hdu hdoj 1159 Common Subsequence
Unify 桌面电话说明书
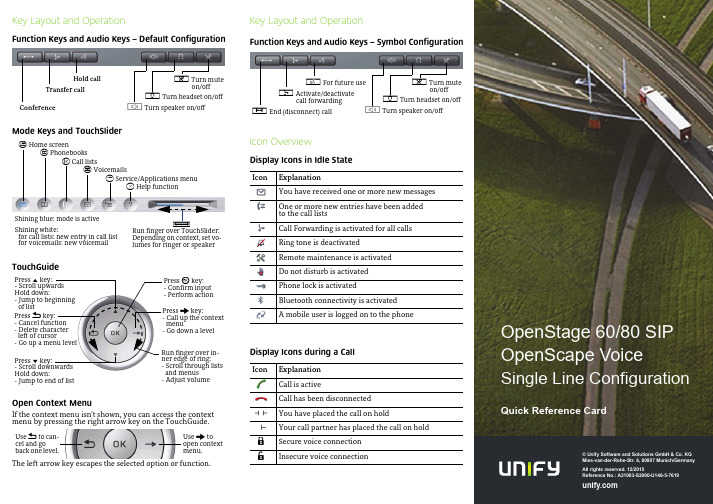
© Unify Software and Solutions GmbH & Co. KGMies-van-der-Rohe-Str. 6, 80807 Munich/GermanyAll rights reserved. 12/2015Reference No.: A31003-S2000-U146-5-7619Key Layout and OperationFunction Keys and Audio Keys – Default ConfigurationMode Keys and TouchSliderTouchGuideOpen Context MenuIf the context menu isn’t shown, you can access the contextmenu by pressing the right arrow key on the TouchGuide.The left arrow key escapes the selected option or function.Hold callTransfer callConferencep Turn muteon/offo Turn headset on/offn Turn speaker on/offt Home screenu Phonebooksw Call listsx Voicemailsv Service/Applications menuy Help functionRun finger over TouchSlider:Depending on context, set vo-lumes for ringer or speakerXShining blue: mode is activeShining white:for call lists: new entry in call listfor voicemails: new voicemailPress m key:- Scroll upwardsHold down:- Jump to beginningof listPress l key:- Scroll downwardsHold down:- Jump to end of listPress g key:- Call up the contextmenu- Go down a levelPress h key:- Cancel function- Delete characterleft of cursor- Go up a menu levelPress i key:- Confirm input- Perform actionRun finger over in-ner edge of ring:- Scroll through listsand menus- Adjust volumeUse g toopen contextmenu.Use h to can-cel and goback one level.Key Layout and OperationFunction Keys and Audio Keys – Symbol ConfigurationIcon OverviewDisplay Icons in Idle StateDisplay Icons during a CallIcon ExplanationYou have received one or more new messagesOne or more new entries have been addedto the call listsCall Forwarding is activated for all callsRing tone is deactivatedRemote maintenance is activatedDo not disturb is activatedPhone lock is activatedBluetooth connectivity is activatedA mobile user is logged on to the phoneIcon ExplanationCall is activeCall has been disconnectedYou have placed the call on holdYour call partner has placed the call on holdSecure voice connectionInsecure voice connectionq For future user Activate/deactivatecall forwardings End (disconnect) callp Turn muteon/offo Turn headset on/offn Turn speaker on/off{}OpenStage60/80SIPOpenScape VoiceSingle Line ConfigurationQuick Reference CardUsing your OpenStagePlace a Call•Lift handset, dial number and press i, or•dial number and lift handset, or•for handsfree call: dial number and press i.Answer a Call•Lift handset, or•for handsfree call: press n.End a Call•Hang up,or•to end a handsfree call: press n.Use the headset•Place a call: dial number and press i.•Answer or end a call: press o.Redial a Number1.Select "Redial" in the context menu and press i.2.Lift handset to use handset mode.Hold or Retrieve a Call•During a call press pre-programmed Hold key.•To retrieve a call: press pre-programmed Hold key.Make a Conference Call1.During a call with party A, press pre-programmed Conferencekey.2.Enter the phone number for party B and press i.3.Once connected with party B, press pre-programmed Confe-rence key.You are now connected in a conference with party A and B. Transfer a CallBlind transfer (no consultation):1.During the call with party A, select"Blind transfer" in the con-text menu and press i.2.Enter the phone number of party B and press i.Semi-attended transfer (transfer while ringing):1.During the call with party A, press pre-programmed Transferkey.2.Enter the phone number of party B and press i.3.When the phone starts to ring, select "Complete transfer"and press i.Attended transfer (with consultation):1.During the call with party A, press pre-programmed Transferkey.2.Enter the phone number of party B and press i.3.Announce the call to party B.4.Select "Complete Xfer" in the context menu and press ing your OpenStageProgramm Call Forwarding1.Press pre-programmed Call Forwarding Key.2.Select "Set a forwarding destiantion" and press i.3.Enter the destination number and press i.4.Select "Save&Exit" in the menu and press i.5.Press v to return to phone mode.Turn Call Forwarding on or off for All Calls•Press r for turning Call Forwarding on/off.Dial from the Call List1.Press w.2.The "Missed" tab will appear first, press w repeatedly for"Dialed", "Received", or "Forwarded" tabs.3.Select the desired phone number and press i.Activate Callback while calling1.Enter the destination phone number and press i.2.If there is no answer or the line is busy: select "Callback"from the context menu and press i.Deflect a Call while ringing1.Select "Deflect" from the context menu and press i.2.Enter a destination phone number and press i.Use Mute during a call•Press p for turning mute on or off.Switch to Speakerphone Mode during a Call•US mode:press n and hang up.•Default mode: hold down n until you hang up.Switch to Handset Mode during a Call•Lift handset.Save a Function to a Key1.Press and hold the desired programmable keyuntil a popup appears.2.Press i to confirm entering programming mode.3.Select "Normal" or "Shifted" and press i.4.Select desired function and press i.5.Define an appropriate key label and press i.6.In some cases: enter additional parameters and press i.7.Select "Save&Exit" in the context menu and press i.8.Press v to return to phone mode.List of Programmable Functions* only visible if provided by adminFunction ExplanationUnallocated Clears the keySelected dialing Dials a pre-defined numberRepeat dialing Calls the last dialed numberForward all calls Forwards all incoming callsForward no reply Forwards calls only if they are notansweredForward busy Forwards calls only when the line isbusyRinger off Switches the ringer off/onHold Places a call on holdAlternate Switches between two callsBlind transfer call Transfers a call without consultationTransfer call Transfers a call with consultationDeflect Deflects a call to another destinationShift Switches to the shifted key levelConference Places a conference callHeadset Answers a call using the headsetDo not disturb Switches the ringer off; callers hear thebusy signalGroup pickup Picks up a group callRepertory dial Dials pre-defined numbers and controlsequencesShow phone screen Toggles features hosted by OpenScapeVoiceMobility Login/Logoff for mobile usersDirected pickup Picks up another ringing phoneCallback Requests an automatic call back(busy/no answer)Cancel callbacks Cancels a callback requestConsultation Puts an active call on hold and providesa prompt for dialingDSS*Dials a pre-defined internal numberCall Waiting Notifies of a second incoming call whilein active callImmediate ring Ringing keyset line without delay(Executive/Assistant configuration)Preview Preview line details for shared linesAICS Zip tone*For headset operation only:auto answer and alert toneStart application Launches an application (short cut)Built in fwd Turns Call Forwarding on/off。
subsequence的用法
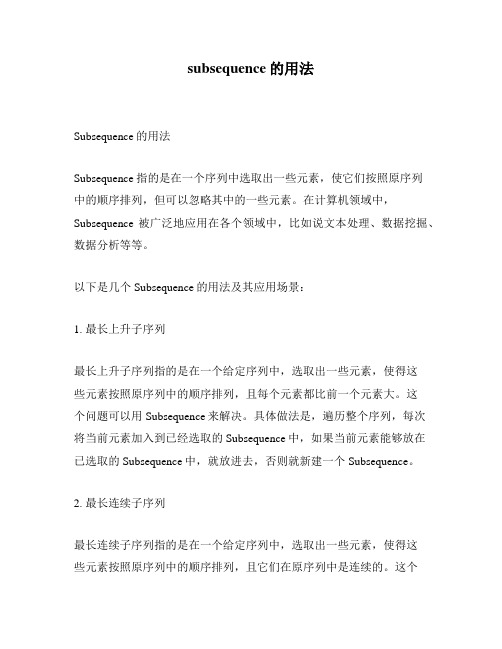
subsequence的用法Subsequence的用法Subsequence指的是在一个序列中选取出一些元素,使它们按照原序列中的顺序排列,但可以忽略其中的一些元素。
在计算机领域中,Subsequence被广泛地应用在各个领域中,比如说文本处理、数据挖掘、数据分析等等。
以下是几个Subsequence的用法及其应用场景:1. 最长上升子序列最长上升子序列指的是在一个给定序列中,选取出一些元素,使得这些元素按照原序列中的顺序排列,且每个元素都比前一个元素大。
这个问题可以用Subsequence来解决。
具体做法是,遍历整个序列,每次将当前元素加入到已经选取的Subsequence中,如果当前元素能够放在已选取的Subsequence中,就放进去,否则就新建一个Subsequence。
2. 最长连续子序列最长连续子序列指的是在一个给定序列中,选取出一些元素,使得这些元素按照原序列中的顺序排列,且它们在原序列中是连续的。
这个问题也可以用Subsequence来解决。
具体做法是,遍历整个序列,在当前元素不断增加的情况下,记录当前连续的Subsequence的长度,用一个变量max_length来记录最长的Subsequence长度。
3. 序列匹配序列匹配指的是给定两个序列,找到它们中最长的一个相同的Subsequence。
这个问题可以用动态规划来解决。
具体做法是,建立一个二维矩阵,行数与列数分别为两个序列的长度,然后利用动态规划算法,找到最长匹配Subsequence。
4. 生物信息学中的应用Subsequence在生物信息学中的应用非常广泛。
比如说,生物学家可以将DNA序列转换为字符串序列,然后利用Subsequence算法来寻找相同的DNA序列。
这种方法在基因组测序中非常常见。
总结Subsequence虽然是一个简单的概念,但它在计算机领域中的应用非常广泛,无论是最长上升子序列、最长连续子序列、序列匹配还是生物信息学中的应用,都离不开Subsequence。
HDUOJ训练题

OJ输入输出训练:HDOJ 1089 ~HDOJ 1096一、C语言基础练习1001 计算两点间的距离HDOJ 2001 1002 第几天?HDOJ 2005 1003 平方和与立方和HDOJ 2007 1004 水仙花数HDOJ 2010 1005 素数判定HDOJ 2012 1006 数列有序!HDOJ 2019 1007 发工资咯:)HDOJ 2021 1008 海选女主角HDOJ 2022 1009 求平均成绩HDOJ 2023 1010 汉字统计HDOJ 2030 1011 进制转换HDOJ 2031 1012 杨辉三角HDOJ 2032 1013 人见人爱A+B HDOJ 2033 1014 人见人爱A-B HDOJ 2034 1015 亲和数HDOJ 2040 1016 Sum Problem HDOJ 1001 1017 A + B Problem II HDOJ 1002 1018 Let the Balloon Rise HDOJ 1004 1019 Elevator HDOJ 1008 1020 FatMouse' Trade HDOJ 1009 1021 As Easy As A+B HDOJ 1040 1022 The Hardest Problem Ever HDOJ 1048 1023 Climbing Worm HDOJ 1049 1024 Text Reverse HDOJ 1062 1025 An Easy Task HDOJ 1076 1026 What Is Your Grade? HDOJ 1084二、简单数学题1001 最小公倍数HDOJ 1108 1002 Least Common Multiple HDOJ 1019 1003 人见人爱A^B HDOJ 0235 1004 Rightmost Digit HDOJ 1061 1005 Fibonacci Again HDOJ 1021 1006 Number Sequence HDOJ 1005 1007 The area HDOJ 1071 1008 吃糖果HDOJ 1205 1009 Sky数HDOJ 2097 1010 Box of Bricks HDOJ 20881011 简易版之最短距离HDOJ 20831012 Fibbonacci Number HDOJ 20701013 Coin Change HDOJ 20691014 A + B Again HDOJ 20571015 Lowest Common Multiple Plus HDOJ 20281016 Can you solve this equation? HDOJ 21991017 Strange fuction HDOJ 28991018 Pseudoprime numbers HDOJ 19051019 Delta-wave HDOJ 10301020 月之数HDOJ 25021021 又见GCD HDOJ 25041022 找新朋友HDOJ 12861023 七夕节HDOJ 12151024 完数HDOJ 1406三、递推求解1001 超级楼梯HDOJ 20411002 不容易系列之二HDOJ 20421003 一只小蜜蜂... HDOJ 20441004 不容易系列之(3)——LELE的RPG难题HDOJ 20451005 骨牌铺方格HDOJ 20461006 折线分割平面HDOJ 20501007 母牛的故事HDOJ 20181008 下沙的沙子有几粒?HDOJ 12671009 自共轭Ferrers图HDOJ 12461010 汉诺塔II HDOJ 12071011 悼念512汶川大地震遇难同胞——重建希望小学HDOJ 2190 1012 Children’s Queue HDOJ 12971013 Tiling_easy version HDOJ 25011014 统计问题HDOJ 25631015 Buy the Ticket HDOJ 11331016 Game of Connections HDOJ 11341017 Computer Transformation HDOJ 10411018 Children’s Queue HDOJ 12971019 The Number of Paths HDOJ 12931020 "下沙野骆驼"ACM夏令营HDOJ 129四、简单典型DP1001 数塔HDOJ 20841002 Super Jumping! Jumping! Jumping! HDOJ 10871003 免费馅饼HDOJ 11761004 Common Subsequence HDOJ 11591005 搬寝室HDOJ 14211006 Humble Numbers HDOJ 10581007 Max Sum HDOJ 10031008 Max Sum Plus Plus HDOJ 10241009 FatMouse's Speed HDOJ 11601010 Bone Collector HDOJ 26021011 Piggy-Bank HDOJ 11141012 I NEED A OFFER! HDOJ 12031013 悼念512汶川大地震遇难同胞——珍惜现在,感恩生活HDOJ 2191 1014 Coins HDOJ 2844五、简单博弈1001 Brave Game HDOJ 18461002 Good Luck in CET-4 Everybody! HDOJ 18471003 Fibonacci again and again HDOJ 18481004 Rabbit and Grass HDOJ 18491005 Being a Good Boy in Spring Festival HDOJ 18501006 kiki's game HDOJ 21471007 Public Sale HDOJ 21491008 悼念512汶川大地震遇难同胞——选拔志愿者HDOJ 21881009 丑数游戏1010 YLF's Game六、半程测试1001 CD HDOJ 37631002 Alaska HDOJ 37641003 Celebrity Split HDOJ 37651004 Knight's Trip HDOJ 37661005 Paintball HDOJ 37671006 Shopping HDOJ 37681007 Stack Machine HDOJ 37691008 Ideas HDOJ 37701009 HST HDOJ 37711010 Tunnelling the Earth H DOJ 3772七、母函数1001 Ignatius and the Princess III HDOJ 10281002 Square Coins HDOJ 13981003 Holding Bin-Laden Captive! HDOJ 10851004 Big Event in HDU HDOJ 11711005 Fruit HDOJ 21521006 The Balance HDOJ 1709八、并查集1001 How Many Tables HDOJ 1213 1002 小希的迷宫HDOJ 1272 1003 Is It A Tree? HDOJ 1325 1004 More is better HDOJ 1856 1005 Constructing Roads HDOJ 1102 1006 畅通工程HDOJ 1232 1007 还是畅通工程HDOJ 1233 1008 畅通工程HDOJ 1863 1009 畅通工程再续HDOJ 1875 1010 继续畅通工程HDOJ 1879共26 + 24 + 20 + 14 + 10 + 6 + 10 = 110 题。
代码
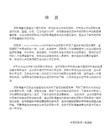
.learn 22993 暗影烈焰
.learn 40029 恶魔之火
.learn 40496 混乱重击
.learn 40497 混乱冲锋
.learn 40592 战争震击
.learn 41126 火焰爆裂
.learn 40936 战争践踏
.learn 23965 立即治疗
.modify speed # 将目标移动速度设定为初始速度的#倍(#=0.1-10)
.modify bwalk # 将目标移后退度设定为初始速度的#倍(#=0.1-10)
.modify swim # 将目标游泳速度设定为初始速度的#倍(#=0.1-10)
.modify fly # 将目标飞行速度设定为初始速度的#倍(#=0.1-10)
.character learn 42005 血沸 每秒600(24s)
.character learn 23537 激怒 提升物理伤害能力
.character learn 41431 符文护盾 吸收50000伤害,提高施法和攻击速度100%,免疫法术打断
.modify spell # 修改spell
.learn命令:
.learn # 学习spell id为#的技能
.unlearn # 忘记spell id为#的技能
.learn all 学习所有GM默认的魔法
.learn all_lang 学习所有语言
.learn all_myclass 学习自己职业的全部可用魔法(仅限自己)
.character learn all_lang //学习所有语言
.character advancesk [技能代码] 375 技能熟练
用Python计算最长公共子序列和最长公共子串(转)
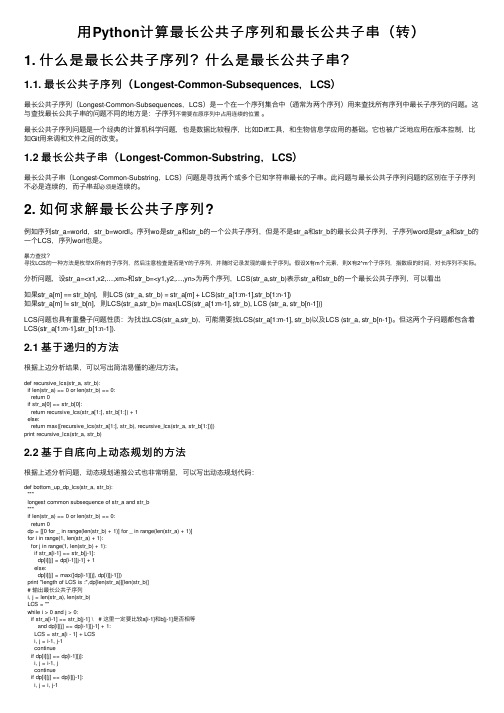
⽤Python计算最长公共⼦序列和最长公共⼦串(转)1. 什么是最长公共⼦序列?什么是最长公共⼦串?1.1. 最长公共⼦序列(Longest-Common-Subsequences,LCS)最长公共⼦序列(Longest-Common-Subsequences,LCS)是⼀个在⼀个序列集合中(通常为两个序列)⽤来查找所有序列中最长⼦序列的问题。
这与查找最长公共⼦串的问题不同的地⽅是:⼦序列不需要在原序列中占⽤连续的位置。
最长公共⼦序列问题是⼀个经典的计算机科学问题,也是数据⽐较程序,⽐如Diff⼯具,和⽣物信息学应⽤的基础。
它也被⼴泛地应⽤在版本控制,⽐如Git⽤来调和⽂件之间的改变。
1.2 最长公共⼦串(Longest-Common-Substring,LCS)最长公共⼦串(Longest-Common-Substring,LCS)问题是寻找两个或多个已知字符串最长的⼦串。
此问题与最长公共⼦序列问题的区别在于⼦序列不必是连续的,⽽⼦串却必须是连续的。
2. 如何求解最长公共⼦序列?例如序列str_a=world,str_b=wordl。
序列wo是str_a和str_b的⼀个公共⼦序列,但是不是str_a和str_b的最长公共⼦序列,⼦序列word是str_a和str_b的⼀个LCS,序列worl也是。
暴⼒查找?寻找LCS的⼀种⽅法是枚举X所有的⼦序列,然后注意检查是否是Y的⼦序列,并随时记录发现的最长⼦序列。
假设X有m个元素,则X有2^m个⼦序列,指数级的时间,对长序列不实际。
分析问题,设str_a=<x1,x2,…,xm>和str_b=<y1,y2,…,yn>为两个序列,LCS(str_a,str_b)表⽰str_a和str_b的⼀个最长公共⼦序列,可以看出如果str_a[m] == str_b[n],则LCS (str_a, str_b) = str_a[m] + LCS(str_a[1:m-1],str_b[1:n-1])如果str_a[m] != str_b[n],则LCS(str_a,str_b)= max{LCS(str_a[1:m-1], str_b), LCS (str_a, str_b[n-1])}LCS问题也具有重叠⼦问题性质:为找出LCS(str_a,str_b),可能需要找LCS(str_a[1:m-1], str_b)以及LCS (str_a, str_b[n-1])。
acm 程序设计 ppt课件

{ f(i,j)=
f(i-1,j-1)+1 (a[i]==b[j]) max(f(i-1,j),f(i,j-1)) (a[i]!=b[j])
由于f(i,j)只和f(i-1,j-1), f(i-1,j)和f(i,j-1)有 关, 而在计算f(i,j)时, 只要选择一个合适 的顺序, 就可以保证这三项都已经计算出 来了, 这样就可以计算出f(i,j). 这样一直 推到f(len(a),len(b))就得到所要求的解了.
29 2020/5/11
二、动态规划的基本步骤
动态规划算法通常用于求解具有某 种最优性质的问题。在这类问题中,可 能会有许多可行解。每一个解都对应于 一个值,我们希望找到具有最优值(最 大值或最小值)的那个解。设计一个动 态规划算法,通常可以按以下几个步骤 进行:
30 2020/5/11
(1)找出最优解的性质,并刻画其结构特征。 (2)递归地定义最优值。 (3)以自底向上的方式计算出最优值。 (4)根据计算最优值时得到的信息,构造一个
f[i] = max(f[i], f[j]+1) (1<=j<i,且Mice[i].W> Mice[j].W,Mice[i].S < Mice[j].S)
其中,初始条件为f[i]=1 (i=1, 2, ..., n)。
20 2020/5/11
Qestion:
两个问题有本 质区别吗?
21 2020/5/11
为(n-2)*2=4,而另外两条直线既可能平行也可能相交,因此可能交点
数为:
(n-2)*2+0=4 或者
(n-2)*2+1=5
4、 第四条直线不与任何一条直线平行,交点数为:
(n-3)*3+0=3 或者 (n-3)*3+2=5 或者 (n-3)*3+3=6
TIME-WARPED LONGEST COMMON SUBSEQUENCE ALGORITHM FOR MUSIC RETRIEVAL
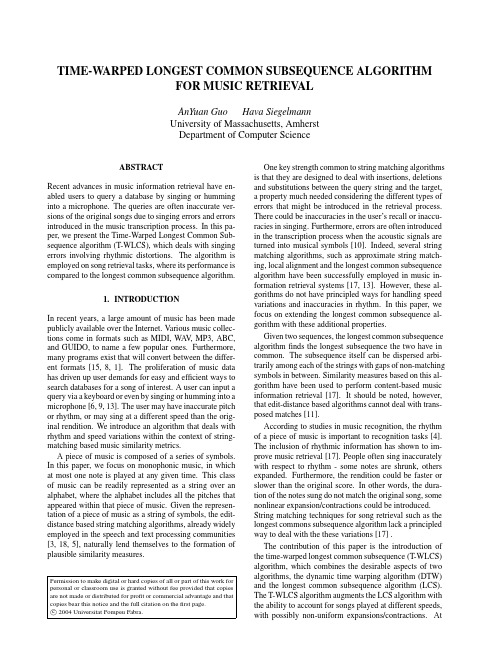
ABSTRACT Recent advances in music information retrieval have enabled users to query a database by singing or humming into a microphone. The queries are often inaccurate versions of the original songs due to singing errors and errors introduced in the music transcription process. In this paper, we present the Time-Warped Longest Common Subsequence algorithm (T-WLCS), which deals with singing errors involving rhythmic distorБайду номын сангаасions. The algorithm is employed on song retrieval tasks, where its performance is compared to the longest common subsequence algorithm. 1. INTRODUCTION In recent years, a large amount of music has been made publicly available over the Internet. Various music collections come in formats such as MIDI, WAV, MP3, ABC, and GUIDO, to name a few popular ones. Furthermore, many programs exist that will convert between the different formats [15, 8, 1]. The proliferation of music data has driven up user demands for easy and efficient ways to search databases for a song of interest. A user can input a query via a keyboard or even by singing or humming into a microphone [6, 9, 13]. The user may have inaccurate pitch or rhythm, or may sing at a different speed than the original rendition. We introduce an algorithm that deals with rhythm and speed variations within the context of stringmatching based music similarity metrics. A piece of music is composed of a series of symbols. In this paper, we focus on monophonic music, in which at most one note is played at any given time. This class of music can be readily represented as a string over an alphabet, where the alphabet includes all the pitches that appeared within that piece of music. Given the representation of a piece of music as a string of symbols, the editdistance based string matching algorithms, already widely employed in the speech and text processing communities [3, 18, 5], naturally lend themselves to the formation of plausible similarity measures.
a11-chap15(4)-dp 算法导论 教学课件

Given two sequences X= <x1, x2, ..., xm> and Y= <y1, y2, ..., yn>, how to find a maximum-length common subsequence of X and Y .
(设zk≠xm, 令Z’= <z1 , ... , zk , xm>,则 Z’ 是 X 和 Y 的相同子序列,且 length(Z’)=k+1 ⇒ Z’ 是比 Z 更长的子序列 ⇒ 与题设 Z 是LCS矛盾)
Now show Zk-1 is an LCS of Xm-1 and Yn-1. Clearly, it’s a CS. Now suppose there exists a CS W of Xm-1 and Yn-1 that’s longer than Zk-1 ⇒length(W) ≥ k. Make subsequence W’ by appending xm to W. W’ is CS of X and Y , length(W’)≥k+1 ⇒ contradicts Z being an LCS.
举出 X 的所有子序列,逐项核查这些子序列是否为 Y 的子序列)
Each subsequence of X corresponds to a subset of the
indices {1, 2, ..., m} of X. There are 2m subsequences of
X . Exponential time, impractical for long sequences.
湘潭大学ACM-ICPC新人训练导引

0 POJ 1306 Combinations
3 HDU 2074 叠筐
0 POJ 1503 Integer Inquiry
3 HDU 2516 取石子游戏
0 POJ 1936 All in All
3 HDU 2540 遮挡判断
0 POJ 1979 Red and Black
3 HDU 3809 Decrypt coordinate
期越来越快,一天刷 20-30 道水题完全无难度的。主要不要一味的刷水题过瘾,需要结合学
习的知识点,有针对性地做题,这样才能保证上水平。低水平重复是没有什么意义的。
3. 三人行必有我师。善于与周围的队员交流,乐于分享自己的学习感悟。团队的进步 和个人的进步是相互促进的。善于利用网络资源,比如 QQ 群等,和全国的 ACMer 进行交
7. 建议有一定基础的同学在网上多多参加比赛,比如 USACO,TopCoder,Codeforce,
HDOJ,ZJU,FZU 等,经常有比赛的。国内流行的做题网站如下表。
名称
地址
备注
USACO Training
/usacogate
OI 风格,进阶练习模式
USACO Contest
五、 知识点及推荐习题
考虑到时间关系,暂时推荐大家从刘汝佳的《算法竞赛入门经典》入手,掌握基本的知
识点。预计到下个学期前半段,大家能掌握这本书的大部分内容就已经很不错的。这个学期
马上进入考试阶段,暂时不会有集训或者讲座的活动。
作业题列表及 OJ 地址 难度 题目
难度 题目
0 HDU 2030 字符统计
34th ACM-ICPC 亚洲分区赛-上海 34th ACM-ICPC 亚洲分区赛-哈尔滨
Longest common subsequence
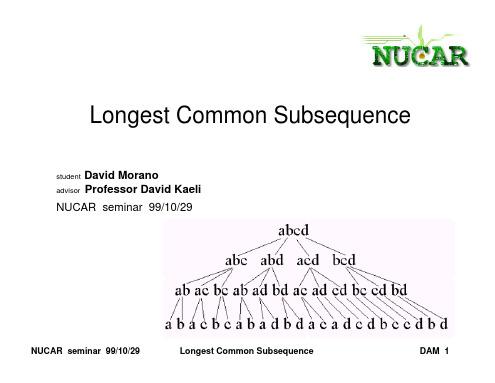
NUCAR seminar 99/10/29
Longest Common Subsequence
DAM 5
CPLEX Solver
• difficult to express in AMPL • no easy way to parametize the AMPL model • exceedingly long execution times for even simple problem instances ! • all but useless for real problem solving needs ! • is its running time just a : – constant times the exponential bound – a polynominal function times the bound – or the product of two or more expontentials ?
NUCAR seminar 99/10/29
Longest Common Subsequence
DAM 10
Exhaustive Algorithms
t Exhaustive #5
• expands out the shortest input string according to a binary counting order (counting down from all ones) • characters set in the counter are considered, characters not set are ignored • to speed it up, no possible subsequence is considered that is shorter than the incumbent LCS found so far
ACM题目分类
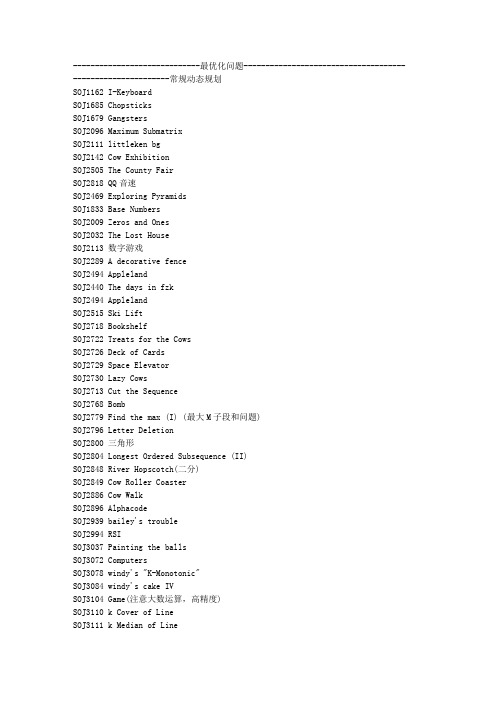
-----------------------------最优化问题------------------------------------- ----------------------常规动态规划SOJ1162 I-KeyboardSOJ1685 ChopsticksSOJ1679 GangstersSOJ2096 Maximum SubmatrixSOJ2111 littleken bgSOJ2142 Cow ExhibitionSOJ2505 The County FairSOJ2818 QQ音速SOJ2469 Exploring PyramidsSOJ1833 Base NumbersSOJ2009 Zeros and OnesSOJ2032 The Lost HouseSOJ2113 数字游戏SOJ2289 A decorative fenceSOJ2494 ApplelandSOJ2440 The days in fzkSOJ2494 ApplelandSOJ2515 Ski LiftSOJ2718 BookshelfSOJ2722 Treats for the CowsSOJ2726 Deck of CardsSOJ2729 Space ElevatorSOJ2730 Lazy CowsSOJ2713 Cut the SequenceSOJ2768 BombSOJ2779 Find the max (I) (最大M子段和问题)SOJ2796 Letter DeletionSOJ2800 三角形SOJ2804 Longest Ordered Subsequence (II)SOJ2848 River Hopscotch(二分)SOJ2849 Cow Roller CoasterSOJ2886 Cow WalkSOJ2896 AlphacodeSOJ2939 bailey's troubleSOJ2994 RSISOJ3037 Painting the ballsSOJ3072 ComputersSOJ3078 windy's "K-Monotonic"SOJ3084 windy's cake IVSOJ3104 Game(注意大数运算,高精度)SOJ3110 k Cover of LineSOJ3111 k Median of LineSOJ3123 Telephone WireSOJ3142 Unfriendly Multi Permutation SOJ3213 PebblesSOJ3219 Cover UpSOJ3263 FunctionSOJ3264 Evil GameSOJ3339 graze2SOJ3341 SkiSOJ3352 The Baric BovineSOJ3503 Banana BoxesSOJ3633 Matches's GameSOJ3636 理想的正方形SOJ3711 Mountain RoadSOJ3723 Robotic Invasionnankai1134 Relation Orderingsrm150--div1--500----------------背包问题SOJ2222 Health PowerSOJ2749 The Fewest CoinsSOJ2785 Binary PartitionsSOJ2930 积木城堡SOJ3172 FishermanSOJ3300 Stockholm CoinsSOJ3360 Buying HaySOJ3531 Number Pyramids----------------状态DPSOJ2089 lykooSOJ2768 BombSOJ2819 AderSOJ2842 The TSP problemSOJ3025 Artillery(状态DP)SOJ3088 windy's cake VIIISOJ3183 Fgjlwj's boxesSOJ3259 Counting numbersSOJ3262 Square Fields(二分+状态DP) SOJ3371 Mixed Up CowsSOJ3631 Shopping Offers----------------树状DPSOJ 1870 Rebuilding RoadsSOJ 2136 Apple(树形依赖背包n*C算法)SOJ 2514 Milk Team SelectSOJ 2199 Apple TreeSOJ 3295 Treeland ExhibitionSOJ 3635 World Cup 2010hdoj1561 The more, The BetterPKU1655 Balancing ActPKU3107 GodfatherPKU3345 Bribing FIPAPKU2378 Tree CuttingPKU3140 Contestants DivisionPKU3659 Cell Phone Network---------------配合数据结构的优化DPSOJ 2702 AlannaSOJ 2978 TasksSOJ 3234 Finding SeatsSOJ 3540 股票交易-------------- 斜率优化SOJ 3710 特别行动队SOJ 3734 搬家SOJ 3736 Lawrence of Arabia---------------四边形不等式SOJ 1702 Cutting SticksSOJ 2775 Breaking Strings--------------- 最优化之排序(思考两个元素之间的先后关系,以此得出一个二元比较关系,并验证此关系可传递,反对称,进而排序)SOJ2509 The Milk QueueSOJ2547 cardsSOJ2850 Protecting the FlowersSOJ2957 Setting ProblemsSOJ3167 ComputerSOJ3331 Cards(2547加强版)SOJ3327 Dahema's Computer(通过此题学会排序)-----------------最优化之必要条件枚举(思考最优解所具有的性质,得出最优解的一个强必要条件,在此基础上枚举)SOJ3317 FGJ's PlaneSOJ3429 Food portion sizes--------------------------------贪心---------------------------------------SOJ1078 BlueEyes' ScheduleSOJ1203 Pass-MurailleSOJ1673 Gone FishingSOJ2574 pieSOJ2645 Buy One Get One FreeSOJ2701 In a CycleSOJ2876 Antimonotonicity(经典模型 O(n)算法)SOJ3343 Tower--------------------------------搜索--------------------------------------- SOJ1106 DWeepSOJ1626 squareSOJ2061 8 puzzleSOJ2485 SudokuSOJ1045 SticksSOJ2736 FliptileSOJ2771 Collecting StonesSOJ2715 Maze BreakSOJ2518 Magic Cow ShoesSOJ2829 binary strings(双向BFS)SOJ3005 Dropping the stonesSOJ3136 scu07t01的迷宫(BFS预处理然后枚举交汇点)SOJ3330 Windy's Matrix(BFS)--------------------------------DFA---------------------------------------- ---------------状态矩阵SOJ1826 Number SequenceSOJ1936 FirepersonsSOJ2552 Number of TilingsSOJ2919 Matrix Power Series (学习矩阵的快速乘法从此开始)SOJ2920 Magic BeanSOJ3021 Quad TilingSOJ3046 Odd Loving BakersSOJ3176 E-stringSOJ3246 Tiling a Grid With DominoesSOJ3323 K-Satisfied NumbersSOJ3337 Wqb's Word----------------DFA+DPSOJ1112 Repeatless Numbers(DFA+二分)SOJ2913 Number SubstringSOJ2826 Apocalypse SomedaySOJ3128 windy和水星 -- 水星数学家 1SOJ3182 Windy numbers---------------------------------图论-----------------------------------------------------------最短路SOJ1697 Cashier EmploymentSOJ2325 Word TransformationSOJ2427 Daizi's path systemSOJ2468 CatcusSOJ2751 Wormholes(SPFA判断负圈回路的存在性)SOJ2932 道路SOJ3160 Clear And Present DangerSOJ3335 Windy's Route(最短路径的分层图思想)SOJ3346 Best Spot(N^3放心的写)SOJ3423 Revamping Trails---------------------查分约束SOJ1687 Intervals---------------------最小生成树SOJ1169 NetworkingSOJ2198 HighwaysSOJ3366 Watering HoleSOJ3427 Dark roads---------------------强连通分支SOJ2832 Mars city---------------------2-SATSOJ3535 Colorful DecorationHDU3062 Party---------------------拓扑排序SOJ1075 BlueEyes and Apples (II)---------------------无向连通图上的割点和割边问题SOJ1935 ElectricityWHU145 Railway---------------------二分图的匹配------------------最大匹配SOJ1183 Girls and BoysSOJ1186 CoursesSOJ2035 The Tiling ProblemSOJ2077 Machine ScheduleSOJ2160 Optimal MilkingSOJ2342 Rectangles(Beloved Sons 模型)SOJ2472 Guardian of DecencySOJ2681 平方数 2SOJ2737 AsteroidsSOJ2764 Link-up GameSOJ2806 LED DisplaySOJ2958 Weird FenceSOJ3043 Minimum CostSOJ3038 Beloved Sons(简单贪心一下)SOJ3453 Stock ChartsZOJ3265 Strange Game---------------最佳匹配SOJ1981 Going HomeWHU1451 Special Fish---------------------最近公共祖先问题SOJ1187 Closest Common AncestorsSOJ1677 How far awaySOJ3023 NetworkSOJ3098 Bond---------------------其他SOJ3013 treeSOJ3056 Average distance(树上的DFS)---------------------------------网络流------------------------------------- ---------------------最大流POJ 1273 Drainage DitchesPOJ 1274 The Perfect Stall (二分图匹配)POJ 1698 Alice's ChancePOJ 1459 Power NetworkPOJ 2112 Optimal Milking (二分)POJ 2455 Secret Milking Machine (二分)POJ 3189 Steady Cow Assignment (枚举)POJ 1637 Sightseeing tour (混合图欧拉回路)POJ 3498 March of the Penguins (枚举汇点)POJ 1087 A Plug for UNIXPOJ 1149 Pigs (构图题)ZOJ 2760 How Many Shortest Path (边不相交最短路的条数)POJ 2391 Ombrophobic Bovines (必须拆点,否则有BUG)WHU 1124 Football Coach (构图题)SGU 326 Perspective (构图题,类似于 WHU 1124)UVa 563 CrimewaveUVa 820 Internet BandwidthPOJ 3281 Dining (构图题)POJ 3436 ACM Computer FactoryPOJ 2289 Jamie's Contact Groups (二分)SGU 438 The Glorious Karlutka River =) (按时间拆点)SGU 242 Student's Morning (输出一组解)SGU 185 Two shortest (Dijkstra 预处理,两次增广,必须用邻接阵实现,否则 MLE) HOJ 2816 Power LinePOJ 2699 The Maximum Number of Strong Kings (枚举+构图)ZOJ 2332 GemsJOJ 2453 Candy (构图题)SOJ 2414 Leapin' LizardsSOJ 2835 Pick Up PointsSOJ 3312 Stockholm KnightsSOJ 3353 Total Flow--------------------最小割SOJ2662 PlaygroundSOJ3106 Dual Core CPUSOJ3109 Space flightSOJ3107 SelectSOJ3185 Black and whiteSOJ3254 Rain and FgjSOJ3134 windy和水星 -- 水星交通HOJ 2634 How to earn moreZOJ 2071 Technology Trader (找割边)HNU 10940 CoconutsZOJ 2532 Internship (找关键割边)POJ 1815 Friendship (字典序最小的点割集)POJ 3204 Ikki's Story I - Road Reconstruction (找关键割边)POJ 3308 ParatroopersPOJ 3084 Panic RoomPOJ 3469 Dual Core CPUZOJ 2587 Unique Attack (最小割的唯一性判定)POJ 2125 Destroying The Graph (找割边)ZOJ 2539 Energy MinimizationZOJ 2930 The Worst ScheduleTJU 2944 Mussy Paper (最大权闭合子图)POJ 1966 Cable TV Network (无向图点连通度)HDU 1565 方格取数(1) (最大点权独立集)HDU 1569 方格取数(2) (最大点权独立集)HDU 3046 Pleasant sheep and big big wolfPOJ 2987 Firing (最大权闭合子图)SPOJ 839 Optimal Marks (将异或操作转化为对每一位求最小割)HOJ 2811 Earthquake Damage (最小点割集)2008 Beijing Regional Contest Problem A Destroying the bus stations ( BFS 预处理 )(http://acmicpc-live-archive.uva.es/nuevoportal/data/problem.php?p=4322)ZOJ 2676 Network Wars (参数搜索)POJ 3155 Hard Life (参数搜索)ZOJ 3241 Being a Hero-----------------有上下界ZOJ 2314 Reactor Cooling (无源汇可行流)POJ 2396 Budget (有源汇可行流)SGU 176 Flow Construction (有源汇最小流)ZOJ 3229 Shoot the Bullet (有源汇最大流)HDU 3157 Crazy Circuits (有源汇最小流)-----------------最小费用流HOJ 2715 Matrix3HOJ 2739 The Chinese Postman ProblemPOJ 2175 Evacuation Plan (消一次负圈)POJ 3422 Kaka's Matrix Travels (与 Matrix3 类似)POJ 2516 Minimum Cost (按物品种类多次建图)POJ 2195 Going HomePOJ 3762 The Bonus Salary!BUAA 1032 Destroying a PaintingPOJ 2400 Supervisor, Supervisee (输出所有最小权匹配)POJ 3680 IntervalsHOJ 2543 Stone IVPOJ 2135 Farm TourSOJ 3186 SegmentsSOJ 2927 终极情报网SOJ 3634 星际竞速HDU 3376 Matrix Again-----------------------------------数据结构--------------------------------- -----------------------------------基础数据结构----------------------栈SOJ2511 MooooSOJ3085 windy's cake V(经典栈与单调性的结合)SOJ3279 hm 与 zx 的故事系列2SOJ3329 Maximum Submatrix II(转化为上面两题的模型)---------------------双端队列SOJ2978 TasksSOJ3139 Sliding Window(双端队列最经典的应用)SOJ3636 理想的正方形-------------------- --------------高级数据结构---------------------线段树SOJ1862 Choice PearsSOJ2057 The manager's worrySOJ2249 Mayor's postersSOJ2309 In the Army NowSOJ2436 Picture puzzle gameSOJ2556 Find the PermutationSOJ2562 The End of CorruptionSOJ2719 Corral the Cows(线段树+二分)SOJ2740 Balanced LineupSOJ2745 零序列SOJ2776 Matrix SearchingSOJ2808 Thermal Death of the UniverseSOJ2822 Buy TicketsSOJ2937 TetrisSOJ2938 Apple Tree(先DFS获得欧拉序列)SOJ2965 capitally playersSOJ2968 Matrix(二维线段树)SOJ3019 Count ColorSOJ3022 Difference Is Beautiful( RMQ+二分经典模型)SOJ3086 windy's cake VI(二维线段树)SOJ3099 A Simple Problem with IntegersSOJ3248 MousetrapSOJ3321 Windy's Sequence IISOJ3370 Light SwitchingSOJ3640 Special Subsequence---------------------树状数组SOJ2309 In the Army Now---------------------归并排序思想SOJ2906 Ultra-QuickSortSOJ2431 Cows distribute food(利用归并排序求逆序数:nlogn) SOJ2497 Number sequenceSOJ2559 What is the Rank?SOJ2728 MooFestSOJ3009 Stones for AmySOJ3010 K-th NumberSOJ3147 K-th number---------------------并查集SOJ1824 The SuspectsSOJ1953 keySOJ2245 Ubiquitous ReligionsSOJ2389 Journey to TibetSOJ2438 PetSOJ2490 Math teacher's testPOJ2832 How many pairs?POJ2821 Auto-Calculation MachineSOJ2979 食物链SOJ3282 Kingdom of HeavenSOJ3417 Skyscrapers------------------------块状链表SOJ3032 Big StringSOJ3035 反转序列----------------------------------- 字符串---------------------后缀数组SOJ1948 sekretarkaSOJ3045 Long Long MessageSOJ3075 回文子串SOJ3296 Windy's S---------------------KMPSOJ2652 OulipoSOJ2307 String MatchingSOJ3014 Seek the Name, Seek the FameSOJ3596 Article Decryption--------------------trie树SOJ3076 相同字符串SOJ3336 DiarySOJ3596 Article Decryption---------------------------------组合数学及数论----------------------------- SOJ1839 Relatives(Euler函数)SOJ1942 FotoSOJ2714 Mountains (II)SOJ2668 C(n,k)SOJ2666 分解 n!SOJ2106 GCD & LCM InverseSOJ2498 Count primeSOJ2238 Let it Bead(置换群-polya定理的应用)SOJ2924 完美交换(置换群)SOJ2638 Cow Sorting(置换群)-------------费马小定理SOJ 3578 H1N1's Problem--------------------------容斥原理SOJ3191 Free squareSOJ3082 windy's cake IISOJ3502 The Almost Lucky NumbersSOJ3547 Coprime----------------------------------博弈论------------------------------------SOJ1128 控制棋SOJ1866 Games(诡异的博弈)SOJ2197 A Funny GameSOJ2188 A multiplication gameSOJ2403 Black and white chessSOJ2477 Simple GameSOJ2687 草稿纸 2SOJ2688 草稿纸 3SOJ2836 Pick Up Points IISOJ2845 JangeSOJ2922 A New Tetris GameSOJ2993 NimSOJ3066 JohnSOJ3132 windy和水星 -- 水星游戏 1SOJ3133 windy和水星 -- 水星游戏 2SOJ3174 Good gameSOJ3307 Stockholm GameSOJ3446 Nim or not NimSOJ3461 Nim-kSOJ3463 Ordered NimSOJ3468 Flip CoinsSOJ3548 gameSOJ3584 Baihacker and Oml-----------------------------------计算几何---------------------------------SOJ1138 WallSOJ1102 Picnic。
TD信息元素详解
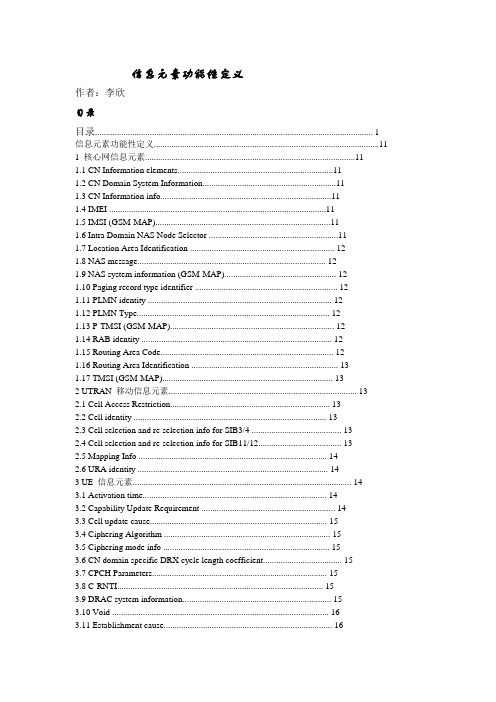
信息元素功能性定义作者:李欣目录目录 (1)信息元素功能性定义 (11)1 核心网信息元素 (11)1.1 CN Information elements (11)1.2 CN Domain System Information (11)1.3 CN Information info (11)1.4 IMEI (11)1.5 IMSI (GSM-MAP) (11)1.6 Intra Domain NAS Node Selector (11)1.7 Location Area Identification (12)1.8 NAS message (12)1.9 NAS system information (GSM-MAP) (12)1.10 Paging record type identifier (12)1.11 PLMN identity (12)1.12 PLMN Type (12)1.13 P-TMSI (GSM-MAP) (12)1.14 RAB identity (12)1.15 Routing Area Code (12)1.16 Routing Area Identification (13)1.17 TMSI (GSM-MAP) (13)2 UTRAN 移动信息元素 (13)2.1 Cell Access Restriction (13)2.2 Cell identity (13)2.3 Cell selection and re-selection info for SIB3/4 (13)2.4 Cell selection and re-selection info for SIB11/12 (13)2.5 Mapping Info (14)2.6 URA identity (14)3 UE 信息元素 (14)3.1 Activation time (14)3.2 Capability Update Requirement (14)3.3 Cell update cause (15)3.4 Ciphering Algorithm (15)3.5 Ciphering mode info (15)3.6 CN domain specific DRX cycle length coefficient (15)3.7 CPCH Parameters (15)3.8 C-RNTI (15)3.9 DRAC system information (15)3.10 Void (16)3.11 Establishment cause (16)3.12 Expiration Time Factor (16)3.13 Failure cause (16)3.14 Failure cause and error information (16)3.15 Initial UE identity (16)3.16 Integrity check info (16)3.17 Integrity protection activation info (17)3.18 Integrity protection Algorithm (17)3.19 Integrity protection mode info (17)3.20 Maximum bit rate (17)3.21 Measurement capability (17)3.22 Paging cause (17)3.23 Paging record (17)3.24 PDCP capability (17)3.25 Physical channel capability (18)3.26 Protocol error cause (18)3.27 Protocol error indicator (18)3.28 RB timer indicator (18)3.29 Redirection info (18)3.30 Re-establishment timer (18)3.31 Rejection cause (18)3.32 Release cause (18)3.33 RF capability FDD (19)3.34 RLC capability (19)3.35 RLC re-establish indicator (19)3.36 RRC transaction identifier (19)3.37 Security capability (19)3.38 START (19)3.39 Transmission probability (19)3.40 Transport channel capability (20)3.41 UE multi-mode/multi-RAT capability (20)3.42 UE radio access capability (20)3.43 UE Timers and Constants in connected mode (21)3.44 UE Timers and Constants in idle mode (21)3.45 UE positioning capability (21)3.46 URA update cause (21)3.47 U-RNTI (21)3.48 U-RNTI Short (21)3.49 UTRAN DRX cycle length coefficient (21)3.50 Wait time (21)3.51 UE Specific Behavior Information 1 idle (21)3.52 UE Specific Behavior Information 1 interRAT (22)4 无线承载信息元素 (22)4.0 Default configuration identity (22)4.1 Downlink RLC STATUS info (22)4.2 PDCP info (22)4.3 PDCP SN info (22)4.4 Polling info (22)4.5 Predefined configuration identity (23)4.6 Predefined configuration value tag (23)4.7 Predefined RB configuration (23)4.8 RAB info (23)4.9 RAB info Post (23)4.10 RAB information for setup (23)4.11 RAB information to reconfigure (24)4.12 NAS Synchronization indicator (24)4.13 RB activation time info (24)4.14 RB COUNT-C MSB information (24)4.15 RB COUNT-C information (24)4.16 RB identity (24)4.17 RB information to be affected (24)4.18 RB information to reconfigure (25)4.19 RB information to release (25)4.20 RB information to setup (25)4.21 RB mapping info (25)4.22 RB with PDCP information (25)4.23 RLC info (25)4.24 Signaling RB information to setup (26)4.25 Transmission RLC Discard (26)5 传输信道信息元素 (26)5.1 Added or Reconfigured DL TrCH information (26)5.2 Added or Reconfigured UL TrCH information (27)5.3 CPCH set ID (27)5.4 Deleted DL TrCH information (27)5.5 Deleted UL TrCH information (27)5.6 DL Transport channel information common for all transport channels (27)5.7 DRAC Static Information (27)5.8 Power Offset Information (28)5.9 Predefined TrCH configuration (28)5.10 Quality Target (28)5.11 Semi-static Transport Format Information (28)5.12 TFCI Field 2 Information (28)5.13 TFCS Explicit Configuration (28)5.14 TFCS Information for DSCH (TFCI range method) (29)5.15 TFCS Reconfiguration/Addition Information (29)5.16 TFCS Removal Information (29)5.17 Void (29)5.18 Transport channel identity (29)5.19 Transport Format Combination (TFC) (29)5.20 Transport Format Combination Set (29)5.21 Transport Format Combination Set Identity (29)5.22 Transport Format Combination Subset (29)5.23 Transport Format Set (29)5.24 UL Transport channel information common for all transport channels (30)6 物理信道信息元素 (30)6.1 AC-to-ASC mapping (30)6.2 AICH Info (30)6.3 AICH Power offset (30)6.4 Allocation period info (30)6.5 Alpha (30)6.6 ASC Setting (30)6.7 Void (31)6.8 CCTrCH power control info (31)6.9 Cell parameters Id (31)6.10 Common timeslot info (31)6.11 Constant value (31)6.12 CPCH persistence levels (31)6.13 CPCH set info (31)6.14 CPCH Status Indication mode (31)6.15 CSICH Power offset (32)6.16 Default DPCH Offset Value (32)6.17 Downlink channelisation codes (32)6.18 Downlink DPCH info common for all RL (32)6.19 Downlink DPCH info common for all RL Post (32)6.20 Downlink DPCH info common for all RL Pre (32)6.21 Downlink DPCH info for each RL (32)6.22 Downlink DPCH info for each RL Post (33)6.23 Downlink DPCH power control information (33)6.24 Downlink information common for all radio links (33)6.25 Downlink information common for all radio links Post (33)6.26 Downlink information common for all radio links Pre (33)6.27 Downlink information for each radio link (33)6.28 Downlink information for each radio link Post (33)6.29 Void (33)6.30 Downlink PDSCH information (33)6.31 Downlink rate matching restriction information (34)6.32 Downlink Timeslots and Codes (34)6.33 DPCH compressed mode info (34)6.34 DPCH Compressed Mode Status Info (34)6.35 Dynamic persistence level (34)6.36 Frequency info (34)6.37 Individual timeslot info (35)6.38 Individual Timeslot interference (35)6.39 Maximum allowed UL TX power (35)6.40 Void (35)6.41 Midamble shift and burst type (35)6.42 PDSCH Capacity Allocation info (35)6.43 PDSCH code mapping (36)6.44 PDSCH info (36)6.45 PDSCH Power Control info (36)6.46 PDSCH system information (36)6.47 PDSCH with SHO DCH Info (36)6.48 Persistence scaling factors (36)6.49 PICH Info (36)6.50 PICH Power offset (37)6.51 PRACH Channelisation Code List (37)6.52 PRACH info (for RACH) (37)6.53 PRACH partitioning (37)6.54 PRACH power offset (37)6.55 PRACH system information list (37)6.56 Predefined PhyCH configuration (38)6.57 Primary CCPCH info (38)6.58 Primary CCPCH info post (38)6.59 Primary CCPCH TX Power (38)6.60 Primary CPICH info (38)6.61 Primary CPICH Tx power (38)6.62 Primary CPICH usage for channel estimation (38)6.63 PUSCH info (38)6.64 PUSCH Capacity Allocation info (38)6.65 PUSCH power control info (39)6.66 PUSCH system information (39)6.67 RACH transmission parameters (39)6.68 Radio link addition information (39)6.69 Radio link removal information (39)6.70 SCCPCH Information for FACH (39)6.71 Secondary CCPCH info (39)6.72 Secondary CCPCH system information (40)6.73 Secondary CPICH info (40)6.74 Secondary scrambling code (40)6.75 SFN Time info (40)6.76 SSDT cell identity (40)6.77 SSDT information (40)6.78 STTD indicator (40)6.79 TDD open loop power control (41)6.80 TFC Control duration (41)6.81 TFCI Combining Indicator (41)6.82 TGPSI (41)6.83 Time info (41)6.84 Timeslot number (41)6.85 TPC combination index (41)6.86 TSTD indicator (41)6.87 TX Diversity Mode (41)6.88 Uplink DPCH info (41)6.89 Uplink DPCH info Post (42)6.90 Uplink DPCH info Pre (42)6.91 Uplink DPCH power control info (42)6.92 Uplink DPCH power control info Post (42)6.93 Uplink DPCH power control info Pre (42)6.94 Uplink Timeslots and Codes (42)6.95 Uplink Timing Advance (42)6.96 Uplink Timing Advance Control (43)7 测量信息元素 (43)7.1 Additional measurements list (43)7.2 Cell info (43)7.3 Cell measured results (43)7.4 Cell measurement event results (44)7.5 Cell reporting quantities (44)7.6 Cell synchronization information (44)7.7 Event results (44)7.8 FACH measurement occasion info (45)7.9 Filter coefficient (45)7.10 HCS Cell re-selection information (45)7.11 HCS neighboring cell information (45)7.12 HCS Serving cell information (45)7.13 Inter-frequency cell info list (46)7.14 Inter-frequency event identity (46)7.15 Inter-frequency measured results list (46)7.16 Inter-frequency measurement (46)7.17 Inter-frequency measurement event results (47)7.18 Inter-frequency measurement quantity (47)7.19 Inter-frequency measurement reporting criteria (47)7.20 Inter-frequency measurement system information (47)7.21 Inter-frequency reporting quantity (47)7.22 Inter-frequency SET UPDATE (48)7.23 Inter-RAT cell info list (48)7.24 Inter-RAT event identity (48)7.25 Inter-RAT info (48)7.26 Inter-RAT measured results list (48)7.27 Inter-RAT measurement (49)7.28 Inter-RAT measurement event results (49)7.29 Inter-RAT measurement quantity (49)7.30 Inter-RAT measurement reporting criteria (49)7.31 Inter-RAT measurement system information (50)7.32 Inter-RAT reporting quantity (50)7.33 Intra-frequency cell info list (50)7.34 Intra-frequency event identity (50)7.35 Intra-frequency measured results list (50)7.36 Intra-frequency measurement (50)7.37 Intra-frequency measurement event results (51)7.38 Intra-frequency measurement quantity (51)7.39 Intra-frequency measurement reporting criteria (51)7.40 Intra-frequency measurement system information (51)7.41 Intra-frequency reporting quantity (52)7.42 Intra-frequency reporting quantity for RACH reporting (52)7.43 Maximum number of reported cells on RACH (52)7.44 Measured results (52)7.45 Measured results on RACH (52)7.46 Measurement Command (52)7.47 Measurement control system information (53)7.48 Measurement Identity (53)7.49 Measurement reporting mode (53)7.50 Measurement Type (53)7.51 Measurement validity (53)7.52 Observed time difference to GSM cell (53)7.53 Periodical reporting criteria (53)7.54 Primary CCPCH RSCP info (54)7.55 Quality measured results list (54)7.56 Quality measurement (54)7.57 Quality measurement event results (54)7.58 Quality measurement reporting criteria (54)7.59 Quality reporting quantity (54)7.60 Reference time difference to cell (54)7.61 Reporting Cell Status (55)7.62 Reporting information for state CELL_DCH (55)7.63 SFN-SFN observed time difference (55)7.64 Time to trigger (55)7.65 Timeslot ISCP info (55)7.66 Traffic volume event identity (55)7.67 Traffic volume measured results list (55)7.68 Traffic volume measurement (55)7.69 Traffic volume measurement event results (56)7.70 Traffic volume measurement object (56)7.71 Traffic volume measurement quantity (56)7.72 Traffic volume measurement reporting criteria (56)7.73 Traffic volume measurement system information (56)7.74 Traffic volume reporting quantity (56)7.75 UE internal event identity (56)7.76 UE internal measured results (57)7.77 UE internal measurement (57)7.78 UE internal measurement event results (57)7.79 UE internal measurement quantity (57)7.80 UE internal measurement reporting criteria (57)7.81 Void (58)7.82 UE Internal reporting quantity (58)7.83 UE Rx-Tx time difference type 1 (58)7.84 UE Rx-Tx time difference type 2 (58)7.85 UE Transmitted Power info (58)7.86 UE positioning Ciphering info (58)7.87 UE positioning Error (58)7.88 UE positioning GPS acquisition assistance (59)7.89 UE positioning GPS almanac (59)7.90 UE positioning GPS assistance data (59)7.91 UE positioning GPS DGPS corrections (59)7.92 UE positioning GPS ionospheric model (59)7.93 UE positioning GPS measured results (59)7.94 UE positioning GPS navigation model (60)7.95 UE positioning GPS real-time integrity (60)7.96 UE positioning GPS reference time (60)7.97 UE positioning GPS UTC model (61)7.98 UE positioning IPDL parameters (61)7.99 UE positioning measured results (61)7.100 UE positioning measurement (61)7.101 UE positioning measurement event results (61)7.102 Void (62)7.103 UE positioning OTDOA assistance data for UE-assisted (62)7.104 Void (62)7.105 UE positioning OTDOA measured results (62)7.106 UE positioning OTDOA neighbor cell info (62)7.107 UE positioning OTDOA quality (63)7.108 UE positioning OTDOA reference cell info (63)7.109 UE positioning position estimate info (64)7.110 UE positioning reporting criteria (64)7.111 UE positioning reporting quantity (64)7.112 T ADV info (65)8 其它信息元素 (65)8.1 BCCH modification info (65)8.2 BSIC (65)8.3 CBS DRX Level 1 information (65)8.4 Cell Value tag (65)8.5 Inter-RAT change failure (65)8.6 Inter-RAT handover failure (66)8.7 Inter-RAT UE radio access capability (66)8.8 Void (66)8.9 MIB Value tag (66)8.10 PLMN Value tag (66)8.11 Predefined configuration identity and value tag (66)8.12 Protocol error information (66)8.13 References to other system information blocks (66)8.14 References to other system information blocks and scheduling blocks (67)8.15 Rplmn information (67)8.16 Scheduling information (67)8.17 SEG COUNT (67)8.18 Segment index (67)8.19 SIB data fixed (67)8.20 SIB data variable (67)8.21 SIB type (67)8.22 SIB type SIBs only (67)9 ANSI-41 Information elements (68)10 Multiplicity values and type constraint values (68)信息元素功能性定义消息是由多个信息元素组合而成,信息元素根据其功能的不同划分为:核心网域信息元素、UTRAN 移动信息元素、UE 信息元素、无线承载信息元素、传输信道信息元素、物理信道信息元素和测量信息元素。
ABSTRACT Online Event-driven Subsequence Matching over Financial Data Streams

Online Event-driven Subsequence Matching over Financial Data StreamsHuanmei Wu∗Betty Salzberg†Donghui ZhangCollege of Computer and Information ScienceNortheastern UniversityBoston,MA02215{maggiewu,salzberg,donghui}@ABSTRACTSubsequence similarity matching in time series databases is an im-portant research area for many applications.This paper presents a new approximate approach for automatic online subsequence simi-larity matching over massive data streams.With a simultaneous on-line segmentation and pruning algorithm over the incoming stream, the resulting piecewise linear representation of the data stream fea-tures high sensitivity and accuracy.The similarity definition is based on a permutation followed by a metric distance function, which provides the similarity search withflexibility,sensitivity and scalability.Also,the metric-based indexing methods can be applied for speed-up.To reduce the system burden,the event-driven simi-larity search is performed only when there is a potential event.The query sequence is the most recent subsequence of piecewise data representation of the incoming stream which is automatically gen-erated by the system.The retrieved results can be analyzed in dif-ferent ways according to the requirements of specific applications. This paper discusses an application for future data movement pre-diction based on statistical information.Experiments on real stock data are performed.The correctness of trend predictions is used to evaluate the performance of subsequence similarity matching. 1.INTRODUCTIONMany applications generate data streams and there is an increas-ing need to maintain statistical information online.Stream databases are distinguished from conventional databases in several aspects. Raw data is too large to be stored in a traditional database for efficient data management.Querying on the stream database is difficult in set-oriented data management systems.Because the data is changing constantly,a single-pass search over the stream is mandatory since it is infeasible or impossible to rewind the stream.∗This work is part of CenSSIS,the Center for Subsurface Sens-ing and Imaging Systems,under the Engineering Research Cen-ters Program of the National Science Foundation(Award#EEC-9986821).†This work is partially supported by NSF grant IIS-0073063.Permission to make digital or hard copies of all or part of this work for personal or classroom use is granted without fee provided that copies are not made or distributed for profit or commercial advantage,and that copies bear this notice and the full citation on thefirst page.To copy otherwise,to republish,to post on servers or to redistribute to lists,requires prior specific permission and/or a fee.SIGMOD2004June13-18,2004,Paris,France.Copyright2004ACM1-58113-859-8/04/06...$5.00.The answers of the query usually are approximate and partial an-swers.Examples of stream databases can be found in stock market quotes,sensor data,telecommunication systems,and network man-agement.Subsequence matching in time series databases tries tofind sub-sequences from the large data sequences in the database that are similar to a given query sequence.It is important in data mining and is used for pattern matching,future movement prediction,new pattern identification,rule discovery and computer aided diagno-sis.Stream data are naturally ordered in time.Some streams are ordered in afixed time interval and can be treated as stream time series directly.Some streams come in irregularly and special pro-cedures are needed in order to apply time series techniques.For example,there are thousands of stock transactions every second, which may be carried out at any time and there are different num-bers of transactions at different times.Existing techniques on time series subsequence matching mainly focus on discovering the similarity between an online querying sub-sequence and a traditional database.The queried data are static and are accessed using an index.Research in time series data streams is in its preliminary stage.Only some basic statistical measures such as moving averages and standard derivation have been addressed. There is recent research[17,18,29,38]on similarity matching over data streams.The papers[38]treat pair-wise correlated statistics in an online fashion,focusing on similarity for whole data streams, not on subsequence similarity.The papers[17,18]treat similarity-based continuous pattern queries with prediction,which can be extended to answer nearest k neighbors on a stream time series. Last,[29]uses an index structure for K-NN search on data streams. In contrast,we are investigating application-guided subsequence matching over onlinefinancial data streams and online query sub-sequences.Our database is a dynamic stream database which stores recentfinancial data.It will be automatically updated as new stream data comes in.So our database includes the most recent historical data.The query subsequence is automatically generated based on the current state of the data stream.And our new similarity measure satisfies the special requirements offinancial data analysis. Subsequence similarity offinancial data streams has its unique properties.First,according to Elliott Wave Theory[15],the move-ment of the stock market can be predicted by observing and iden-tifying a repetitive pattern of waves.Based on this wave theory, the online piecewise linear representation of the stream data should be in an up-down-up-down repetitive pattern(the zigzag shape). Keogh et al.[26]summarized four well-known algorithms for time series segmentation.None of them has addressed the zigzag re-quirement.The result of the compression algorithm in[14]is in zigzag shape,but the algorithm does not satisfy the real time re-Figure1:Subsequence similarity with different relative posi-tions:(a)Two subsequences differ in the relative positions of the lower points;(b)Two subsequences differ in the relative positions of the upperpoints.Figure2:Subsequence similarity with time scaling and ampli-tude rescaling.quirements for online stock data analysis because it has a longer delay to identify an extreme point when the extrema ratio is large. It is necessary to have a new online segmentation algorithm that can quickly and accurately identify potentially important points.Sec-ond,the relative position of the upper and lower end points plays an important role in subsequence similarity.Figure1shows two examples of two pairs of subsequences which would be considered similar using existing subsequence similarity measures.But tech-nical analysis offinancial data is also concerned with the relative position of the upper end points as well as the relative position of the lower end points.The two pairs of subsequences in Figure1 would not be considered similar byfinancial data analysts.Third, subsequence similarity should beflexible with regard to time shift-ing and scaling,price shifting and amplitude rescaling(Amplitude is the value difference of two adjacent end points).Financial data technical analysis assumes that the amplitude difference is more important than the time difference.For example,in Figure2,all four subsequences are derived from a sequence with time scaling, or amplitude rescaling,or both.The pairs S1and S2,S3and S4 have the same amplitude changes,but different time changes,and the pairs S1and S3,S2and S4have the same time changes but dif-ferent amplitude changes.According tofinancial analysts,S1and S2,S3and S4are more similar while S1and S3,S2and S4are less similar.A new subsequence similarity definition that allows amplitude rescaling(but with limitations)is required.Our new online event-driven subsequence similarity matching takes into account and gracefully handles the special properties of financial data analysis.We make the following main contributions:1.We propose a3-tier online simultaneous segmentation andpruning algorithm.It takes a rawfinancial data stream asinput and produces a stream of piecewise linear representa-tion end points.The end points are in an upper-lower-upper-lower repetitive pattern(the zigzag shape).This tiered seg-mentation and pruning algorithm provides the piecewise lin-ear representation with high sensitivity and accuracy.Thealgorithm runs in linear time and with constant memory.2.We explore an alternative similarity measure for subsequencematching,where a metric distance function is defined basedon a permutation of the subsequence.The permutation en-sures two subsequences have the same relative positions.Thedistance function controls the extent of amplitude rescaling.The new definition provides subsequence similarity searchwith sensitivity,flexibility and scalability.Any existing metric-based indexing technology can be employed for search speed-up.3.We perform event-driven subsequence similarity matchingover an up-to-date database using the end points of the piece-wise linear representation.The query will be carried out onlywhen there is a new end point.The automatically generatedquery subsequence is the most recent subsequence of the endpoints,which reflects the most recent information of the rawfinancial data stream.A new mechanism that can turn on oroff the search engine is enabled.4.We apply a new definition of trend forfinancial data streamsusing the results of subsequence similarity search to predictfuture data movement.Our definition of trend does not placeany restrictions on the characteristics of the stock streams onwhich it is applied.The market can be a bull market,a bearmarket or a no-trend market.Our event-driven subsequencesimilarity search is more accurate in seizing critical pointsfor a trend period than algorithms which search at all timeinstances.In addition,our approach is30times faster thansearching at all time instances.The rest of the paper is organized as follows.Section2briefly discusses related work on subsequence matching and data stream processing.Section3describes our strategy for data processing over incoming streams.The subsequence similarity matching of the resulting piecewise linear representation is explained in detail in Section4.One application of our similarity search for trend pre-diction is discussed in Section5.Section6presents our experiment results and Section7concludes this paper and provides some future research directions.2.RELATED WORKSimilarity search in time series is useful for many data mining applications.Agrawal et al.[2]hasfirst introduced whole sequence similarity matching.Faloutsos et al.[13]generalized it to subse-quence similarity matching.The basic idea is to transform the se-quence into the frequency domain using a Discrete Fourier Trans-formation(DFT).Then thefirst few features are extracted and theEuclidean distance is used as the similarity distance function.Mul-tidimensional indexing methods such as the R*-tree[5]can be ap-plied for fast search.In subsequence matching,the R*-tree stores only Minimum Bounding Rectangles(MBR).New research based on subsequence search has grown in several aspects.New meth-ods in constructing MBRs reduce false negatives[30].Keogh et al.proposed Piecewise Aggregate Approximation(PAA)to reduce the dimensionality and to support fast sequence matching using R-trees.Other feature extraction functions,such as the Discrete Wavelet Transformation(DWT)[8,20],Adaptive Piecewise Con-stant Approximation(APAC)[24],and Single Value Decomposi-tion(SVD)[28]have been proposed to reduce the dimensionality of time series data.New distance functions such as Dynamic Time Warping[32,35]and Longest Common Subsequences[11]have been explored to overcome the brittleness of the Euclidean distance measure or its variations[2,13,33].Data streams have attracted more research interest recently[1,3, 4,12,17,18,19,21,22,29,31,38].Babu et al.[3]showed how to define and evaluate continuous queries over data streams.Some ba-sic statistics over data streams have been studied.Datar et al.[12] studied single stream statistics using sliding windows.Gehrke et al.[19]studied statistics for correlated aggregates over multiple data streams using histograms.Gao et al.[17,18]introduce a new strategy of continuous queries with prediction on a stream time se-ries.Liu et al.[29]treat KNN search over data streams using index structures.Zhu et al.[38]proposed a new method for statistics over thousands of data streams.Their research focuses on pair-wise cor-relation using a grid-based data structure.Data stream clustering algorithms include STREAM[22,31],Fractal Clustering[4],and CluStream[1].STREAM aims to provide guaranteed performance of data stream clustering and CluStream is developed for clustering large evolving data streams.Stock data analysis has attracted researchers for years.Autore-gressive and moving average are long used techniques[23]for stock market prediction.In thefield of data mining,intensive re-search has been done on the application of neural networks to stock market prediction[27].Stock trends can be also predicted based on the association of trends with news articles[16].Fink and Pratt ap-plied subsequence similarity matching in compressed time series by identifying major extrema[14].The previous work does not concern the real time requirements of onlinefinancial data analy-sis.For instance,the t-test based piecewise segmentation in[16] works on static historical time series in the training phase.The compression algorithm in[14]runs in an online fashion.But it will take longer delay time to identify the previous extremum,which is not practical for stock trading where early detection of a potential end point is critical.Our work differs from previous research in several aspects.The problem addressed here is online subsequence search overfinan-cial data steams and we have addressed the special requirements of financial data technical analysis.Our distance measure for subse-quence similarity is a metric distance function based on a permuta-tion.The subsequence matching process is triggered by new online events.Our database maintains up-to-date information with newly arrived data,not previously obtained data.3.ONLINE DATA STREAM PROCESSING Translating massive data streams into manageable data for the database,which can be queried and indexed upon is an important step for data stream subsequence similarity matching.This sec-tion discusses the data preprocessing steps before similarity search which result in piecewise linear representation of incoming streams. The process of data stream aggregation,segmentation and pruning is explained in more detail below.3.1Aggregation and SmoothingPiecewise linear representation of the data streams requires the data streams to have onefixed value for each time interval.The incoming data streams may arrive at any time.Aggregation over raw data streams is both necessary and important for practical ap-plications.A stream may acquire different aggregate values for dif-ferent purposes.For example,in stock market analysis,the open, high(MAX),low(MIN),close,and volume(SUM)values of one quote over a time interval(minutes,hours,days,months or years) are very important information.Aggregation makes sure there is a unique value for each time instance over afixed time interval.If we draw the data movement with time,we can see a lot of shorter-time random oscillation over a longer-term trend.We need tofilter out the noise before further data processing.We use the standard moving average which is widely used in thefinancial market[6]to smooth the data:MA p(i)=1pij=i−p+1X(j)where X(i)is the value for i=1,2,...,n and n is the number of periods.MA p(i)calculates the p-interval moving average time series which assigns equal weight to every point in the averaging interval.By smoothing through the moving average,shorter-term noise will befiltered out while a clean trend signal is generated. 3.2Piecewise linear representationPiecewise Linear Representation uses line segments to approxi-mate a time series[14,26].Our approach is new because we adopt a tiered online segmentation and pruning strategy.We do not seg-ment over the price stream directly,instead we segment over one financial indicator,Bollinger Band Percent(%b)[6],to be thefirst base input for line segmentation.Then we prune over the end points of the%b line segments based on some criteria of%b.Thefinal line segments over the raw data stream are obtained by pruning on the previous line segments with criteria based on the raw price stream. We will explain in detail why we choose%b to do the segmentation and how the tiered structure provides high sensitivity and accuracy in the online segmentation.3.2.1%b indicatorBollinger Bands[6]are widely usedfinancial indicators which provide relative definitions of high and low values for time series. The bands are curves drawn above and below a moving average by a measure of standard derivation.An example of time series and Bollinger bands is shown in Figure3b.The three curves are de-fined as follows:middle band=p-period moving averageupper band=middle band+2·p-period standard deviation lower band=middle band-2·p-period standard deviation%b,shown in Figure3c,is another popular indicator derived from Bollinger Bands.%b tells us the current state within the bands.The formula for%b is the following:%b=close price−lower bandband band%b is chosen to be thefirst base for linear segmentation because of the following.First,%b has a smoothed moving trend similar to the price movement.If the price moves in an up trend,%b is also in an up trend.And if the price is in a down trend,%b is also in a downFigure 3:Piecewise linear representation (PLR).(a)Raw financial price stream data;(b)Raw stream data with Bollinger Bands;(c)Thecorresponding stream of %b values;(d)PLR of %b without pruning;(e)PLR of %b with pruning only on %b during segmentation;(f)PLR of raw stream data with pruning on %b and raw data during segmentation.trend.The upper and lower end points of %b correspond to the upper and lower end points of the raw price data.Second,%b is a normalized value of the real price.Most %b values are between -1and 2no matter what real price values are.So we can set a uniform segmentation threshold for %b which we could not do over the real price.For example,if the average price of a stock is $1.00,a change of $0.20may be considered as a big movement.But to a stock with an average price about $100.00,$0.20difference can only be considered as noise.Third,%b is very sensitive to the price change.It will manifest the price change accurately without any delay.So segmentation over %b is more suitable than segmentation directly over the raw price data stream.3.2.2SegmentationSegmentation is based on the %b values.For each time instance,there is a corresponding %b value.Segmentation over %b finds optimized upper and lower end points of the piecewise linear rep-resentation for %b.Figure 3d shows the segmentation results of Figure 3c.Our segmentation algorithm is different from others not only because of a different definition of upper and lower end points but also the resulting end points of our segmentation are in the zigzag shape which is not the case in other algorithms.Our segmentation algorithm uses a sliding window with varying size.The sliding window can only contain at most m points,begin-ning after the last identified end point and ending right before the current point,as shown in Figure 4.If there are more than m points between the last end point and the current point,only the last m points are contained in the sliding window.The segmentation tries to find a possible upper or lower point only in the current sliding window.An upper point is defined as follows (the definition of a lower point is symmetric and thus is omitted here):Suppose the current point is P j (X j ,t j ).The upper point P i (X i ,t i )is a point in the current sliding window that satisfies:1.X i =max(X values of current sliding window );2.X i >X j +δ(where δis the given error threshold);3.P i (X i ,t i )is the last one satisfying the above two conditions .Figure 4shows an example of an upper point.Here,P j =P 13is the current point.The previous identified end point is P 3,sotheFigure 4:A sliding window which finds an upper point.Sup-pose that m =10,δ=1.0,P 3is the last identified end point,P 13is the current point.The actual sliding window size m is 8.P 10is a new upper point.sliding window currently contains m =9points starting from P 4.Both P 9and P 10have the maximum value,but only P 10is found as a upper point because it is the last one with the maximal value in the sliding window.Another thing needed to be mentioned here is the delay time ,which is the time difference between the actually time of an end point and the time when it is identifies as an end point.Although the upper point is at t 10,it is only identified at t 13.The delay time for identifying t 10is t 13−t 10.The threshold δplays an important role in the delay and the number of line seg-ments.A smaller δwill reduce the delay time but result in a larger number of short line segments,some of which may still be noise.A larger δwill decrease the number of line segments but with longer delay.If δis too large,some useful information will be filtered out.There is a tradeoff between the delay time and more accurate piece-wise linear representation.We propose an optimized algorithm for simultaneous online segmentation and pruning.The new algorithm will reduce the delay time yet will give more accurate piecewise linear representation.3.2.3PruningBefore going into detail for our online segmentation and pruning algorithm,we first introduce the rationale and approach for prun-ing.To the best of our knowledge,no other published algorithm does pruning.Pruning is the process to remove noise-like line seg-ments along with the segmentation process.Segmentation tries to find potential end points using a smaller threshold δs ,so new end points can be identified with shorter delay time.Pruning is smooth-ing over recently identified end points.Noise introduced by small δs will be filtered out by the pruning process and more accurate line segments are generated.This segmentation and pruning mech-anism helps to quickly identify a new end point yet with accurate piecewise linear representation.The shorter delay time is very im-portant for real time applications such as stock data analysis.The end points are generally critical points for stock transactions.The earlier such points are identified,the better the chances are for prof-itable stock trading.The pruning process itself is a two-step process.First,%b is used in the filter step.But when mapping %b pruned end points onto raw data,the piecewise linear representation on raw data may still have some noise.It is possible that the %b data values change considerably while the raw data values change very little.So we need a refinement step.Pruning on the raw data stream not only removes the oscillations of a trend,but also enforces the zigzag shape.Under rare conditions,the end points mapped directly from %b end points may not be in the zigzag shape.Figure 3e and 3f shows the pruning results on both %b and on the raw stream data.The thick dotted line segments are new line segments generated by the pruning process.The corresponding filled line segments covered by dotted lines are removed.The actual technique for pruning is following.If the absolute %b or raw data values of two adjacent end points (called the amplitude )differs by less than a certain value,that line segment should be removed.Note there may be different values for pruning on %b from those used in pruning the raw data stream.The tricky part is we must keep the zigzag shape of the end points,so we must remove two adjacent end points at the same time.This creates aproblem as shown in Figure 5.Here,the line segment −→cd is under the pruning threshold,so pruning is needed.There are several waysto remove −→cd .In online segmentation and pruning,at each new end point,we check the previous line segment for pruning.For example,in Fig-ure 5,at the time when end point e is identified,line segment −→cd is tested for pruning.First we check the need for pruning on %b.If needed,pruning is carried out.Then the system waits for next stream data to come in and no pruning on raw data is done.If no pruning on %b is needed,the same line segment is checked for pruning on raw data.So there is at most one pruning at each end point.The pruning algorithm is the same for pruning on both %b and raw data.We compare the last end point with the third last end point to see which one gives a better piecewise linear representation.If the two points are upper points,the one with the larger value will be kept.Otherwise,if both lower points,the one with the smaller value will be kept.Figure 5gives an example for pruning with the last end point as a lower point.End points e and c are compared.If e has smaller value,end points c and d will be removed from the endpoints stream,and a new line segment −→be is generated (Figure 5b).If c has smaller value,end points d and e will be removed.Linesegment −→bc will remain (Figure 5b).3.3Online segmentation and pruningOur online subsequence similarity matching is based on the sim-ilarity between two subsequences of end points.A single-pass for online segmentation and pruning is mandatory.To reduce the time delay in identifying end points and improve piecewise linear repre-sentation,we use different thresholds for segmentation andprun-Figure 5:Two possible ways for pruning line segment cd .ing:a smaller threshold δs for segmentation over %b,a largerthreshold δb p for pruning over %b,and a separate δdp for pruning over raw stream data.A smaller threshold for segmentation will ensure the sensitivity and reduce delay.A larger pruning thresh-old will filter out noise.Our experiments show that δs 0.02,δbp1.5are suitable for most stock prices.The value of δd p is flexible and varies according to different users.Experiments have shown that10%to 20%of the price change over the trading pe-riod has reasonable results.For instance,for intra-day trading,ifa quote’s average daily price change is $1.50,δdp between $0.15to $0.30all can achieve pretty good results.The online segmentation and pruning are running simultaneously.Whenever an upper/lower point is identified by the segmentation process,the previous line segment is checked for pruning as men-tioned in Section 3.2.3.To better explain the online segmentation and pruning algorithm,an animation of the process is illustrated in Figure 6.Suppose now we are after the time when t 0is identified as an upper point (Figure 6a).As time goes on,P(t 1)is identified to be a potential lower point (Figure 6b).A temporary line seg-ment −−→t 0t 1is generated.The line segment immediately before t 0is checked for pruning.Since the amplitude of the line segment on%b is larger than δb p ,and that of raw stream is larger than δdp ,nei-ther pruning on %b nor on raw stream is needed.Similarly,end points P(t 2)and P(t 3)are identified as potential end points without pruning (Figure 6c).A pruning is encountered when P(t 4)is identified as a potentialupper point (Figure 6d).The line segment −−→t 2t 3is checked for prun-ing.Since the amplitude of −−→t 2t 3is less than δbp ,a pruning process is required.The last end point P(t 4)and the third last end point P(t 2)are compared for a better Piecewise Linear Representation on %b.Since both points are upper points,the one with the larger value will be kept.Here,the value at t 4is larger,end points P(t 2)and P(t 3)are removed,and line segments −−→t 1t 2−−→t 2t 3−−→t 3t 4on both%b and the raw stream are removed.A new line segment −−→t 1t 4is created.Continuing the segmentation and pruning process to time t 5,a new potential lower end point is identified without pruning.An-other pruning process is encountered at time t 6when a new po-tential upper point is identified (Figure 6e).The amplitude for theprevious line segment −−→t 4t 5on %b is larger than δbp ,so no pruningon %b is required.But the amplitude of −−→t 4t 5is less than thresh-old δdp ,pruning on raw data stream is required.By comparing the raw price values at t 4and t 6,The end point at t 6is kept while end points t 4and t 5are removed.As a summary for Figure 6,for time t 0to t 6,two end points on the raw data stream are identified,i.e.,the end points at t 1and t 6.All other potential end points are removed by pruning on either %b or the raw stream.The end points of %b are only a temporary tool and will not be kept in the final piecewise linear representation of the raw data stream.Also we have the following observations:•If an end point has one following line segment whose ampli-tude is larger than the pruning threshold on both %b and raw。
An ISS Small-Gain Theorem for General Networks, Juni
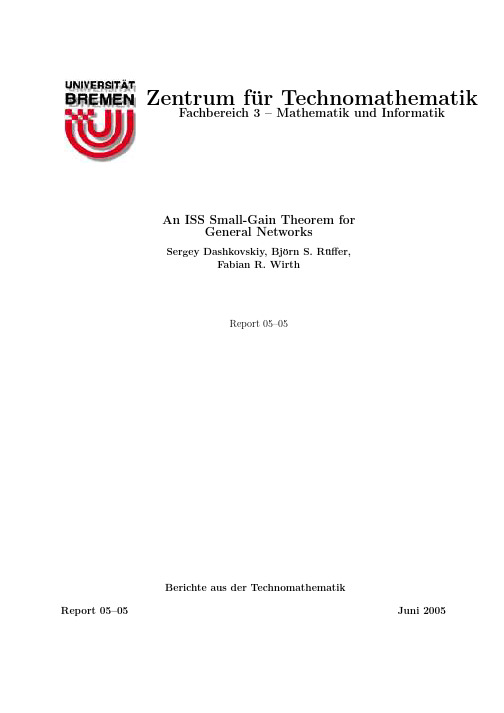
, , Universit¨ at Bremen, Zentrum f¨ ur Technomathematik, Postfach 330440, 28334 Bremen, Germany, ∗ , † Phone +49-421-218-9407, ‡ Phone +49-421-218-2763, ∗ , † , ‡ Fax +49-421-218-4235, {dsn,rueffer,fabian}@math.uni-bremen.de
Report 05–05
Berichte aus der Technomathematik Report 05–05 Juni 2005
An ISS Small-Gain Theorem for General Networks
Sergey Dashkovskiy∗ Bj¨ orn S. R¨ uffer† June 7, 2005 Fabian R. Wirth‡
Zentrum fu ¨ r Technomathematik
Fachbereich 3 – Mathematik und Informatik
An ISS Small-Gain Theorem for General Networks
Sergey Dashkovskiy, Bj¨ orn S. Ru ¨ ffer, Fabian R. Wirth
1
In particular, we obtain a general condition that guarantees input-to-state stability of a general system described as an interconnection of several ISS subsystems. The earliest interconnection result on ISS systems states that cascades of ISS systems are again ISS, see e.g., [11, 12, 13]. Furthermore, small-gain theorems for the case of two ISS systems in a feedback interconnection have been obtained in [3, 4, 5]. These results state in one way or another that if the composition of the gain functions of ISS subsystems is smaller than the identity, then the whole system is ISS. The papers [3, 4, 5] use different approaches to the formulation of small-gain conditions that yield sufficient stability criteria: In [4] the proof is based on the properties of KL and K∞ functions. This approach requires that the composition of the gains is smaller than the identity in a robust sense, see below for the precise statement. We show in Example 12 that within the context of this approach the robustness condition cannot be weakened. The result in that paper also covers practical ISS results, which we do not treat here. An ISS-Lyapunov function for the feedback system is constructed in [5] as some combination of the corresponding ISS-Lyapunov functions of both subsystems. The key assumption of the proof in that paper is that the gains are already provided in terms of the Lyapunov functions, by which the authors need not resort to a robust version of the small-gain condition. The proof of the small-gain theorem in [3] is based on the ISDS property and conditions for asymptotic stability of the feedback loop without inputs are derived. These results will turn out to be special cases of our main result. General stability conditions for large scale interconnected systems have been obtained by various authors in other contexts. In [8] sufficient conditions for the asymptotic stability of a composite system are stated in terms of the negative definiteness of some test matrix. This matrix is defined through the given Lyapunov functions of the interconnected subsystems. Similarly, in [9] conditions for the stability of interconnected systems in terms of Lyapunov functions of the individual systems are obtained. ˇ In [10] Siljak considers structural perturbations and their effects on the stability of composite systems using Lyapunov theory. The method is to reduce each subsystem to a one-dimensional one, such that the stability properties of the reduced aggregate representation imply the same stability properties of the original aggregate system. ¯, In some cases the aggregate representation gives rise to an interconnection matrix W ¯ yield asymptotic stability such that quasi dominance or negative definiteness of W of the composite system. In [17] small-gain type theorems for general interconnected systems with linear gains can be found. These results are of the form that the spectral radius of a gain matrix should be less than one to conclude stability. The result obtained here may be regarded as a nonlinear generalization in the same spirit. In this paper we consider a system which consists of two or more ISS subsystems. We provide conditions by which the stability question of the overall system can be reduced to consideration of stability of the subsystems. We choose an approach using estimates involving KL and K∞ functions to prove the ISS stability result for general interconnected systems. The generalized small-gain condition we obtain is,
Monotonic subsequences in dimensions higher
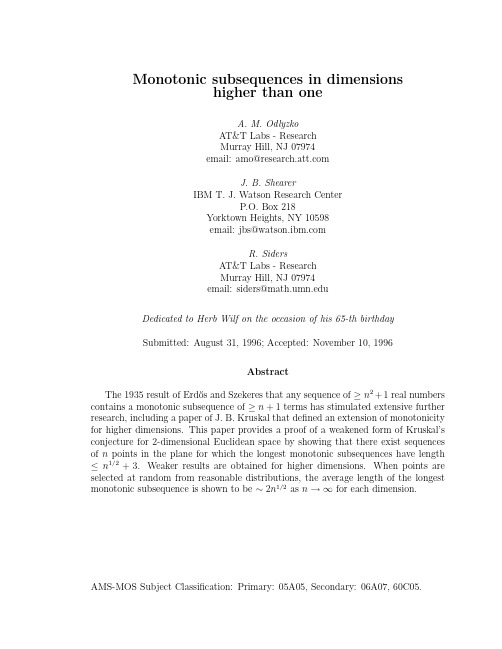
Monotonic subsequences in dimensionshigher than oneA.M.OdlyzkoAT&T Labs-ResearchMurray Hill,NJ07974email:amo@J.B.ShearerIBM T.J.Watson Research CenterP.O.Box218Yorktown Heights,NY10598email:jbs@R.SidersAT&T Labs-ResearchMurray Hill,NJ07974email:siders@Dedicated to Herb Wilf on the occasion of his65-th birthdaySubmitted:August31,1996;Accepted:November10,1996AbstractThe1935result of Erd˝o s and Szekeres that any sequence of≥n2+1real numbers contains a monotonic subsequence of≥n+1terms has stimulated extensive further research,including a paper of J.B.Kruskal that defined an extension of monotonicity for higher dimensions.This paper provides a proof of a weakened form of Kruskal’s conjecture for2-dimensional Euclidean space by showing that there exist sequences of n points in the plane for which the longest monotonic subsequences have length ≤n1/2+3.Weaker results are obtained for higher dimensions.When points are selected at random from reasonable distributions,the average length of the longest monotonic subsequence is shown to be∼2n1/2as n→∞for each dimension.AMS-MOS Subject Classification:Primary:05A05,Secondary:06A07,60C05.Monotonic subsequences in dimensionshigher than oneA.M.OdlyzkoAT&T Labs-ResearchMurray Hill,NJ07974email:amo@J.B.ShearerIBM T.J.Watson Research CenterYorktown Heights,NY10598email:jbs@R.SidersAT&T Labs-ResearchMurray Hill,NJ07974email:siders@Dedicated to Herb Wilf on the occasion of his65-th birthday1.IntroductionA sequence y1,...,y k of real numbers is said to be monotonic if either y1≤y2≤···≤y k or y1≥y2≥···≥y k.A classic theorem of Erd˝o s and Szekeres[4]states that every sequence of m2+1real numbers has a monotonic subsequence of m+1 terms.Moreover,there do exist sequences of m2real numbers with no monotonic subsequences of length greater than m.This extremal result has led to research on a range of related problems in both extremal and average behavior.For references,see the survey of Mike Steele[13],and[11].The result of Erd˝o s and Szekeres stimulated the question of what happens when in a sequence x1,...,x n,the real numbers x j are replaced by vectors x j from d-dimensional Euclidean space.Thefirst problem is to define what is meant by mono-tonicity for a subsequence in dimension d≥2.One way to do this is to say that asubsequence x i1,...,x ik,1≤i1<···<i k≤n,is monotonic if it is monotonic ineach coordinate(when the x j are presented in somefixed coordinate system).N.G. de Bruijn showed(see[8])that for this definition,a complete answer can be obtained from the Erd˝o s-Szekeres result.From a sequence of m2d+1vectors in d,a monotonic subsequence of m+1terms can be chosen,and this is best possible.A different generalization to higher dimensions,this time to relation spaces,was considered by J.B.Kruskal[8].In this case he was also able to obtain a complete answer using the Erd˝o s-Szekeres result.In this note we consider yet another generalization of monotonicity to vectors ind that was proposed by Kruskal in[8].It is more natural geometrically than the one considered by de Bruijn in that it is independent of the choice of the coordinatesystem.There are several definitions(all shown to be equivalent to each other in[8]).The one we will work with says that a sequence y1,...,y k,with y j∈ d for each j,is monotonic if there exists some nonzero w∈ d such that the sequence ofinner products(y1,w),...,(y k,w)is a monotonic sequence of real numbers.With this definition of monotonicity,any sequence of d+1points is monotonic.Also, since any nonzero w can be chosen,it is immediate by the Erd˝o s-Szekeres result[4] that a monotonic subsequence of≥ n1/2 points can be chosen from any sequence of n points.Kruskal conjectured that to guarantee the existence of a monotonic subsequence of length k≥d+1,it is necessary and sufficient that the total number of points n satisfy n≥k2−kd−k+d+1.If Kruskal’s conjecture is true,then for every d,there will be sequences of points in d with longest monotonic subsequences of length(1+o(1))n1/2as n→∞.As an aside,suppose we take y1,...,y n to be any of the sequences that are ex-tremal for the Erd˝o s-Szekeres result,so that the y j are real numbers,and the longestmonotonic subsequence among them has length n1/2 .Let us now construct a se-quence in d by placing the y j on a line,say x j=(y j,0,...,0)for1≤j≤n.Then for any w∈ d with nonzerofirst coordinate,the longest monotonic subsequence of (x1,w),...,(x n,w)will have length n1/2 .However,for w=(0,1,0,...,0),we will have(x j,w)=0for all j,so for this w we will obtain a monotonic subsequence of length n.This shows that if we required strict monotonicity for the subsequences of the(x j,w),the problem would have a trivial solution.We will show in Section2that if d isfixed and z1,...,z n are any n points in d that are in general position,then as n→∞,almost all permutations x1,...,x n of z1,...,z n will have their longest monotonic subsequence of length(2+o(1))n1/2 as n→∞.In particular,if the z j are chosen independently at random from some continuous distribution on d(say uniform on the unit cube),and are permuted at random,then we will get maximal monotonic subsequences of length(2+o(1))n1/2 as n→∞with high probability.Since most random choices give longest monotonic subsequences not much longerthan2n1/2for any d≥2,we get(asymptotically for n→∞)within a factor of2of what Kruskal’s conjecture predicts.However,that is the most that this method can do for us.On the other hand,in Section3we present an explicit construction of a sequence in d=2for which the longest monotonic subsequence has length≤n1/2+3. This shows that for d=2Kruskal’s conjecture is asymptotically tight.We expect that similar although more complicated constructions exist for all d≥3,and therefore that the asymptotic form of Kruskal’s conjecture is true for all dimensions.We do not know whether the exact form of Kruskal’s conjecture is correct.For d=2,we can improve our bound to≤n1/2+2,but we do not know whether our construction can be modified to give the full conjecture.2.Average behaviorUlam [15]was apparently the first one to ask about the distribution of L n ,the length of the longest increasing subsequence in a permutation of n distinct real num-bers.After initial work of Baer and Brock [1]and Hammersley [6],Logan and Shepp[9]and Vershik and Kerov [16]proved the conjecture that L n tends to 2n 1/2in prob-ability as n →∞.Later it was shown by Frieze [5]that the distribution of L n is concentrated near its mean.Frieze’s result was subsequently sharpened by Bol-lob´a s and Brightwell [2]and Talagrand [14].Some of the fine structure details of the distribution of L n are still unknown.For full references,numerical evidence,and conjectures about the distribution of L n ,see [10]and [11].In this paper we will use only two results.One follows from the lower bound of Logan and Shepp and of Vershik and Kerov:Proposition 2.1For every >0,Prob(L n >(2− )n 1/2)→1as n →∞.(2.1)The other result is a weak form of the upper bound that follows from the work of Frieze,of Bollob´a s and Brightwell,and of Talagrand.The result we will actually use follows also from the one-sided concentration result of J.-H.Kim [7],which is simpler to prove,but yields surprisingly strong bounds.(We will use only a weak version of Kim’s result.)Proposition 2.2For all α, >0,there is a constant C =C (α, )such thatProb(L n >(2+ )n 1/2)≤Cn −α.(2.2)Let us now consider points z 1,...,z n ∈ d that are in general position (no 3on a line,no 4in a plane,etc.).For any nonzero w ∈ d ,permuting the z j induces a permutation of the inner products (z j ,w ).Hence Proposition 2.1shows immediately that if we permute the z j ,the resulting sequences x 1,...,x n will almost always have monotonic subsequences of length ≥(2+o (1))n 1/2as n →∞.Suppose again that z 1,...,z n ∈ d are in general position,and suppose that x 1,...,x n is a permutation of z 1,...,z n .In determining monotonicity of subsequences of x 1,...,x n ,we only need to consider directions w that satisfy d −1linearly inde-pendent constraints of the form (w,z i −z j )=0.(Suppose we move w continuously without hitting any additional conditions (w,z i )=(w,z j ),and without destroying any conditions of this type that held before.Then the relative positions of the (w,z i )do not change,and when we do add an additional relation (w,z i )=(w,z j ),longest monotone subsequences can only grow.)However,there are fewer than n 2 d −1such directions w .For each w ,a random permutation of z 1,...,z n gives a random per-mutation of the n −2(d −1)numbers (w,z j )for which (w,z j )is unique.We apply Proposition 2.2to those,and conclude that the probability of a monotone subse-quence of length ≥(2+ )n 1/2+2d is ≤n −10d for n sufficiently large.Hence the probability of a monotone subsequence of length ≥(2+ )n 1/2+2d for any of the ≤n 2d directions w that need to be considered is o (1)as n →∞.Combining the results proved above,we obtain the following result.Theorem2.1If z1,...,z n∈ d are in general position,and are permuted at ran-dom,then for any >0,the length M n of the longest monotonic subsequence in the permuted sequence satisfiesProb((2− )n1/2≤M n≤(2+ )n1/2)→1as n→∞.(2.3) The restriction to general positions in Theorem2.1is important,since z1=z2=···=z n=0produces dramatically different behavior.Theorem2.1determines the typical asymptotic behavior of the length of the longest monotonic subsequence in d.The same methods can also be used to study the expected lengths of unimodal and related subsequences,if those notions are extended to d in the same way.(For d=1,these questions were answered by Chung[3]and Steele[12].)3.Extremal sequencesSection2showed that for any d≥2,there do exist sequences x1,...,x n∈ d with longest monotonic subsequences of length(2+o(1))n1/2as n→∞.That is within a factor of2of what Kruskal’s conjecture predicts.In this section we show that for d=2,we can construct sequences of points that gain that factor of2,and so come close to proving Kruskal’s conjecture.(Our construction yields sequences that in which the longest monotonic subsequences are longer by at most2than those predicted to exist by Kruskal’s conjecture.A careful examination of our proof shows that we can decrease our upper bound by1,and thus be at most1worse than Kruskal’s conjecture.)Theorem3.1For any n∈ +,there exists a sequence of points x1,...,x n∈ 2 which has no monotonic subsequence of length> n1/2 +2.Proof.Wefirst use induction to construct,for all k∈ +and0< <1/10,a sequence of points z1,...,z k∈ 2with the following properties:(i)the angle between any two lines determined by pairs of the z j is< .(ii)If the line through z1and z k is turned in a counterclockwise direction,the projections of the z j on that line appearfirst in the orderz1,z2,...,z k.Then,as the line keeps turning,z1moves past z2,so the order becomesz2,z1,z3,...,z k.Then z1moves past z3,...,z k,in turn,while the order of those points remains unchanged,until the order becomesz2,z3,...,z k,z1.Next,as the line continues turning,z k moves past z k −1,then past z k −2,...,and finally z 2to create the order of projectionsz k ,z 2,z 3,...,z k −1,z 1.Next,z 2moves past z 3,then z 4,...,until the order isz k ,z 3,z 4,...,z k −1,z 2,z 1,and then z k −1starts moving past z k −2,....In the end,when the rotation reaches 180◦,we see the reversed orderz k ,z k −1,...,z 2,z 1.(iii)If we look at the points z j in the order z 1,z k ,z 2,z k −1,z 3,z k −2,...,then anypoint z j lies inside the triangle determined by the preceding three points in this ordering.To start the induction,for k =1we choose z 1to be a point,for k =2let z 1and z 2be any two distinct points,and for k =3let z 1,z 2,and z 3be the three points in Fig.1that are labeled z 1,z 2,and z k ,respectively.Suppose that we can construct I (k −2, )for any 0< <1/10.We next proceed to construct I (k, )for any with 0< <1/10as follows.Let z 1=(0,0),z k =(4,0),and scale and translateI (k −2, /1000)so that if its points are z 1,...,z k −2,thenz 1=(2,− /10),z k −2=(3,−49 /1000).(See Fig.1for this construction.)If we then let z j =z j −1,2≤j ≤k −1,we easilysee that the sequence z 1,z 2,...,z k satisfies all the conditions for I (k, ).z z k2z k-1z 1Figure 1:Construction of points z 1,z 2,...,z k .We now proceed to prove Theorem 3.1.It suffices to construct a sequence x 1,...,x n satisfying the conditions of this theorem for n =m 2.Let z 1,...,z n be the sequence of points I (n,1/100)constructed above.We now rearrange them into the sequence12345678910111213141516Figure2:Diamond configuration for n=16,used to define the sequence x1, (x16)x1,...,x n.To do this,we write the numbers1,2,...,n into a regular diamond with 1on top,2and3beneath it(in that order,although any ordering of this or other rows would do just as well),4,5,and6beneath them,and so on,until we get n in the bottom row.(See Fig.2for n=16.)Read the points left to right,reading the columns from top to bottom for the column headed by1and all the columns to the left of that one,and reading the columns to the right of the central one from bottom to top.For the case n=16illustrated in Fig.2,we obtain the ordering7,4,11,2,8,14,1,5,12,16,15,9,3,13,6,10.(3.1)In general,if the sequence is s1,...,s n,we define z j=x sj for1≤j≤n.(For n=16,we have z1=x7,z2=x4,z3=x11,and so one.)We now look at projections of the x j onto a line that rotates counterclockwise, and starts parallel to the x-axis.Wefirst examine just those directions for which the projections of the z j in that direction have the orderingz n,z n−1,...,z n−t,z t+2,z t+3,...,z n−t−2,z n−t−1,z t+1,z t,...,z2,z1.(3.2) The ordering of the projections of the x j is obtained from the same diamond we started with,but after interchanging the t+1extreme pairs of points in the initial ordering.For example,for n=16and t=3,we interchange the pairs of labels(7,10), (4,6),(11,13),and(2,3)in the diamond of Fig.2.(See Fig.3for an illustration.) The interchanges in the diamond always involve pairs of points in the same row (except when at the end we interchange points inside the central column,first1with n,then5with n−4,and so on).Hence an increasing subsequence of projections must move to the right or down in the diamond,and so has at most m=n1/2elements. Similarly,a decreasing subsequence has to move left or up,and so also has at most m elements.It remains to consider projections intermediate between those that give arrange-ments of the form(3.2).However,these projections differ from those given by(3.2) in the positioning of at most two points.Hence any monotonic subsequence of our sequence has length≤m+2=n1/2+2.QED13265410897131211141516Figure3:Diamond configuration for n=16and t=3. References[1]R.M.Baer and P.Brock,Natural sorting over permutation spaces,p.22(1968),385–410.[2]B.Bollob´a s and G.Brightwell,The height of a random partial order:concentration of measure,Ann.Appl.Prob.2(1992),1009–1018.[3]F.R.K.Chung,On unimodal subsequences,b.Theory A29(1980),267–279.[4]P.Erd˝o s and G.Szekeres,A combinatorial theorem in geometry,Com-positio Math.2(1935),463-470.[5]A.Frieze,On the length of the longest monotone subsequence in arandom permutation,Ann.Appl.Prob.1(1991),301–305.[6]J.M.Hammersley,A few seedlings of research,pp.345–394in Proc.6th Berkeley Symp.Math.Stat.Prob.,Univ.California Press,1972.[7]J.-H.Kim,On the longest increasing subsequences of random permu-tations-a concentration result,b.Th.A76(1996),148–155.[8]J.B.Kruskal,Monotonic subsequences,Proc.Amer.Math.Soc.4(1953),264-274.[9]B.F.Logan and L.A.Shepp,A variational problem for random Youngtableaux,Advances Math.26(1977),206–222.[10]A.M.Odlyzko,B.Poonen,H.Widom,and H.S.Wilf,On the distri-bution of longest increasing subsequences in random permutations,inpreparation.[11]A.M.Odlyzko and E.M.Rains,On longest increasing subsequencesin random permutations,to be published.[12]J.M.Steele,Long unimodal subsequences:A problem of F.R.K.Chung,Discrete Math.33(1981),223–225.[13]J.M.Steele,Variations on the monotone subsequence theme of Erd˝o sand Szekeres,pp.111-131in Discrete Probability and Algorithms,D.Aldous,P.Diaconis,J.Spencer,and J.M.Steele,eds.,Springer,1995.[14]M.Talagrand,Concentration of measure and isoperimetric inequalitiesin product spaces,Publ.Math.Inst.Hautes Etud.Sci.81(1995),73-205.[15]S.M.Ulam,Monte Carlo calculations in problems of mathematicalphysics,pp.261–281in Modern Mathematics for the Engineer,E.F.Beckenbach,ed.,McGraw-Hill,1961.[16]A.M.Vershik and C.V.Kerov,Asymptotics of the Plancheral measureof the symmetric group and a limiting form for Young tableaux,Dokl.Akad.Nauk USSR,233(1977),1024–1027.(In Russian.)。
POJ:3061-Subsequence(尺取法模板详解)
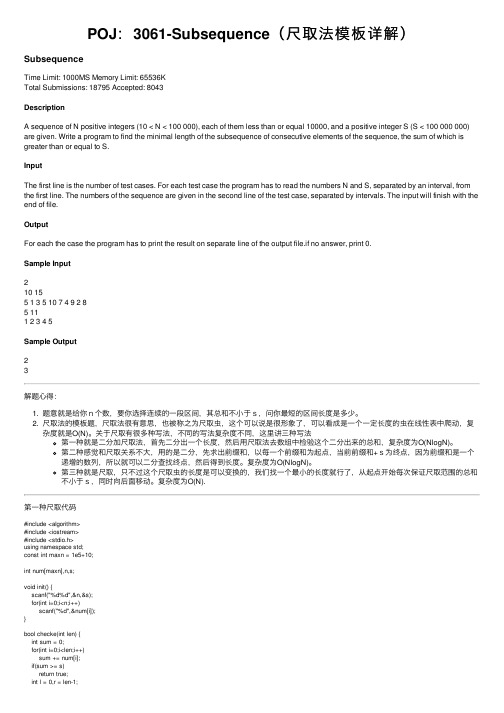
POJ:3061-Subsequence(尺取法模板详解)SubsequenceTime Limit: 1000MS Memory Limit: 65536KTotal Submissions: 18795 Accepted: 8043DescriptionA sequence of N positive integers (10 < N < 100 000), each of them less than or equal 10000, and a positive integer S (S < 100 000 000) are given. Write a program to find the minimal length of the subsequence of consecutive elements of the sequence, the sum of which is greater than or equal to S.InputThe first line is the number of test cases. For each test case the program has to read the numbers N and S, separated by an interval, from the first line. The numbers of the sequence are given in the second line of the test case, separated by intervals. The input will finish with the end of file.OutputFor each the case the program has to print the result on separate line of the output file.if no answer, print 0.Sample Input210 155 1 3 5 10 7 4 9 2 85 111 2 3 4 5Sample Output23解题⼼得:1. 题意就是给你n个数,要你选择连续的⼀段区间,其总和不⼩于s,问你最短的区间长度是多少。
Longest common subsequence
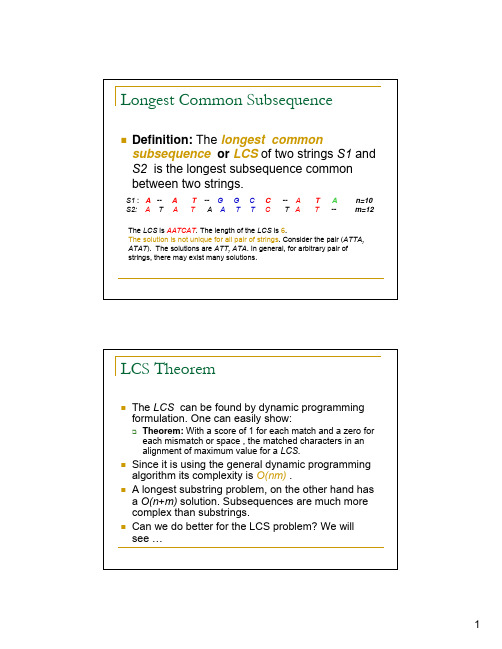
If I is an IS of π with length equal to the size of a cover C of π, then I is a LIS of π and C is the smallest cover of size c.
5
Proof
If I is an increasing sequence, it cannot contain more than one element from a decreasing sequence. This means that no increasing subsequence can have size more than the size of any cover C, that is, if a maximum of one element from each can participate in any increasing sequence. Thus, an IS derived from this decomposition can have a maximum length of |C |=c. Conversely, C must be the smallest. If not, let c’ be the length of a cover C’ such that |C’|=< c i.e., if we derive IS from C, it must contain more than one element from one of the decreasing sequence of C’, which is not possible. Hence C has to be of smallest size.
- 1、下载文档前请自行甄别文档内容的完整性,平台不提供额外的编辑、内容补充、找答案等附加服务。
- 2、"仅部分预览"的文档,不可在线预览部分如存在完整性等问题,可反馈申请退款(可完整预览的文档不适用该条件!)。
- 3、如文档侵犯您的权益,请联系客服反馈,我们会尽快为您处理(人工客服工作时间:9:00-18:30)。
一、问题描述
Common Subsequence
Time Limit: 2000/1000 MS (Java/Others) Memory Limit: 65536/32768 K (Java/Others)
Total Submission(s): 9876 Accepted Submission(s): 4062
Problem Description
A subsequence of a given sequence is the given sequence with some elements (possible none) left out. Given a sequence X = <x1, x2, ..., xm> another sequence Z = <z1, z2, ..., zk> is a subsequence of X if there exists a strictly increasing sequence <i1, i2, ..., ik> of indices of X such that for all j = 1,2,...,k, xij = zj. For example, Z = <a, b, f, c> is a subsequence of X = <a, b, c, f, b, c> with index sequence <1, 2, 4, 6>. Given two sequences X and Y the problem is to find the length of the maximum-length common subsequence of X and Y.
The program input is from a text file. Each data set in the file contains two strings representing the given sequences. The sequences are separated by any number of white spaces. The input data are correct. For each set of data the program prints on the standard output the length of the maximum-length common subsequence from the beginning of a separate line.
Sample Input
abcfbc abfcab
programming contest
abcd mnp
Sample Output
4
2
Source
Southeastern Europe 2003
Recommend
Ignatius
二、问题分析与算法实现
三、参考代码
//dp[i][j]表示{xi}和{yj}的最长公共子序列的长度
//i=0 || j=0 dp[i][j]=0;
//xi==yj dp[i][j]=dp[i-1][j-1]+1
//xi!=yi dp[i][j]=max{dp[i-1][j],dp[i][j-1]}
#include <iostream>
#include <string>
using namespace std;
int dp[2000][2000];
void cal(string str1, string str2)
{
int i,j;
int len1,len2;
len1=str1.length ();
len2=str2.length ();
for(i=0;i<=len1;i++)
dp[i][0]=0;
for(j=0;j<=len2;j++)
dp[0][j]=0;
for(i=1;i<=len1;i++)
for(j=1;j<=len2;j++)
{
if(str1[i-1]==str2[j-1])
dp[i][j]=dp[i-1][j-1]+1;
else
dp[i][j]=dp[i-1][j]>dp[i][j-1]?dp[i-1][j]:dp [i][j-1];
}
/*
for(i=1;i<=len1;i++)
{
for(j=1;j<=len2;j++)
{
cout<<dp[i][j]<<" ";
}
cout<<endl;
}
*/
}
int main()
{
string str1,str2;
while(cin>>str1>>str2)
{
cal(str1,str2);
cout<<dp[str1.length ()][str2.length ()]<<endl; }
return 0;
}
蔡尚真代码;阮凯云 2011-10-25整理。