, 超过了一页时, 就可以试试longtable 宏包所定义的longtable 环境\usepackage{slashbox} % 在表格中绘制斜线\usepackage{fancyhdr} % 更改页眉的宏包, 并可在页眉插入图片\usepackage{times} % Times Roman + Helvetica + Courier\usepackage{amsmath} % 数学符号宏包AMS-LaTeX, 如下面的\overset需要此宏包%页面的设置\special{papersize=21cm,29.7cm} \setlength{\textwidth}{15cm}\setlength{\textheight}{23cm} \setlength{\evensidemargin}{0.46cm}\setlength{\oddsidemargin}{0.46cm} \setlength{\topmargin}{-1.84cm}\setlength{\headheight}{2.9cm} \setlength{\headsep}{0.4cm}%字号设置\newcommand{\chuhao}{\fontsize{42pt}{\baselineskip}\selectfont}\newcommand{\xiaochuhao}{\fontsize{36pt}{\baselineskip}\selectfont}\newcommand{\yihao}{\fontsize{26pt}{\baselineskip}\selectfont}\newcommand{\xiyihao}{\fontsize{24pt}{\baselineskip}\selectfont}\newcommand{\erhao}{\fontsize{22pt}{\baselineskip}\selectfont}\newcommand{\xiaoerhao}{\fontsize{18pt}{\baselineskip}\selectfont}\newcommand{\sanhao}{\fontsize{16pt}{\baselineskip}\selectfont}\newcommand{\xiaosanhao}{\fontsize{15pt}{\baselineskip}\selectfont}\newcommand{\sihao}{\fontsize{14pt}{\baselineskip}\selectfont}\newcommand{\xiaosihao}{\fontsize{12pt}{\baselineskip}\selectfont}\newcommand{\wuhao}{\fontsize{10.5pt}{\baselineskip}\selectfont}\newcommand{\xiaowuhao}{\fontsize{9pt}{\baselineskip}\selectfont}\newcommand{\liuhao}{\fontsize{7.5pt}{\baselineskip}\selectfont}\newcommand{\xiaoliuhao}{\fontsize{6.5pt}{\baselineskip}\selectfont}\newcommand{\qihao}{\fontsize{5.5pt}{\baselineskip}\selectfont}\newcommand{\bahao}{\fontsize{5pt}{\baselineskip}\selectfont}%页眉的设置, 要用到fancyhdr宏包\pagestyle{fancy} \fancyhead{} \fancyfoot{}\fancyhead[L]{\footnotesize Team \# 189}\fancyhead[R]{\footnotesize Page\ \thepage\ of\ 42}\fancypagestyle{plain}{%\fancyhead[L]{\footnotesize Team \# 189}\fancyhead[R]{\footnotesize Page\ \thepage\ of\ 42}}\setcounter{secnumdepth}{4}%更改\theparagraph的编号样式\makeatletter\renewcommand{\theparagraph}{\@arabic\c@paragraph}\makeatother%章节格式的设置\titleformat{\section}{\erhao\bf}{}{0em}{}[]\titleformat{\subsection}{\xiaoerhao\bf}{}{0em}{}[]\titleformat{\subsubsection}{\sanhao\bf}{}{0em}{}[]\titleformat{\paragraph}[hang]{\vspace*{0.5ex}\sihao\bf}{\hspace*{1em}\theparagraph)}{0.5em }{}[\vspace*{-0.5ex}]%更改插图的标题\renewcommand{\figurename}{\wuhao\bf\sf Figure}\renewcommand{\captionlabeldelim}{\ }%更改表格的标题\renewcommand{\tablename}{\wuhao\bf\sf Table}%更改图形或表格与其标题的间距\setlength{\abovecaptionskip}{10pt}\setlength{\belowcaptionskip}{10pt}%定义产生不浮动图形和表格的标题的命令\figcaption和\tabcaption\makeatletter\newcommand\figcaption{\def\@captype{figure}\caption}\newcommand\tabcaption{\def\@captype{table}\caption}\makeatother%自定义的可以调整粗细的水平线命令, 用于绘制表格, 调用格式\myhline{0.5mm}. \makeatletter\def\myhline#1{%\noalign{\ifnum0=`}\fi\hrule \@height #1 \futurelet\reserved@a\@xhline}\makeatother%第一层列表序号为带圈的阿拉伯数字\renewcommand{\labelenumi}{\textcircled{\arabic{enumi}}}%更改脚注设置\renewcommand{\thefootnote}{\fnsymbol{footnote}}\begin{document}\begin{CJK*}{GBK}{song}\CJKtilde\title{\bf\yihao Aviation Baggage Screening\\{\&} Flight Schedule}\author{}\date{}\maketitle\section{Introduction}Following the terrorist attacks on September 11, 2001, there isintense interest in improving the security screening process forairline passengers and their baggage. Airlines and airports areconsidered high-threat targets for terrorism, so aviation securityis crucial to the safety of the air-travelling public. Bombs andexplosives have been known to be introduced to aircraft by holdbaggage and cargo, carried on by passengers, and hidden withinaircraft supplies.At present To Screen or Not to Screen, that is a Hobson's choice.US Current laws mandate 100{\%} screening of all checked bags at the 429 passenger airports throughout the nation by explosive detection systems(EDS) by the end of the Dec 31 2003. However, because the manufacturers arenot able to produce the expected number of EDS required to meet the federal mandate, so it is significant to determine the correct number of devicesdeploy at each airport, and to take advantage of them effectively.The Transportation Security Administration (TSA) needs a complicatedanalysis on how to allocate limited device and how to best use them.Our paper contains the mathematical models to determine the number of EDSsand flight schedules for all airports in Midwest Region. We also discuss theETD devices as the additional security measures and the future developmentof the security systems.\section{Assumption and Hypothesis}\begin{itemize}\item The passengers who will get on the same airplane will arrive uniformly, namely the distribution is flat.\item The detection systems, both EDS and ETD, operate all the time during peak hour, except downtime.\item The airline checks the passengers randomly, according to its claim.\item The passengers, who are just landing and leave out, do not have to be checked through EDS or ETD.\item According to the literature, the aircraft loads approximately equal among the sets of departing flight during the peak hour.\item The landing flight did not affect the departure of the plane.\item Once a passenger arrives, he can go to EDS to be checked, except he has to wait in line.\item Once passengers finish screening, they can broad on the plane in no time.\item During peak hours, a set of flights departs at the same time every the same minutes.\item All the runways are used as much as possible during peak hours.\item The maximum number of the baggage is two, which a passenger can carry on plane. []\item The detection machine examines the bags at the same speed.\item EDS cannot make mistakes that it detect a normal object as an explosive.\end{itemize}\section{Variable and Definition}\begin{longtable}{p{100pt}p{280pt}}\caption{Variables}\\ %第一页表头的标题\endfirsthead %第一页的标题结束\caption{(continued)}\\ %第二页的标题\endhead %第二页的标题结束\hline\hline\textbf{Symbol}&\textbf{Description}\\\hline$n_{ij}$&The airplane number of the $i^{\mathrm{th}}$ type in the $j^{\mathrm{th}}$ flight set\\\hline${NP}_i\:(i=1,2,\ldots)$&The number of passengers on each airplanes of the same type.\\\hline$\xi_{ij}\:(i,j = 1,2,\cdots)$&The number of baggage on each airplane of the $j^{\mathrm{th}}$ flights\\\hline$a$&The maximal number of airplanes type\\\hline$B_j^{set}$&The total baggage number of each set of flight\\\hline${NF}_i$&Number of airplanes of each type\\\hline$\bar{\rho}$&The mean value of passengers' baggage coming per minute in every flight set\\ \hline$N_{set}$&The number of flight sets\\\hline$B_{total}$&The total number of checked baggage during the peak hour\\\hline$H_{peak}$&The length of the peak hour\\\hline$T_{set}$&The time length during which each flight set's passengers wait to be checked\\\hline$\Delta t$&The time interval between two consecutive flight set\\\hline$N_{EDS}$&The number of all the EDSs\\\hline$N_{shadow}$&The number of flight sets whose passengers will be mixed up before being checked\\\hline$v_{EDS}$&The number of baggage checking by one EDS per minute\\\hline$\rho_j$&The number of passengers' baggage coming per minute in one flight set\\\hline$N_{runway}$&The number of an airport's runway\\\hline\\*[-2.2ex]${\bar{B}}^{set}$&The mean value of checked baggage number of every flight set\\\hline$M$&The security cost\\\hline\hline\label{tab1}\end{longtable}\subsubsection{Definition:}\begin{description}\item[Flight set] A group of flights take off at the same time\item[The length of peak hour] The time between the first set of flight and the last set\end{description}\section{Basic Model}During a peak hour, many planes and many passengers would departfrom airports. Therefore, It is difficult to arrange for thepassengers to enter airports. If there are not enough EDSs forpassengers' baggage to check, it will take too long time for themto enter. That would result in the delay of airplanes. On thecontrary, if there are too many EDSs, it will be a waste. It isour task to find a suitable number of EDSs for airport. In orderto reach this objective, we use the linear programming method tosolve it.\subsection{Base analysis}The airplanes are occupied at least partly. The passengers'baggage would be checked by EDSs before they get on the airplanes.We have assumed that every passenger carry two baggages. Thisassumption would simplify the problem. According to the data fromthe problem sheet, we can obtain the useful information thatairlines claim 20{\%} of the passengers do not check any luggage,20{\%} check one bag, and the remaining passengers check two bags.Therefore, we can gain the total number of passengers' baggagethat should be carried on one plane: $\xi_{ij}$. Moreover, we canget the equation that calculate $\xi_{ij}$:\[\xi_{ij}={NP}_i\times 20\%+{NP}_i\times 60\%\times 2\]We define the matrix below as airplane baggage number matrix:\[\overset{\rightharpoonup}{\xi}_j=\left[\xi_{1j}\quad\xi_{2j}\quad\cdots\quad\xi_{ij}\quad\cdots\ right]\]We define the matrix below as flight schedule matrix:\[\left[\begin{array}{llcl}n_{11}&n_{12}&\cdots&n_{1N_{set}}\\n_{21}&n_{22}&\cdots&n_{2N_{set}}\\\multicolumn{4}{c}\dotfill\\n_{a1}&n_{a2}&\cdots&n_{aN_{set}}\end{array}\right]\]In this matrix, $n_{ij}$ is the airplane number of the$i^{\mathrm{th}}$ type in the $j^{\mathrm{th}}$ flight set whichwill take off. Apparently, this value is an integer.We define the matrix below as flight set baggage number matrix:\[\left[B_1^{set}\quad B_2^{set}\quad\cdots\quad B_j^{set}\quad\cdots\quad B_a^{set}\right] \]It is clear that they meet the relation below:\begin{equation}\begin{array}{cl}&\left[\xi_{1j}\quad\xi_{2j}\quad\cdots\quad\xi_{ij}\quad\cdots\right]\cdot\left[\begin{array}{llcl}n_{11}&n_{12}&\cdots&n_{1N_{set}}\\n_{21}&n_{22}&\cdots&n_{2N_{set}}\\\multicolumn{4}{c}\dotfill\\n_{a1}&n_{a2}&\cdots&n_{aN_{set}}\end{array}\right]\\=&\left[B_1^{set}\quad B_2^{set}\quad\cdots\quad B_j^{set}\quad\cdots\quad B_a^{set}\right]\end{array}\label{Flight:baggage}\end{equation}Then, we know:\[B_j^{set}=\sum\limits_{i=1}^a\xi_{ij}\times n_{ij}\]There are some constraints to the equation (\ref{Flight:baggage}).First, for each set of flight, the total number of airplanesshould be less than the number of runways. Second, the totalairplane number of the same type listed in the equation(\ref{Flight:baggage}) from every set of flight should be equal tothe actual airplane number of the same type during the peak hour.We can express them like these:\[\sum\limits_{i=1}^a n_{ij}\le N_{runway}\quad\quad\sum\limits_{j=1}^b n_{ij}={NF}_i \]We should resolve the number of flight sets. According to our assumptions,during the peak hour, the airlines should make the best use of the runways.Then get the number of flight sets approximately based on the number of allthe airplanes during the peak hour and that of the runways. We use anequation below to express this relation:\begin{equation}N_{set}=\left\lceil\frac{\sum\limits_{j=1}^{N_{set}}\sum\limits_{i=1}^an_{ij}}{N_{runway}}\right\rceil\label{sets:number}\end{equation}The checked baggage numbers of each flight set are equal to eachother according to our assumption. We make it based on literature.It can also simplify our model. We define $\bar{B}^{set}$ as themean value of checked baggage number of every flight set.Moreover, We define $\bar{\rho}$ as the mean value of checkedbaggage number of every flight set per minute:\[\bar{B}^{set}=\frac{B_{total}}{N_{set}}\]\[\bar{\rho}=\frac{\bar{B}^{set}}{T_{set}}=\frac{B_{total}}{T_{set}N_{set}}=\frac{B_{total}\ Delta t}{T_{set}H_{peak}}\]The course of passengers' arrival and entering airport isimportant for us to decide the number of EDSs and to make theflights schedule. Therefore, we should analyze this processcarefully. Passengers will arrive between forty-five minutes andtwo hours prior to the departure time, and the passengers who willget on the same airplane will arrive uniformly. Then we can getthe flow density of all checked baggage at any time duringpassengers' entering. This value is the sum of numbers ofpassengers' checked baggage coming per minute. To calculate thisvalue, firstly, we should obtain flow density of each flight set'schecked baggage. We define $\rho_j $, namely the number of checkedbaggage per minute of one flight set:\[\rho_j=\frac{B_j^{set}}{T_{set}}\]Secondly, we draw graphic to help us to understand. We userectangle to express the time length for all the passengers of oneflight set to come and check bags. In the graphic, the black partis the period for them to come. During the white part, nopassengers for this flight set come. According to the problemsheet, the former is 75 minute, and the latter is 45 minute. Thelength of rectangle is 120 minute. $T_{set}$ is the period duringwhich all passengers of one flight set wait to be checked. Sincewe have assumed that each time interval between two consecutiveflight set is same value, we define $\Delta t$ as it. Observe thesection that value we want to solve is $\sum\limits_j\rho_j$. Moreover, we can get another important equation from the graphic below:\begin{equation}N_{set}=\frac{H_{peak}}{\Delta t}\label{PeakHour}\end{equation}\begin{figure}[hbtp]\centering\includegraphics[width=298.2pt,totalheight=141.6pt]{fig01.eps}\caption{}\label{fig1}\end{figure}Each EDS has certain capacity. If the number of EDSs is $N_{EDS}$ and one EDS can check certain number of baggage per minute (Thatis checking velocity, marked by $v_{EDS}$), the total checking capacity is $N_{EDS}\cdot\frac{v_{EDS}}{60}$. $v_{EDS}$ is between 160 and 210.Now we can easily decide in what condition the passengers can be checked without delay:\[\sum\limits_j\rho_j\le v_{EDS}\]The passengers have to queue before being checked:$\sum\limits_j\rho_j>v_{EDS}$Well then, how can we decide how many $\rho_j$? It depends on how many flight sets whose passengers will be mixed up before being checked. We note it as $N_{shadow} $. Return to the Figure\ref{fig1}, we can know:\[N_{shadow}=\left\lfloor\frac{T_{set}}{\Delta t}\right\rfloor\]\begin{figure}%[htbp]\centering\includegraphics[width=240pt,totalheight=131.4pt]{fig02.eps}\caption{}\label{fig2}\end{figure}From Figure \ref{fig1} and Figure \ref{fig2}, we can get theresult as follows:\begin{enumerate}\item If $N_{shadow}\le N_{set}$, namely $H_{peak}>T_{set}$, then $\sum\limits_{j=1}^{N_{shadow}}\rho _j\le N_{EDS}\frac{v_{EDS}}{60}$\renewcommand{\theequation}{\arabic{equation}a}That is:\begin{equation}N_{EDS}\ge\frac{60}{v_{EDS}}\sum\limits_{j=1}^{N_{shadow}}\rho_j\approx\frac{60}{v_{ EDS}}N_{shadow}\bar{\rho}=\frac{60B_{total}\Deltat}{v_{EDS}T_{set}H_{peak}}N_{shadow}\label{EDS:number:a}\end{equation}\item If $N_{shadow}>N_{set}$, namely $H_{peak}\le T_{set}$, then $\sum\limits_{j=1}^{N_{set}}\rho_j\le N_{EDS}\frac{v_{EDS}}{60}$\setcounter{equation}{3}\renewcommand{\theequation}{\arabic{equation}b}That is:\begin{equation}N_{EDS}\ge\frac{60}{v_{EDS}}\sum\limits_{j=1}^{N_{set}}\rho_j\approx\frac{60}{v_{EDS} }N_{set}\bar{\rho}=\frac{60B_{total}\Delta t}{v_{EDS}T_{set}H_{peak}}N_{set}\label{EDS:number:b}\end{equation}\end{enumerate}\subsection{The number of EDSs}Then we begin to resolve the number of EDSs assisted by the linearprogramming method.EDS is operational about 92{\%} of the time. That is to say, whenever it isduring a peak hour, there are some EDSs stopping working. Then the workingefficiency of all the EDSs is less than the level we have expected.Therefore, the airline has to add more EDSs to do the work, which can bedone with less EDSs without downtime.We use binomial distribution to solve this problem. $N$ is the number ofactual EDSs with downtime and $k$ is the number of EDSs without downtime. Ifprobability is $P$, we can get the equation below:\[\left(\begin{array}{c}N\\k\end{array}\right)\cdot98\%^k\cdot(1-98\%)^{N-k}=P\]We can obtain $N$ when we give $P$ a certain value. In this paper,$P$ is 95{\%}. The $N_{EDS}$ is the actual number we obtainthrough the equation above.Now we have assumed that passengers can be checked unless be delayed by the people before him once he arrives at airport. Apparently, if the time length between two sets of flight is short, the density of passengers will begreat. It will bring great stress to security check and may even make some passengers miss their flight. To resolve this question, the airline has toinstall more EDSs to meet the demand. However, this measure will cost much more money. Consequently, we have to set a suitable time interval between two set of flight.Based on the base analysis above. We can use the equation(\ref{sets:number}) to decide the number of flight sets $N_{set}$assuming we know the number of runways of a certain airport. Thenbased on the equation (\ref{PeakHour}), we can decide the peakhour length $H_{peak}$ when we assume a time interval between two consecutive flight sets. Then we use \textcircled{1} and\textcircled{2} to decide which to choose between equation(\ref{EDS:number:a}) and equation (\ref{EDS:number:b}). In consequence, we can obtain the minimum of EDSs number.If we choose different numbers of runways and the time intervalsbetween two flight sets, we can get different EDSs numbers. Inthis paper that followed, we gain a table of some value of$N_{runway}$ and $\Delta t$ with the corresponding EDSs numbers. Moreover, we draw some figure to reflect their relation.For a certain airport, its number of runway is known. Givencertain time interval ($\Delta t$), we can get the length of thepeak hour ($H_{peak}$). When the $N_{runway}$ is few enough,perhaps $H_{peak}$ is too long to be adopted. However, for acertain airline, they can decide the time interval of their ownpeak hour. In this given time interval, they could find theminimum of $N_{runway}$ through the Figure \ref{fig3}. We draw asketch map to describe our steps.\begin{figure}[hbtp]\centering\includegraphics[width=352.8pt,totalheight=214.2pt]{fig03.eps}\caption{}\label{fig3}\end{figure}\subsection{The Flight Schedule }According to the base analysis, we can know that the flightschedule matrix and $\Delta t$ is one form of flight timetable. In``The number of EDSs'', we can get suitable $\Delta t$. Then weshould resolve the flight schedule matrix.Because we have assumed that the checked baggage numbers of each flight setare equal to each other. It can be described as follows:\[\left\{\begin{array}{l}\rho_j\approx\bar{\rho}\\B_j^{set}\approx\bar{B}^{set}\end{array}\right.\begin{array}{*{20}c}\hfill&{j=1,2,\cdots,N_{set}}\hfill\end{array}\]The flight schedule matrix subject to this group:\[\left\{\begin{array}{ll}\sum\limits_{j=1}^{N_{set}}n_{ij}={NF}_i&i=1,2,\cdots\\\sum\limits_{i=1}^a n_{ij}\le N_{runway}&j=1,2,\cdots,N_{set}\\n_{ij}\ge0,&\mathrm{and}\:n_{ij}\:\mathrm{is}\:\mathrm{a}\:\mathrm{Integer} \end{array}\right.\]In order to make the best use of runway, we should make$\sum\limits_{i=1}^a n_{ij}$ as great as we can unless it exceed$N_{runway}$.Then we can see that how to resolve the flight schedule matrix is a problemof divide among a group of integers. This group is all the numbers of eachflight passengers' baggage in one flight set. We program for this problemusing MA TLAB and we get at least one solution in the end. However, thematrix elements we have obtained are not integer, we have to adjust them tobe integers manually.\subsection{Results and Interpretation for Airport A and B}The number of passengers in a certain flight (${NP}_i$), the timelength of security checking ($T_{set}$), the checking velocity ofEDS ($v_{EDS}$), and the number of baggage carried by onepassenger are random.\subsubsection{Data Assumption:}\begin{itemize}\item $T_{set}$ is 110 minutes, which is reasonable for airline.\item To simplify the problem, we assume that every passenger carry 2 baggage. If some of thepassengers carry one baggage, the solution based on 2 baggages per passenger meets therequirement.\item The number of runways in airport A and airport B is 5.\end{itemize}\subsubsection{Airport A:}Once the number of runway and the number of the flights aredecided, the flight schedule matrix is decided, too. We producethis matrix using MATLAB. This matrix companied by $\Delta t$ isthe flight schedule for airport A. $\Delta t$ will be calculatedin (\ref{Flight:baggage}), (\ref{sets:number}) and(\ref{PeakHour}).We calculate $N_{EDS}$ and make the flight timetable in threeconditions. The three conditions and the solution are listed asfollowed:\paragraph{Every flight are fully occupied}The checking speed of EDS is 160 bags/hour.\begin{table}[htbp]\centering\caption{}\begin{tabular}{*{11}c}\myhline{0.4mm}$\mathbf{\Deltat(\min)}$&\textbf{2}&\textbf{4}&\textbf{6}&\textbf{8}&\textbf{10}&\textbf{12}&\textbf{14} &\textbf{16}&\textbf{18}&\textbf{20}\\\myhline{0.4mm}$N_{EDS}(\ge)$&31&31&31&31&31&29&24&22&20&17\\\hline$H_{peak}(\min)$&20&40&60&80&100&120&140&160&180&200\\\myhline{0.4mm}\end{tabular}\label{tab2}\end{table}We assume that the suitable value of $H_{peak}$ is 120 minutes.Then the suitable value of $\Delta t$ is about 12 minutes, and$N_{EDS}$ is 29 judged from Figure \ref{fig4}. Certainly, we canwork $\Delta t$ and $N_{EDS}$ out through equation.\begin{figure}[htbp]\centering\includegraphics[width=294.6pt,totalheight=253.2pt]{fig04.eps}\caption{}\label{fig4}\end{figure}\paragraph{Every flight is occupied by the minimal number of passengers onstatistics in the long run.}The checking speed of EDS is 210 bags/hour.\begin{table}[htbp]\centering\caption{}\begin{tabular}{*{11}c}\myhline{0.4mm}$\mathbf{\Deltat(\min)}$&\textbf{2}&\textbf{4}&\textbf{6}&\textbf{8}&\textbf{10}&\textbf{12}&\textbf{14} &\textbf{16}&\textbf{18}&\textbf{20}\\\myhline{0.4mm}$N_{EDS}(\ge)$&15&15&15&15&15&14&13&12&10&7\\\hline$H_{peak}(\min)$&20&40&60&80&100&120&140&160&180&200\\\myhline{0.4mm}\end{tabular}\label{tab3}\end{table}We assume that the suitable value of $H_{peak}$ is 120 minutes.Then the suitable value of $\Delta t$ is about 12 minutes, and$N_{EDS}$ is 14 judging from Figure \ref{fig5}. Certainly, we canwork $\Delta t$ and $N_{EDS}$ out through equation.\begin{figure}[htbp]\centering\includegraphics[width=294.6pt,totalheight=253.2pt]{fig05.eps}\caption{}\label{fig5}\end{figure}\paragraph{${NP}_i$ and $v_{EDS}$ are random value produced by MATLAB.}\begin{table}[htbp]\centering\caption{}\begin{tabular}{*{11}c}\myhline{0.4mm}$\mathbf{\Deltat(\min)}$&\textbf{2}&\textbf{4}&\textbf{6}&\textbf{8}&\textbf{10}&\textbf{12}&\textbf{14} &\textbf{16}&\textbf{18}&\textbf{20}\\\myhline{0.4mm}$N_{EDS}(\ge)$&15&22&21&21&15&17&21&16&13&14\\\hline$H_{peak}(\min)$&20&40&60&80&100&120&140&160&180&200\\\myhline{0.4mm}\end{tabular}\label{tab4}\end{table}We assume that the suitable value of $H_{peak}$ is 120 minutes.Then the suitable value of $\Delta t$ is about 12 minutes, and$N_{EDS}$ is 17 judging from Figure \ref{fig6}. Certainly, we canwork $\Delta t$ and $N_{EDS}$ out through equation.\begin{figure}[htbp]\centering\includegraphics[width=294.6pt,totalheight=249.6pt]{fig06.eps}\caption{}\label{fig6}\end{figure}\subsubsection{Interpretation:}By analyzing the results above, we can conclude that when$N_{EDS}$ is 29, and $\Delta t$ is 12, the flight schedule willmeet requirement at any time. The flight schedule is:\\[\intextsep]\begin{minipage}{\textwidth}\centering\tabcaption{}\begin{tabular}{c|*{8}c|c|c}\myhline{0.4mm}\backslashbox{\textbf{Set}}{\textbf{Type}}&\textbf{1}&\textbf{2}&\textbf{3}&\textbf{4}&\te xtbf{5}&\textbf{6}&\textbf{7}&\textbf{8}&\textbf{Numbers of Bags}&\textbf{Numbers of Flights}\\\myhline{0.4mm}1&2&0&0&0&2&1&0&0&766&5\\\hline2&2&0&2&0&2&0&0&0&732&4\\\hline3&0&1&1&1&2&0&0&0&762&4\\\hline4&0&1&0&0&2&1&0&0&735&4\\\hline5&0&1&0&0&2&1&0&0&735&5\\\hline6&2&0&0&0&1&0&0&1&785&5\\\hline7&2&0&0&0&2&0&1&0&795&5\\\hline8&0&1&0&0&2&1&0&0&735&4\\\hline9&2&0&0&0&2&1&0&0&766&5\\\hline10&0&0&0&2&2&0&0&0&758&5\\\hlineTotal&10&4&3&3&19&5&1&1&7569&46\\\myhline{0.4mm}\end{tabular}\label{tab5}\end{minipage}\\[\intextsep]We have produced random value for ${NP}_i$ and $v_{EDS}$. On thiscondition, the number of EDSs is 17, which is less than 29 that wedecide for the airport A. That is to say our solution can meet thereal requirement.\subsubsection{Airport B:}\paragraph{The passenger load is 100{\%}}The checking speed of EDS is 160 bags/hour.\begin{table}[htbp]\centering。
美赛数模论文格式
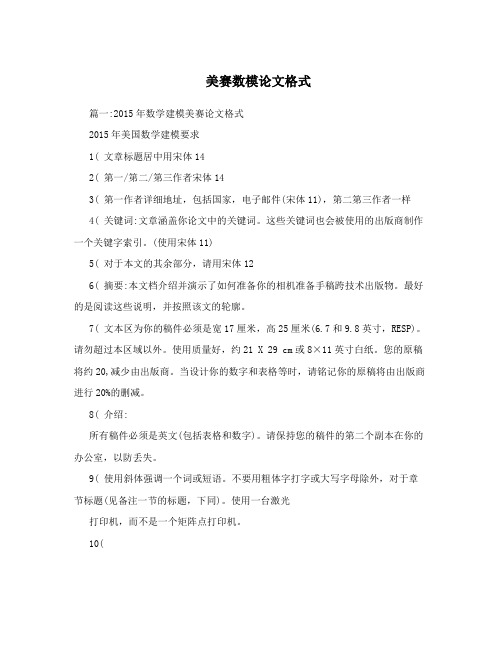
美赛数模论文格式篇一:2015年数学建模美赛论文格式2015年美国数学建模要求1( 文章标题居中用宋体142( 第一/第二/第三作者宋体143( 第一作者详细地址,包括国家,电子邮件(宋体11),第二第三作者一样4( 关键词:文章涵盖你论文中的关键词。
这些关键词也会被使用的出版商制作一个关键字索引。
(使用宋体11)5( 对于本文的其余部分,请用宋体126( 摘要:本文档介绍并演示了如何准备你的相机准备手稿跨技术出版物。
最好的是阅读这些说明,并按照该文的轮廓。
7( 文本区为你的稿件必须是宽17厘米,高25厘米(6.7和9.8英寸,RESP)。
请勿超过本区域以外。
使用质量好,约21 X 29 cm或8×11英寸白纸。
您的原稿将约20,减少由出版商。
当设计你的数字和表格等时,请铭记你的原稿将由出版商进行20%的删减。
8( 介绍:所有稿件必须是英文(包括表格和数字)。
请保持您的稿件的第二个副本在你的办公室,以防丢失。
9( 使用斜体强调一个词或短语。
不要用粗体字打字或大写字母除外,对于章节标题(见备注一节的标题,下同)。
使用一台激光打印机,而不是一个矩阵点打印机。
10(文本组织:标题:标题用粗体,首字母大写,其他字母小写。
二级标题作为接下来段落的一部分页码:不要打印页码:请在底部(打字区外)附近的左上角用淡蓝色的铅笔轻轻编号在每一张纸上。
, 脚注:脚注应该是单倍行距和文字分开。
理想地,脚注出现的其参考的页面上,并且被放置在文本的下方,由一个水平线从文本分离。
表格:(参考:表格1,表格2…)应该作为文本的一部分,(用1,2注明)这样做可以避免文档的混淆。
描述性的标题应该置于每个表格之上。
标题应该是自包含的,在表格的下面或者旁边放着。
表格的单位应该用方括号【】注视。
如果方括号无法使用,则用大括号{}或普通括号()图形:(参考图形1,图形2…)也作为文本的一部分,要留下足够的空间让图形的说明不会和文本混淆。
- 1、下载文档前请自行甄别文档内容的完整性,平台不提供额外的编辑、内容补充、找答案等附加服务。
- 2、"仅部分预览"的文档,不可在线预览部分如存在完整性等问题,可反馈申请退款(可完整预览的文档不适用该条件!)。
- 3、如文档侵犯您的权益,请联系客服反馈,我们会尽快为您处理(人工客服工作时间:9:00-18:30)。
A The L TEXTemplate for MCM Version 5.0
AT {L EX Studio }
November 3, 2014
2
Team # 0000
Page 3 of ??
1
Introduction
Although a hitter might expect a model of the bat-baseball collision to yield insight into how the bat breaks, how the bat imparts spin on the ball, how best to swing the bat, and so on, we model only the sweet spot. ˘ Tan ˇ impact loThere are at least two notions of where the sweet spot should beâA cation on the bat that either • minimizes the discomfort to the hands, or • maximizes the outgoing velocity of the ball. We focus exclusively on the second definition. • the initial velocity and rotation of the ball, • the initial velocity and rotation of the bat, • the relative position and orientation of the bat and ball, and • the force over time that the hitter hands applies on the handle. We assume that the ball is not rotating and that its velocity at impact is perpendicular to the length of the bat. We assume that everything occurs in a single plane, and we will argue that the hands?interaction is negligible. In the frame of reference of the center of mass of the bat, the initial conditions are completely specified by • the angular velocity of the bat, • the velocity of the ball, and • the position of impact along the bat. The location of the sweet spot depends not on just the bat alone but also on the pitch and on the swing. The simplest model for the physics involved has the sweet spot at the center of percussion [Brody 1986], the impact location that minimizes discomfort to the hand. The model assumes the ball to be a rigid body for which there are conjugate points: An impact at one will exactly balance the angular recoil and linear recoil at the other. By gripping at one and impacting at the other (the center of percussion), the hands experience minimal shock and the ball exits with high velocity. The center of percussion depends heavily on the moment of inertia and the location of the hands. We cannot accept this model because it both erroneously equates the two definitions of sweet spot and furthermore assumes incorrectly that the bat is a rigid body. Another model predicts the sweet spot to be between nodes of the two lowest natural frequencies of the bat [Nathan 2000]. Given a free bat allowed to oscillate, its oscillations can be decomposed into fundamental modes of various frequencies. Different geometries and materials have different natural frequencies of oscillation. The resulting wave shapes suggest how to excite those modes (e.g., plucking a string at the node of a vibrational mode will not excite that mode).
pj =
Team # 0000
Page 5 of ??
Peaks
5
0
−5
2 0 −2 y −3 −2 −1 x 0 1 2
Team # 0000
Page 4 of ??
A Theorem 1.1. Proof. The proof of theorem.
1.1
Other Assumptions
Although Mr. Gore has expressed concerns to some associates about the damage a brokered convention could cause, several associates said he was hopeful that one candidate would soon break through, sparing the party such an outcome. He told a close friend recently that his decision not to endorse “feels like the right thing” and that he remained optimistic the race “is going to tip at some point,” the friend said. • • • • Under the above and basic assumptions, we can set out to construct our model (show our approach in detail).
Abstract
We determine the sweet spot on a baseball bat. We capture the essential physics of the ball-bat impact by taking the ball to be a lossy spring and the bat to be an Euler-Bernoulli beam. To impart some intuition about the model, we begin by presenting a rigid-body model. Next, we use our full model to reconcile various correct and incorrect claims about the sweet spot found in the literature. Finally, we discuss the sweet spot and the performances of corked and aluminum bats, with a particular emphasis on hoop modes.
2
Analysis of the Problem
Although Mr. Gore has expressed concerns to some associates about the damage a brokered convention could cause, several associates said he was hopeful that one candidate would soon break through, sparing the party such an outcome. He told a close friend recently that his decision not to endorse “feels like the right thing” and that he remained optimistic the race “is going to tip at some point,” the friend said.(??) a2 a11 a12 a13 a21 a22 a23 = Opposite cos−1 θ arcsin θ Hypotenuse a31 a32 a33 Although Mr. Gore has expressed concerns to some associates about the damage a brokered convention could cause, several associates said he was hopeful that one candidate would soon break through, sparing the party such an outcome. He told a close friend recently that his decision not to endorse “feels like the right thing” and that he remained optimistic the race “is going to tip at some point,” the friend said. 0, if j is odd j/2 r! (−1) , if j is even (1)