lecture8 Matrix Completion
《IEEEsignalprocessingletters》期刊第19页50条数据
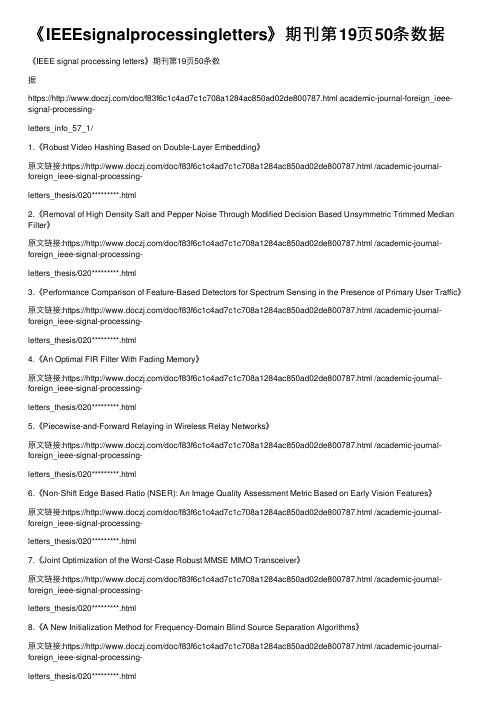
《IEEEsignalprocessingletters》期刊第19页50条数据《IEEE signal processing letters》期刊第19页50条数据https:///doc/f83f6c1c4ad7c1c708a1284ac850ad02de800787.html academic-journal-foreign_ieee-signal-processing-letters_info_57_1/1.《Robust Video Hashing Based on Double-Layer Embedding》原⽂链接:https:///doc/f83f6c1c4ad7c1c708a1284ac850ad02de800787.html /academic-journal-foreign_ieee-signal-processing-letters_thesis/020*********.html2.《Removal of High Density Salt and Pepper Noise Through Modified Decision Based Unsymmetric Trimmed Median Filter》原⽂链接:https:///doc/f83f6c1c4ad7c1c708a1284ac850ad02de800787.html /academic-journal-foreign_ieee-signal-processing-letters_thesis/020*********.html3.《Performance Comparison of Feature-Based Detectors for Spectrum Sensing in the Presence of Primary User Traffic》原⽂链接:https:///doc/f83f6c1c4ad7c1c708a1284ac850ad02de800787.html /academic-journal-foreign_ieee-signal-processing-letters_thesis/020*********.html4.《An Optimal FIR Filter With Fading Memory》原⽂链接:https:///doc/f83f6c1c4ad7c1c708a1284ac850ad02de800787.html /academic-journal-foreign_ieee-signal-processing-letters_thesis/020*********.html5.《Piecewise-and-Forward Relaying in Wireless Relay Networks》原⽂链接:https:///doc/f83f6c1c4ad7c1c708a1284ac850ad02de800787.html /academic-journal-foreign_ieee-signal-processing-letters_thesis/020*********.html6.《Non-Shift Edge Based Ratio (NSER): An Image Quality Assessment Metric Based on Early Vision Features》原⽂链接:https:///doc/f83f6c1c4ad7c1c708a1284ac850ad02de800787.html /academic-journal-foreign_ieee-signal-processing-letters_thesis/020*********.html7.《Joint Optimization of the Worst-Case Robust MMSE MIMO Transceiver》原⽂链接:https:///doc/f83f6c1c4ad7c1c708a1284ac850ad02de800787.html /academic-journal-foreign_ieee-signal-processing-letters_thesis/020*********.html8.《A New Initialization Method for Frequency-Domain Blind Source Separation Algorithms》原⽂链接:https:///doc/f83f6c1c4ad7c1c708a1284ac850ad02de800787.html /academic-journal-foreign_ieee-signal-processing-letters_thesis/020*********.html9.《A Method For Fine Resolution Frequency Estimation From Three DFT Samples》原⽂链接:https:///doc/f83f6c1c4ad7c1c708a1284ac850ad02de800787.html /academic-journal-foreign_ieee-signal-processing-letters_thesis/020*********.html10.《Position-Patch Based Face Hallucination Using Convex Optimization》原⽂链接:https:///doc/f83f6c1c4ad7c1c708a1284ac850ad02de800787.html /academic-journal-foreign_ieee-signal-processing-letters_thesis/020*********.html11.《Signal Fitting With Uncertain Basis Functions》原⽂链接:https:///doc/f83f6c1c4ad7c1c708a1284ac850ad02de800787.html /academic-journal-foreign_ieee-signal-processing-letters_thesis/020*********.html12.《Optimal Filtering Over Uncertain Wireless Communication Channels》原⽂链接:https:///doc/f83f6c1c4ad7c1c708a1284ac850ad02de800787.html /academic-journal-foreign_ieee-signal-processing-letters_thesis/020*********.html13.《The Student's -Hidden Markov Model With Truncated Stick-Breaking Priors》原⽂链接:https:///doc/f83f6c1c4ad7c1c708a1284ac850ad02de800787.html /academic-journal-foreign_ieee-signal-processing-letters_thesis/020*********.html14.《IEEE Signal Processing Society Information》原⽂链接:https:///doc/f83f6c1c4ad7c1c708a1284ac850ad02de800787.html /academic-journal-foreign_ieee-signal-processing-letters_thesis/020*********.html15.《Acoustic Model Adaptation Based on Tensor Analysis of Training Models》原⽂链接:https:///doc/f83f6c1c4ad7c1c708a1284ac850ad02de800787.html /academic-journal-foreign_ieee-signal-processing-letters_thesis/020*********.html16.《On Estimating the Number of Co-Channel Interferers in MIMO Cellular Systems》原⽂链接:https:///doc/f83f6c1c4ad7c1c708a1284ac850ad02de800787.html /academic-journal-foreign_ieee-signal-processing-letters_thesis/020*********.html17.《Period Estimation in Astronomical Time Series Using Slotted Correntropy》原⽂链接:https:///doc/f83f6c1c4ad7c1c708a1284ac850ad02de800787.html /academic-journal-foreign_ieee-signal-processing-letters_thesis/020*********.html18.《Multidimensional Shrinkage-Thresholding Operator and Group LASSO Penalties》原⽂链接:https:///doc/f83f6c1c4ad7c1c708a1284ac850ad02de800787.html /academic-journal-foreign_ieee-signal-processing-letters_thesis/020*********.html19.《Enhanced Seam Carving via Integration of Energy Gradient Functionals》letters_thesis/020*********.html20.《Backtracking-Based Matching Pursuit Method for Sparse Signal Reconstruction》原⽂链接:https:///doc/f83f6c1c4ad7c1c708a1284ac850ad02de800787.html /academic-journal-foreign_ieee-signal-processing-letters_thesis/020*********.html21.《Performance Bounds of Network Coding Aided Cooperative Multiuser Systems》原⽂链接:https:///doc/f83f6c1c4ad7c1c708a1284ac850ad02de800787.html /academic-journal-foreign_ieee-signal-processing-letters_thesis/020*********.html22.《Table of Contents》原⽂链接:https:///doc/f83f6c1c4ad7c1c708a1284ac850ad02de800787.html /academic-journal-foreign_ieee-signal-processing-letters_thesis/020*********.html23.《Bayesian Estimation With Imprecise Likelihoods: Random Set Approach》原⽂链接:https:///doc/f83f6c1c4ad7c1c708a1284ac850ad02de800787.html /academic-journal-foreign_ieee-signal-processing-letters_thesis/020*********.html24.《Low-Complexity Channel-Estimate Based Adaptive Linear Equalizer》原⽂链接:https:///doc/f83f6c1c4ad7c1c708a1284ac850ad02de800787.html /academic-journal-foreign_ieee-signal-processing-letters_thesis/020*********.html25.《Tensor Versus Matrix Completion: A Comparison With Application to Spectral Data》原⽂链接:https:///doc/f83f6c1c4ad7c1c708a1284ac850ad02de800787.html /academic-journal-foreign_ieee-signal-processing-letters_thesis/020*********.html26.《Joint DOD and DOA Estimation for MIMO Array With Velocity Receive Sensors》原⽂链接:https:///doc/f83f6c1c4ad7c1c708a1284ac850ad02de800787.html /academic-journal-foreign_ieee-signal-processing-letters_thesis/020*********.html27.《Regularized Subspace Gaussian Mixture Models for Speech Recognition》原⽂链接:https:///doc/f83f6c1c4ad7c1c708a1284ac850ad02de800787.html /academic-journal-foreign_ieee-signal-processing-letters_thesis/020*********.html28.《Handoff Optimization Using Hidden Markov Model》原⽂链接:https:///doc/f83f6c1c4ad7c1c708a1284ac850ad02de800787.html /academic-journal-foreign_ieee-signal-processing-letters_thesis/020*********.html29.《Standard Deviation for Obtaining the Optimal Direction in the Removal of Impulse Noise》letters_thesis/020*********.html30.《Energy Detection Limits Under Log-Normal Approximated Noise Uncertainty》原⽂链接:https:///doc/f83f6c1c4ad7c1c708a1284ac850ad02de800787.html /academic-journal-foreign_ieee-signal-processing-letters_thesis/020*********.html31.《Joint Subspace Learning for View-Invariant Gait Recognition》原⽂链接:https:///doc/f83f6c1c4ad7c1c708a1284ac850ad02de800787.html /academic-journal-foreign_ieee-signal-processing-letters_thesis/020*********.html32.《GMM-Based KLT-Domain Switched-Split Vector Quantization for LSF Coding》原⽂链接:https:///doc/f83f6c1c4ad7c1c708a1284ac850ad02de800787.html /academic-journal-foreign_ieee-signal-processing-letters_thesis/020*********.html33.《Complexity Reduced Face Detection Using Probability-Based Face Mask Prefiltering and Pixel-Based Hierarchical-Feature Adaboosting》原⽂链接:https:///doc/f83f6c1c4ad7c1c708a1284ac850ad02de800787.html /academic-journal-foreign_ieee-signal-processing-letters_thesis/020*********.html34.《RLS Algorithm With Convex Regularization》原⽂链接:https:///doc/f83f6c1c4ad7c1c708a1284ac850ad02de800787.html /academic-journal-foreign_ieee-signal-processing-letters_thesis/020*********.html35.《Solvability of the Zero-Pinning Technique to Orthonormal Wavelet Design》原⽂链接:https:///doc/f83f6c1c4ad7c1c708a1284ac850ad02de800787.html /academic-journal-foreign_ieee-signal-processing-letters_thesis/020*********.html36.《Power Spectrum Blind Sampling》原⽂链接:https:///doc/f83f6c1c4ad7c1c708a1284ac850ad02de800787.html /academic-journal-foreign_ieee-signal-processing-letters_thesis/020*********.html37.《Noise Folding in Compressed Sensing》原⽂链接:https:///doc/f83f6c1c4ad7c1c708a1284ac850ad02de800787.html /academic-journal-foreign_ieee-signal-processing-letters_thesis/020*********.html38.《Fast Maximum Likelihood Scale Parameter Estimation From Histogram Measurements》原⽂链接:https:///doc/f83f6c1c4ad7c1c708a1284ac850ad02de800787.html /academic-journal-foreign_ieee-signal-processing-letters_thesis/020*********.html39.《Elastic-Transform Based Multiclass Gaussianization》原⽂链接:https:///doc/f83f6c1c4ad7c1c708a1284ac850ad02de800787.html /academic-journal-foreign_ieee-signal-processing-letters_thesis/020*********.html40.《Improving Detection of Acoustic Signals by Means of a Time and Frequency Multiple Energy Detector》原⽂链接:https:///doc/f83f6c1c4ad7c1c708a1284ac850ad02de800787.html /academic-journal-foreign_ieee-signal-processing-letters_thesis/020*********.html41.《Efficient Multiple Kernel Support Vector Machine Based Voice Activity Detection》原⽂链接:https:///doc/f83f6c1c4ad7c1c708a1284ac850ad02de800787.html /academic-journal-foreign_ieee-signal-processing-letters_thesis/020*********.html42.《Performance Analysis of Dual-Hop AF Systems With Interference in Nakagami-$m$ Fading Channels》原⽂链接:https:///doc/f83f6c1c4ad7c1c708a1284ac850ad02de800787.html /academic-journal-foreign_ieee-signal-processing-letters_thesis/020*********.html43.《Illumination Normalization Based on Weber's Law With Application to Face Recognition》原⽂链接:https:///doc/f83f6c1c4ad7c1c708a1284ac850ad02de800787.html /academic-journal-foreign_ieee-signal-processing-letters_thesis/020*********.html44.《A Robust Replay Detection Algorithm for Soccer Video》原⽂链接:https:///doc/f83f6c1c4ad7c1c708a1284ac850ad02de800787.html /academic-journal-foreign_ieee-signal-processing-letters_thesis/020*********.html45.《Regularized Adaptive Algorithms-Based CIR Predictors for Time-Varying Channels in OFDM Systems》原⽂链接:https:///doc/f83f6c1c4ad7c1c708a1284ac850ad02de800787.html /academic-journal-foreign_ieee-signal-processing-letters_thesis/020*********.html46.《A Novel Semi-Blind Selected Mapping Technique for PAPR Reduction in OFDM》原⽂链接:https:///doc/f83f6c1c4ad7c1c708a1284ac850ad02de800787.html /academic-journal-foreign_ieee-signal-processing-letters_thesis/020*********.html47.《Widely Linear Simulation of Continuous-Time Complex-Valued Random Signals》原⽂链接:https:///doc/f83f6c1c4ad7c1c708a1284ac850ad02de800787.html /academic-journal-foreign_ieee-signal-processing-letters_thesis/020*********.html48.《A Generalized Poisson Summation Formula and its Application to Fast Linear Convolution》原⽂链接:https:///doc/f83f6c1c4ad7c1c708a1284ac850ad02de800787.html /academic-journal-foreign_ieee-signal-processing-letters_thesis/020*********.html49.《Multiple-Symbol Differential Sphere Detection Aided Differential Space-Time Block Codes Using QAM Constellations》原⽂链接:https:///doc/f83f6c1c4ad7c1c708a1284ac850ad02de800787.html /academic-journal-foreign_ieee-signal-processing-letters_thesis/020*********.html50.《Low Rank Language Models for Small Training Sets》原⽂链接:https:///doc/f83f6c1c4ad7c1c708a1284ac850ad02de800787.html /academic-journal-foreign_ieee-signal-processing-letters_thesis/020*********.html。
四元数矩阵补全代码

四元数矩阵补全代码四元数矩阵补全是一种在矩阵中填补缺失值的技术。
这种技术可以通过使用四元数来处理矩阵中的缺失值,从而提高矩阵的鲁棒性和精度。
以下是一个四元数矩阵补全的代码实现:```pythonimport numpy as npfrom scipy.optimize import minimize# 定义四元数类class Quaternion:def __init__(self, a, b, c, d):self.a = aself.b = bself.c = cself.d = d# 定义四元数加法def __add__(self, q2):return Quaternion(self.a + q2.a, self.b + q2.b, self.c + q2.c, self.d + q2.d)# 定义四元数减法def __sub__(self, q2):return Quaternion(self.a - q2.a, self.b - q2.b, self.c -q2.c, self.d - q2.d)# 定义四元数数乘def __mul__(self, q2):a = self.a * q2.a - self.b * q2.b - self.c * q2.c - self.d * q2.db = self.a * q2.b + self.b * q2.a + self.c * q2.d - self.d * q2.cc = self.a * q2.c - self.b * q2.d + self.c * q2.a + self.d * q2.bd = self.a * q2.d + self.b * q2.c - self.c * q2.b + self.d * q2.areturn Quaternion(a, b, c, d)# 定义四元数共轭def conj(self):return Quaternion(self.a, -self.b, -self.c, -self.d)# 定义四元数求模def norm(self):return np.sqrt(self.a ** 2 + self.b ** 2 + self.c ** 2 + self.d ** 2)# 定义四元数单位化def normalize(self):norm = self.norm()if norm == 0:return Quaternion(0, 0, 0, 0)else:return Quaternion(self.a / norm, self.b / norm, self.c / norm, self.d / norm)# 定义四元数逆def inv(self):return self.conj() * (1 / self.norm() ** 2)# 定义四元数矩阵补全类class QuaternionMatrixCompletion:def __init__(self, X, p, q, r):self.X = Xself.p = pself.q = qself.r = r# 定义四元数矩阵补全目标函数def objective(self, theta):A = Quaternion(theta[:self.p],theta[self.p:self.p+self.q],theta[self.p+self.q:self.p+2*self.q], theta[-self.r:])Z = np.zeros((self.p, self.q), dtype=Quaternion)for i in range(self.p):for j in range(self.q):if not np.isnan(self.X[i][j]):Z[i][j] = Quaternion(self.X[i][j], 0, 0, 0)E = np.linalg.norm(A.dot(Z).flatten() - self.X.flatten(), ord=2)return E# 定义四元数矩阵补全方法def complete(self):theta = np.random.rand(self.p + 2 * self.q + self.r) # 初始化参数向量bounds = [(None, None)] * self.p + [(0, None)] * self.q + [(None, None)] * self.q + [(None, None)] * self.r # 参数上下界res = minimize(self.objective, theta, bounds=bounds) # 最小化目标函数A = Quaternion(res.x[:self.p],res.x[self.p:self.p+self.q],res.x[self.p+self.q:self.p+2*self.q], res.x[-self.r:])X_hat = A.dot(Z) # 恢复矩阵return X_hat```这个代码实现了一个四元数矩阵补全的类,其中包括了四元数的基本运算,以及矩阵补全的目标函数和方法。
机器学习中矩阵低秩与稀疏近似
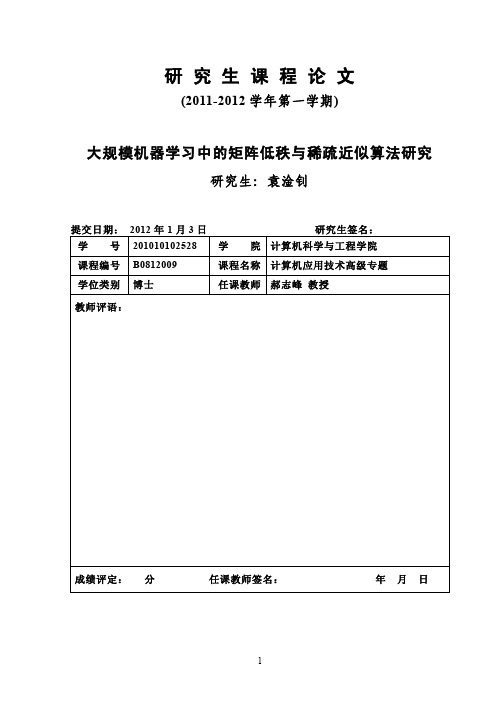
3、课程论文用 A4 纸双面打印。字体全部用宋体简体,题目 要求用小二号字加粗,标题行要求用小四号字加粗,正文内容要求 用小四号字;经学院同意,课程论文可以用英文撰写,字体全部用 Times New Roman,题目要求用 18 号字加粗;标题行要求用 14 号字加粗,正文内容要求用 12 号字;行距为 2 倍行距(方便教师 批注);页边距左为 3cm、右为 2cm、上为 2.5cm、下为 2.5cm;其 它格式请参照学位论文要求。
1.2 l0正则
l0正则是最直接最根本的稀疏学习技术。然而不幸的是,它具有组合的性质,是 个非凸正则子,难于分析。最小化l0范数是一个NP难的问题,在理论和实践中,均只 存在指数复杂度(相对于向量维数)的算法。一般来说,绝大多数算法对求l0只能得 到一个非精确解,有的直接求解最接近l0正则的凸l1正则(显然在lp正则中,p越少越
3
华南理工大学工学博士研究生课程论文
a) p ≥ 1
b) 0 < p < 1
图 1 当p ≥ 1与0 < p < 1时,lp正则子的形状示意图。
接近l0正则),也有的研究者使用如下函数逼近来逼近l0: x 0 ≈ i log(ε + |xi|),其 中ε是一个很小的正数,它是为了避免出现log 0数值上的无意义。但对于需要直接优
2
华南理工大学工学博士研究生课程论文
统计学习是当今机器学习领域的主流技术。向量空间的统计学习算法已经比较 成熟,近几年来,许多研究者主要把目光放在矩阵空间上。与向量空间相比,基于矩 阵空间的学习技术由于缺少扩展性,会随着问题的大小在空间和时间复杂度上分别 呈二次方与三次方增长,所以如何逼近一个目标矩阵而令机器学习技术更鲁棒更精 确更适合于大规模的情况已成为当今机器学习领域十分热门的话题。受到支持向量 机、压缩感知和非负矩阵分解等技术的启发,基于稀疏和低秩性质的假设,人们开发 了一系列基于矩阵方法的机器学习算法。
矩阵集中不等式MATRIX CONCENTRATION INEQUALITIES
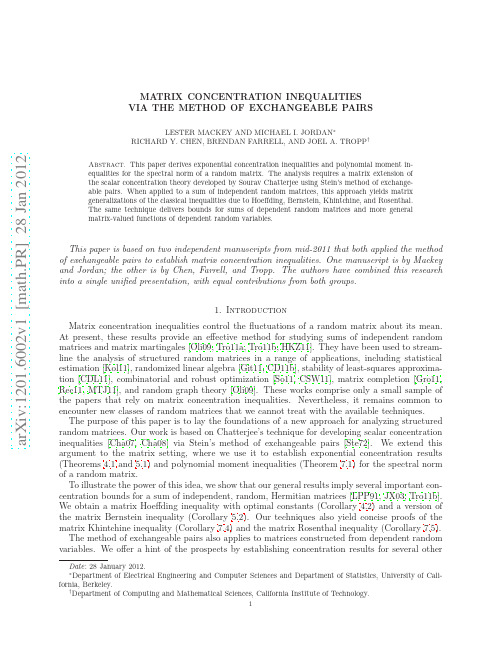
LESTER MACKEY AND MICHAEL I. JORDAN∗ RICHARD Y. CHEN, BRENDAN FARRELL, AND JOEL A. TROPP†
This paper is based on two independent manuscripts from mid-2011 that both applied the method of exchangeable pairs to establish matrix concentration inequalities. One manuscript is by Mackey and Jordan; the other is by Chen, Farrell, and Tropp. The authors have combined this research into a single unified presentation, with equal contributions from both groups. 1. Introduction Matrix concentration inequalities control the fluctuations of a random matrix about its mean. At present, these results provide an effective method for studying sums of independent random matrices and matrix martingales [Oli09, Tro11a, Tro11b, HKZ11]. They have been used to streamline the analysis of structured random matrices in a range of applications, including statistical estimation [Kol11], randomized linear algebra [Git11, CD11b], stability of least-squares approximation [CDL11], combinatorial and robust optimization [So11, CSW11], matrix completion [Gro11, Rec11, MTJ11], and random graph theory [Oli09]. These works comprise only a small sample of the papers that rely on matrix concentration inequalities. Nevertheless, it remains common to encounter new classes of random matrices that we cannot treat with the available techniques. The purpose of this paper is to lay the foundations of a new approach for analyzing structured random matrices. Our work is based on Chatterjee’s technique for developing scalar concentration inequalities [Cha07, Cha08] via Stein’s method of exchangeable pairs [Ste72]. We extend this argument to the matrix setting, where we use it to establish exponential concentration results (Theorems 4.1 and 5.1) and polynomial moment inequalities (Theorem 7.1) for the spectral norm of a random matrix. To illustrate the power of this idea, we show that our general results imply several important concentration bounds for a sum of independent, random, Hermitian matrices [LPP91, JX03, Tro11b]. We obtain a matrix Hoeffding inequality with optimal constants (Corollary 4.2) and a version of the matrix Bernstein inequality (Corollary 5.2). Our techniques also yield concise proofs of the matrix Khintchine inequality (Corollary 7.4) and the matrix Rosenthal inequality (Corollary 7.5). The method of exchangeable pairs also applies to matrices constructed from dependent random variables. We offer a hint of the prospects by establishing concentration results for several other
平面四边形八节点等参元matlab程序
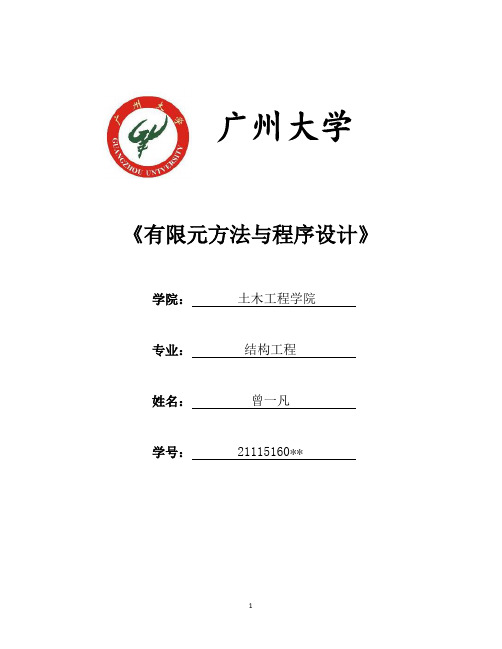
广州大学《有限元方法与程序设计》学院:土木工程学院专业:结构工程姓名:***学号: **********% 平面四边形八节点等参元MATLAB程序% 变量说明&(2015级——结构工程——曾一凡)% YOUNG POISS THICK% 弹性模量泊松比厚度% NPOIN NELEM NVFIX NFORCE% 总结点数单元数约束结点个数受力结点数% COORD LNODS FORCE% 结构节点整体坐标数组单元定义数组结点力数组% ALLFORCE FIXED HK DISP% 总体荷载向量约束信息数组总体刚度矩阵结点位移向量% 1本程序计算了节点位移和单元中心应力并输出到nonde8out.txt文本里% 2在第四页举了一个实例用MATLAB算出结果再用ANSYS算出的结果对比%======================主程序=====================format short e %设定输出类型clear %清除内存变量FP1=fopen('nonde8.txt','rt'); %打开初始数据文件FP2=fopen('nonde8out.txt','wt'); %打开文件存放计算结果NPOIN=fscanf(FP1,'%d',1); %结点数NELEM=fscanf(FP1,'%d',1); %单元数NFORCE=fscanf(FP1,'%d',1); %作用荷载的结点个数NVFIX=fscanf(FP1,'%d',1); %约束数YOUNG=fscanf(FP1,'%e',1); %弹性模量POISS=fscanf(FP1,'%f',1); %泊松比THICK=fscanf(FP1,'%f',1);%厚度LNODS=fscanf(FP1,'%d',[8,NELEM])';%单元结点号数组(逆时针)COORD=fscanf(FP1,'%f',[2,NPOIN])'; % 结点号x,y坐标(整体坐标下) FORCE=fscanf(FP1,'%f',[3,NFORCE])';%结点力数组% 节点力:结点号、X方向力(向右正),Y方向力(向上正)FIXED=fscanf(FP1,'%d',NVFIX)';% 约束信息:约束对应的位移编码(共计NVFIX 组)EK=zeros(2*8,2*8); % 单元刚度矩阵并清零HK=zeros(2*NPOIN,2*NPOIN); % 张成总刚矩阵并清零X=zeros(1,8); %存放单元8个x方向坐标的向量并清零Y=zeros(1,8); %存放单元8个y方向坐标的向量并清零%--------------------------求总刚-------------------------------for i=1:NELEM % 对单元个数循环for m=1:8% 对单元结点个数循环X(m)=COORD(LNODS(i,m),1); %单元8个x方向坐标的向量Y(m)=COORD(LNODS(i,m),2); %单元8个y方向坐标的向量endEK=eKe(X,Y,YOUNG,POISS,THICK);%调用单元刚度矩阵a=LNODS(i,:); %临时向量,用来记录当前单元的结点编号for j=1:8 %对行进行循环---按结点号循环for k=1:8 %对列进行循环---按结点号循环HK((a(j)*2-1):a(j)*2,(a(k)*2-1):a(k)*2)=HK((a(j)*2-1):a(j)*2,(a(k)*2-1):a(k)*2)+...EK(j*2-1:j*2,k*2-1:k*2); % 单刚子块叠加到总刚中endendendALLFORCE=FOECEXL(NPOIN,NFORCE,FORCE); % 调用函数生成荷载向量%-------------------------处理约束--------------------------------for j=1:NVFIX % 对约束个数进行循环N1=FIXED(j);HK(1:2*NPOIN,N1)=0; HK(N1,1:2*NPOIN)=0; HK(N1,N1)=1;% 将零位移约束对应的行、列变成零,主元变成1ALLFORCE(N1)=0;endDISP=HK\ALLFORCE; % 方程求解,HK先求逆再与力向量左乘得到位移%-------------------------求应力---------------------------------stress=zeros(3,NELEM); % 应力向量并清零for i=1:NELEM % 对单元个数进行循环D=(YOUNG/(1-POISS*POISS))*[1 POISS 0;POISS 1 0;0 0 (1-POISS)/2]; %弹性矩阵for k=1:8 % 对单元结点个数进行循环N2=LNODS(i,k); % 取单元结点号U(k*2-1:k*2)=DISP(N2*2-1:N2*2); %从总位移向量中取出当前单元的结点位移B=eBe(X,Y,0,0); %调用单元中心的应变矩阵endstress(:,i)=D*B*U';end%===============计算单元刚度矩阵函数===================function EK=eKe(X,Y,YOUNG,POISS,THICK)EK=zeros(16,16); % 张成16*16矩阵并清零D=(YOUNG/(1-POISS*POISS))*[1 POISS 0;POISS 1 0;0 0 (1-POISS)/2]; %弹性矩阵%高斯积分采用3*3个积分点A1=5/9;A2=8/9;A3=5/9; %对应积分的加权系数A=[A1 A2 A3];r=(3/5)^(1/2);x=[-r 0 r]; %积分点for i=1:3for j=1:3B=eBe(X,Y,x(i),x(j)); %调用应变矩阵BJ=Jacobi(X,Y,x(i),x(j)); %调用雅可比矩阵JEK=EK+A(i)*A(j)*B'*D*B*det(J)*THICK;endend%===============计算雅可比矩阵函数===================function J=Jacobi(X,Y,s,t)[N_s,N_t]=DHS(s,t);x_s=0;y_s=0;x_t=0;y_t=0;for j=1:8x_s=x_s+N_s(j)*X(j);y_s=y_s+N_s(j)*Y(j);x_t=x_t+N_t(j)*X(j);y_t=y_t+N_t(j)*Y(j);endJ=[x_s y_s;x_t y_t];%===============计算应变矩阵函数===================function B=eBe(X,Y,s,t)[N_s,N_t]=DHS(s,t);J=Jacobi(X,Y,s,t);B=zeros(3,16);for i=1:8B1=J(2,2)*N_s(i)-J(1,2)*N_t(i);B2=-J(2,1)*N_s(i)+J(1,1)*N_t(i);B(1:3,2*i-1:2*i)=[B1 0;0 B2;B2 B1];endB=B/det(J);%===============计算形函数的求导函数================ ==function [N_s,N_t]=DHS(s,t)N_s(1)=1/4*(1-t)*(s-t-1)+1/4*(1+s)*(1-t);N_s(2)=1/2*(1+t)*(1-t);N_s(3)=1/4*(1+t)*(s+t-1)+1/4*(1+s)*(1+t);N_s(4)=1/2*(1-s)*(1+t)-1/2*(1+s)*(1+t);N_s(5)=-1/4*(1+t)*(-s+t-1)-1/4*(1-s)*(1+t);N_s(6)=-1/2*(1+t)*(1-t);N_s(7)=-1/4*(1-t)*(-s-t-1)-1/4*(1-s)*(1-t);N_s(8)=1/2*(1-s)*(1-t)-1/2*(1+s)*(1-t);N_t(1)=-1/4*(1+s)*(s-t-1)-1/4*(1+s)*(1-t);N_t(2)=1/2*(1+s)*(1-t)-1/2*(1+s)*(1+t);N_t(3)=1/4*(1+s)*(s+t-1)+1/4*(1+s)*(1+t);N_t(4)=1/2*(1+s)*(1-s);N_t(5)=1/4*(1-s)*(-s+t-1)+1/4*(1-s)*(1+t);N_t(6)=1/2*(1-s)*(1-t)-1/2*(1-s)*(1+t);N_t(7)=-1/4*(1-s)*(-s-t-1)-1/4*(1-s)*(1-t);N_t(8)=-1/2*(1+s)*(1-s);end%===============计算总荷载矩阵函数===================function ALLFORCE=FOECEXL(NPOIN,NFORCE,FORCE) % 本函数生成荷载向量ALLFORCE=zeros(2*NPOIN,1); % 张成特定大小的向量,并赋值0for i=1:NFORCEALLFORCE((FORCE(i,1)*2-1):FORCE(i,1)*2)=FORCE(i,2:3);%FORCE(i,1)为作用点,FORCE(i,2:3)为x,y方向的结点力end%-------------------------输出节点位移和单元中心应力-------------------------------for i=1:NPOINfprintf(FP2,'x%d=%d\n',i, DISP(2*i-1)); %输出结点x方向的位移fprintf(FP2,'y%d=%d\n',i, DISP(2*i)); %输出结点y方向的位移endfor j=1:NELEMfprintf(FP2,'%d x=%f\n',j,stress(1,j)); %输出单元x方向的应力fprintf(FP2,'%d y=%f\n',j,stress(2,j)); %输出单元y方向的应力fprintf(FP2,'%d xy=%f\n',j,stress(3,j)); %输出单元切应力end%------------------------实例计算并用ANSYS进行对比结果----------------------------如图所示一个4m*1m悬臂梁,在3节点作用1*105N的竖向力,参数如下:弹性模量2.0*108,泊松比0.3,划分成四个单元,每个单元八个节点,单元尺寸是1m*1m。
Matrix Completion from a Few Entries
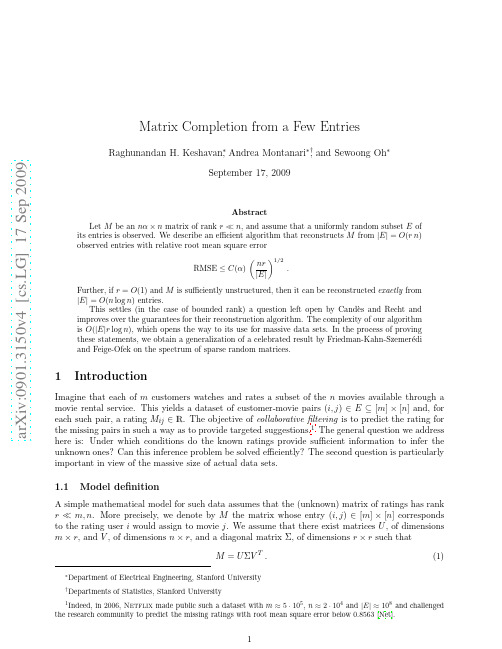
1
Introduction
Imagine that each of m customers watches and rates a subset of the n movies available through a movie rental service. This yields a dataset of customer-movie pairs (i, j ) ∈ E ⊆ [m] × [n] and, for each such pair, a rating Mij ∈ R. The objective of collaborative filtering is to predict the rating for the missing pairs in such a way as to provide targeted suggestions.1 The general question we address here is: Under which conditions do the known ratings provide sufficient information to infer the unknown ones? Can this inference problem be solved efficiently? The second question is particularly important in view of the massive size of actual data sets.
It turns out that, if |E | = Θ(n), this algorithm performs very poorly. The reason is that the matrix M E contains columns and rows with Θ(log n/ log log n) non-zero (revealed) entries. The largest singular values of M E are of order Θ( log n/ log log n). The corresponding singular vectors are highly concentrated on high-weight column or row indices (respectively, for left and right singular vectors). Such singular vectors are an artifact of the high-weight columns/rows and do not provide useful information about the hidden entries of M . This motivates the definition of the following operation (hereafter the degree of a column or of a row is the number of its revealed entries). Trimming. Set to zero all columns in M E with degree larger that 2|E |/n. Set to 0 all rows with degree larger than 2|E |/m. Figure 1 shows the singular value distributions of M E and M E for a random rank-3 matrix M . The surprise is that trimming (which amounts to ‘throwing out information’) makes the underlying rank-3 structure much more apparent. This effect becomes even more important when the number of revealed entries per row/column follows a heavy tail distribution, as for real data. In terms of the above routines, our algorithm has the following structure. Spectral Matrix Completion( matrix M E ) 1: Trim M E , and let M E be the output; 2: Project M E to Tr (M E ); 3: Clean residual errors by minimizing the discrepancy F (X, Y ). 2
colmap八点法计算本质矩阵
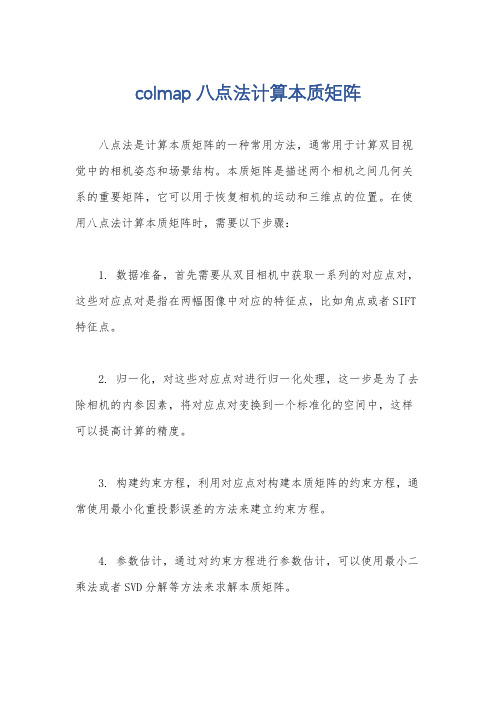
colmap八点法计算本质矩阵
八点法是计算本质矩阵的一种常用方法,通常用于计算双目视觉中的相机姿态和场景结构。
本质矩阵是描述两个相机之间几何关系的重要矩阵,它可以用于恢复相机的运动和三维点的位置。
在使用八点法计算本质矩阵时,需要以下步骤:
1. 数据准备,首先需要从双目相机中获取一系列的对应点对,这些对应点对是指在两幅图像中对应的特征点,比如角点或者SIFT 特征点。
2. 归一化,对这些对应点对进行归一化处理,这一步是为了去除相机的内参因素,将对应点对变换到一个标准化的空间中,这样可以提高计算的精度。
3. 构建约束方程,利用对应点对构建本质矩阵的约束方程,通常使用最小化重投影误差的方法来建立约束方程。
4. 参数估计,通过对约束方程进行参数估计,可以使用最小二乘法或者SVD分解等方法来求解本质矩阵。
5. 约束处理,由于计算得到的矩阵可能不满足本质矩阵的性质,需要对其进行约束处理,比如通过SVD分解将本质矩阵约束为满足
特定条件的形式。
6. 解算结果验证,最后,需要对计算得到的本质矩阵进行验证,通常可以通过对极约束、三角化等方法来验证本质矩阵的准确性。
总的来说,八点法计算本质矩阵是一个复杂的过程,需要对双
目图像进行深入的理解和处理,同时需要熟练掌握相关的数学知识
和计算机视觉算法。
同时,还需要注意处理数据的精度和噪声等因素,以获得准确的本质矩阵计算结果。
求解低秩矩阵填充的改进的交替最速下降法
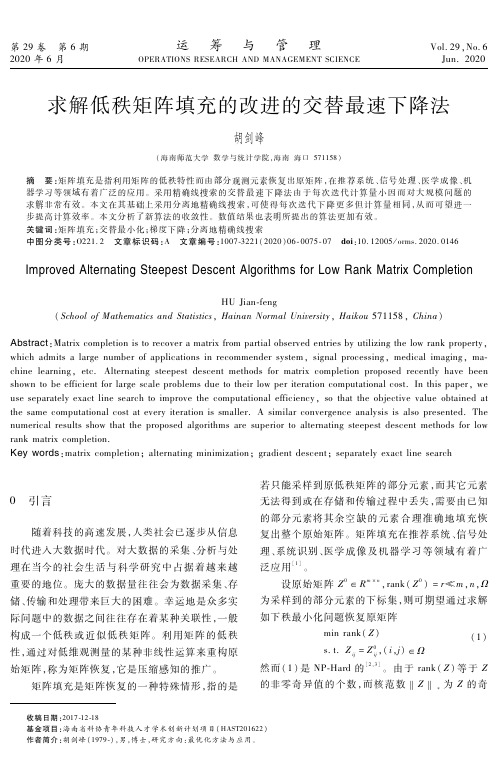
第29卷 第6期运 筹 与 管 理Vol.29,No.62020年6月OPERATIONSRESEARCHANDMANAGEMENTSCIENCEJun.2020收稿日期:2017 12 18基金项目:海南省科协青年科技人才学术创新计划项目(HAST201622)作者简介:胡剑峰(1979 ),男,博士,研究方向:最优化方法与应用。
求解低秩矩阵填充的改进的交替最速下降法胡剑峰(海南师范大学数学与统计学院,海南海口571158)摘 要:矩阵填充是指利用矩阵的低秩特性而由部分观测元素恢复出原矩阵,在推荐系统、信号处理、医学成像、机器学习等领域有着广泛的应用。
采用精确线搜索的交替最速下降法由于每次迭代计算量小因而对大规模问题的求解非常有效。
本文在其基础上采用分离地精确线搜索,可使得每次迭代下降更多但计算量相同,从而可望进一步提高计算效率。
本文分析了新算法的收敛性。
数值结果也表明所提出的算法更加有效。
关键词:矩阵填充;交替最小化;梯度下降;分离地精确线搜索中图分类号:O221.2 文章标识码:A 文章编号:1007 3221(2020)06 0075 07 doi:10.12005/orms.2020.0146ImprovedAlternatingSteepestDescentAlgorithmsforLowRankMatrixCompletionHUJian feng(SchoolofMathematicsandStatistics,HainanNormalUniversity,Haikou571158,China)Abstract:Matrixcompletionistorecoveramatrixfrompartialobservedentriesbyutilizingthelowrankproperty,whichadmitsalargenumberofapplicationsinrecommendersystem,signalprocessing,medicalimaging,machinelearning,etc.Alternatingsteepestdescentmethodsformatrixcompletionproposedrecentlyhavebeenshowntobeefficientforlargescaleproblemsduetotheirlowperiterationcomputationalcost.Inthispaper,weuseseparatelyexactlinesearchtoimprovethecomputationalefficiency,sothattheobjectivevalueobtainedatthesamecomputationalcostateveryiterationissmaller.Asimilarconvergenceanalysisisalsopresented.Thenumericalresultsshowthattheproposedalgorithmsaresuperiortoalternatingsteepestdescentmethodsforlowrankmatrixcompletion.Keywords:matrixcompletion;alternatingminimization;gradientdescent;separatelyexactlinesearch0 引言随着科技的高速发展,人类社会已逐步从信息时代进入大数据时代。
一种多维度矩阵的cmc神经网络模型
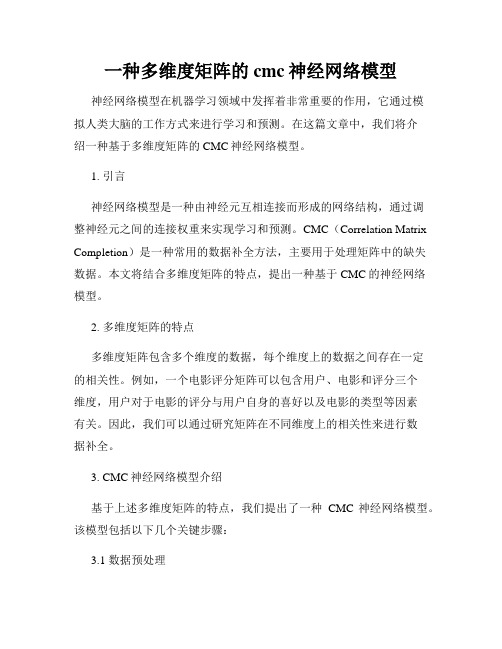
一种多维度矩阵的cmc神经网络模型神经网络模型在机器学习领域中发挥着非常重要的作用,它通过模拟人类大脑的工作方式来进行学习和预测。
在这篇文章中,我们将介绍一种基于多维度矩阵的CMC神经网络模型。
1. 引言神经网络模型是一种由神经元互相连接而形成的网络结构,通过调整神经元之间的连接权重来实现学习和预测。
CMC(Correlation Matrix Completion)是一种常用的数据补全方法,主要用于处理矩阵中的缺失数据。
本文将结合多维度矩阵的特点,提出一种基于CMC的神经网络模型。
2. 多维度矩阵的特点多维度矩阵包含多个维度的数据,每个维度上的数据之间存在一定的相关性。
例如,一个电影评分矩阵可以包含用户、电影和评分三个维度,用户对于电影的评分与用户自身的喜好以及电影的类型等因素有关。
因此,我们可以通过研究矩阵在不同维度上的相关性来进行数据补全。
3. CMC神经网络模型介绍基于上述多维度矩阵的特点,我们提出了一种CMC神经网络模型。
该模型包括以下几个关键步骤:3.1 数据预处理首先,我们对输入的多维度矩阵进行预处理。
对于缺失的数据,我们可以使用相关维度上的均值或者通过其他方法进行补全。
3.2 神经网络结构接下来,我们构建一个多层神经网络用于学习矩阵中的相关性。
该神经网络包括输入层、隐藏层和输出层。
输入层接收预处理后的矩阵数据,隐藏层通过调整连接权重来学习数据之间的相关性,输出层用于进行预测。
3.3 反向传播算法为了准确预测缺失的数据,我们使用反向传播算法来训练神经网络。
该算法通过计算预测值与真实值之间的差异来更新连接权重,直到达到预定的训练误差。
3.4 数据补全在经过训练后,我们可以使用训练好的神经网络来进行数据补全。
对于缺失的数据,我们将其作为输入送入神经网络,通过神经网络的预测输出来进行数据补全。
4. 实验结果与分析我们针对一个电影评分矩阵进行了实验,使用了上述提出的CMC神经网络模型进行数据补全。
Toeplitz矩阵填充的中值修正的奇异值阈值算法
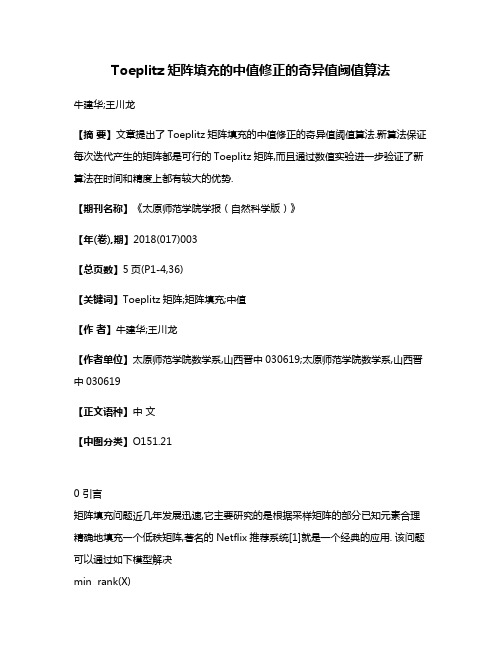
Toeplitz矩阵填充的中值修正的奇异值阈值算法牛建华;王川龙【摘要】文章提出了Toeplitz矩阵填充的中值修正的奇异值阈值算法.新算法保证每次迭代产生的矩阵都是可行的Toeplitz矩阵,而且通过数值实验进一步验证了新算法在时间和精度上都有较大的优势.【期刊名称】《太原师范学院学报(自然科学版)》【年(卷),期】2018(017)003【总页数】5页(P1-4,36)【关键词】Toeplitz矩阵;矩阵填充;中值【作者】牛建华;王川龙【作者单位】太原师范学院数学系,山西晋中030619;太原师范学院数学系,山西晋中030619【正文语种】中文【中图分类】O151.210 引言矩阵填充问题近几年发展迅速,它主要研究的是根据采样矩阵的部分已知元素合理精确地填充一个低秩矩阵,著名的Netflix推荐系统[1]就是一个经典的应用. 该问题可以通过如下模型解决min rank(X)(1)s.t. Xij=Mij(i.j)∈Ω.其中X,M∈Rn1×n2,并且M表示采样矩阵,Ω∈{1,…,n1}×{1,…,n2}表示采样矩阵已知元素的下标集合.事实上,由于矩阵关于秩是不连续的,所以模型(1)解决此问题存在一定的困难,所以Cand′es和Recht[2]提出模型(1)可以转化为如下凸优化问题: min ‖X*‖(2)s.t. Xij=Mij(i.j)∈Ω.其中矩阵X的核范数用表示,σk(X)表示矩阵X∈Rn1×n2的第k个大奇异值. 后来,针对模型(2),一些经典算法被提出,如奇异值阈值算法(the singular value thresholding,简称SVT)[3]、加速的近似梯度算法(the accelerated proximal gradient algorithm,简称APG)[4]、增广Lagrange乘子算法(the augmented Lagrange multiplier algorithm,简称ALM)[5]. 更多算法参考文献[6-10]. 针对Toeplitz矩阵,王川龙等人提出了Toeplitz矩阵填充的保结构算法[11],均值算法[12]等;对于Hankel矩阵,王川龙等人提出了Hankel矩阵填充的基于F模和无穷模保结构阈值算法[13-14].此外,,Qiao等人[15]根据Lanczos方法和FFT技术提出的Hankel矩阵的奇异值分解算法其复杂度为O(n2logn),但是一般矩阵的复杂度为O(n3),并且Toeplitz矩阵可以通过行列变换转化为Hankel矩阵,因为计算奇异值分解时间在整个矩阵填充中所占比例很大,所以新算法在时间上占一定的优势.为了方便,我们先来给出一些定义.定义1 一个n×n的Toeplitz矩阵T∈Rn1×n2有如下形式:显然,Toeplitz矩阵是由其第一行和第一列共2n-1个元素决定.定义2([16]) (奇异值分解(SVD)). 秩为r的矩阵X∈Rn1×n2,一定存在正交矩阵U∈Rn1×r和V∈Rn2×r,满足其中σ1≥σ2≥…≥σr>0.定义3([3]) (奇异值阈值算子). 对于任意参数τ≥0,矩阵的秩是r,X∈Rn1×n2,则存在X=UΣrVT,奇异值阈值算子Dτ定义为Dτ(X)=UDτ(Σ)VT, Dτ(Σ)=diag({σi-τ}+)其中符号说明:为方便起见,Rn1×n2表示n1×n2的实矩阵全体,Xij表示矩阵X位于(i,j)的元素,X=(x1,…,xn)表示矩阵X的一个列划分,‖X‖F和‖X‖*分别代表X的Frobenius和核范数,矩阵X和Y的内积〈X,Y〉=trace(XTY)表示,Ω∈{-n+1,…,n-1}表示观察到矩阵对角线的下标集合,集合Ω的补集用表示,集合Ω的正交投影PΩ满足:并且令1 算法在本节中,我们提出了Toeplitz矩阵填充的一种新算法,上述问题可以转化为如下凸优化模型min ‖X‖*(3)s.t. PΩ(X)=PΩ(M)其中X,M均是Toeplitz矩阵,Ω∈{-n+1,…,n-1}. 并且为了方便,用[Uk,Σk,Vk]τk=lansvd(Yk)表示对矩阵Yk进行奇异值分解.算法1(Mid-SVT算法)第一步:给定下标集合Ω,样本矩阵PΩ(M),参数τ0,0<c<1,误差ε及初始矩阵Y0=PΩ(M),k:=0.第二步:计算矩阵(Yk)的奇异值[Uk,Σk,Vk]τk=lansvd(Yk).令第三步:计算令第四步:如果停止;否则令τk+1=cτk,k:=k+1;转第二步.2 数值结果本节通过数值实验比较Mid-SVT算法与ALM算法表示取样密度,其中m表示已知的Toeplitz矩阵的元素个数,t(SVD)表示奇异值分解的时间,表示每次实验输出的最优解,#iter表示算法的迭代步数,此外,我们用秒表示时间单文,对于Mid-SVT算法中的参数我们分别取τ0=0.2‖PΩ(M)‖2,c=0.8,ε=10-8,对于ALM算法中的参数采用文献[5]的建议.表1 算法Mid-SVT和算法ALM算法的比较结果(p=0.1)size(n×n)秩(r)算法#iter 时间(s)t(SVD)‖Y^M‖F‖M‖F 1 00010Mid-SVTALM546284.153 2209.316 114.682185.1910.001 90.612 1 1 50010Mid-SVTALM5372173.651 7407.359 030.485362.2850.003 80.682 8 2 00010Mid-SVTALM5577387.771 6759.093 268.476645.4100.002 90.0913 2 50010Mid-SVTALM5485805.134 21.5518e+03132.6571374.1440.004 10.387 7 3 00010Mid-SVTALM55841.0630e+032.109 6e+03173.6031 802.8870.001 10.013 0 4 00010Mid-SVTALM56892.254 2e+034.377 2e+03432.7263 952.6720.001 20.005 7表2 算法Mid-SVT和算法ALM算法的比较结果(p=0.2)size(n×n)秩(r)算法#iter 时间(s)t(SVD)‖Y^M‖F‖M‖F 1 00010Mid-SVTALM556255.532 492.855 16.31974.4613.500 7e-060.228 1 1 50010Mid-SVTALM537296.294 6198.368 910.644172.4884.432 3e-060.148 2 2 00010Mid-SVTALM5677187.597 8514.804 226.624463.7177.452 8e-040.081 4 2 50010Mid-SVTALM6085365.206 1733.390 149.469652.9670.004 60.018 4 3 00010Mid-SVTALM5488793.5421.663 2e+03103.5411 493.1847.265 0e-050.005 6 4 00010Mid-SVTALM56841.402 3e+032.609 6e+03253.6322 302.7240.001 40.023 1表3 算法Mid-SVT和算法ALM算法的比较结果(p=0.3)size(n×n)秩(r)算法#iter 时间(s)t(SVD)‖Y^M‖F‖M‖F 1 00010Mid-SVTALM526233.386 278.321 23.43459.1635.016 6e-060.031 2 1 50010Mid-SVTALM527280.616 9189.598 06.028163.2324.022 1e-060.007 2 2 00010Mid-SVTALM5077149.172 8219.541 020.967171.5514.102 8e-061.374 1e-04 2 50010Mid-SVTALM5983257.113 6377.644 829.124298.1738.613 3e-060.001 4 3 00010Mid-SVTALM5185314.629 0631.619944.157548.0134.135 7e-060.006 6 4 00010Mid-SVTALM5189465.116 51.599 7e+0367.2791 384.6857.527 4e-060.001 2表4 算法Mid-SVT和算法ALM算法的比较结果(p=0.4)size(n×n)秩(r)算法#iter 时间(s)t(SVD)‖Y^M‖F‖M‖F 1 00010Mid-SVTALM516226.843 943.574 62.99434.7234.508 2e-060.001 4 1 50010Mid-SVTALM527266.1817164.73275.769142.8196.112 5e-060.010 3 200010Mid-SVTALM507684.112 3219.541 012.331171.5413.862 7e-061.374 1e-04 2 50010Mid-SVTALM5584163.818 0348.897 624.763292.5266.2095e-060.001 5 3 00010Mid-SVTALM5284193.523 4495.708428.161314.5644.398 8e-060.001 8 4 00010Mid-SVTALM5089346.713 81.090 4e+0356.193902.5643.765 3e-060.002 0注:由于采样矩阵M随机产生Toeplitz矩阵PΩ(M),其位置元素的位置不同,因此,个别实验的数据有所波动.3 总结在本文中,我们提出了Toeplitz矩阵填充的一种新算法——中值修正算法,根据表1-表4数据,我们可以看出新算法在填充总时间、最终误差等方面都比ALM算法优势明显,尤其是当p=0.1,p=0.2的时候,ALM算法收敛效果不理想,而新算法有较好的收敛性. 此外,从整体上看,随着采样率p的增大,计算时间越少,这也是合理的. 参考文献:【相关文献】[1] BENNETT J, LANNING S. The netflix prize[A]. In Proceeding of KDD Cup and Workshop, 2007[2] CAND`ES E J,RECHT B. Exact matrix completion via convex optimization[J]. Foundations of C-Omputational Mathematics, 2009,9(6):717-772[3] CAI J F,CANDES E J,SHEN Z.A singular value thresholding algorithm for matrix completion[J].SIAM Journal Optimization, 2010, 20(4): 1956-1982[4] TOH K C, YUN S. An accelerated proximal gradient algorithm for under norm regularized least Squares problems[J]. Pacific Journal of Optimization, 2010,6(3): 615-640 [5] LIN Z, CHEN M, WU L, et al. The augmented Lagrange multiplier method for exact recovery of Corrputed low-rank matrices[M]. UTUC Technicial Report UIUL-ENG-09-2214, 2010[6] FAZE M. Matrix rank minimization with application[D].Stanford University, 2002[7] FEZEL M, HINDI H, BOYED S P. Log-det heuristic for matrix rank minimization with applicati-ons to Hankel and Euclidean distance matrices[J]. In Proceedings of theAmerican Control Conference, 2003, 3: 2156-2162[8] MA S, GOLDFARB D,CHEN L.Fixed point and bregman iterative methods for matrix rank mini-Mization[J]. Mathematical Programming, 2011,128(1): 321-353[9] CAND`es E J, PLAN Y. Matrix completion with noise[J]. Proceeding of the IEEE, 2010,98(9):925-926[10] CAI J F, OSHER S.Fast singular value thresholding without singular value decomposition[J].Methods and Applications of Ananlysis. 2013, 20: 335-352[11] 王川龙,李超.Toeplitz矩阵填充的保结构算法[J].中国科学(数学),2016,46(8):1191-1206[12] WANG C L,LI C.A mean value algorithm for toeplitz matrix completion[J].Applied Ma-thmatics Letters,2015,41:35-40[13] WANG C L, ZHANG J M.基于F-模的Hankel矩阵填充的保结构阈值算法[J].数值计算与计算机应用,2017,39(1):60-72[14] WANG C L,ZHANG J M.基于l∞-模的Hankel矩阵填充的保结构阈值算法[J].应用数学学报(已接受)[15] XU W, QIAL S. A fast singular value alforithm for square Hankel matrices[J]. Linear Algebra and its Applications, 2008, 428(2): 550-563[16] GOLUB G H, VAN Loan C F. Matrix Computations (3rd edn). Johns Hopkins University Press,2008,428(2):550-563。
InductiveMatrixCompletionBasedonGraphNeuralNe。。。
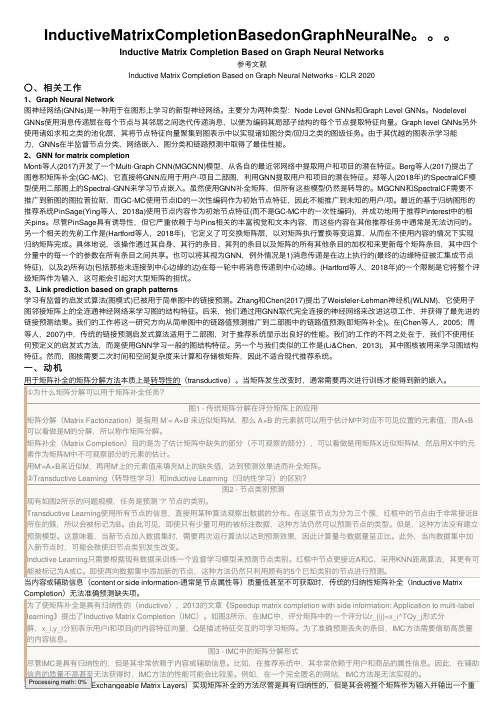
InductiveMatrixCompletionBasedonGraphNeuralNe。
Inductive Matrix Completion Based on Graph Neural Networks参考⽂献Inductive Matrix Completion Based on Graph Neural Networks - ICLR 2020〇、相关⼯作1、Graph Neural Network图神经⽹络(GNNs)是⼀种⽤于在图形上学习的新型神经⽹络。
主要分为两种类型:Node Level GNNs和Graph Level GNNs。
Nodelevel GNNs使⽤消息传递层在每个节点与其邻居之间迭代传递消息,以便为编码其局部⼦结构的每个节点提取特征向量。
Graph level GNNs另外使⽤诸如求和之类的池化层,其将节点特征向量聚集到图表⽰中以实现诸如图分类/回归之类的图级任务。
由于其优越的图表⽰学习能⼒,GNNs在半监督节点分类、⽹络嵌⼊、图分类和链路预测中取得了最佳性能。
2、GNN for matrix completionMonti等⼈(2017)开发了⼀个Multi-Graph CNN(MGCNN)模型,从各⾃的最近邻⽹络中提取⽤户和项⽬的潜在特征。
Berg等⼈(2017)提出了图卷积矩阵补全(GC-MC),它直接将GNN应⽤于⽤户-项⽬⼆部图,利⽤GNN提取⽤户和项⽬的潜在特征。
郑等⼈(2018年)的SpectralCF模型使⽤⼆部图上的Spectral-GNN来学习节点嵌⼊。
虽然使⽤GNN补全矩阵,但所有这些模型仍然是转导的。
MGCNN和SpectralCF需要不推⼴到新图的图拉普拉斯,⽽GC-MC使⽤节点ID的⼀次性编码作为初始节点特征,因此不能推⼴到未知的⽤户/项。
最近的基于归纳图形的推荐系统PinSage(Ying等⼈,2018a)使⽤节点内容作为初始节点特征(⽽不是GC-MC中的⼀次性编码),并成功地⽤于推荐Pinterest中的相关pins。
《Matrix培训》PPT课件

一个预先组装的 “一体化云基础设施” 解决 方案 :由集成设计的软硬件与基于HP适应 性基础设施模式的服务发布组成,简化订
购、处理、实现与实施的每一步骤。
ERP
CRM
Data Warehouse
Database
Mail and Messaging
File, Print, Infrastructure
未来的业务将构建在融合基础设施之电源散热管理软件网络服务器存储融合基础设施融合基础设施延伸虚拟化价值到所有基础设施加速体现业务价值虚拟化的模块化的融合基础设施bladesystemmatrix揭开matrix面纱虚拟联接虚拟化lan和san联接insight容量规划orchestratio灾难恢复storageworksevasanmatrixsupportsanyclasscertifiedfcsantargetintegrityproliant刀片服务器一体化服务融合的融合的基础设施基础设施matrixmatrixorchestratioorchestrationn环境环境合作伙伴集成合作伙伴集成与简化交付与简化交付sharedstorageintegrityproliantcomputeconvergedvirtual8gbfcdrautofailoverworkflowautomationselfserviceportalicapfinancingsetupinstallincludedfactoryexpressintegrationpartnerappsnetworkmgmtoscapacityplanningbladesystemmatrix单域管理达1000个物理或虚拟服务器bladematrix融合基础设施体系结构虚拟资源池集成的计算内存存储网络灵活的结构一次性连线动态装配始终可预知的基础设施运营环境使能共享服务管理数据中心智能能耗管理跨系统与跨设备的智能能源消耗监控服务请求服务交付利用matrix融合基础设施解决基础设施供应自动化业务部门选择应用访问自助服务门户挑选应用基础设施模板资源自动供应工具确定资源验证资源分配合适的规模
博世矩阵培训资料

4
Internal | STCN/PRM | 2009 | Security System | © Robert Bosch GmbH 2009. All rights reserved, also regarding any disposal, exploitation, reproduction, editing, distribution, as well as in the event of applications for industrial property rights.
Security Systems
9
Internal | STCN/PRM | 2009 | Security System | © Robert Bosch GmbH 2009. All rights reserved, also regarding any disposal, exploitation, reproduction, editing, distribution, as well as in the event of applications for industrial property rights.
Security Systems
1
Internal | STCN/PRM | 2009 | Security System | © Robert Bosch GmbH 2009. All rights reserved, also regarding any disposal, exploitation, reproduction, editing, distribution, as well as in the event of applications for industrial property rights.
MATLAB选修课讲义八讲
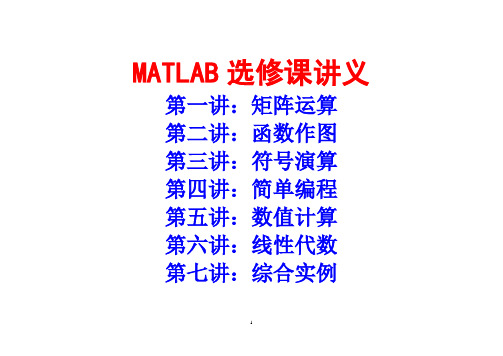
MATLAB选修课讲义第一讲:矩阵运算第二讲:函数作图第三讲:符号演算第四讲:简单编程第五讲:数值计算第六讲:线性代数第七讲:综合实例第一讲:矩阵运算1.基本操作启动退出终止(Alt+. 或Ctrl +C)翻页召回命令分隔符,禁显符;续行符…注释符%设置显示格式format 常用:short,short g,long 清除变量clear关闭图形close清除图形clf演示Demo帮助help2.基本常数pi I j inf eps NaN exp(1)3.算术运算+ - * /, \, ^ sqrt .*./.^4.内部函数(一般都有数组运算功能)sin(x) tan(x) asin(x) atan(x)abs(x) round(x) floor(x) ceil(x)log(x) log10(x) length(v) size(A) sign(x) [y, p]=sort(x)5.矩阵运算(要熟练掌握)(1)矩阵生成:手工输入:[1 2 3; 4 5 6]; 1:2:10输入数组: linspace(a, b, n)命令输入:zeros(m,n) ones(m,n) eye(n)magic(n) rand(m, n)diag(A) diag ( [a11 a22 . . . a nn] ) (2)矩阵操作赋值A(i, j) =2 A(2, :)=[1 2 3]删除A( [2,3], :)=[ ] 添加A(6,8)=5定位find(A>0) 定位赋值A(A<0)= -1 由旧得新B=A([2,3,1], :) B=A([1,3],[2,1])矩阵拼接C=[A, B] C=[A; B]定位矩阵B=(A>1) B=(A==1)下三角阵tril(A) 上三角阵triu(A)左右翻转fliplr(A) 上下翻转flipud(A)重排矩阵reshape(A, m, n)(3)矩阵运算:转置A’和A+B 差A-B 积A*B左除A\b(=A-1 b)右除b/A(=b A-1 )幂A^k点乘A.*B 点除A./B 点幂A.^2行列式det(A) 数量积dot(a,b) 向量积cross(a,b)行最简形rref(A) 逆矩阵inv(A) 迹trace(A)矩阵秩rank(A) 特征值eig(A) 基础解系null(A,’r’) 方程组特解x=A\b注意:2+A,sin(A)练习一:矩阵操作1、用尽可能简单的方法生成下列矩阵:102000100012101/21/31/1112040022002311/31/41/12,,,0330600054082210010191/111/121/20000750⎡⎤⎡⎤⎡⎤⎡⎤⎢⎥-⎢⎥⎢⎥⎢⎥⎢⎥-⎢⎥⎢⎥⎢⎥⎢⎥-⎢⎥⎢⎥⎢⎥⎢⎥-⎢⎥⎢⎥⎢⎥⎢⎥--⎣⎦⎣⎦⎣⎦⎢⎥-⎣⎦2、设有分块矩阵⎪⎪⎭⎫ ⎝⎛=⨯⨯⨯2232233S O R E A ,⎪⎪⎭⎫⎝⎛⋅=⨯⨯⨯23222233E O J R E B ,其中23,E E 是单位矩阵,32⨯O 是零矩阵,23⨯R 是随机矩阵,⎪⎪⎭⎫ ⎝⎛=⨯011022S ,J是2阶全1矩阵,验证B A =2。
recosystem 推荐系统:矩阵分解方法说明书
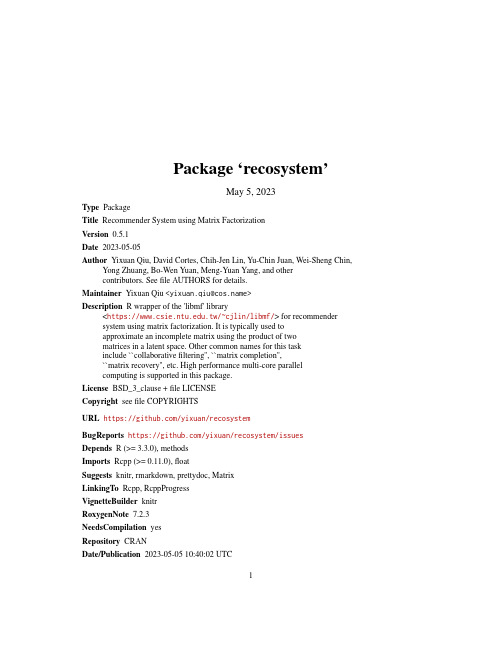
Package‘recosystem’May5,2023Type PackageTitle Recommender System using Matrix FactorizationVersion0.5.1Date2023-05-05Author Yixuan Qiu,David Cortes,Chih-Jen Lin,Yu-Chin Juan,Wei-Sheng Chin, Yong Zhuang,Bo-Wen Yuan,Meng-Yuan Yang,and othercontributors.Seefile AUTHORS for details.Maintainer Yixuan Qiu<*******************>Description R wrapper of the'libmf'library<https://.tw/~cjlin/libmf/>for recommendersystem using matrix factorization.It is typically used toapproximate an incomplete matrix using the product of twomatrices in a latent space.Other common names for this taskinclude``collaborativefiltering'',``matrix completion'',``matrix recovery'',etc.High performance multi-core parallelcomputing is supported in this package.License BSD_3_clause+file LICENSECopyright seefile COPYRIGHTSURL https:///yixuan/recosystemBugReports https:///yixuan/recosystem/issuesDepends R(>=3.3.0),methodsImports Rcpp(>=0.11.0),floatSuggests knitr,rmarkdown,prettydoc,MatrixLinkingTo Rcpp,RcppProgressVignetteBuilder knitrRoxygenNote7.2.3NeedsCompilation yesRepository CRANDate/Publication2023-05-0510:40:02UTC1R topics documented:data_source (2)output (4)output_format (5)predict (6)Reco (8)train (8)tune (11)Index14 data_source Specifying Data SourceDescriptionFunctions in this page are used to specify the source of data in the recommender system.They are intended to provide the input argument of functions such as$tune(),$train(),and$predict().Currently three data formats are supported:datafile(via function data_file()),data in mem-ory as R objects(via function data_memory()),and data stored as a sparse matrix(via function data_matrix()).Usagedata_file(path,index1=FALSE,...)data_memory(user_index,item_index,rating=NULL,index1=FALSE,...)data_matrix(mat,...)Argumentspath Path to the datafile.index1Whether the user indices and item indices start with1(index1=TRUE)or0 (index1=FALSE)....Currently unused.user_index An integer vector giving the user indices of rating scores.item_index An integer vector giving the item indices of rating scores.rating A numeric vector of the observed entries in the rating matrix.Can be specified as NULL for testing data,in which case it is ignored.mat A dgTMatrix(if it has ratings/values)or ngTMatrix(if it is binary)sparse ma-trix,with users corresponding to rows and items corresponding to columns.DetailsIn$tune()and$train(),functions in this page are used to specify the source of training data.data_file()expects a textfile that describes a sparse matrix in triplet form,i.e.,each line in the file contains three numbersrow col valuerepresenting a number in the rating matrix with its location.In real applications,it typically looks likeuser_index item_index ratingThe‘smalltrain.txt’file in the‘dat’directory of this package shows an example of training data file.If the sparse matrix is given as a dgTMatrix or ngTMatrix object(triplets/COO format defined in the Matrix package),then the function data_matrix()can be used to specify the data source.If user index,item index,and ratings are stored as R vectors in memory,they can be passed to data_memory()to form the training data source.By default the user index and item index start with zeros,and the option index1=TRUE can be set if they start with ones.From version0.4recosystem supports two special types of matrix factorization:the binary matrix factorization(BMF),and the one-class matrix factorization(OCMF).BMF requires ratings to take value from−1,1,and OCMF requires all the ratings to be positive.In$predict(),functions in this page provide the source of testing data.The testing data have the same format as training data,except that the value(rating)column is not required,and will be ignored if it is provided.The‘smalltest.txt’file in the‘dat’directory of this package shows an example of testing datafile.ValueAn object of class"DataSource"as required by$tune(),$train(),and$predict().Author(s)Yixuan Qiu<https://statr.me>See Also$tune(),$train(),$predict()4output output Exporting Factorization MatricesDescriptionThis method is a member function of class"RecoSys"that exports the user score matrix P and the item score matrix Q.Prior to calling this method,model needs to be trained using member function$train().The common usage of this method isr=Reco()r$train(...)r$output(out_P=out_file("mat_P.txt"),out_Q=out_file("mat_Q.txt"))Argumentsr Object returned by Reco().out_P An object of class Output that specifies the output format of the user matrix, typically returned by function out_file(),out_memory()or out_nothing().out_file()writes the matrix into afile,with each row representing a user andeach column representing a latent factor.out_memory()exports the matrix intothe return value of$output().out_nothing()means the matrix will not beexported.out_Q Ditto,but for the item matrix.ValueA list with components P and Q.They will befilled with user or item matrix if out_memory()isused in the function argument,otherwise NULL will be returned.Author(s)Yixuan Qiu<https://statr.me>ReferencesW.-S.Chin,Y.Zhuang,Y.-C.Juan,and C.-J.Lin.A Fast Parallel Stochastic Gradient Method for Matrix Factorization in Shared Memory Systems.ACM TIST,2015.W.-S.Chin,Y.Zhuang,Y.-C.Juan,and C.-J.Lin.A Learning-rate Schedule for Stochastic Gradient Methods to Matrix Factorization.PAKDD,2015.W.-S.Chin,B.-W.Yuan,M.-Y.Yang,Y.Zhuang,Y.-C.Juan,and C.-J.Lin.LIBMF:A Library for Parallel Matrix Factorization in Shared-memory Systems.Technical report,2015.See Also$train(),$predict()output_format5 Examplestrain_set=system.file("dat","smalltrain.txt",package="recosystem")r=Reco()set.seed(123)#This is a randomized algorithmr$train(data_file(train_set),out_model=file.path(tempdir(),"model.txt"),opts=list(dim=10,nmf=TRUE))##Write P and Q matrices to filesP_file=out_file(tempfile())Q_file=out_file(tempfile())r$output(P_file,Q_file)head(read.table(P_file@dest,header=FALSE,sep=""))head(read.table(Q_file@dest,header=FALSE,sep=""))##Skip P and only export Qr$output(out_nothing(),Q_file)##Return P and Q in memoryres=r$output(out_memory(),out_memory())head(res$P)head(res$Q)output_format Specifying Output FormatDescriptionFunctions in this page are used to specify the format of output results.They are intended to provide the argument of functions such as$output()and$predict().Currently there are three types of output:out_file()indicates that the result should be written into afile,out_memory()makes the result to be returned as R objects,and out_nothing()means the result is not needed and will not be returned.Usageout_file(path,...)out_memory(...)out_nothing(...)Argumentspath Path to the outputfile....Currently unused.ValueAn object of class"Output"as required by$output()and$predict().Author(s)Yixuan Qiu<https://statr.me>See Also$output(),$predict()predict Recommender Model PredictionsDescriptionThis method is a member function of class"RecoSys"that predicts unknown entries in the rating matrix.Prior to calling this method,model needs to be trained using member function$train().The common usage of this method isr=Reco()r$train(...)r$predict(test_data,out_pred=out_file("predict.txt")Argumentsr Object returned by Reco().test_data An object of class"DataSource"that describes the source of testing data,typi-cally returned by function data_file(),data_memory(),or data_matrix().out_pred An object of class Output that specifies the output format of prediction,typ-ically returned by function out_file(),out_memory()or out_nothing().out_file()writes the result into afile,out_memory()exports the vector of pre-dicted values into the return value of$predict(),and out_nothing()meansthe result will be neither returned nor written into afile(but computation willstill be conducted).Author(s)Yixuan Qiu<https://statr.me>ReferencesW.-S.Chin,Y.Zhuang,Y.-C.Juan,and C.-J.Lin.A Fast Parallel Stochastic Gradient Method for Matrix Factorization in Shared Memory Systems.ACM TIST,2015.W.-S.Chin,Y.Zhuang,Y.-C.Juan,and C.-J.Lin.A Learning-rate Schedule for Stochastic Gradient Methods to Matrix Factorization.PAKDD,2015.W.-S.Chin,B.-W.Yuan,M.-Y.Yang,Y.Zhuang,Y.-C.Juan,and C.-J.Lin.LIBMF:A Library for Parallel Matrix Factorization in Shared-memory Systems.Technical report,2015.See Also$train()Examples##Not run:train_file=data_file(system.file("dat","smalltrain.txt",package="recosystem")) test_file=data_file(system.file("dat","smalltest.txt",package="recosystem"))r=Reco()set.seed(123)#This is a randomized algorithmopts_tune=r$tune(train_file)$minr$train(train_file,out_model=NULL,opts=opts_tune)##Write predicted values into fileout_pred=out_file(tempfile())r$predict(test_file,out_pred)##Return predicted values in memorypred=r$predict(test_file,out_memory())##If testing data are stored in memorytest_df=read.table(test_file@source,sep="",header=FALSE)test_data=data_memory(test_df[,1],test_df[,2])pred2=r$predict(test_data,out_memory())##Compare resultsprint(scan(out_pred@dest,n=10))head(pred,10)head(pred2,10)##If testing data are stored as a sparse matrixif(require(Matrix)){mat=Matrix::sparseMatrix(i=test_df[,1],j=test_df[,2],x=-1,repr="T",index1=FALSE)test_data=data_matrix(mat)pred3=r$predict(test_data,out_memory())print(head(pred3,10))}##End(Not run)Reco Constructing a Recommender System ObjectDescriptionThis function simply returns an object of class"RecoSys"that can be used to construct recom-mender model and conduct prediction.UsageReco()ValueReco()returns an object of class"RecoSys"equipped with methods$train(),$tune(),$output() and$predict(),which describe the typical process of building and tuning model,exporting fac-torization matrices,and predicting results.See their help documents for details.Author(s)Yixuan Qiu<https://statr.me>ReferencesW.-S.Chin,Y.Zhuang,Y.-C.Juan,and C.-J.Lin.A Fast Parallel Stochastic Gradient Method for Matrix Factorization in Shared Memory Systems.ACM TIST,2015.W.-S.Chin,Y.Zhuang,Y.-C.Juan,and C.-J.Lin.A Learning-rate Schedule for Stochastic Gradient Methods to Matrix Factorization.PAKDD,2015.W.-S.Chin,B.-W.Yuan,M.-Y.Yang,Y.Zhuang,Y.-C.Juan,and C.-J.Lin.LIBMF:A Library for Parallel Matrix Factorization in Shared-memory Systems.Technical report,2015.See Also$tune(),$train(),$output(),$predict()train Training a Recommender ModelDescriptionThis method is a member function of class"RecoSys"that trains a recommender model.It will read from a training data source and create a modelfile at the specified location.The modelfile contains necessary information for prediction.The common usage of this method isr=Reco()r$train(train_data,out_model=file.path(tempdir(),"model.txt"),opts=list())Argumentsr Object returned by Reco().train_data An object of class"DataSource"that describes the source of training data,typi-cally returned by function data_file(),data_memory(),or data_matrix().out_model Path to the modelfile that will be created.If passing NULL,the model will bestored in-memory,and model matrices can then be accessed under r$model$matrices.opts A number of parameters and options for the model training.See section Param-eters and Options for details.Parameters and OptionsThe opts argument is a list that can supply any of the following parameters:loss Character string,the loss function.Default is"l2",see below for details.dim Integer,the number of latent factors.Default is10.costp_l1Numeric,L1regularization parameter for user factors.Default is0.costp_l2Numeric,L2regularization parameter for user factors.Default is0.1.costq_l1Numeric,L1regularization parameter for item factors.Default is0.costq_l2Numeric,L2regularization parameter for item factors.Default is0.1.lrate Numeric,the learning rate,which can be thought of as the step size in gradient descent.Default is0.1.niter Integer,the number of iterations.Default is20.nthread Integer,the number of threads for parallel computing.Default is1.nbin Integer,the number of bins.Must be greater than nthread.Default is20.nmf Logical,whether to perform non-negative matrix factorization.Default is FALSE.verbose Logical,whether to show detailed information.Default is TRUE.The loss option may take the following values:For real-valued matrix factorization,"l2"Squared error(L2-norm)"l1"Absolute error(L1-norm)"kl"Generalized KL-divergenceFor binary matrix factorization,"log"Logarithmic error"squared_hinge"Squared hinge loss"hinge"Hinge lossFor one-class matrix factorization,"row_log"Row-oriented pair-wise logarithmic loss"col_log"Column-oriented pair-wise logarithmic lossAuthor(s)Yixuan Qiu<https://statr.me>ReferencesW.-S.Chin,Y.Zhuang,Y.-C.Juan,and C.-J.Lin.A Fast Parallel Stochastic Gradient Method for Matrix Factorization in Shared Memory Systems.ACM TIST,2015.W.-S.Chin,Y.Zhuang,Y.-C.Juan,and C.-J.Lin.A Learning-rate Schedule for Stochastic Gradient Methods to Matrix Factorization.PAKDD,2015.W.-S.Chin,B.-W.Yuan,M.-Y.Yang,Y.Zhuang,Y.-C.Juan,and C.-J.Lin.LIBMF:A Library for Parallel Matrix Factorization in Shared-memory Systems.Technical report,2015.See Also$tune(),$output(),$predict()Examples##Training model from a data filetrain_set=system.file("dat","smalltrain.txt",package="recosystem")train_data=data_file(train_set)r=Reco()set.seed(123)#This is a randomized algorithm#The model will be saved to a filer$train(train_data,out_model=file.path(tempdir(),"model.txt"),opts=list(dim=20,costp_l2=0.01,costq_l2=0.01,nthread=1) )##Training model from data in memorytrain_df=read.table(train_set,sep="",header=FALSE)train_data=data_memory(train_df[,1],train_df[,2],rating=train_df[,3])set.seed(123)#The model will be stored in memoryr$train(train_data,out_model=NULL,opts=list(dim=20,costp_l2=0.01,costq_l2=0.01,nthread=1) )##Training model from data in a sparse matrixif(require(Matrix)){mat=Matrix::sparseMatrix(i=train_df[,1],j=train_df[,2],x=train_df[,3],repr="T",index1=FALSE)train_data=data_matrix(mat)r$train(train_data,out_model=NULL,opts=list(dim=20,costp_l2=0.01,costq_l2=0.01,nthread=1)) }tune11 tune Tuning Model ParametersDescriptionThis method is a member function of class"RecoSys"that uses cross validation to tune the model parameters.The common usage of this method isr=Reco()r$tune(train_data,opts=list(dim=c(10L,20L),costp_l1=c(0,0.1),costp_l2=c(0.01,0.1),costq_l1=c(0,0.1),costq_l2=c(0.01,0.1),lrate=c(0.01,0.1)))Argumentsr Object returned by Reco().train_data An object of class"DataSource"that describes the source of training data,typi-cally returned by function data_file(),data_memory(),or data_matrix().opts A number of candidate tuning parameter values and extra options in the model tuning procedure.See section Parameters and Options for details.ValueA list with two components:min Parameter values with minimum cross validated loss.This is a list that can be passed to the opts argument in$train().res A data frame giving the supplied candidate values of tuning parameters,and one column show-ing the loss function value associated with each combination.Parameters and OptionsThe opts argument should be a list that provides the candidate values of tuning parameters and some other options.For tuning parameters(dim,costp_l1,costp_l2,costq_l1,costq_l2,and lrate),users can provide a numeric vector for each one,so that the model will be evaluated on each combination of the candidate values.For other non-tuning options,users should give a single value.If a parameter or option is not set by the user,the program will use a default one.See below for the list of available parameters and options:dim Tuning parameter,the number of latent factors.Can be specified as an integer vector,with default value c(10L,20L).12tune costp_l1Tuning parameter,the L1regularization cost for user factors.Can be specified as a numeric vector,with default value c(0,0.1).costp_l2Tuning parameter,the L2regularization cost for user factors.Can be specified as a numeric vector,with default value c(0.01,0.1).costq_l1Tuning parameter,the L1regularization cost for item factors.Can be specified as a numeric vector,with default value c(0,0.1).costq_l2Tuning parameter,the L2regularization cost for item factors.Can be specified as a numeric vector,with default value c(0.01,0.1).lrate Tuning parameter,the learning rate,which can be thought of as the step size in gradient descent.Can be specified as a numeric vector,with default value c(0.01,0.1).loss Character string,the loss function.Default is"l2",see section Parameters and Options in $train()for details.nfold Integer,the number of folds in cross validation.Default is5.niter Integer,the number of iterations.Default is20.nthread Integer,the number of threads for parallel computing.Default is1.nbin Integer,the number of bins.Must be greater than nthread.Default is20.nmf Logical,whether to perform non-negative matrix factorization.Default is FALSE.verbose Logical,whether to show detailed information.Default is FALSE.progress Logical,whether to show a progress bar.Default is TRUE.Author(s)Yixuan Qiu<https://statr.me>ReferencesW.-S.Chin,Y.Zhuang,Y.-C.Juan,and C.-J.Lin.A Fast Parallel Stochastic Gradient Method for Matrix Factorization in Shared Memory Systems.ACM TIST,2015.W.-S.Chin,Y.Zhuang,Y.-C.Juan,and C.-J.Lin.A Learning-rate Schedule for Stochastic Gradient Methods to Matrix Factorization.PAKDD,2015.W.-S.Chin,B.-W.Yuan,M.-Y.Yang,Y.Zhuang,Y.-C.Juan,and C.-J.Lin.LIBMF:A Library for Parallel Matrix Factorization in Shared-memory Systems.Technical report,2015.See Also$train()Examples##Not run:train_set=system.file("dat","smalltrain.txt",package="recosystem")train_src=data_file(train_set)r=Reco()set.seed(123)#This is a randomized algorithmres=r$tune(train_src,tune13 opts=list(dim=c(10,20,30),costp_l1=0,costq_l1=0,lrate=c(0.05,0.1,0.2),nthread=2))r$train(train_src,opts=res$min)##End(Not run)Index∗modelsReco,8data_file,6,9,11data_file(data_source),2data_matrix,6,9,11data_matrix(data_source),2data_memory,6,9,11data_memory(data_source),2data_source,2out_file,4,6out_file(output_format),5out_memory,4,6out_memory(output_format),5out_nothing,4,6out_nothing(output_format),5output,4,5,6,8,10output_format,5predict,2–6,6,8,10Reco,4,6,8,9,11train,2–4,6–8,8,11,12tune,2,3,8,10,1114。
c课程设计矩阵复杂
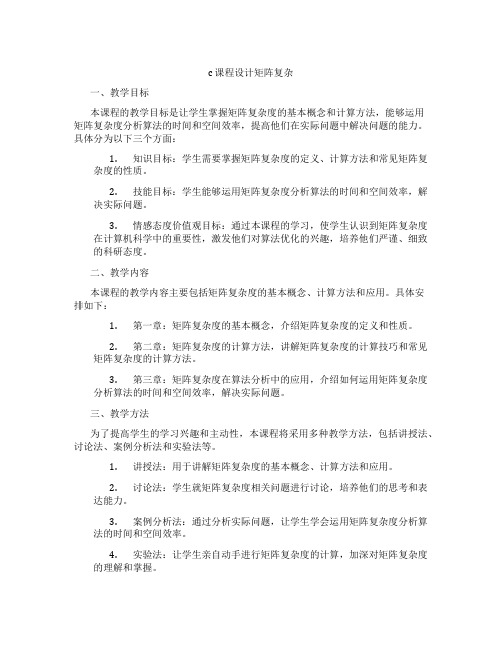
c 课程设计矩阵复杂一、教学目标本课程的教学目标是让学生掌握矩阵复杂度的基本概念和计算方法,能够运用矩阵复杂度分析算法的时间和空间效率,提高他们在实际问题中解决问题的能力。
具体分为以下三个方面:1.知识目标:学生需要掌握矩阵复杂度的定义、计算方法和常见矩阵复杂度的性质。
2.技能目标:学生能够运用矩阵复杂度分析算法的时间和空间效率,解决实际问题。
3.情感态度价值观目标:通过本课程的学习,使学生认识到矩阵复杂度在计算机科学中的重要性,激发他们对算法优化的兴趣,培养他们严谨、细致的科研态度。
二、教学内容本课程的教学内容主要包括矩阵复杂度的基本概念、计算方法和应用。
具体安排如下:1.第一章:矩阵复杂度的基本概念,介绍矩阵复杂度的定义和性质。
2.第二章:矩阵复杂度的计算方法,讲解矩阵复杂度的计算技巧和常见矩阵复杂度的计算方法。
3.第三章:矩阵复杂度在算法分析中的应用,介绍如何运用矩阵复杂度分析算法的时间和空间效率,解决实际问题。
三、教学方法为了提高学生的学习兴趣和主动性,本课程将采用多种教学方法,包括讲授法、讨论法、案例分析法和实验法等。
1.讲授法:用于讲解矩阵复杂度的基本概念、计算方法和应用。
2.讨论法:学生就矩阵复杂度相关问题进行讨论,培养他们的思考和表达能力。
3.案例分析法:通过分析实际问题,让学生学会运用矩阵复杂度分析算法的时间和空间效率。
4.实验法:让学生亲自动手进行矩阵复杂度的计算,加深对矩阵复杂度的理解和掌握。
四、教学资源本课程将采用教材《矩阵复杂度分析与应用》作为主要教学资源,同时辅以参考书、多媒体资料和实验设备等。
教学资源将支持教学内容和教学方法的实施,丰富学生的学习体验。
五、教学评估本课程的评估方式包括平时表现、作业、考试等方面,以全面客观地评价学生的学习成果。
具体安排如下:1.平时表现:通过课堂讨论、提问等环节,评估学生的参与程度和思考能力。
2.作业:布置适量的课后作业,让学生巩固所学知识,培养实际应用能力。
- 1、下载文档前请自行甄别文档内容的完整性,平台不提供额外的编辑、内容补充、找答案等附加服务。
- 2、"仅部分预览"的文档,不可在线预览部分如存在完整性等问题,可反馈申请退款(可完整预览的文档不适用该条件!)。
- 3、如文档侵犯您的权益,请联系客服反馈,我们会尽快为您处理(人工客服工作时间:9:00-18:30)。
Σ = Σ1I2
0
0 diag(σ3 · · · σn)
Q0 0I
Q0
QT 0
=
Σ
,
0I
0I
QT 0 0I
where QQT = I. Then we have
UΣVT = U Q 0 Σ QT 0 VT .
0I
0I
In general, for any Ui and Vi that satisfy A = UiΣViT , we can rewrite in the following form as
tr(X−1) − ttr(X−1YX−1) + t2tr((X−1Y)2X−1) − · · · − tr(X−1)
= lim
t↓0
t
= −tr(X−1YX−1)
= −tr(X−2Y)
8.3 Subgradient
Definition 8.2. If function f is convex and proper (which means domf = {x ∈ E|f (x) < ∞} is non-empty), φ is said to be subgradient of f (x) at xˆ, if it satisfies φ, x − xˆ ≤ f (x) − f (xˆ) for all x ∈ E.
Let Z = (zij) denote user i rates movie j with ranking zij ∈ {1, 2, 3, 4, 5}. However, some movies are not rated by any people, i.e. zij is missing. Our purpose is to complete the ranking matrix. Let X be the m × n complete matrix and Ω = {(i, j)|zij is observed}. We can reasonably suppose that X is a low rank matrix. Our purpose is to minimize the error between Z and X with the constraint that X is low rank, i.e.
simply say f is differentiable (on E).
Example 8.1. f (X) = log |X|, X ∈ Sn++, find f (X; Y), where Y ∈ Sn++.
Solution:
log |(X + tY)| − log |X|
f (X, Y) = lim
8-4
There are three examples of Schatten p-norm:
• p = 1, A ∗ =
n i=1
σi.
• p = 2, A F =
n i=1
σi2.
• p = ∞, A ∞ = σ1. We call σ1 the spectrum radius, and A ∞ is also called spectral norm.
f (xˆ; d) = lim
(2)
t↓0
t
when the limit exists. When the directional derivative f (xˆ; d) is actually linear in d, that
is f (xˆ; d) = a, d for some element a of E. Then, we say f is (Gaˆteanx) differentiable at xˆ with (Gˆateanx) derivative ∇f (xˆ) = a. If f is differentiable at every point in E, then we
1 min X2
(zij − xij )2
(i,j)∈Ω
s.t. X a low rank matrix
Since X is a low rank matrix, we cannot merely use Z ≈ UT X as an approximation of Z. We need to add a sparse matrix S, i.e. Z = X + S, for a matrix can always be represented as the sum of a low rank matrix and a sparse matrix. Then our objective function is:
=
X−
1 2
X−
1 2
YX−
1 2
X
1 2
,
we
have
X−1Y
∼
X−
1 2
YX−
1 2
,
i.e.
X−1Y
and
X−
1 2
YX−
1 2
have the same eigenvalues.
Consider that X and Y are positive definite
symmetric
matrices,
then
X−
1 2
=
(X−
1 2
)T
= 0 and for any z = 0, zT Yz > 0.
Hence
zT
X−
1 2
YX−
1 2
z
>
0
and
X−
1 2
YX−
1 2
is
positive
definite.
Example 8.2. f (X) = tr(AT X), A ∈ Rp×m, X ∈ Rp×n, find f (X; Y)
Lemma 8.1. Let A and R be given m × n matrices, · is Schatten p-norm, φ(·) is the corresponding norm on singular vector, then there is a SVD of A such that
Machine Learning
Matrix Completion
Lecture Notes 8: Matrix Completion
Professor: Zhihua Zhang
Scribe:Yuxi Zhang, Ruotian Luo
8 Matrix Completion
8.1 Problem Background
min
X,S
1 2
Z−X−S
2 F
+
λ1
X
∗ + λ2
S
1
(1)
However, this objective function is not differentiable. Therefore, we need to introduce the definition of directional derivative and subgradient.
t↓0
t
log |X(I + tX−1Y)| − log |X|
=| + log |I + tX−1Y| − log |X|
= lim
t↓0
t
log |I + tX−1Y|
= lim
t↓0
t
= lim
n i=1
log
(1
+
tλi
(X−1
Y))
t↓0
t
=
lim
t↓0
n i=1
Proposition 8.1. For any convex proper function f : E → (−∞, ∞), the point xˆ is a global minimizer of f iff the condition 0 ∈ ∂f (xˆ) holds.
Recall the definition of general matrix norms: Definition 8.3. For A ∈ Rm×n, A is a function of A which satisfies the following conditions.
1. A ≥ 0
2. A = 0 iff A = 0
3. A + B ≤ A + B
8-3
4. αA = |α| A According to the definition, it’s easy to derive that matrix norm is convex:
αA + (1 − α)B ≤ α A + (1 − α) B
8-2
Solution:
tr(AT (X + tY)) − tr(AT X)
f (X, Y) = lim
t↓0
t
tr(AT X) + tr(tAT Y)) − tr(AT X)
= lim
t↓0
t
= tr(AT Y)
= A, Y
Example 8.3. f (X) = tr(X−1), find f (X, Y)
Addition condition: Definition 8.4. A matrix norm · is called consistent if:
AB ≤ A B .
We here consider a kind of norm function which satisfy UT AV = A , U,V are orthogonal matrices. UT U = UUT = I and VT V = VVT = I. A = UΣVT , A is m × n matrix, U is m × m matrix; Σ is m × n; V is n × n.