Trajectory Pattern Mining
英语演讲——采矿工程专业简介

From Mining Engineering of School of Mines: 矿业学院采矿工程专业 贺雪峰 HeXuefeng 孙超群 SunCaoqun 安成龙 AnChenglong 张国营 ZhangGuoying 梁 栋 Liangdong 刘 伟 Liuwei 卢志鹏 LuZhipeng
Mining Engineering采矿工程
Mining Engineering采矿工程
1
Introduction of Mining Engineering采矿工程概论
2
Mining Technology挖掘技术
3
Coal Mining Production System煤矿生产系统
4
Roadway Supporting Patterns巷道的支护方式
中国13大煤炭基地: 神东,山西北部,山西东部和内蒙古东部,云南,贵州,河南, 山东西部,山西西部,山西中部,北部和南部的安徽,河北中部, 宁夏东部,山西北部。
Mining technology挖掘技术
Blasting mining technology 爆破开采技术
.
1
2
Conventionally mechanized mining technology传统的机械化开采技术 Fully mechanized mining technology 综采技术
采矿业本身是工业的 龙头行业,承担为工 业企业提供能源及动 力的重任,在国民经 济发展中地位据重要。
Introduction介绍
According to the statistics, Chinese miner deaths each year about 6000, but certainly far more than this number, it is USA 100 times, even 10 times of India. Some people say: "the death of a American soldiers, will die 7 China miners". 对公统计,中国矿工 每年死亡人数大概有 6000,但肯定远远不 止这个数,就目前都 是美国100倍,甚至是 印度的10倍。 有人说:“死一个美 国士兵,就会死7个中 国矿工”。
group based trajectory model

group based trajectory model
基于群体的轨迹模型(Group-Based Trajectory Model,GBTM)是一种用于描述和分析群体中个体随时间变化的发展轨迹的统计方法。
这种模型可以应用于各种领域,如心理学、社会学、教育学等,以揭示不同个体在某一特定领域内的发展模式。
GBTM的基本原理是,假设群体中的个体在发展过程中会呈现出不同的轨迹,而这些轨迹可以由一组潜在的类别来描述。
每个类别代表了一种特定的发展模式,例如,在心理学中,可能存在的类别包括“健康成长”、“逐渐恶化”或“快速恢复”等。
通过统计方法,GBTM能够识别出这些潜在类别,并将个体分配到相应的类别中,从而揭示出群体内不同发展模式的存在。
使用GBTM进行分析时,通常需要收集个体在多个时间点上的数据。
这些数据可以是连续的,如考试成绩、心理健康评分等,也可以是离散的,如职业选择、婚姻状况等。
通过对这些数据进行建模,GBTM能够揭示出不同发展轨迹的形状、速度和稳定性等特征。
GBTM的优点在于它能够处理大量数据,并从中提取出有意义的信息。
它能够识别出群体内不同的发展模式,并揭示这些模式与个体特征之间的关系。
此外,GBTM还能够预测个体未来的发展轨迹,从而为干预和决策提供依据。
然而,GBTM也存在一些局限性。
首先,它假设群体中的个体发展轨迹是固定的,而实际上个体的发展可能会受到多种因素的影响而发生变化。
其次,GBTM在处理复杂
数据时可能会遇到困难,例如当存在多个潜在类别或数据存在缺失时。
最后,使用GBTM 进行分析需要一定的统计知识和经验,否则可能会导致结果的误解或误用。
2-frequent-pattern--d

频繁,但满足passing down 条件,则需要进一步 考察子结点。
“level passage threshold”:一般设置为当前层的 minisupp和下一层的minisupp之间的一个值。
8
去掉冗余的多层规则
例如:
Desktop Computer→b/w printer (support=0.08, confidence=0.7)
IBM Desktop Computer→b/w printer (support=0.02, confidence=0.72) (冗余)
如果一条规则的支持度ຫໍສະໝຸດ 可信度接近于它的预期值,则说它是冗余的。预期值是由它的祖先规则和子项目
在父项目中所占的比例决定的。例如:
上例中IBM Desktop Computer占Desktop Computer
比例为0.25的话,则“预期”支持度为0.02.
9
Mining Multi-Dimensional Association
Single-dimensional rules:
“bins”中。这些“bins”在数据挖掘的过程中可以进一 步结合起来。动态
3. 基于距离的AR:数值属性的离散化是为了捕捉数据间
的语义. 动态离散过程考虑数据点之间的距离,故称为基 于距离的AR
11
Mining Quantitative Associations
数值属性在挖掘之前被离散为以区间段表示的分类属性,如果需要的话分类 属性还可以用更一般的高层概念取代。
3
多层挖掘算法
❖自顶向下,逐层挖掘
使用统一的minisupp 优点: 简单 缺点: 如果minisupp 过大, 低层会丢失很多规则 如果minisupp 过小, 高层会产生很多无用规则
基于ais数据的船舶航行轨迹预测
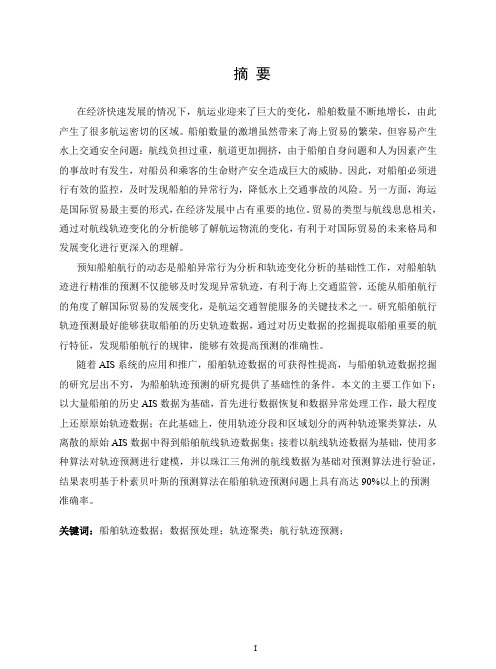
摘要在经济快速发展的情况下,航运业迎来了巨大的变化,船舶数量不断地增长,由此产生了很多航运密切的区域。
船舶数量的激增虽然带来了海上贸易的繁荣,但容易产生水上交通安全问题:航线负担过重,航道更加拥挤,由于船舶自身问题和人为因素产生的事故时有发生,对船员和乘客的生命财产安全造成巨大的威胁。
因此,对船舶必须进行有效的监控,及时发现船舶的异常行为,降低水上交通事故的风险。
另一方面,海运是国际贸易最主要的形式,在经济发展中占有重要的地位。
贸易的类型与航线息息相关,通过对航线轨迹变化的分析能够了解航运物流的变化,有利于对国际贸易的未来格局和发展变化进行更深入的理解。
预知船舶航行的动态是船舶异常行为分析和轨迹变化分析的基础性工作,对船舶轨迹进行精准的预测不仅能够及时发现异常轨迹,有利于海上交通监管,还能从船舶航行的角度了解国际贸易的发展变化,是航运交通智能服务的关键技术之一。
研究船舶航行轨迹预测最好能够获取船舶的历史轨迹数据,通过对历史数据的挖掘提取船舶重要的航行特征,发现船舶航行的规律,能够有效提高预测的准确性。
随着AIS系统的应用和推广,船舶轨迹数据的可获得性提高,与船舶轨迹数据挖掘的研究层出不穷,为船舶轨迹预测的研究提供了基础性的条件。
本文的主要工作如下:以大量船舶的历史AIS数据为基础,首先进行数据恢复和数据异常处理工作,最大程度上还原原始轨迹数据;在此基础上,使用轨迹分段和区域划分的两种轨迹聚类算法,从离散的原始AIS数据中得到船舶航线轨迹数据集;接着以航线轨迹数据为基础,使用多种算法对轨迹预测进行建模,并以珠江三角洲的航线数据为基础对预测算法进行验证,结果表明基于朴素贝叶斯的预测算法在船舶轨迹预测问题上具有高达90%以上的预测准确率。
关键词:船舶轨迹数据;数据预处理;轨迹聚类;航行轨迹预测;AbstractWith the rapid development of economy, the shipping industry has been developing rapidly, and the number of ships has been increasing. The surge in the number of vessels at sea has brought prosperity of trade, but it is easy to cause the problem of water traffic safety: route burden, channel congestion caused by the ship's own problems and human factors in the accident, the crew and passengers of the life and property safety threat. Therefore, it is necessary to carry out effective monitoring on the ship, find out the abnormal behavior of the ship in time, and reduce the risk of water traffic accidents. On the other hand, shipping is the most important form of international trade, which plays an important role in economic development. The type of trade is closely related to the route. Through the analysis of the change of the route, we can understand the change of shipping logistics, which is beneficial to the further understanding of the future pattern and development of international trade.To predict the dynamic navigation is the basis of the analysis of ship monitoring and track changes in the work, the accurate prediction of the ship trajectory can not only detect the abnormal trajectory for marine traffic, but also from the ship's point of view to understand the development and change of international trade, shipping and transportation is one of the key technologies of intelligent service.With the application and popularization of the AIS system, the availability of ship trajectory data is improved, which provides the basic conditions for ship trajectory data mining. The main work of this thesis is as follows: in the history of a large number of ships based on the AIS data, the first data recovery and data processing work, to restore the original data on the maximum extent, clean the available data; on this basis, two kinds of trajectory clustering algorithm using trajectory segmentation and region division, get the ship route trajectory data set from the original AIS data in a discrete;Then take the route trajectory data is based on the combination of various methods of trajectory prediction modeling, and to route data in the Pearl River Delta for verification based on the prediction algorithm, the results show that the prediction algorithm based on Naive Bayesian with up to 90% accuracy in the prediction of ship trajectory.Keywords: Ship trajectory data; data pretreatment; trajectory clustering; navigation trajectory prediction;目录第一章绪论 (1)1.1 研究背景 (1)1.2 研究现状 (1)1.2.1 数据恢复 (2)1.2.2轨迹聚类 (2)1.2.3船舶航行轨迹预测 (4)1.3 研究内容 (6)1.4 技术路线 (7)1.5 论文结构安排 (8)第二章相关理论基础 (10)2.1船舶航行轨迹预测 (10)2.2轨迹相似性度量 (10)2.3 总结 (12)第三章 AIS数据采集及预处理 (13)3.1 数据采集 (13)3.2 船舶航线轨迹数据提取 (14)3.2.1 基于船舶航行状态的航线轨迹数据提取 (14)3.2.2 基于船舶航速和采集时间间隔的轨迹数据提取 (15)3.2.3 航线轨迹数据样例 (16)3.3 缺失值处理 (17)3.3.1 问题描述 (17)3.3.2缺失值识别 (17)3.3.3 缺失值插补方法 (18)3.3.4 缺失数据插补 (20)3.3.5 数据实验 (21)3.4 异常数据处理 (24)3.5 总结 (25)第四章基于AIS数据的船舶航线聚类 (27)4.1 航线聚类定义与描述 (27)4.2 航线聚类算法 (27)4.2.1 基于轨迹分段的航线聚类算法 (28)4.2.2 基于航行区域相似度的航线聚类算法 (40)4.3 轨迹聚类结果评价指标 (45)4.4 数据实验 (46)4.4.1 实验数据 (46)4.4.2 模型参数设置 (46)4.4.3实验结果 (48)4.5总结 (50)第五章基于AIS数据的船舶航行轨迹预测 (51)5.1 船舶轨迹预测的定义与描述 (51)5.2 轨迹统计分析 (51)5.3 基于AIS数据的船舶航行轨迹预测算法 (53)5.3.1 基于概率统计的船舶航行轨迹预测算法 (53)5.3.2 基于船舶轨迹相似度的船舶航行轨迹预测算法 (57)5.3.3 基于加权KNN的船舶航行轨迹预测算法 (58)5.3.4 基于朴素贝叶斯的船舶航行轨迹预测算法 (60)5.4 实验分析 (63)5.4.1 基础数据 (63)5.4.2 实验设置 (64)5.4.3 实验结果 (66)5.5总结 (69)第六章总结和展望 (70)6.1 工作总结 (70)6.2未来展望 (70)参考文献 (71)攻读硕士学位期间取得的成果 (78)致谢 (79)第一章绪论第一章绪论1.1 研究背景航运是国际贸易最主要的形式,在经济全球化的环境下,航运业得到飞速的发展,船舶越造越大,种类愈来愈多,由此在国内和国际上产生很多航运密切的热点区域,如珠江三角洲。
离散数学英文DMAv7_10.1_2_3_4 (1)

Roadmaps
This is a GPS trajectory dataset collected in (Microsoft Research Asia) GeoLife project by 182 users in a period of over two years (from April 2007 to August 2012). This trajectory dataset can be used in many research fields, such as mobility pattern mining, user activity recognition, location-based social networks, location privacy, and location recommendation. The following heat maps visualize its distribution in Beijing.
Graph Theory
Rosen 7th ed., ch. 10
Chapter 10
Graphs
图
图/Graph:
论
可直观地表示离散对象之间的相 互关系,研究它们的共性和特性,以 便解决具体问题。
10.1 图的概念/Introduction of Graph 10.2 图的术语/Graph Terminology 10.3 图的表示与同构/ Representing Graph and Graph Isomorphism 10.4 连通性/Connectivity
10.2 图的术语/Graph Terminology
[定义]相邻和关联:
在无向图G中,若e=(a,b) ∈E,则称a 与/connect。a、b称为边e的端点或结束顶点 /endpoint. 在有向图G中,若e=(a,b)∈E,即箭头由 a到b,称a相邻到b,或a关联或联结b。a称为e 的起点/initial vertex,b称为e的终点/terminal or end vertex。
基于群组与密度的轨迹聚类算法

第47卷第4期Vol.47No.4计算机工程Computer Engineering2021年4月April2021基于群组与密度的轨迹聚类算法俞庆英1,2,赵亚军1,2,叶梓彤1,2,胡凡1,2,夏芸1,2(1.安徽师范大学计算机与信息学院,安徽芜湖241002;2.安徽师范大学网络与信息安全安徽省重点实验室,安徽芜湖241002)摘要:现有基于密度的聚类方法主要用于点数据的聚类,不适用于大规模轨迹数据。
针对该问题,提出一种利用群组和密度的轨迹聚类算法。
根据最小描述长度原则对轨迹进行分段预处理找出具有相似特征的子轨迹段,通过两次遍历轨迹数据集获取基于子轨迹段的群组集合,并采用群组搜索代替距离计算减少聚类过程中邻域对象集合搜索的计算量,最终结合群组和密度完成对轨迹数据集的聚类。
在大西洋飓风轨迹数据集上的实验结果表明,与基于密度的TRACLUS轨迹聚类算法相比,该算法运行时间更短,聚类结果更准确,在小数据集和大数据集上的运行时间分别减少73.79%和84.19%,且运行时间的减幅随轨迹数据集规模的扩大而增加。
关键词:群组;密度;群组可达;邻域搜索;轨迹聚类开放科学(资源服务)标志码(OSID):中文引用格式:俞庆英,赵亚军,叶梓彤,等.基于群组与密度的轨迹聚类算法[J].计算机工程,2021,47(4):100-107.英文引用格式:YU Qingying,ZHAO Yajun,YE Zitong,et al.Trajectory clustering algorithm based on group and density[J]. Computer Engineering,2021,47(4):100-107.Trajectory Clustering Algorithm Based on Group and DensityYU Qingying1,2,ZHAO Yajun1,2,YE Zitong1,2,HU Fan1,2,XIA Yun1,2(1.School of Computer and Information,Anhui Normal University,Wuhu,Anhui241002,China;2.Anhui Provincial Key Laboratory of Network and Information Security,Anhui Normal University,Wuhu,Anhui241002,China)【Abstract】The existing density-based clustering methods are mainly used for point data clustering,and not suitable for large-scale trajectory data.To address the problem,this paper proposes a trajectory clustering algorithm based on group and density. According to the principle of Minimum Description Length(MDL),the trajectories are preprocessed by segments to find out the sub trajectories with similar characteristics.The group set based on the sub trajectories is obtained by traversing the trajectories dataset twice,and the group search is used to replace the distance calculation to reduce the calculation amount required for the neighborhood object set search in the clustering process.Finally,the trajectory data set is clustered by combining the group and density.Experimental results on Atlantic hurricane track dataset show that,compared with the density-based TRACLUS track clustering algorithm,the running time of the proposed algorithm is less and the clustering results are more accurate.The running time on the small dataset and large dataset is reduced by73.79%and84.19%respectively,and the reduction of running time increases with the expansion of track dataset.【Key words】group;density;group reachability;neighborhood search;trajectory clusteringDOI:10.19678/j.issn.1000-3428.00574250概述随着定位、通信和存储技术的快速发展,车辆行驶轨迹数据、用户活动轨迹数据以及飓风轨迹数据等大量移动对象的轨迹数据可被搜集和存储。
基于语义轨迹模式的移动轨迹去匿名化攻击方法

90 80 70 60 504
30 201
• ■ TP-attack
POI-attack
—a— PIT-attack
长公共子序列中任意两个连续的语义停留点之间的重叠时间 比,表示最长公共子序列中发生的重叠转 移时间,表示相邻停留区域转移过程中发生 的所有转移时间。
因此,轨迹模式D和勺之间的相似度计算如式(4 )所示:
ZHANG Wenshuai3, ZHANG Haojun3, YANG Weidong3, XU Zhenqiangb
(a. School of Information Science and Engineering, b. School of Artificial Intelligence and Big Data, Henan University of Technology, Zhengzhou Henan 450001, China) Abstract: The author proposes a de-anonymization attack method based on semantic trajectory patterns. The semantic trajectory pattern mining algor让hm is used to obtain the frequent semantic trajectory pattern set of each moving object, which is used as the trajectory feature to construct its behavior profile, and the corresponding similarity is further designed. Measure and experiment on real trajectory data sets. Experimental results show that this method can obtain a relatively high deanonymization success rate. Keywords: privacy protection; de-anonymization attacks; mobile trace; frequent pattern mining; similarity measure
实用轮胎英语词汇

OTR Tire English层级plyratingPRPlyRating星级starratingLILoadIndex轮辋rim层数numberofplies带束层belt胎侧胶sidewallrubber胎冠crown胎肩shoulder胎肩垫胶shoulderwedge胎肩区shoulderarea胎里tyrecavity胎面tread定向花纹directionaltreadpattern断面高度sectionheight断面宽度sectionwidth防擦线kerbingrib钢丝包胶wirecovering钢丝圈beadring高宽比(H/S)aspectratio(H/S)隔离胶insulationrubber公路花纹highwaytreadpattern拱形轮胎archtyre骨架材料frameworkmaterial光胎面smoothtread横向花纹transversepattern花纹沟treadgroove花纹加强盘tie-barofpattern花纹角度patternangle花纹块patternblock花纹条patternrib花纹纹深度patterndepth花纹细缝patternsipe缓冲层breaker缓冲胶片breakerstrip混合花纹dualpurposetreadpattern 宽基轮胎widebasetyre轮胎tyre(tire)轮胎标志tyremarking轮胎规格tyresize轮胎系列tyreseries内衬层insideliner内胎innertube内胎胎身tubebody气密层innerliner三角胶apex沙漠轮胎sandtyre实心轮胎solidtyre速度符号speedsymbol 胎侧sidewall胎面过渡胶胎面花纹胎面基部胎面基部胶胎面磨耗标志胎面行驶面胎圈胎体椭圆形轮胎外胎外直径无内胎轮胎斜交轮胎新胎雪泥花纹有内胎轮胎圆柱实心轮胎越野花纹越野轮胎装配线装饰胎侧子午线轮胎纵向花纹作业环境Openpitmine=地下矿采沙场采石场伐木场建筑集装箱码头设备经销商政府公共工程设备清单攻城机械列表Terminal测试胎用户transitionrubberoftreadtreadpatterntreadbasetreadslabbasetreadwearindicatortread capbeadcarcassellipticaltyrecoveroveralldiametertubelesstyrediagonaltyrenewtyrem udandsnowpatterntubedtyrecylindricalbasesolidtyrecross-countrytreadpatterncross-countrytyrefittinglinedecorativesidewallradialplytyrecircumferentialpatternOperating conditionsurfaceminingUndergroundmineSandquarryGravelquarryLoggingConstructi onShippingcontainerEquipmentdealerGovermentpublicworkEquipmentlistOTRvehicle listenduserTestaccount大客户LargeaccountTreadworn-out花纹磨光小客户SmallaccountRock块状花Treaddesign花纹设计Rib条形花Treadcompounds胎面配方Smooth光面胎Treaddepth花纹深度Smooth extra deep tread光面超加深花纹Heat resistance耐高温CompetitivetreadcompoundtypesSeparation脱层Standard标准配方Beltedgeseparation带束层边部脱层Cut resistance耐切割Beadseparation胎圈脱层punctureandtear穿刺和撕裂Rockimpact岩石撞击Excellent traction优秀的牵引力Sidewallimpact胎侧撞击Improved fuel economy改进燃油经济性Rockdrillingintread胎面岩石刺扎operator comfort操控舒适性Resistance to flats, punctures, tear耐变形,穿刺和撕裂Treadseoaration胎面脱层Enhanced traction加强牵引力Tread shoulder heat separation胎肩热脱层Ability to work in all environments能在各种环境下作业Tread groove cracking 花纹沟裂口Tread chunking胎面掉块Low overall operating costs降低总体运营成本Treadcut胎面划伤decreased downtime减少停机时间Tire brandincreaseuptime增加正常运营时间Customer engineering客户工程machinerycomponents机械部件Industrycode工业代码distance per hour时速Sidewall marking胎侧标示size轮胎型号Ton-km-per-hourTKPH值forklift叉车RatedTKPH额定TKPH值articulateddumper铰链式卡车Job TKPH现场TKPH值containerhandler集装箱调运车Work-capacity-factorWCF值crane起重机Load负荷/Rated load额定负荷WideBasetire宽基轮胎Load distribution负荷分配TRATireandRimAssociationETRTOEuropeanTyreandRimTechnicalOrganizationFrant / rear前轴/后轴Pressure / rated pressure气压/额定气压Calculate / calculationv,vt计算Failed tire inspection失效胎鉴定calculate the TKPH figures计算TKPH值OEM配套商scenen.现场Dealer经销商On-site service现场服务Net weight空车净重Tire tracking轮胎跟踪Payload货物重量TireretreadandrepairprogramsGVW=gross vehicle weight=NET+Payload轮胎翻新和维修方案Roundtrip往返全程Waiting=4.5 minutesLoading=4.1 minutesDumping卸载Hauling=19.0 minutesDumping=2.0 minutesAverage speed平均速度Return=14.0 minutesHauling运输Total Minutes/Cycle=43.60 minutesDump truck刚性自卸车Hours/Cycle=43.60/60=.727Articulated dump truck铰卡Cycles/Day=24/.727=33Kms/Day=33.0x12=396.3Undergurunddozer地下推土机AvgSpeed=396.3/24=16.5 KPHGrader平地机平路机SitestudyatChangjiangCoppermineLoader装载机Tread depth gauge胎面花纹深度计★Pressureoughttobeadjustmenttomatchmaximumoverloads.Foreach1%increas einload,theinflationpressuremustbeincreasedby2%.(OVER)。
一种保持轨迹数据高可用性的隐式位置访问隐私保护技术
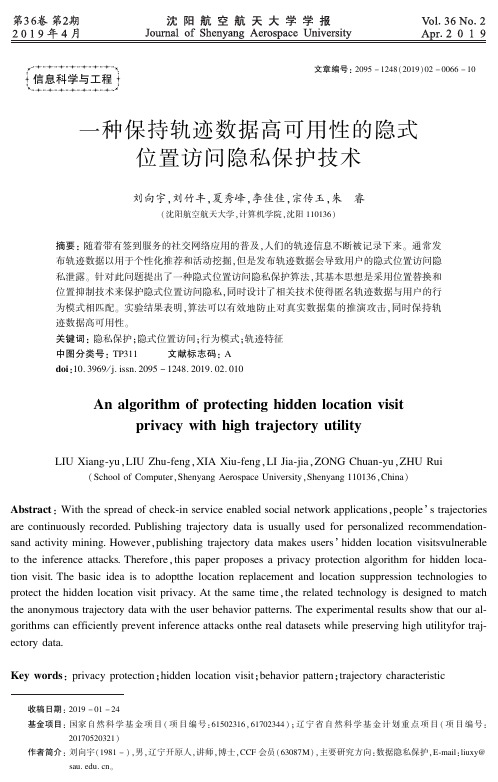
一种保持轨迹数据高可用性的隐式 位置访问隐私保护技术
刘向宇ꎬ刘竹丰ꎬ夏秀峰ꎬ李佳佳ꎬ宗传玉ꎬ朱 睿
( 沈阳航空航天大学ꎬ计算机学院ꎬ沈阳 110136)
摘要: 随着带有签到服务的社交网络应用的普及ꎬ人们的轨迹信息不断被记录下来ꎮ 通常发 布轨迹数据以用于个性化推荐和活动挖掘ꎬ但是发布轨迹数据会导致用户的隐式位置访问隐 私泄露ꎮ 针对此问题提出了一种隐式位置访问隐私保护算法ꎬ其基本思想是采用位置替换和 位置抑制技术来保护隐式位置访问隐私ꎬ同时设计了相关技术使得匿名轨迹数据与用户的行 为模式相匹配ꎮ 实验结果表明ꎬ算法可以有效地防止对真实数据集的推演攻击ꎬ同时保持轨 迹数据高可用性ꎮ 关键词: 隐私保护ꎻ隐式位置访问ꎻ行为模式ꎻ轨迹特征 中图分类号: TP311 文献标志码: A doi:10. 3969 / j. issn. 2095 - 1248. 2019. 02. 010
虽然用户可能在某些敏感位置进行位置访 问时避免签到ꎬ但用户却难以注意到隐式位置 访问隐私的泄露ꎮ 如图 1( a) 所示为用户 u 的 轨迹集合 Tꎬ从 t1 到 t5 分别是 u 产生的五条轨 迹ꎮ 而轨迹 t1 以及各个位置在地图上的方位 展示在了图 1(b)中ꎬ各个位置的语义信息展示 在了图 1( c) 中ꎮ 注意在本文中 T 都认为是一
( School of ComputerꎬShenyang Aerospace UniversityꎬShenyang 110136ꎬChina)
Abstract: With the spread of check ̄in service enabled social network applicationsꎬpeople’ s trajectories are continuously recorded������ Publishing trajectory data is usually used for personalized recommendation ̄ sand activity mining������ Howeverꎬpublishing trajectory data makes users’ hidden location visitsvulnerable to the inference attacks������ Thereforeꎬthis paper proposes a privacy protection algorithm for hidden loca ̄ tion visit������ The basic idea is to adoptthe location replacement and location suppression technologies to protect the hidden location visit privacy������ At the same timeꎬthe related technology is designed to match the anonymous trajectory data with the user behavior patterns������ The experimental results show that our al ̄ gorithms can efficiently prevent inference attacks onthe real datasets while preserving high utilityfor traj ̄ ectory data������
基于网页日志的频繁模式挖掘
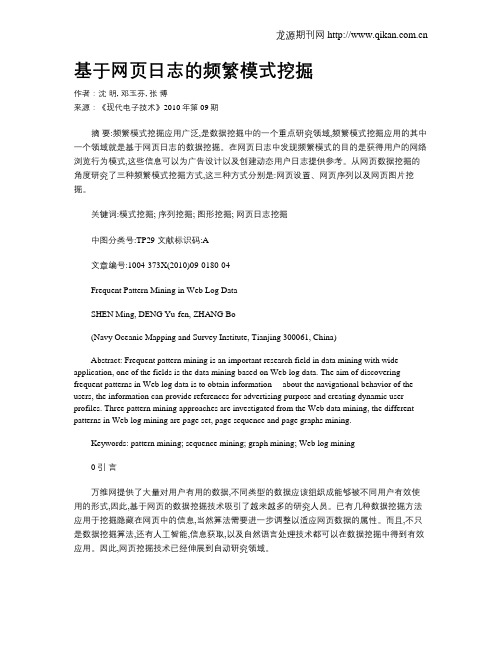
基于网页日志的频繁模式挖掘作者:沈明, 邓玉芬, 张博来源:《现代电子技术》2010年第09期摘要:频繁模式挖掘应用广泛,是数据挖掘中的一个重点研究领域,频繁模式挖掘应用的其中一个领域就是基于网页日志的数据挖掘。
在网页日志中发现频繁模式的目的是获得用户的网络浏览行为模式,这些信息可以为广告设计以及创建动态用户日志提供参考。
从网页数据挖掘的角度研究了三种频繁模式挖掘方式,这三种方式分别是:网页设置、网页序列以及网页图片挖掘。
关键词:模式挖掘; 序列挖掘; 图形挖掘; 网页日志挖掘中图分类号:TP29 文献标识码:A文章编号:1004-373X(2010)09-0180-04Frequent Pattern Mining in Web Log DataSHEN Ming, DENG Yu-fen, ZHANG Bo(Navy Oceanic Mapping and Survey Institute, Tianjing 300061, China)Abstract: Frequent pattern mining is an important research field in data mining with wide application, one of the fields is the data mining based on Web log data. The aim of discoveringavigational behavior of the users, the information can provide references for advertising purpose and creating dynamic user profiles. Three pattern mining approaches are investigated from the Web data mining, the different patterns in Web log mining are page set, page sequence and page graphs mining.Keywords: pattern mining; sequence mining; graph mining; Web log mining0 引言万维网提供了大量对用户有用的数据,不同类型的数据应该组织成能够被不同用户有效使用的形式,因此,基于网页的数据挖掘技术吸引了越来越多的研究人员。
Chapter 6- Mining Association Rules in Large Databases

For rule A C: support = support({A C}) = 50% confidence = support({A C})/support({A}) = 66.6%
使用频繁项集产生关联规则
数计学院 陈晓云
Data Mining: Concepts and Techniques
13
The Apriori Algorithm
连接步(Join Step): 设Lk-1是频繁k-1项集,候选k项集Ckis
generated by joining Lk-1with itself
Data Mining: Concepts and Techniques 4
数计学院 陈晓云
强规则: 同时满足最小支持度阀值和最小值信度阀值 的规则称为强规则。 项集:项的集合。 含k个项的集合称为k-项集 支持计数(支持数,频率,计数) 包含项集的事务数。
数计学院 陈晓云
Data Mining: Concepts and Techniques
几篇论文:Apriori算法在学生成绩管理中的应用
基于APRIORI算法和兴趣度的糖耐量实验数据关 联规则挖掘和筛选 基于关联规则的日志分析系统的研究与设计 Data Mining: Concepts and Techniques 数计学院 陈晓云 聚类思想在挖掘关联规则中的运用
2
Examples. 规则形式: ―Body ead [support, confidence]‖. buys(x, ―尿布”) buys(x, ―啤酒”) [0.5%, 60%] major(x, ―CS‖) ^ takes(x, ―DB‖) grade(x, ―A‖) [1%, 75%] 支持度和臵信度是两个规则的兴趣度度量,分别放映发 现规则的有用性和确定性。
Process Mining学习笔记(一) _ 面子与里子
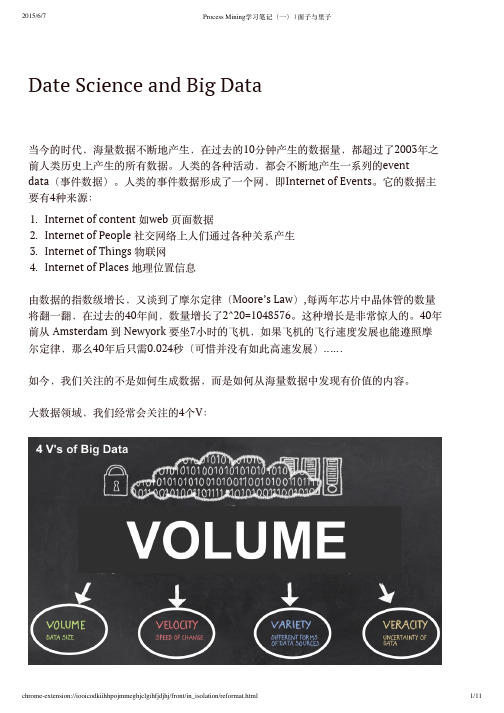
Date Science and Big Data当今的时代,海量数据不断地产生,在过去的10分钟产生的数据量,都超过了2003年之前人类历史上产生的所有数据。
人类的各种活动,都会不断地产生一系列的eventdata(事件数据)。
人类的事件数据形成了一个网,即Internet of Events。
它的数据主要有4种来源:1. Internet of content 如web 页面数据2. Internet of People 社交网络上人们通过各种关系产生3. Internet of Things 物联网4. Internet of Places 地理位置信息由数据的指数级增长,又谈到了摩尔定律(Moore’s Law),每两年芯片中晶体管的数量将翻一翻,在过去的40年间,数量增长了2^20=1048576。
这种增长是非常惊人的。
40年前从 Amsterdam 到 Newyork 要坐7小时的飞机,如果飞机的飞行速度发展也能遵照摩尔定律,那么40年后只需0.024秒(可惜并没有如此高速发展)……如今,我们关注的不是如何生成数据,而是如何从海量数据中发现有价值的内容。
大数据领域,我们经常会关注的4个V:大数据的4V1. Volume(容量):海量数据2. Velocity(速度):数据在不断的变化3. Variety(多样):数据的多样性,文本,图象,音视频等4. Veracity(真实):数据的真实性数据科学领域,我们会提出以下的4个问题:1. What happened?过去发生了什么?2. Why did it happend?为什么会发生?3. What will happen?将会发生什么?(做预测Prediction)4. What is the best that can happend?如何更好的发生?这门课程集中在基于过程process的数据,利用 event data,来改进过程。
Spatiotemporalfrequentpatternminingforpublicsafety时空频繁模式挖掘的公共安全

Art courtesy of Thomas Kinkade Pastoral House
A Few Applications using Visual Similarity Search
Adviser: Prof. Nikolaos Papanikolopoulos
*Contact:
Talk Outline
❖ Introduction
❖ Problem Statement
❖ Algorithms for Similarity Search in ❖ Matrix Valued Data ❖ High Dimensional Vector Data
6. A. Cherian, V. Morellas, and N. Papanikolopoulos. Approximate Nearest Neighbors via Dictionary Learning, Proceedings of SPIE, 2019. (Chapters 5,6,7)
Courtesy: Google Street View
3D Scene Reconstruction: Technical Analysis
• Typically SIFT point descriptors (128D) are used as point descriptors
• Each image produces several thousand SIFT descriptors (let us say 10K SIFTs/image)
7. S. Sra, and A. Cherian. Generalized Dictionary Learning for Symmetric Positive Definite Matrices with Application to Nearest Neighbor Retrieval, European Conference on Machine Learning (ECML), 2019. (Chapter 8)
Data Mining分析方法
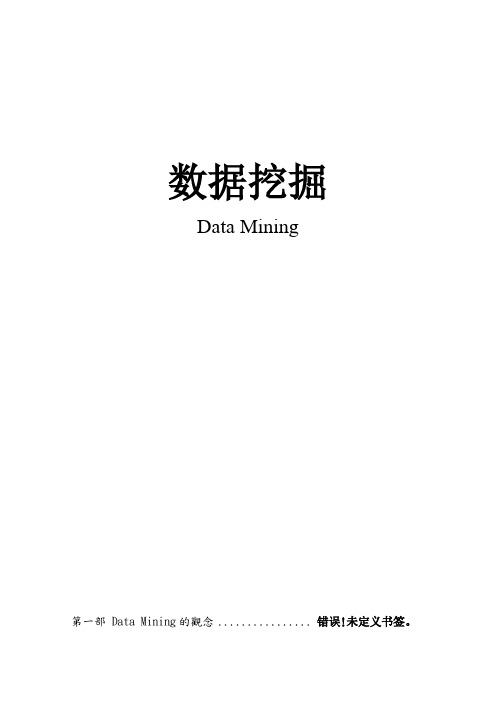
数据挖掘Data Mining第一部Data Mining的觀念 ............................. 错误!未定义书签。
第一章何謂Data Mining ..................................................... 错误!未定义书签。
第二章Data Mining運用的理論與實際應用功能............. 错误!未定义书签。
第三章Data Mining與統計分析有何不同......................... 错误!未定义书签。
第四章完整的Data Mining有哪些步驟............................ 错误!未定义书签。
第五章CRISP-DM ............................................................... 错误!未定义书签。
第六章Data Mining、Data Warehousing、OLAP三者關係為何. 错误!未定义书签。
第七章Data Mining在CRM中扮演的角色為何.............. 错误!未定义书签。
第八章Data Mining 與Web Mining有何不同................. 错误!未定义书签。
第九章Data Mining 的功能................................................ 错误!未定义书签。
第十章Data Mining應用於各領域的情形......................... 错误!未定义书签。
第十一章Data Mining的分析工具..................................... 错误!未定义书签。
第二部多變量分析.......................................... 错误!未定义书签。
利用广泛靶标代谢组学表征熊胆粉对大鼠胆汁酸组的影响
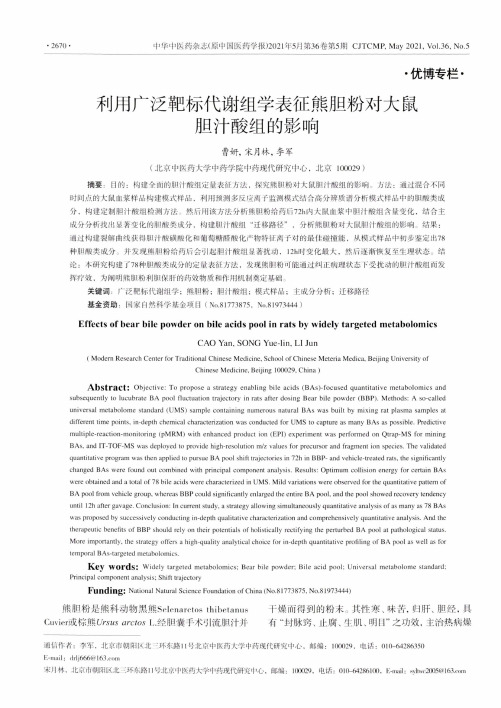
•2670 •t1华中医药杂志(原中国医药学报)2021年5月第36卷第5期CJTCMP,May 2021,Vol.36, No.5•优博专栏.利用广泛靶标代谢组学表征熊胆粉对大鼠胆汁酸组的影响曹姘,宋月林,李军(北京中医药大学中药学院中药现代研究中心,北京100029)摘要:0的:构建全面的胆汁酸组定量表征方法,探究熊胆粉对大鼠胆汁酸组的影响,方法:通过混合不同 时间点的大鼠血浆样品构建模式样品,利用预测多反应离子监测模式结合高分辨质谱分析模式样品中的胆酸类成分,构建定制胆汁酸组检测方法。
然后用该方法分析熊胆粉给药后72h内大鼠血浆中胆汁酸组含M变化,结合主成分分析找出显著变化的胆酸类成分,构建胆汁酸组“迁移路径”,分析熊胆粉对大鼠胆汁酸组的影响结果:通过构建裂解曲线获得胆汁酸磺酸化和葡萄糖醛酸化产物特征离子对的最佳碰撞能,从模式样品中初步鉴定出78种胆酸类成分。
并发现熊胆粉给药后会引起胆汁酸组显著扰动,I2h时变化最大,然后逐渐恢复至生理状态。
结论:本研究构建了78种胆酸类成分的定量表征方法,发现熊胆粉可能通过纠正病理状态下受扰动的胆汁酸组而发挥疗效,为阐明熊胆粉利胆保肝的药效物质和作用机制奠定基础,关键词:广泛靶标代谢组学;熊胆粉;胆汁酸组;模式样品;主成分分析;迁移路径基金资助:国家A然科学基金项目(No.81773875, N〇.81973444)Effects of bear bile powder on bile acids pool in rats by widely targeted metabolomicsCAO Yan,SONG Yue-lin,LI Jun(Modem Research Center for Traditional Chinese Medicine, School of Chinese Meteria Medica, Beijing University ofChinese Medicine, Beijing 100029, China )Abstract: Objective: To propose a strategy enabling bile acids (BAs)-focused quantitative metabolomics and subsequently to lucubrate BA pool fluctuation trajectory in rats after dosing Bear bile powder (BBP). Methods: A so-calleduniversal metabolome standard (UMS) sample containing numerous natural BAs was built by mixing rat plasma samples atdifferent time points, in-depth chemical characterization was conducted for UMS to capture as many BAs as possible. Predictivemultiple-reaction-monitoring (pMRM) with enhanced product ion (EPI) experiment was performed on Qtrap-MS for miningBAs, and IT-TOF-MS was deployed to provide high-resolution m/z values for precursor and fragment ion species. The validatedquantitative program was then applied to pursue BA pool shift trajectories in 72h in BBP- and vehicle-treated rats, the significantlychanged BAs were found out combined with principal component analysis. Results: Optimum collision energy for certain BAswere obtained and a total of 78 bile acids were characterized in UMS. Mild variations were observed for the quantitative pattern ofBA pool from vehicle group, whereas BBP could significantly enlarged the entire BA pool, and the pool showed recovery tendencyuntil 12h after gavage. Conclusion: In current study, a strategy allowing simultaneously quantitative analysis of as many as 78 BAswas proposed by successively conducting in-depth qualitative characterization and comprehensively quantitative analysis. And thetherapeutic benefits of BBP should rely on their potentials of holistically rectifying the perturbed BA pool at pathological status.More importantly, the strategy offers a high-quality analytical choice for in-depth quantitative profiling of BA pool as well as fortemporal BAs-targeted metabolomics.K e y W o r d s:Widely targeted metabolomics; Bear bile powder; Bile acid pool; Universal metabolome standard;Principal component analysis; Shift trajectoryFunding! National Natural Science Foundation of China (No.81773875, No.81973444)熊胆粉是熊科动物黑熊Selenarctos thihHanus干燥而得到的粉末。
PatternRecognition
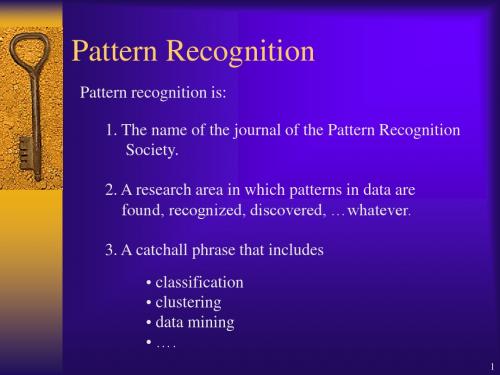
16
Decision Trees
#holes
0 moment of inertia
1 #strokes
2
#strokes
<t
best axis direction
0
t #strokes 2 x 4 w
0
1 0 1
60
90
-
/
1
0
A
8
B
17
Decision Tree Characteristics
20
Information Gain
The information gain of an attribute A is the expected reduction in entropy caused by partitioning on this attribute. |Sv| Gain(S,A) = Entropy(S) ----- Entropy(Sv) v Values(A) |S| where Sv is the subset of S for which attribute A has value v.
8
Classification using nearest class mean
Compute the
Euclidean distance between feature vector X and the mean of each class.
Choose closest class,
if close enough (reject otherwise)
The data is converted to a discrete structure (such as a grammar or a graph) and the techniques are related to computer science subjects (such as parsing and graph matching).
甜菜夜蛾幼虫增殖Gr NPV的研究
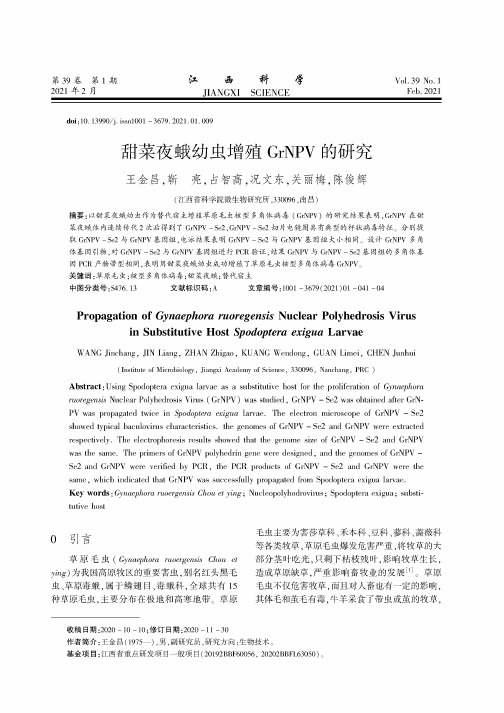
第39卷第1期注為科修Vol.39No.1 2021年2月JIANGXI SCIENCE Feb.2021doi:10.13990/j.&(1001-3679.2021.01.009甜菜夜蛾幼虫增殖GrNPV的研究王金昌,靳亮,占智高,况文东,关丽梅,陈俊辉(江西省科学院微生物研究所,330096,南昌)摘要:以甜菜夜蛾幼虫作为替代宿主增殖草原毛虫核型多角体病毒(GrNPV)的研究结果表明,GrNPV在甜菜夜蛾体内连续传代2次后得到了GrNPV-Se2,GrNPV-Se2切片电镜图具有典型的杆状病毒特征。
分别提取GrNPV-Se2与GrNPV基因组,电泳结果表明GrNPV-Se2与GrNPV基因组大小相同。
设计GrNPV多角体基因引物,对GrNPV-Se2与GrNPV基因组进行PCR验证,结果GrNPV与GrNPV-Se2基因组的多角体基因PCR产物带型相同,表明用甜菜夜蛾幼虫成功增殖了草原毛虫核型多角体病毒GrNPV。
关键词:草原毛虫;核型多角体病毒;甜菜夜蛾;替代宿主中图分类号:S476.13文献标识码:A文章编号:1001-3679(2021)01-041-04Propagation of Gynaephora ruoregensis Nuclear Polyhedrosis Vimsii Substitutive Host Spodoptera exigua LarvaeWANG Jinchang,JIN Liang,ZHAN Zhigao,KUANG Wendong,GUAN Limei,CHEN Junhui (Institute of Microbiology,Jiangxi Academy of Science,330096,Nanchang,PRC)Abstract:Using Spodopera exigua larvae as a substitutive host for the proliferation of Gynaephora ruoregensis NucOar Polyhedrosis Virus(GrNPV)was studid,GrNPV-Se2was obtained afteN GrNPV was propaaated twicc in Spodoptera exigua larvae.The eictron microscope of GrNPV一Se2 showed typiccl baculovirus characteristics.the gnomes of GrNPV一Se2and GrNPV wero extracted respectively.The eictrophoresis oesuys showed that the genome size of GrNPV一Se2and GrNPV was the same.The primero of GrNPV polyhedriv gene wcro designed,and the genomes of GrNPV一Se2and GrNPV wero arified by PCR,the PCR products of GrNPV-Se2and GrNPV we r e the same,which indicated that GrNPV was successfully propagated from Spodoptero exigua larvae.'ey words:Gnaeppora ruoergensis Choo eg ygg;Nucleopolyhodrovirus;SpodopttN exigua;substitutive host0引言草原毛虫(Gnaeppora reoergensis Chou et ying)为我国高原牧区的重要害虫,别名红头黑毛虫、草原毒蛾,属于鳞翅目、毒蛾科,全球共有15种草原毛虫,主要分布在极地和高寒地带。
- 1、下载文档前请自行甄别文档内容的完整性,平台不提供额外的编辑、内容补充、找答案等附加服务。
- 2、"仅部分预览"的文档,不可在线预览部分如存在完整性等问题,可反馈申请退款(可完整预览的文档不适用该条件!)。
- 3、如文档侵犯您的权益,请联系客服反馈,我们会尽快为您处理(人工客服工作时间:9:00-18:30)。
13 of 90
Extension of the work proposed by [Laube 2004, 2005] Gudmundsson(2006)
Computes the longest duration flock patterns The longest pattern has the longest duration And has at least a minimal number of trajectories
Encounter: At least m entities will be concurrently inside the same circular region of radius r, assuming they move with the same speed and direction.
Frequent groups are computed with the algorithm Apriori
Group pattern: time, distance, and minsup
9/28/2013
Tutorial on Spatial and Spatio-Temporal Data Mining (ICDM 2010)
9/28/2013
Tutorial on Spatial and Spatio-Temporal Data Mining (ICDM 2010)
1 of 90
Trajectory Data (Giannotti 2007 – www.geopkdd.eu)
Spatio-temporal Data Represented as a set of points, located in space and time T=(x1,y1, t1), …, (xn, yn, tn) => position in space at time ti was (xi,yi)
9 of 90
General Geometric Trajectory Patterns
9/28/2013
Tutorial on Spatial and Spatio-Temporal Data Mining (ICDM 2010)
10 of 90
Relative Motion Patterns (Laube 2004)
Semantic-based spatio-temporal data mining
Deal with sparse data also Patterns are computed based on the semantics of the data Trajectories are pre-processed to enrich the data
(e.g. traffic jam at some moment if cars keep moving in the same direction)
Leadership
Encounter
Flock
9/28/2013
Tutorial on Spatial and Spatio-Temporal Data Mining (ICDM 2010)
20% 5% 7% 60% ? 8%
= cell
9/28/2013
Tutorial on Spatial and Spatio-Temporal Data Mining (ICDM 2010)
6 of 90
Trajectory Data Mining Methods
9/28/2013
Tutorial on Spatial and Spatio-Temporal Data Mining (ICDMTutorial on Spatial and Spatio-Temporal Data Mining (ICDM 2010)
14 of 90
Frequent Trajectory Patterns
9/28/2013
Tutorial on Spatial and Spatio-Temporal Data Mining (ICDM 2010)
Group together similar trajectories For each group produce a summary
= cell
9/28/2013
Tutorial on Spatial and Spatio-Temporal Data Mining (ICDM 2010)
4 of 90
7 of 90
Spatio-Temporal Data Mining Methods
Two approaches:
Geometry-based spatio-temporal data mining:
Density-based clustering methods Focus on physical similarity Consider only geometrical properties of trajectories (space and time)
Flock pattern: At least m entities are within a region of radius r and move in the same direction during a time interval >= s (e.g. traffic jam)
Leadership: At least m entities are within a circular region of radius r, they
Tutorial on Spatial and Spatio-Temporal Data Mining (ICDM 2010)
9/28/2013
8 of 90
Geometry-based Trajectory Data Mining Methods
9/28/2013
Tutorial on Spatial and Spatio-Temporal Data Mining (ICDM 2010)
12 of 90
Relative Motion Patterns (Laube 2004)
Recurrence: at least m entities visit a circular region at least k times
F1 F1
Recurrence
F1 F1
9/28/2013
Tutorial on Spatial and Spatio-Temporal Data Mining (ICDM 2010)
move in the same direction, and at least one of the entities is heading in that direction for at least t time steps. (e.g. bird migration, traffic accident)
16 of 90
Co-Location Patterns (Cao 2006)
Co-location episoids in spatio-temporal data Trajectories are spatially close in a time window and move together
Gudmundsson (2007)
proposes approximate algorithms for computing the patterns leadership, encounter, convergence, and flock Focus relies on performance issues
Proposed 5 kinks of trajectory patterns based on movement, direction, and location: convergence, encounter, flock, leadership, and recurrence
Convergence: At least m entities pass through the same circular region of radius r, not necessarily at the same
15 of 90
Frequent Mobile Group Patterns (Hwang, 2005)
A group pattern is a set of trajectories close to each other (with distance less than a given minDist) for a minimal amount of time (minTime) Direction is not considered
5 of 90
Mining Trajectories: classification models
Fosca Giannotti 2007 – www.geopkdd.eu
Extract behaviour rules from history Use them to predict behaviour of future users
Mining Trajectories : Frequent patterns
Fosca Giannotti 2007 – www.geopkdd.eu
Frequent followed paths
= cell
9/28/2013
Tutorial on Spatial and Spatio-Temporal Data Mining (ICDM 2010)
Tutorial on Spatial and Spatio-Temporal Data Mining (ICDM – 2010)