Tseng(2001)
企业外部知识搜索双元与创新绩效:以创新复杂性和产业竞争压力为调节
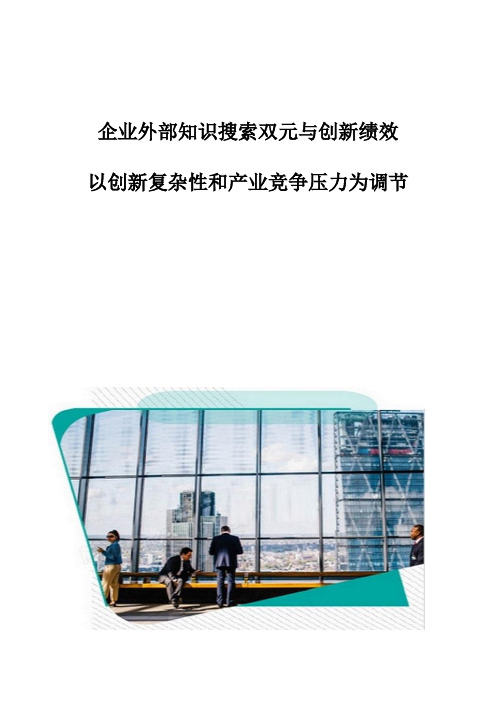
企业外部知识搜索双元与创新绩效以创新复杂性和产业竞争压力为调节开放式创新已成为企业的主导创新模式,然而已有研究对于企业“是否应该以及是否能够同时实施本地和国际知识搜索战略,在什么条件下实施更为有利”的问题并没有给出满意的答案。
本研究创造性地整合组织双元与外部知识搜索理论,界定了外部知识搜索双元的两个维度(平衡维度和联合维度)的含义,并实证检验了外部知识搜索双元的平衡维度和联合维度对创新绩效的影响,以及创新复杂性和产业竞争压力对上述影响的调节效应。
基于中国219家制造企业的问卷调查结果表明:外部知识搜索双元的平衡维度和联合维度对创新绩效均具有显著正向影响;创新复杂性、产业竞争压力均正向调节外部知识搜索双元的两个维度与创新绩效的关系。
研究结论对组织双元、外部知识搜索以及创新管理理论和实践具有重要启示。
一引言实施国际化发展战略已成为中国企业在全球化背景下实现创新追赶的重要战略举措。
通过进入国际市场,企业一方面能够在国际舞台上寻找创新资源和学习机会,进而提升企业创新能力和竞争力;另一方面,企业还能够在国际市场上利用已有的技术和营销优势,进而扩大市场份额(Luo & Tung,2007)。
根据中华人民共和国商务部发布的《中国对外投资合作发展报告》,中国企业对外直接投资连续10年(2002-2011年)保证增长势头。
2011年,中国对外直接投资流量达到746.5亿美元,较上年增长8.5%,名列世界第六位。
由此可见,国际市场已成为中国企业竞相追逐的战略市场。
然而,中国企业缺乏国际化所需的技术知识、营销知识和国际化运营经验,企业在国际化过程中不可避免地会遭受各种障碍,如外来者障碍(liability of foreignness)、新到者障碍(liability of newness)(Zaheer,1995),而企业外部网络作为一种重要的知识获取渠道,为中国企业克服国际化障碍提供了契机(Oviatt & McDougall,1994;Tseng et al.,2007;Yu,Gilbert & Oviatt,2011)。
舒淇
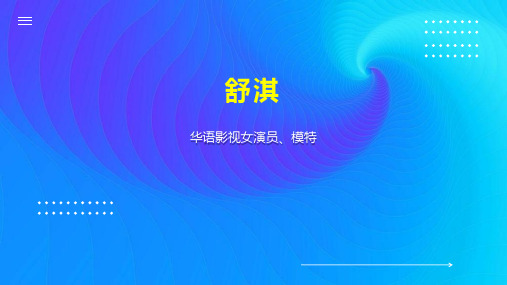
主要作品
社会活动
传递“珍惜爱,分享爱”使命的地球天使(5张)2008年5月15日,舒淇现身北京出席某品牌旗舰店开幕活动, 捐出自己的活动酬劳给汶川灾区。舒淇还表示由其投资的主题餐厅也将营业收入捐献灾区。
2010年3月25日,舒淇向西南旱灾捐款10万元 。
2011年6月5日,由舒淇创意并倡导的“地球天使”在台北诞生;10月29日,舒淇发起的“地球天使”公益活 动正式启动 。
个人生活
家庭 舒淇和冯德伦(2张)舒淇的爸爸从事普通的文职工作,妈妈主要是操持家务,家里还有个弟弟 。 感情 1997年,舒淇与冯德伦因拍摄电影《美少年之恋》而相识,并传出绯闻 。 2009年,两人在冯德伦的寓所约会,冯德伦还为舒淇做饭。此后两人不时被人拍到一起出游及约会 。 2014年3月,舒淇上传了一张冯德伦与爱猫对望吃饭的照片,随后,舒淇的经纪人默认了两人的恋情 。 2016年9月3日,舒淇和冯德伦通过寰亚宣传总监的**发布结婚消息 。
2012年6月5日,舒淇在台北出席“地球天使”环保公益活动。
人物评价
舒淇长得很好看,无论穿长裙还是西装,骨子里总透着一股懒洋洋 。(《时代人物》评)她领悟力强,善 于学习,是娱乐圈女星转型的典范 。(新华网评)她现场的反应非常快,而且很自然 。(导演侯孝贤评)在 《风尘三侠之红拂女》中,舒淇饰演的红拂女美艳不可方物,她前半部冷静、善良、独立、有思想、有主见,后 半部单纯、可爱、天真表现得也很到位,前后判若两人,可以说舒淇将红拂女身上的风尘气息演绎的内敛深邃, 仿佛她只是一个遗落在人间的仙女般 。(1905电影网评)
面条粉改良剂
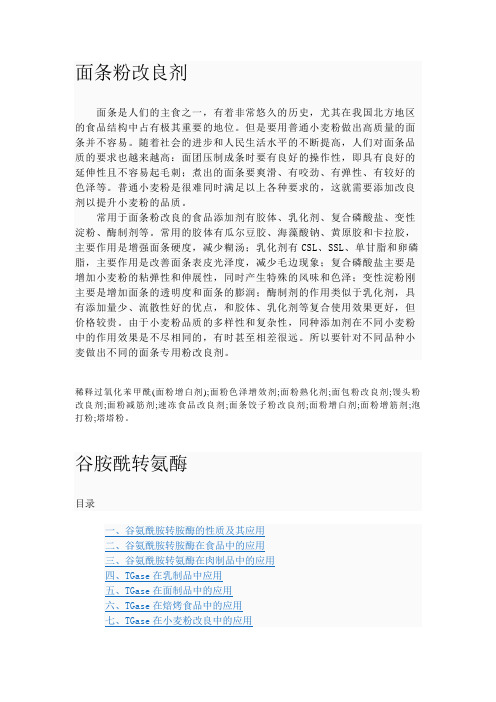
面条粉改良剂面条是人们的主食之一,有着非常悠久的历史,尤其在我国北方地区的食品结构中占有极其重要的地位。
但是要用普通小麦粉做出高质量的面条并不容易。
随着社会的进步和人民生活水平的不断提高,人们对面条品质的要求也越来越高:面团压制成条时要有良好的操作性,即具有良好的延伸性且不容易起毛刺;煮出的面条要爽滑、有咬劲、有弹性、有较好的色泽等。
普通小麦粉是很难同时满足以上各种要求的,这就需要添加改良剂以提升小麦粉的品质。
常用于面条粉改良的食品添加剂有胶体、乳化剂、复合磷酸盐、变性淀粉、酶制剂等。
常用的胶体有瓜尔豆胶、海藻酸钠、黄原胶和卡拉胶,主要作用是增强面条硬度,减少糊汤;乳化剂有CSL、SSL、单甘脂和卵磷脂,主要作用是改善面条表皮光泽度,减少毛边现象;复合磷酸盐主要是增加小麦粉的粘弹性和伸展性,同时产生特殊的风味和色泽;变性淀粉刚主要是增加面条的透明度和面条的膨润;酶制剂的作用类似于乳化剂,具有添加量少、流散性好的优点,和胶体、乳化剂等复合使用效果更好,但价格较贵。
由于小麦粉品质的多样性和复杂性,同种添加剂在不同小麦粉中的作用效果是不尽相同的,有时甚至相差很远。
所以要针对不同品种小麦做出不同的面条专用粉改良剂。
稀释过氧化苯甲酰(面粉增白剂);面粉色泽增效剂;面粉熟化剂;面包粉改良剂;馒头粉改良剂;面粉减筋剂;速冻食品改良剂;面条饺子粉改良剂;面粉增白剂;面粉增筋剂;泡打粉;塔塔粉。
谷胺酰转氨酶目录编辑本段谷氨酰胺转胺酶(Transglutaminase,简称TGase)是一种硫醇酶,能够促使蛋白质分子内交联,蛋白质分子间交联以及蛋白质和氨基酸之间的交联。
TGase能够催化蛋白质中谷氨酰胺残基的γ-酰胺基和赖氨酸的ε-氨基之间进行酰胺基转移反应,形成ε-(γ-谷酰胺)-赖氨酸的异型肽键,从而改变蛋白质的功能性质。
TGase具有较好的pH稳定性,最适pH为6.0,但在pH5.0-8.0范围内该酶均有较高活性;同时TGase也具有较高的热稳定性,最适温度为50℃左右,在45-55℃都有较高活性。
杰森 斯坦森简介

杰森 斯坦森 --------
制作人:p13市场营销四班 王亚茹
基本信息
中文名:杰森·斯坦森 外文名:Jason Statham 别 名:贾森·斯塔森 国 籍:美国 星 座:狮子座 身 高:180cm 出生地:英国 出生日期:1967年7月26 日 职 业:演员,运动员 代表作品 《两杆大烟枪》、《敢死 队》
经典剧照
非常人贩
蜂 鸟
家园防线
机械师 敢死队
英国国家队跳水运动员、服 装模特、喜剧演员、动作巨 星~~所有这些看似八竿子打 不着的标签,都属于同一个 人,他就是堪称影坛“最成 功业余演员”的杰森·斯坦森。 他被誉为“最强动作演员”, 并且,你有没有发现,他一 直在“对抗着全世界“ 他拍摄过数不完的精彩电影: 玩命追踪、怒火攻心、死亡 飞车、暂告安全、敢死队、 铁血精英、波茨坦广场、非 常人贩……那么他为什被称 为“21世纪最强动作演员” 呢,大概有以下几个理由。杰森·斯坦森 主要作品
▪ 巴西任务 ▪ 帕克 ▪ 十三 ( 2007) ▪ 乱战 ( 2007) ▪ 游侠 ( 2007) ▪ 怒火攻心 ( 2006) ▪ 爆脾气 ( 2006) ▪ 轮转手枪 ( 2005) ▪ 非常人贩2 ( 2005) ▪ 转轮手枪 (2005) ▪ 借刀杀人 ( 2004) ▪ 意大利行动 2003) ▪ 偷天换日 ( 2003) ▪ 非常人贩 ( 2002) ▪ 救世主 ( 2001) ▪ 劣等阵容 ( 2001) 监狱风波 ( 2001) 火星幽灵 ( 2001) ▪ 偷拐抢骗 ( 2000) ▪ 两杆大烟枪 1998) ▪ 暂告安全 ( 2012) ▪ 玩命追踪 ( 2012) ▪ 波茨坦广场 ( 2012)▪ 敢死队2 ( 2012) ▪ 铁血精英 ( 2011) ▪ 机械师 ( 2010) ▪ 敢死队 ( 2010) ▪ 怒火攻心2 ( 2009) ▪ 死亡飞车 ( 2008)▪ 银行抢劫案 (2008) ▪ 非常人贩3 ( 2008) ▪ 地牢围攻 (2007)
2001年《中国射击射箭》第一期
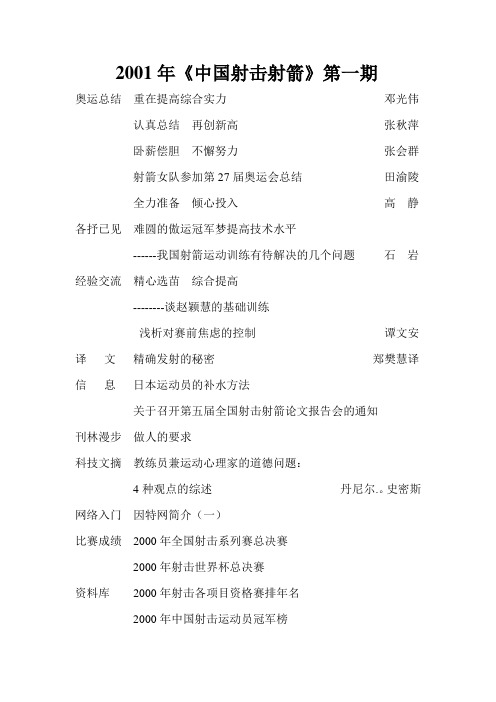
2001年《中国射击射箭》第一期奥运总结重在提高综合实力邓光伟认真总结再创新高张秋萍卧薪偿胆不懈努力张会群射箭女队参加第27届奥运会总结田渝陵全力准备倾心投入高静各抒已见难圆的傲运冠军梦提高技术水平------我国射箭运动训练有待解决的几个问题石岩经验交流精心选苗综合提高--------谈赵颖慧的基础训练浅析对赛前焦虑的控制谭文安译文精确发射的秘密郑樊慧译信息日本运动员的补水方法关于召开第五届全国射击射箭论文报告会的通知刊林漫步做人的要求科技文摘教练员兼运动心理家的道德问题:4种观点的综述丹尼尔.。
史密斯网络入门因特网简介(一)比赛成绩2000年全国射击系列赛总决赛2000年射击世界杯总决赛资料库2000年射击各项目资格赛排年名2000年中国射击运动员冠军榜2001年《中国射击射箭》第二期科研总结射击技术测试分析方法研究及其应用卢德明等明星访谈录我有足够的时间让梦想成真--------访奥运会冠军陶璐娜张秀芝译我想赢得更多的金牌------访奥运会冠军戴蒙德毛松华译浅谈教学的语言艺术秦国富经验交流关于手枪速射训练的几点思考盛浩明移动靶项目的压实稳入响击发技术初探李小江浅谈射击比赛中的信息回避赵贵生浅析赛前训练周期的安排张亚杰移动靶项目准备动作和接靶动作的训练体会申伟女子气手枪实弹基础训练的探讨马健译文立姿射击中腿的功用梁德清译精确发射的秘密郑樊慧译科技文摘悉尼奥运会射箭项目中运动心理学的运用金秉炫信息栏心理讲座制成VCD光盘科技服务信息网络入门因特网简介(二)赵万龙等刊林漫步写学相长比胜利更可怕的…..小辞典体育术语探源资料库2000年射击全国纪录2000年射击世界纪录中国射击运动员平、破纪录一览表2000年全国射击系列赛各项目总积分前八名2001《中国射击射箭》第三期本刊专访抓细抓实再创新高------访中国射击队领队孙盛伟一平经验交流试论我国步枪射击在国际上的位置张英洲参加第六届世界室内射箭锦标赛总结王冀中浅析射箭动作的流畅性谢江浅谈提高射击教练员素质的途径和方法高澍译文扣扳机过程毛松华译精确发射的秘密郑樊慧译科技文摘直接赛前准备德国训练学热点问题研究述评信息栏亚洲射击教练员培训班圆结束2001年全国射箭教练员学习班在莱芜举办心理讲座制成VCD光盘俄罗斯运动员备战2000年悉尼奥运会中的关键问题训练管理常抓不懈于海绢刊林漫步下一次就是你健身”怪”动作网络入门因特网简介(三)朱艺红比赛成绩全国射击系列赛(第一站)飞碟项目全国射击系列赛(第二站)暨锦标赛2001年亚特兰大射击世界杯赛成绩2001年慕尼黑射击世界杯赛成绩2001年韩国射击世界杯赛成绩2001年飞碟射击世界锦标赛赛成绩2001《中国射击射箭》第四期本刊采访九运会在即,冯建中主任细说------赛前准备8要素一平经验交流关于女子手枪项目速射训练的体会谢前乔射击运动与现代人的个性培养李浩坚从难从严是提高训练水平的重要保证高哲铭浅谈基层手枪慢射训练吴京试析青少年射击运动员的心理训练郭风娟注重情感交流积极促进训练张立新译文积极的自我交流方式董文瑾译立射中脚的位置毛松华译科技文摘优秀运动员大赛前竞技状态调控的时间学规律研石岩国外运动员心理训练特点及常用方法国外体育科研发展的几个趋势规则射击竞赛规则的修改、补充条文信息栏第九届全运会射击决赛席位一览表第九届全运会射箭决赛席位一览表小辞典让我们了解奥林匹克刊林漫步做事的辨证法人体妙趣多网络入门因特网简介(四)朱艺红等比赛成绩第十四届金箭大奖赛第九届全运会“亚奥特杯”射箭预赛世界杯飞碟射击比赛亚洲飞碟射击锦标赛全国重点射击学校射击比赛2001《中国射击射箭》第五期本刊采访一次成功的盛会一平九运会前夕,冯建中主任再谈-----赛场指挥艺术一平专家点评提高运动员的内适应水平是赛前心理工作的宗旨刘淑惠本刊视点中国与韩国射箭的差距在哪里?-----第41届世界射箭锦标赛观后感石岩等2004年奥运会射箭项目的发展与对策练国富永远告诉自己“我能行”杨建平经验交流飞碟射手的赛前训练与调控贺承旺明星访谈录取胜是我的目标,模拟训练是我训练的主要内容-----访意大利著名射箭运动员蒂帕郭显德译译文高水平运动训练周期性理论的新动向外部射击姿势-----上体部分毛松华译国际射联设立排名积分制于海娟译报刊文萃国际射联主席伊顿谈-----中国射箭运动面临发展良机中国射箭队取得历史性突破看准差距抓住契机中国射箭大飞跃中国射箭何时白步穿杨精心打造的盛会信息栏射箭教练员岗位培训教材正式出版外语学习系统设备在射运中心落成国际箭联裁判员会议在京召开郭蓓、张秀芝当选为国际箭联官员网络入门因特网简介(五)赵万龙等比赛成绩第41届射箭世界锦标赛成绩全国射击系列赛九运会热身赛成绩全国射箭锦标赛成绩射击世界杯总决赛成绩2001《中国射击射箭》第六期九运会专栏第九届全运会射击比赛调研报告射击调研组九届全运会射箭比赛调研报告射箭调研组关于我国射箭运动发展的几点思考------九运会男子射箭比赛观后感常静春从九运会射箭比赛看射箭运动的技术训练王碧瑶专家点评射击运动员成功路上心理的内适应刘淑慧经验交流关于对上海、浙江、江苏、山东四省射击项目现状的调查报告于海娟谈业务训练与文化学习的关系姚士坤浅谈运动焦虑对射箭成绩的影响张立新译文外部射击姿势-----上体毛松华卧薪尝胆超越自我蔡亚林战胜自我再圆冠军梦杨凌肯定自我找回自信单红难忘的经历赵颖慧信息栏2002年举办射击、射箭教练员岗位培训班关于再次征集射击、射箭科研论文的通知国家射击队、射箭队组队集训射箭教练员岗培教材已出版发行网络入门因特网简介(六)赵万龙等比赛成绩第九届全运会射击比赛成绩第九届全运会射箭比赛成绩。
2001年《中国射击射箭》第一期

2002年《中国射击射箭》第一期本刊专访2002:备战亚运会着眼奥运会------访射运中心主任助理郎维一平观察与思考射箭能成为2004、2008年奥运会我国金牌新的增长点吗?石岩经验交流谈在国家队执教的体会常静春关于步枪立姿训练方法的探讨原莹玫对射击专项化感知觉-----击发感的初探王建才运用激光测试分析系统对射击动作技术的分析研究宛春宁等明星访谈录我的理想是奥运会三连冠-----访悉尼奥运会女子个人冠军尹美进郭显德译译文双向射击的艺术朱萍译放松自己董文瑾译科技文摘优秀运动员大赛前竞技状态调控的时间学规律研究------中短期赛前训练阶段徐本力奥运小知识奥林匹克圣火起源的神话信息栏国际射击教练员培训班在慕尼黑结束于海娟译2002年射击比赛日程2002年射箭比赛日程射运春秋射箭的起源与演变人生感悟成功需要好习惯资料库2001年射击全国纪录2001年射击世界纪录2001年射击业余组全国纪录2001年射箭世界纪录2001年射箭全国纪录2001年奥林匹克淘汰赛全国纪录英语角过海关2002《中国射击射箭》第二期专题报告从乒乓球常胜不衰看教练员必须不断创新徐寅生经验交流借用“外脑”促进我国射击和射箭运动的改进与发展石岩我的教学理念郑青山浅谈射击运动员的心理障碍与矫正李军浅析射手的赛前准备张建伟等关于挖掘老队员潜力问题的讨论柴文斌九运会漫谈浅析射击运动员的赛前焦虑症余晓南正确地面对成功与失败-------赵颖慧九运会夺冠的启示李秀芳谈参加九运会比赛的收获李晖他山之石韩国射箭强大的基础译文双向射击的艺术朱萍译科技文摘优秀运动员大赛前竞技状态调控的时间学规律研究------中短期赛前训练阶段徐本力等对我国高水平射箭运动员体能训练的研究俸晓东等信息栏中国射箭项目发展委员会召开会议肖昊鹏中国射击队组成参加亚运会和世锦赛的初步队伍中国射箭队组成参加亚运会的基本队伍第九、十期全国射击教练员岗位培训班圆满结束心有灵犀差别虚掩的门.射坛春秋古代弓箭的演变比赛成绩2001射击世界杯总决赛(飞碟)刘希付译射击项目参加亚运会、世锦赛选拔赛成绩英语角取行李2002《中国射击射箭》第三期上海世界杯赛专刊实战磨砺总结提高经验交流浅析中韩射箭运动的差距与对策王友群浅谈心理训练在射击比赛中的重要性王荔军译文双向射击的艺术朱萍译科技文摘优秀运动员大赛前竞技状态调控的时间学规律------中短期赛前训练诸训练学因素的时间学特及总体时域调控模型的确定徐本力等专用规则奥运会射击运动项目运动员参赛的专用规则张秀芝译信息栏第四期全国射箭教练员岗位培训(高级、中级)班将举行刊林漫步感谢你的对手比赛成绩全国射击系列赛(第一站)飞碟项目全国射箭冠军赛成绩全国优秀射箭运动员大奖赛全国射击系列赛第二站(女子步枪)2002悉尼世界杯成绩2002年上海世界杯赛成绩2002年亚特兰大世界杯赛成绩英语角接人2002《中国射击射箭》第四期专题报告我国乒乓球常胜规律分析王鼎华综述射箭运动员块打节奏打法的心理学研究思考石岩经验交流脑电测试仪在射击射击训练中的运用赵洪峰等谈青少年女子手枪的规范化训练陈强浅谈运动员的奋斗目标李京龙明星访谈录韩国射箭-----朴敬来和吴教文答日本射箭运动员问郭显德译译文双向射击的艺术石璐译纲还是铅?石璐译科技文摘优秀运动员大赛前竞技状态调控的时间学规律------中短期赛前训练诸训练学因素的时间学特及总体时域调控模型的确定徐本力等亚运信息韩国釜山市简介釜山的交通设施状况比赛成绩全国射击系列赛成绩(第二站)射击世界杯成绩(飞碟)第48届世界射击锦标赛成绩英语角见面的问候语2002《中国射击射箭》第五期比赛总结中国射击队参加第48届世界锦标赛总结许海峰射击世锦赛的启示九运会前夕,冯建中主任再谈-----谈运动员良好习惯的形成赛场指挥艺术于吉平观察思考韩籍教练杨昌勋执教过程中尤为重视的几个问题万兴化经验交流关于运动员临场感觉的初步研究赵洪峰谈多向飞碟“快打”基本功训练方法观后感马进才从飞碟双多向技术分析看该项目的发展趋势王晓浅析射箭运动员心理定势的养成胡斌论立姿射击的果敢快打张志华以射箭训练工作为载体探索实施素质教育途径实验小学谈运用计算机辅助射击训练的体会孟勤浅谈射击运动员比赛前和比赛中的信息回避姚辉仿激光训练器在移动靶训练中的运用王荔军明星访谈录满环(韩国)选手-----李昌浩郭显德译科技文摘训练激励时机选择的策略信息栏“转基因运动员”幽灵初现-------科学家提出基因兴奋预警感悟人生杰里的选择我努力我快乐比赛成绩2002年亚洲飞碟射击锦标赛成绩2002年射击世界杯赛成绩2002年射击世界杯总决赛成绩2002《中国射击射箭》第六期亚会总结中国射击队参加第十四届亚运会总结经验交流男子步枪小口径项目与世界水平差距分析马军中心发火项目的慢射训练与手枪速射慢射训练的差异贺承德适应改革发展需要合理调整训练结构傅继亭调息在射击临场发挥中的应用王艳桃动态分析对飞碟双多向规则可能改变的分析孙盛伟译文双向射击的艺术石璐译高中时代是射箭运动员成长的重要时期郭显德译科技文摘时差反应与运动成绩感悟人生小事情就是大事情比赛成绩第十四届亚运会射击比赛成绩第十四届亚运会射箭比赛成绩2002年世界杯总决赛成绩(飞碟)全国射击系列赛(第三站)成绩全国青少年射击锦标赛成绩全国重点体校射箭锦标赛成绩全国射击系列赛总决赛暨冠军赛成绩全国射箭奥林匹克项目锦标赛成绩。
regularization paths for generalized linear models via coordinate descent

Stanford University
Trevor Hastie
Stanford University
Rob Tibshirani
Stanford University
Abstract We develop fast algorithms for estimation of generalized linear models with convex penalties. The models include linear regression, two-class logistic regression, and multinomial regression problems while the penalties include 1 (the lasso), 2 (ridge regression) and mixtures of the two (the elastic net). The algorithms use cyclical coordinate descent, computed along a regularization path. The methods can handle large problems and can also deal efficiently with sparse features. In comparative timings we find that the new algorithms are considerably faster than competing methods.
JSS
Journal of Statistical Software
January 2010, Volume 33, Issue 1. /
2001法国让·雷诺犯罪动作喜剧极速追杀令
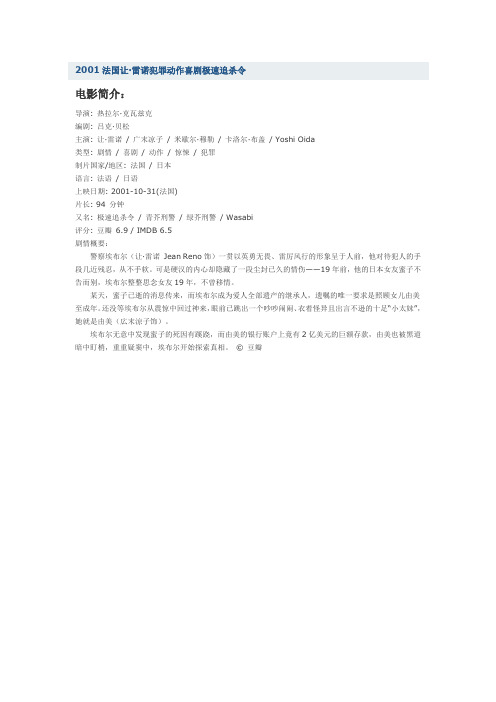
电影简介:
导演: 热拉尔·克瓦兹克
编剧: 吕克·贝松
主演: 让·雷诺/ 广末凉子/ 米歇尔·穆勒/ 卡洛尔·布盖/ Yoshi Oida
类型: 剧情/ 喜剧/ 动作/ 惊悚/ 犯罪
制片国家/地区: 法国/ 日本
语言: 法语/ 日语
上映日期: 2001-10-31(法国)
片长: 94 分钟
又名: 极速追杀令/ 青芥刑警/ 绿芥刑警/ Wasabi
评分: 豆瓣 6.9 / IMDB 6.5
剧情概要:
警察埃布尔(让·雷诺Jean Reno饰)一贯以英勇无畏、雷厉风行的形象呈于人前,他对待犯人的手段几近残忍,从不手软。
可是硬汉的内心却隐藏了一段尘封已久的情伤——19年前,他的日本女友蜜子不告而别,埃布尔整整思念女友19年,不曾移情。
某天,蜜子已逝的消息传来,而埃布尔成为爱人全部遗产的继承人,遗嘱的唯一要求是照顾女儿由美至成年。
还没等埃布尔从震惊中回过神来,眼前已跳出一个吵吵闹闹、衣着怪异且出言不逊的十足“小太妹”,她就是由美(広末涼子饰)。
埃布尔无意中发现蜜子的死因有蹊跷,而由美的银行账户上竟有2亿美元的巨额存款,由美也被黑道暗中盯梢,重重疑窦中,埃布尔开始探索真相。
© 豆瓣。
老人尿失禁的照护技巧TheTechniquesofCaring(精)
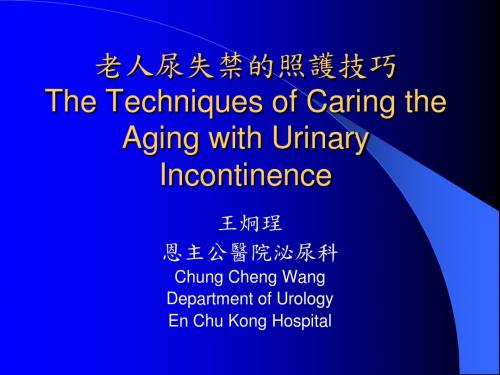
Behavioral Therapy
AHCPR guideline Bladder training: strongly recommended for urge and mixed incontinence and also recommended for stress UI Pelvic floor rehabilitation: strongly recommended for stress UI The first line of treatment [Fantl J 1996]
[Herzog AR 1990]
UI in the Frail Elderly
Frail: decline in physical activity [Bortx WM 2002] Frail elderly: >65, UI, can not go out without assistance, dementia, admitted to a long-term care facility [Fonda D 1998]
[Wyman JF 2000]
Nonpharmacologic Management of UI in Adults
Lifestyle or risk factors modification Scheduled voiding regimens Pelvic floor muscle rehabilitation Devices Supportive intervention
External Occlusive Device
A small single-use device that covers the urethral meatus for women A penile clamp for men Need good manual dexterity Complication: periurethral irritation or penile erosion
审计风云分集介绍
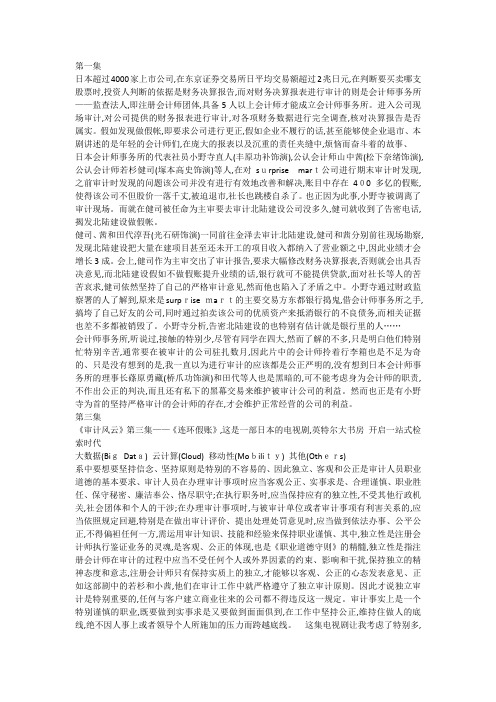
第一集日本超过4000家上市公司,在东京证券交易所日平均交易额超过2兆日元,在判断要买卖哪支股票时,投资人判断的依据是财务决算报告,而对财务决算报表进行审计的则是会计师事务所——监查法人,即注册会计师团体,具备5人以上会计师才能成立会计师事务所。
进入公司现场审计,对公司提供的财务报表进行审计,对各项财务数据进行完全调查,核对决算报告是否属实。
假如发现做假帐,即要求公司进行更正,假如企业不履行的话,甚至能够使企业退市、本剧讲述的是年轻的会计师们,在庞大的报表以及沉重的责任夹缝中,烦恼而奋斗着的故事、日本会计师事务所的代表社员小野寺直人(丰原功补饰演),公认会计师山中茜(松下奈绪饰演),公认会计师若杉健司(塚本高史饰演)等人,在对surprise mart公司进行期末审计时发现,之前审计时发现的问题该公司并没有进行有效地改善和解决,账目中存在400多亿的假账,使得该公司不但股价一落千丈,被迫退市,社长也跳楼自杀了。
也正因为此事,小野寺被调离了审计现场。
而就在健司被任命为主审要去审计北陆建设公司没多久,健司就收到了告密电话,揭发北陆建设做假帐。
健司、茜和田代淳吾(光石研饰演)一同前往金泽去审计北陆建设,健司和茜分别前往现场勘察,发现北陆建设把大量在建项目甚至还未开工的项目收入都纳入了营业额之中,因此业绩才会增长3成。
会上,健司作为主审交出了审计报告,要求大幅修改财务决算报表,否则就会出具否决意见,而北陆建设假如不做假账提升业绩的话,银行就可不能提供贷款,面对社长等人的苦苦哀求,健司依然坚持了自己的严格审计意见,然而他也陷入了矛盾之中。
小野寺通过财政监察署的人了解到,原来是surprise mart的主要交易方东都银行捣鬼,借会计师事务所之手,搞垮了自己好友的公司,同时通过拍卖该公司的优质资产来抵消银行的不良债务,而相关证据也差不多都被销毁了。
小野寺分析,告密北陆建设的也特别有估计就是银行里的人……会计师事务所,听说过,接触的特别少,尽管有同学在四大,然而了解的不多,只是明白他们特别忙特别辛苦,通常要在被审计的公司驻扎数月,因此片中的会计师拎着行李箱也是不足为奇的、只是没有想到的是,我一直以为进行审计的应该都是公正严明的,没有想到日本会计师事务所的理事长蓧原勇藏(桥爪功饰演)和田代等人也是黑暗的,可不能考虑身为会计师的职责,不作出公正的判决,而且还有私下的黑幕交易来维护被审计公司的利益。
谢诚刚 星光映佳集团创始人 董事长兼首席执行官 映佳国际
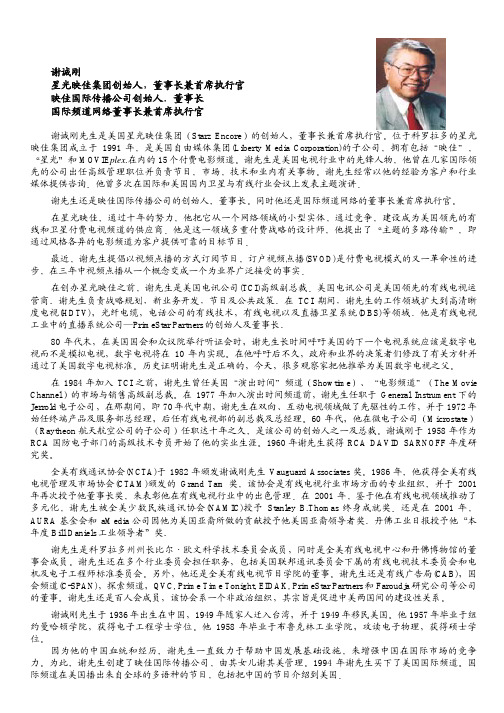
谢诚刚星光映佳集团创始人董事长兼首席执行官映佳国际传播公司创始人董事长国际频道网络董事长兼首席执行官谢诚刚先生是美国星光映佳集团Starz Encore的创始人董事长兼首席执行官位于科罗拉多的星光映佳集团成立于1991年是美国自由媒体集团(Liberty Media Corporation)的子公司拥有包括映佳星光和MOVIE plex.在内的15个付费电影频道谢先生是美国电视行业中的先锋人物他曾在几家国际领先的公司出任高级管理职位并负责节目市场技术和业内有关事物谢先生经常以他的经验为客户和行业媒体提供咨询他曾多次在国际和美国国内卫星与有线行业会议上发表主题演讲谢先生还是映佳国际传播公司的创始人董事长同时他还是国际频道网络的董事长兼首席执行官在星光映佳通过十年的努力他把它从一个网络领域的小型实体通过竞争建设成为美国领先的有线和卫星付费电视频道的供应商他是这一领域多重付费战略的设计师他提出了主题的多路传输即通过风格各异的电影频道为客户提供可靠的目标节目最近谢先生提倡以视频点播的方式订阅节目订户视频点播(SVOD)是付费电视模式的又一革命性的进步在三年中视频点播从一个概念变成一个为业界广泛接受的事实在创办星光映佳之前谢先生是美国电讯公司(TCI)高级副总裁美国电讯公司是美国领先的有线电视运营商谢先生负责战略规划新业务开发节目及公共政策在TCI期间谢先生的工作领域扩大到高清晰度电视(HDTV)光纤电缆电话公司的有线技术有线电视以及直播卫星系统(DBS)等领域他是有线电视工业中的直播系统公司—PrimeStar Partners的创始人及董事长80年代末在美国国会和众议院举行听证会时谢先生长时间呼吁美国的下一个电视系统应该是数字电视而不是模拟电视数字电视将在10年内实现在他呼吁后不久政府和业界的决策者们修改了有关方针并通过了美国数字电视标准历史证明谢先生是正确的今天很多观察家把他推举为美国数字电视之父在1984年加入TCI之前谢先生曾任美国演出时间频道Showtime电影频道The MovieChannel的市场与销售高级副总裁在1977年加入演出时间频道前谢先生任职于General Instrument下的Jerrold电子公司在那期间即70年代中期谢先生在双向互动电视领域做了先驱性的工作并于1972年始任终端产品及服务部总经理后任有线电视部的副总裁及总经理60年代他在微电子公司MicrostateRaytheon航天航空公司的子公司任职达十年之久是该公司的创始人之一及总裁谢诚刚于1958年作为RCA国防电子部门的高级技术专员开始了他的实业生涯1960年谢先生获得RCA DAVID SARNOFF年度研究奖全美有线通讯协会(NCTA)于1982年颁发谢诚刚先生Vauguard Associates奖1986年他获得全美有线电视管理及市场协会(CTAM)颁发的Grand Tam奖该协会是有线电视行业市场方面的专业组织并于2001年再次授予他董事长奖来表彰他在有线电视行业中的出色管理在2001年鉴于他在有线电视领域推动了多元化谢先生被全美少数民族通讯协会(NAMIC)授予Stanley B.Thomas终身成就奖还是在2001年AURA基金会和aMedia公司因他为美国亚裔所做的贡献授予他美国亚裔领导者奖丹佛工业日报授予他本年度Bill Daniels工业领导者奖谢先生是科罗拉多州州长比尔欧文科学技术委员会成员同时是全美有线电视中心和丹佛博物馆的董事会成员谢先生还在多个行业委员会担任职务包括美国联邦通讯委员会下属的有线电视技术委员会和电机及电子工程师标准委员会另外他还是全美有线电视节目学院的董事谢先生还是有线广告局(CAB)国会频道(C-SPAN)探索频道QVC, Prime Time Tonight, EIDAK, PrimeStar Partners和Faroudja研究公司等公司的董事谢先生还是百人会成员该协会系一个非政治组织其宗旨是促进中美两国间的建设性关系谢诚刚先生于1936年出生在中国1949年随家人迁入台湾并于1949年移民美国他1957年毕业于纽约曼哈顿学院获得电子工程学士学位他1958年毕业于布鲁克林工业学院攻读电子物理获得硕士学位因为他的中国血统和经历谢先生一直致力于帮助中国发展基础设施来增强中国在国际市场的竞争力为此谢先生创建了映佳国际传播公司由其女儿谢其美管理1994年谢先生买下了美国国际频道国际频道在美国播出来自全球的多语种的节目包括把中国的节目介绍到美国。
跨文化测量人格

跨文化(中國人)個性測量表CPAI-2引用格式﹕張妙清、梁覺、宋維真、張建新 (2001). 跨文化(中國人)個性測量表CPAI-2.(可向香港特別行政區,香港中文大學心理學系,張妙清教授申請使用) Citation Format: C heung, F. M., Leung, K., Song, W. Z., Zhang, J. X. (2001). The Cross-Cultural (Chinese) Personality Assessment Inventory-2 (CPAI-2). (Available from F. M.Cheung, Department of Psychology, The Chinese University of Hong Kong,Hong Kong SAR.)CPAI始創於九零年代,由香港中文大學張妙清教授、梁覺教授、中國科學院心理研究所宋維真教授、張建新博士、及張建平博士共同發展。
後經張妙清、梁覺、及張建新(以下簡稱作者)於2001年延續並重新進行標準化程序。
CPAI 及CPAI-1的版權歸作者所有。
CPAI包括與中國人文化有關的量表。
為建立CPAI 的研究基礎,作者鼓勵使用整套或部份CPAI量表作實驗性研究。
初版CPAI中,共有22個一般性格量表、12個臨床量表(其中一個臨床量表與一般性格量表重叠)、及三個效度量表。
有關建立CPAI的背景,請參考張妙清等(Cheung, et al., 1996)在Journal of Cross-Cultural Psychology刊登的文章,另張妙清等(Cheung, et al., 1998; 2001)的文章亦有描述CPAI文化相關的層面。
有關英文版的CPAI 及其標準化分數的發展,可分別參閱張妙清等及容耀輝等的文章 (Cheung, Cheung, et al.’s, 2003; Yung et al., 2000)。
不被重视或易忽视的:脑过度灌注

颈动脉重度狭窄,远端供血区低灌注
发病机制
远端动脉代偿性扩张以维持血供 长期极度扩张造成远端动脉自主调节功能耗竭
颅内血管对快速增加的CBF失去调节作用
局部高灌注造成脑水肿(血管源性)及出血
Canovas, et al. Angioplasty, Various Techniques and Challenges in Treatment of Congenital and Acquired Vascular Stenoses[book].2012,10-40. Moulakakis KG,et al. J Vasc Surg[J]. 2009,49:1060–1068 Ivens S, et al. J Neurol[J]. 2010,257:615–620。
注意:只看血管图容易误诊右侧缺血!
目前认为,术后脑血流速度较术前升高超过100%,则提示脑过度灌 注综合征。CT灌注成像(CTP)也有助于脑过度灌注综合征的诊断, Tseng等对比观察55例颈动脉支架成形术患者术前和术后CTP图像, 发现平均通过时间(MTT)与脑过度灌注综合征的发生明显相关。 平均通过时间的延长程度与颅内血管狭窄程度和脑血流量降低程度 呈正相关,脑血流量降低、平均通过时间延长和脑血容量(CBV) 轻度增加提示颅内血管扩张,脑血流自动调节机制受损,术后发生 脑过度灌注综合征的风险增加。
接下来再看看MRI图
图 2 头颅 CT,见左侧大脑半
球脑回肿胀、脑沟消失,相 图 3 头颅 CT,见左侧大
应实质呈高密度(红圈)
脑半球脑回肿胀、脑沟消失
图 4 头颅 CT,见左侧大脑半 球脑回肿胀、脑沟消失,左 大脑半球和左基底节区呈略 高密度(红圈)
图 5、6 头颅 MR FLAIR 序列,左侧大脑皮 层肿胀,呈高信号。
2001年世界电影票房排行

票房单位:百万排名标题制作全球票房国内票房% 海外票房%1 哈利波特与魔法石WB $974.8$317.6 32.6% $657.2 67.4%2 指环王:魔戒现身NL $869.3$313.4 36.0% $556.0 64.0%3 怪物公司BV $525.4$255.9 48.7% $269.5 51.3%4 怪物史莱克DW $484.4$267.7 55.3% $216.7 44.7%5 十一罗汉WB $450.7$183.4 40.7% $267.3 59.3%6 珍珠港BV $449.2$198.5 44.2% $250.7 55.8%7 木乃伊归来Uni. $433.0$202.0 46.7% $231.0 53.3%8 侏罗纪公园III Uni. $368.8$181.2 49.1% $187.6 50.9%9 人猿星球(2001)Fox $362.2$180.0 49.7% $182.2 50.3%10 汉尼拔MGM $351.7$165.1 46.9% $186.6 53.1%11 尖峰时刻2NL $347.3$226.2 65.1% $121.2 34.9%12 美丽心灵Uni. $313.5$170.7 54.5% $142.8 45.5%13 美国派2Uni. $287.6$145.1 50.5% $142.5 49.5%14 BJ单身日记Mira. $281.9$71.5 25.4% $210.4 74.6%15 古墓丽影Par. $274.7$131.2 47.7% $143.5 52.3%16 人工智能WB $235.9$78.6 33.3% $157.3 66.7%17 小岛惊魂Mira. $209.9$96.5 46.0% $113.4 54.0%18 速度与激情Uni. $207.3$144.5 69.7% $62.8 30.3%19 香草天空Par. $203.4$100.6 49.5% $102.8 50.5%20 猫狗大战WB $200.7$93.4 46.5% $107.3 53.5%21 亚特兰蒂斯:失落的帝国BV $186.1$84.1 45.2% $102.0 54.8%22 红磨坊Fox $179.2$57.4 32.0% $121.8 68.0%23 怪医杜立德2Fox $176.1$113.0 64.1% $63.2 35.9%24 天使爱美丽Mira. $173.9$33.2 19.1% $140.7 80.9%25 黑鹰坠落SonR $173.0$108.6 62.8% $64.4 37.2%26 公主日记BV $165.3$108.2 65.5% $57.1 34.5%27 非常小特务Mira. $147.9$112.7 76.2% $35.2 23.8%28 墨西哥人DW $147.8$66.8 45.2% $81.0 54.8%29 剑鱼行动WB $147.1$69.8 47.4% $77.3 52.6%30 间谍游戏Uni. $143.0$62.4 43.6% $80.7 56.4%31 律政俏佳人MGM $141.8$96.5 68.1% $45.3 31.9%32 惊声尖笑2Mira. $141.2$71.3 50.5% $69.9 49.5%33 庸人哈尔Fox $141.1$70.8 50.2% $70.2 49.8%34 美国甜心SonR $138.2$93.6 67.7% $44.6 32.3%35 留住最后一支舞Par. $131.7$91.1 69.1% $40.6 30.9%36 圣战骑士Sony $117.5$56.6 48.1% $60.9 51.9%37 大买卖/鬼计神偷Par. $113.6$71.1 62.6% $42.5 37.4%38 全面追缉令Par. $105.2$74.1 70.4% $31.1 29.6%39 训练日WB $104.9$76.6 73.1% $28.2 26.9%40 天才小子吉米Par. $103.0$80.9 78.6% $22.1 21.4%41 一言不发Fox $100.0$55.0 55.0% $45.0 45.0%42 进化DW $98.4$38.3 39.0% $60.0 61.0%43 我是山姆NL $97.8$40.3 41.2% $57.5 58.8%44 兵临城下Par. $97.0$51.4 53.0% $45.6 47.0%45 婚礼策划人Sony $94.7$60.4 63.8% $34.3 36.2%46 深入敌后Fox $91.8$58.9 64.1% $32.9 35.9%47 高斯福庄园USA $87.8$41.3 47.1% $46.4 52.9%48 拳王阿里Sony $87.7$58.2 66.4% $29.5 33.6%49 亡命寻宝Par. $85.5$56.6 66.2% $28.9 33.8%50 最终幻想:灵魂深处Sony $87.7$58.2 66.4% $29.5 33.6%。
2001年北美电影票房排行榜
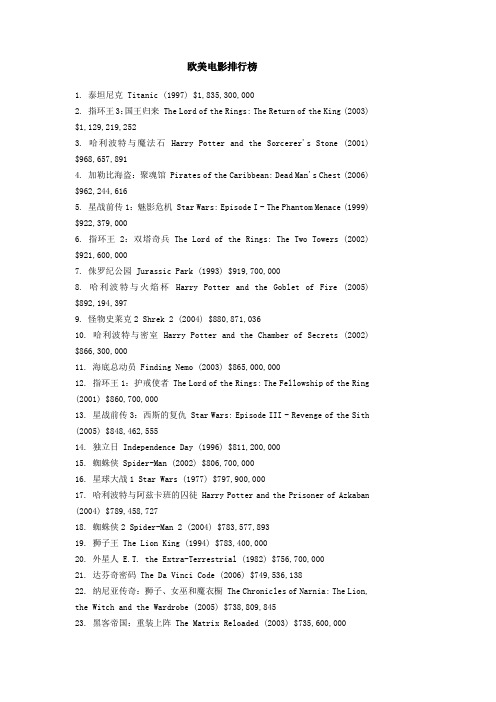
欧美电影排行榜1. 泰坦尼克 Titanic (1997) $1,835,300,0002. 指环王3:国王归来 The Lord of the Rings: The Return of the King (2003) $1,129,219,2523. 哈利波特与魔法石 Harry Potter and the Sorcerer's Stone (2001) $968,657,8914. 加勒比海盗:聚魂馆 Pirates of the Caribbean: Dead Man's Chest (2006) $962,244,6165. 星战前传1:魅影危机 Star Wars: Episode I - The Phantom Menace (1999) $922,379,0006. 指环王2:双塔奇兵 The Lord of the Rings: The Two Towers (2002) $921,600,0007. 侏罗纪公园 Jurassic Park (1993) $919,700,0008. 哈利波特与火焰杯Harry Potter and the Goblet of Fire (2005) $892,194,3979. 怪物史莱克2 Shrek 2 (2004) $880,871,03610. 哈利波特与密室 Harry Potter and the Chamber of Secrets (2002) $866,300,00011. 海底总动员 Finding Nemo (2003) $865,000,00012. 指环王1:护戒使者 The Lord of the Rings: The Fellowship of the Ring (2001) $860,700,00013. 星战前传3:西斯的复仇 Star Wars: Episode III - Revenge of the Sith (2005) $848,462,55514. 独立日 Independence Day (1996) $811,200,00015. 蜘蛛侠 Spider-Man (2002) $806,700,00016. 星球大战1 Star Wars (1977) $797,900,00017. 哈利波特与阿兹卡班的囚徒 Harry Potter and the Prisoner of Azkaban (2004) $789,458,72718. 蜘蛛侠2 Spider-Man 2 (2004) $783,577,89319. 狮子王 The Lion King (1994) $783,400,00020. 外星人 E.T. the Extra-Terrestrial (1982) $756,700,00021. 达芬奇密码 The Da Vinci Code (2006) $749,536,13822. 纳尼亚传奇:狮子、女巫和魔衣橱 The Chronicles of Narnia: The Lion, the Witch and the Wardrobe (2005) $738,809,84523. 黑客帝国:重装上阵 The Matrix Reloaded (2003) $735,600,00024. 阿甘正传 Forrest Gump (1994) $679,400,00025. 灵异第六感The Sixth Sense (1999) $661,500,00026. 加勒比海盗Pirates of the Caribbean: The Curse of the Black Pearl (2003) $653,200,00027. 星战前传2:克隆人的进攻 Star Wars: Episode II - Attack of the Clones (2002) $648,200,00028. 超人总动员The Incredibles (2004) $624,037,57829. 冰河世纪2:消融 Ice Age: The Meltdown (2006) $623,816,54230. 侏罗纪公园2:失落的世界 The Lost World: Jurassic Park (1997) $614,300,00031. 耶稣受难记 The Passion of the Christ (2004) $604,370,94332. 世界之战 War of the Worlds (2005) $591,377,05633. 黑衣人 Men in Black (1997) $587,200,00034. 星球大战3:绝地归来 Star Wars: Episode VI - Return of the Jedi (1983) $572,700,00035. 世界末日 Armageddon (1998) $554,600,00036. 金刚 King Kong (2005) $547,051,26037. 碟中碟2 Mission: Impossible II (2000) $545,300,00038. 小鬼当家 Home Alone (1990) $533,800,00039. 星球大战2:帝国反击 Star Wars: Episode V - The Empire Strikes Back (1980) $533,800,00040. 怪物公司 Monsters, Inc. (2001) $528,900,00041. 后天 The Day After Tomorrow (2004) $527,939,91942. 人鬼情未了Ghost (1990) $517,600,00043. 终结者2 Terminator 2: Judgment Day (1991) $516,800,00044. 阿拉丁 Aladdin (1992) $501,900,00045. 印第安娜琼斯之圣战骑兵 Indiana Jones and the Last Crusade (1989) $494,800,00046. 龙卷风 Twister (1996) $494,700,00047. 玩具总动员2 Toy Story 2 (1999) $485,700,00048. 特洛伊 Troy (2004) $481,228,34849. 拯救大兵瑞恩 Saving Private Ryan (1998) $479,300,00050. 查理和巧克力工厂Charlie and the Chocolate Factory (2005) $471,856,43151. 大白鲨 Jaws (1975) $470,600,00052. 漂亮女人 Pretty Woman (1990) $463,400,00053. 冒牌天神 Bruce Almighty (2003) $458,900,00054. 黑客帝国 The Matrix (1999) $456,300,00055. 角斗士 Gladiator (2000) $456,200,00056. 怪物史莱克 Shrek (2001) $455,100,00057. 碟中碟 Mission: Impossible (1996) $452,500,00058. 珍珠港 Pearl Harbor (2001) $450,400,00059. 11罗汉 Ocean's Eleven (2001) $444,200,00060. X战警3 X-Men: The Last Stand (2006) $439,745,74861. 最后的武士 The Last Samurai (2003) $435,400,00062. 人猿泰山 Tarzan (1999) $435,200,00063. 拜见岳父大人 Meet the Fockers (2004) $432,667,57564. 史密斯夫妇 Mr. & Mrs. Smith (2005) $427,936,10365. 黑衣人2 Men in Black II (2002) $425,600,00066. 007择日在死 Die Another Day (2002) $424,700,00067. 与狼共舞 Dances with Wolves (1990) $424,200,00068. 黑客帝国:矩阵革命 The Matrix Revolutions (2003) $424,000,00069. 荒岛余生 Cast Away (2000) $424,000,00070. 窈窕奶爸 Mrs. Doubtfire (1993) $423,200,00071. 木乃伊归来 The Mummy Returns (2001) $418,700,00072. 终结者3 Terminator 3: Rise of the Machines (2003) $418,200,00073. 木乃伊 The Mummy (1999) $413,300,00074. 蝙蝠侠 Batman (1989) $413,200,00075. 雨人 Rain Man (1988) $412,800,00076. 保镖 The Bodyguard (1992) $410,900,00077. 天兆 Signs (2002) $407,900,00078. 马达加斯加 Madagascar (2005) $406,800,00079. X战警2 X2 (2003) $406,400,00080. 汽车总动员 Cars (2006) $398,155,22281. 乱世佳人 Gone with the Wind (1939) $390,500,00082. 侠盗罗宾汉 Robin Hood: Prince of Thieves (1991) $390,500,00083. 碟中碟3 Mission: Impossible III (2006) $389,582,30984. 印第安娜琼斯之法归奇兵 Raiders of the Lost Ark (1981) $383,900,00085. 火爆浪子 Grease (1978) $379,800,00086. 冰河世纪 Ice Age (2002) $378,300,00087. 美女与野兽 Beauty and the Beast (1991) $378,300,00088. 哥斯拉 Godzilla (1998) $375,800,00089. 男人百分百 What Women Want (2000) $370,800,00090. 亡命天涯 The Fugitive (1993) $368,700,00091. 超人归来 Superman Returns (2006) $368,388,23792. 全民情敌 Hitch (2005/I) $367,600,00093. 真实的谎言 True Lies (1994) $365,200,00094. 虎胆龙威3:纽约大劫案Die Hard: With a Vengeance (1995) $365,000,00095. 诺丁山 Notting Hill (1999) $363,000,00096. 侏罗纪公园3 Jurassic Park III (2001) $362,900,00097. 我为马丽狂 There's Something About Mary (1998) $360,000,00098. 人猿星球 Planet of the Apes (2001) $358,900,00099. 石头城乐园 The Flintstones (1994) $358,500,000100. 玩具总动员 Toy Story (1995) $358,100,000其实我是受过高等教育的,只不过气质上比较农民一点而已。
美利达自行车推销方案
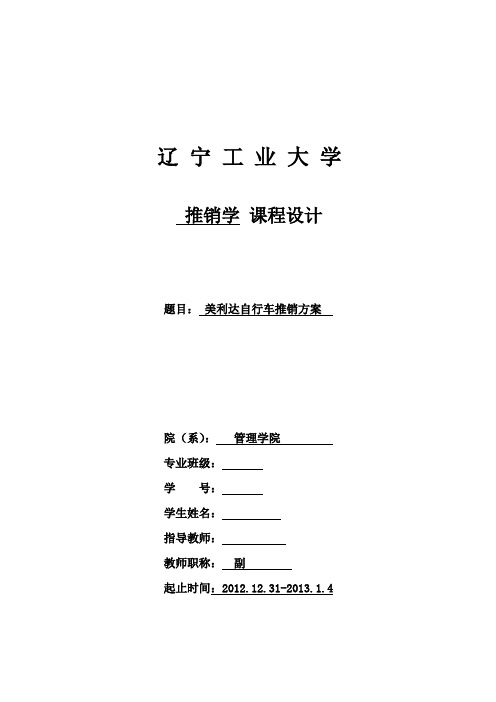
辽宁工业大学推销学课程设计题目:美利达自行车推销方案院(系):管理学院专业班级:学号:学生姓名:指导教师:教师职称:副起止时间:2012.12.31-2013.1.4课程设计任务及评语院(系):管理学院教研室:市场营销学号学生姓名专业班级课程设计(论文)题目美利达自行车推销方案课程设计的任务1.任务:◆掌握推销理论;◆掌握推销技巧;◆掌握推销的步骤;◆为某一具体产品进行推销设计。
2.具体要求:选择一种产品,根据其特点,运用所学推销理论和技巧,选择一种推销模式,进行整个推销过程的情景设计。
包括以下内容:◆寻找顾客根据所选产品,确定潜在顾客范围,选择2种以上寻找顾客的方法◆推销接近根据所选产品,确定接近准备的内容,在网上查找相关公司(潜在顾客),并选择推销接近方法(进行接近情景描述和推销员与顾客的对话描述)。
◆推销洽谈根据所选产品,确定推销洽谈的程序,包括准备的细节,洽谈的方法和策略(进行洽谈场景描述,必须有推销员与顾客的对话描述)。
◆推销定价根据所选产品,分析价格影响因素,确定定价策略,选择报价、让价方法。
◆异议处理根据所选产品,设计异议处理的方法和策略(必须有场景描述及推销员与顾客的对话描述)。
◆推销成交根据所选产品,设计场景,描述如何识别成交信号,选择提示成交的方法,应用促进成交的技巧(必须有场景描述及推销员与顾客的对话描述)。
指导教师评语及成绩成绩:指导教师签字:年月日目录1 美利达自行车 (1)1.1 简介 (1)1.2 发展历程 (2)2美利达(MERIDA)产品特点 (2)2.1碳纤维科技 (2)2.1.1美利达0.9焦点 (2)2.2弹性后叉 (3)2.3压缩车架几何 (3)2.3.1压缩车架的优势 (4)2.3.2压缩几何 (4)2.4 HFS液压成型系统 (4)3寻找顾客 (5)3.1网络搜寻法 (5)3.1.1运动员人群 (5)3.2广告拉动法 (6)3.2.1健身爱好人群 (6)3.2.2自行车健身成时尚 (6)3.2.3自行车健身组织完善 (6)4推销接近 (7)4.1吸引顾客的注意 (7)4.2产品接近法 (7)5推销洽谈 (9)5.1培育消费者的兴趣 (9)5.2激起消费者的购买欲望 (10)5.3产品演示法 (10)6异议处理 (10)6.1价格异议 (10)7推销成交 (11)7.1促成消费者采取购买行为 (11)参考文献 (13)1 美利达自行车1.1 简介美利达自行车(中国)有限公司,系台湾自行车业界中第一家股票上市公司--“美利达工业股份有限公司”在中国大陆独资的子公司,公司始建于1990年,总投资额1228万美元,占地面积11万平方米,拥有一流的自行车生产线,采取比JIS 、CPSC、BS更严格的质量标准生产,年产量可达180万台,所生产的高品质系列山地车、公路车、仿山地车、跑车等行销全球30多个国家和地区,以及国内各主要城市,并已形成高效率的营销系统。
- 1、下载文档前请自行甄别文档内容的完整性,平台不提供额外的编辑、内容补充、找答案等附加服务。
- 2、"仅部分预览"的文档,不可在线预览部分如存在完整性等问题,可反馈申请退款(可完整预览的文档不适用该条件!)。
- 3、如文档侵犯您的权益,请联系客服反馈,我们会尽快为您处理(人工客服工作时间:9:00-18:30)。
JOURNAL OF OPTIMIZATION THEORY AND APPLICATIONS:Vol.109,No.3,pp.475–494,JUNE2001 Convergence of a Block Coordinate DescentMethod for Nondifferentiable Minimization1P.T SENG2Communicated by O.L.MangasarianAbstract.We study the convergence properties of a(block)coordinatedescent method applied to minimize a nondifferentiable(nonconvex)function f(x1,...,x N)with certain separability and regularity proper-ties.Assuming that f is continuous on a compact level set,the sub-sequence convergence of the iterates to a stationary point is shown wheneither f is pseudoconvex in every pair of coordinate blocks from amongN A1coordinate blocks or f has at most one minimum in each of N A2coordinate blocks.If f is quasiconvex and hemivariate in every coordi-nate block,then the assumptions of continuity of f and compactness ofthe level set may be relaxed further.These results are applied to derivenew(and old)convergence results for the proximal minimization algo-rithm,an algorithm of Arimoto and Blahut,and an algorithm of Han.They are applied also to a problem of blind source separation.Key Words.Block coordinate descent,nondifferentiable minimization,stationary point,Gauss–Seidel method,convergence,quasiconvex func-tions,pseudoconvex functions.1.IntroductionA popular method for minimizing a real-valued continuously differen-tiable function f of n real variables,subject to bound constraints,is the (block)coordinate descent method.In this method,the coordinates are par-titioned into N blocks and,at each iteration,f is minimized with respect to one of the coordinate blocks while the other coordinates are heldfixed.This method,which is related closely to the Gauss–Seidel and SOR methods for equation solving(Ref.1),was studied early by Hildreth(Ref.2)and Warga (Ref.3),and is described in various books on optimization(Refs.1and4–1This work was partially supported by the National Science Foundation Grant CCR-9731273. 2Professor,Department of Mathematics,University of Washington,Seattle,Washington.4750022-3239͞01͞0600-0475$19.50͞0 2001Plenum Publishing Corporation476JOTA:VOL.109,NO.3,JUNE200110).Its applications include channel capacity computation(Refs.11–12), image reconstruction(Ref.7),dynamic programming(Refs.13–15),and flow routing(Ref.16).It may be applied also to the dual of a linearly constrained,strictly convex program to obtain various decomposition methods(see Refs.6–7,17–22,and references therein)and parallel SOR methods(Ref.23).Convergence of the(block)coordinate descent method requires typi-cally that f be strictly convex(or quasiconvex or hemivariate)differentiable and,taking into account the bound constraints,has bounded level sets(e.g., Refs.3–4and24–25).Zadeh(Ref.26;also see Ref.27)relaxed the strict convexity assumption to pseudoconvexity,which allows f to have a non-unique minimum along coordinate directions.For certain classes of convex functions,the level sets need not be bounded(see Refs.2,6–7,17,19–22, and references therein).If f is not(pseudo)convex,then an example of Powell(Ref.28)shows that the method may cycle without approaching any stationary point of f.Nonetheless,convergence can be shown for special cases of non(pseudo)convex f,as when f is quadratic(Ref.29),or f is strictly pseudoconvex in each of N A2coordinate blocks(Ref.27),or f has unique minimum in each coordinate block(Ref.8,p.159).If f is not differentiable, the coordinate descent method may get stuck at a nonstationary point even when f is convex(e.g.,Ref.4,p.94).For this reason,it is perceived generally that the method is unsuitable when f is nondifferentiable.However,an exception occurs when the nondifferentiable part of f is separable.Such a structure for f was consideredfirst by Auslender(Ref.4,p.94)in the case where f is strongly convex.This structure is implicit in a decomposition method and projection method of Han(Refs.18,30),for which f is the convex dual functional associated with a certain linearly constrained convex program(see Ref.22for detailed discussions).This structure arises also in least-square problems where an l1-penalty is placed on a subset of the para-meters in order to minimize the support(see Refs.31–33and references therein).Motivated by the preceding works,we consider in this paper the non-differentiable(nonconvex)case where the nondifferentiable part of f is sep-arable.Specifically,we assume that f has the following special form:Nf k(x k),(1)f(x1,...,x N)G f0(x1,...,x N)C∑k G1for some f0:ℜn1C···C n N>ℜ∪{S}and some f k:ℜn k>ℜ∪{S},k G 1,...,N.Here,N,n1,...,n N are positive integers.We assume that f is pro-per,i.e.,f͞≡S.We will refer to each x k,k G1,...,N,as a coordinate block of x G(x1,...,x N).We will show that each cluster point of the iterates generated by the(block)coordinate descent method is a stationary point ofJOTA:VOL.109,NO.3,JUNE2001477 f,provided that f0has a certain smoothness property(see Lemma3.1),f is continuous on a compact level set,and either f is pseudoconvex in every pair of coordinate blocks from among N A1coordinate blocks,or f has at most one minimum in each of N A2coordinate blocks(see Theorem4.1). If f is quasiconvex and hemivariate in every coordinate block,then the assumptions of continuity of f and compactness of the level set may be relaxed further(see Proposition5.1).These results unify and extend some previous results in Refs.4,6,8,26–27.For example,previous results assumed that f is pseudoconvex and that f1,...,f N are indicator functions for closed convex sets,whereas we assume only that f is pseudoconvex in every pair of coordinate blocks from among N A1coordinate blocks,with no additional assumption made on f1,...,f N.Previous results also did not consider the case where f is not continuous on its effective stly, we apply our results to derive new(and old)convergence results for the proximal minimization algorithm,an algorithm of Arimoto and Blahut (Refs.11–12),and an algorithm of Han(Ref.30);see Examples6.1–6.3. We also apply them to a problem of blind source separation described in Refs.31,33;see Example6.4.In our notation,ℜm denotes the space of m-dimensional real column vector.For any x,y∈ℜm,we denote by〈x,y〉the Euclidean inner product of x,y and by͉͉x͉͉the Euclidean norm of x,i.e.,͉͉x͉͉G1.For any set S⊆ℜm,we denote by int(S)the interior of S and denote bdry(S)G S\int(S).For any h:ℜm>ℜ∪{S},we denote by dom h the effective domain of h, i.e.,dom h G{x∈ℜm͉h(x)FS}.For any x∈dom h and any d∈ℜm,we denote the(lower)directional deriva-tive of h at x in the direction d byh′(x;d)G lim inf[h(x Cλd)A h(x)]͞λ.λ↓0We say that h is quasiconvex ifh(x Cλd)⁄max{h(x),h(x C d)},for all x,d andλ∈[0,1];h is pseudoconvex ifh(x C d)¤h(x),whenever x∈dom h and h′(x;d)¤0;see Ref.34,p.146;and h is hemivariate if h is not constant on any line segment belonging to dom h(Ref.1).For any nonempty I⊆{1,...,m},weJOTA:VOL.109,NO.3,JUNE 2001478say that h (x 1,...,x m )is pseudoconvex [respectively,has at most one mini-mum point].2.Block Coordinate Descent MethodWe describe formally the block coordinate descent (BCD)method below.BCD Method.Initialization.Choose any x 0G (x 01,...,x 0N )∈dom f .Iteration r C 1,r ¤0.Given x r G (x r 1,...,x r N )∈dom f ,choose an indexs ∈{1,...,N }and compute a new iteratex r C 1G (x r C 11,...,x r C 1N )∈dom fsatisfyingx r C 1s ∈arg min x s f (x r 1,...,x r s A 1,x s ,x r s C 1,...,x r N ),(2)x r C 1j G x r j ,∀j ≠s .(3)We note that the minimization in (2)is attained ifX 0G {x :f (x )⁄f (x 0)}is bounded and f is lower semicontinuous (lsc)on X 0,so X 0is compact (Ref.35).Alternatively,this minimization is attained if f is convex,has a minimum point,and is hemivariate in each coordinate block (but the level sets of f need not be bounded).To ensure convergence,we need further that each coordinate block is chosen suf ficiently often in the method.In particu-lar,we will choose the coordinate blocks according to the following rule (see,e.g.,Refs.7–8,21,25).Essentially Cyclic Rule.There exists a constant T ¤N such that every index s ∈{1,...,N }is chosen at least once between the r th iteration and the (r C T A 1)th iteration,for all r .A well-known special case of this rule,for which T G N ,is given below.Cyclic Rule.Choose s G k at iterations k ,k C N ,k C 2N ,...,for k G 1,...,N .JOTA:VOL.109,NO.3,JUNE2001479 3.Stationary Points of fWe say that z is a stationary point of f if z∈dom f andf′(z;d)¤0,∀d.We say that z is a coordinatewise minimum point of f if z∈dom f and f(z C(0,...,d k,...,0))¤f(z),∀d k∈ℜn k,(4) for all k G1,...,N.Here and throughout,we denote by(0,...,d k, 0the vector inℜn1C···C n N whose k th coordinate block is d k and whose other coordinates are zero.We say that f is regular at z∈dom f iff′(z;d)¤0,∀d G(d1,...,d N),such that f′(z;(0,...,d k,...,0))¤0,k G1,...,N.(5) This notion of regularity is weaker than that used by Auslender(Ref.4, p.93),which entailsNf′(z;(0,...,d k,...,0)),for all d G(d1,...,d N).f′(z;d)G∑k G1For example,the functionf(x1,x2)Gφ(x1,x2)Cφ(−x1,x2)Cφ(x1,−x2)Cφ(−x1,−x2),whereφ(a,b)G max{0,a C b A1a2C b2},is regular at z G(0,0)in the sense of(5),but is not regular in the sense of Ref.4,p.93.Since(4)impliesf′(z;(0,...,d k,...,0))¤0,for all d k,it follows that a coordinatewise minimum point z of f is a stationary point of f whenever f is regular at z.To ensure regularity of f at z,we consider one of the following smoothness assumptions on f0:(A1)dom f0is open and f0is Gaˆteaux-differentiable on dom f0.(A2)f0is Gaˆteaux-differentiable on int(dom f0)and,for every z∈dom f∩bdry(dom f0),there exist k∈{1,...,N}and d k∈ℜn ksuch that f(z C(0,...,d k,...,0))F f(z).JOTA:VOL.109,NO.3,JUNE2001480Assumption A1was considered essentially by Auslender(Ref.4, Example2on p.94).In contrast to Assumption A1,Assumption A2allows dom f0to include boundary points.We will see an application(Example 6.2)where A2holds but not A1.Lemma3.1.Under A1,f is regular at each z∈dom f.Under A2,f is regular at each coordinatewise minimum point z of f.Proof.Under A1,if z G(z1,...,z N)∈dom f,then z∈dom f0.Under A2,if z G(z1,...,z N)is a coordinatewise minimum point of f,then z∉bdry(dom f0),so z∈int(dom f0).Thus,under either A1or A2,f0is Gaˆteaux-differentiable at z.Fix any d G(d1,...,d N)such thatf′(z;(0,...,d k,...,0))¤0,k G1,...,N.Then,f′(z;d)G〈∇f0(z),d〉C lim infλ↓0∑Nk G1[f k(x k Cλd k)A f k(x k)]͞λ¤〈∇f(z),d〉C∑Nk G1lim infλ↓0[f k(x k Cλd k)A f k(x k)]͞λG〈∇f(z),d〉C∑Nk G1f′k(z k;d k)G∑Nk G1f′(z;(0,...,d k,...,0))¤0.ᮀ4.Convergence Analysis:IOurfirst convergence result unifies and extends a result of Auslender (Ref.4,p.95)for the nondifferentiable convex case and some results of Grippo and Sciandrone(Ref.27),Luenberger(Ref.8,p.159),and Zadeh (Ref.26)for the differentiable case.In what follows,r≡(N A1)mod N means r G N A1,2N A1,3N A1,....Theorem 4.1.Assume that the level set X0G{x:f(x)⁄f(x0)}is compact and that f is continuous on X0.Then,the sequence {x r G(x r1,...,x r N)}r G0,1,...generated by the BCD method using the essen-tially cyclic rule is defined and bounded.Moreover,the following statementsJOTA:VOL.109,NO.3,JUNE2001481 hold:(a)If f(x1,...,x N)is pseudoconvex in(x k,x i)for every i,k∈{1,...,N},and if f is regular at every x∈X0,then every clusterpoint of{x r}is a stationary point of f.(b)If f(x1,...,x N)is pseudoconvex in(x k,x i)for every i,k∈{1,...,N A1},if f is regular at every x∈X0,and if the cyclic ruleis used,then every cluster point of{x r}r≡(N A1)mod N is a stationarypoint of f.(c)If f(x1,...,x N)has at most one minimum in x k for k G2,...,N A1,and if the cyclic rule is used,then every cluster pointz of{x r}r≡(N A1)mod N is a coordinatewise minimum point of f.Inaddition,if f is regular at z,then z is a stationary point of f.Proof.Since X0is compact,an induction argument on r shows thatx r C1is defined,f(x r C1)⁄f(x r),and x r C1∈X0for all r G0,1,....Thus,{x r} is bounded.Consider any subsequence{x r}r∈R,with R⊆{0,1,...},con-verging to some z.For each j∈{1,...,T},{x r A T C1C j}r∈R is bounded,so bypassing to a subsequence,if necessary,we can assume that{x r A T C1C j}r∈R converges to some z j G(z j1,...,z j N),j G1,...,T. Thus,z T A1G z.Since{f(x r)}converges monotonically and f is continuous on X0,weobtain thatf(x0)¤limr→Sf(x r)G f(z1)G···G f(z T).(6)By further passing to a subsequence,if necessary,we can assume that the index s chosen at iteration r A T C1C j,j∈{1,...,T},is the same for all r∈R,which we denote by s j.For each j∈{1,...,T},since s j is chosen at iteration r A T C1C j for r∈R,then(2)and(3)yieldf(x r A T C1C j)⁄f(x r A T C1C j C(0,...,d s j,...,0)),∀d s j,j G1,...,T,x r A T C1C j k G x r A T C jk,∀k≠s j,j G2,...,T.Then,the continuity of f on X0yields in the limit thatf(z j)⁄f(z j C(0,...,d s j,...,0)),∀d s j,j G1,...,T,z j k G z j A1k ,∀k≠s j,j G2,...,T.(7)482JOTA:VOL.109,NO.3,JUNE2001Then,(6)and(7)yieldf(z j A1)⁄f(z j A1C(0,...,d s j,...,0)),∀d s j,j G2,...,T.(8)(a),(b)Suppose that f is regular at every x∈X0and that f(x1,...,x N) is pseudoconvex in(x k,x i)for every i,k∈{s1}∪···∪{s T A1}.This holds under the assumption(a)or under the assumption(b),with{x r}r∈R being any convergent subsequence of{x r}r≡(N A1)mod N.We claim that,for j G 1,...,T A1,f(z j)⁄f(z j C(0,...,d k,...,0)),∀d k,∀k G s1,...,s j.(9) By(7),(9)holds for j G1.Suppose that(9)holds for j G1,...,l A1for some l∈{2,...,T A1}.We show that(9)holds for j G l.From(8),we have that f(z l A1)⁄f(z l A1C(0,...,d s l,...,0)),∀d s l,implying,...,0))¤0.f′(z l A1;(0,...,z l s l A z l A1s lAlso,since(9)holds for j G l A1,we have that,for each k G s1,...,s l A1, f′(z l A1;(0,...,d k,...,0))¤0,∀d k.Since by(6)z l A1∈X0,so f is regular at z l A1,the above two relations imply f′(z l A1;(0,...,d k,...,0)C(0,...,z l s l A z l A1,...,0))¤0,∀d k.s lSince f is pseudoconvex in(x k,x s l),this yields[also using z l G z l A1C ,...,0)]for k G s1,...,s l A1that(0,...,z l s l A z l A1s lf(z l C(0,...,d k,...,0))¤f(z l A1)G f(z l),∀d k.Since we have also that(7)holds with j G l,we see that(9)holds for j G l. By induction,(9)holds for all j G1,...,T A1.Since z T A1G z and(9)holds for j G T A1,then(4)holds for k G s1,...,s T A1.Since z T A1G z and(8)holds(in particular,for j G T),then(4) holds for k G s T also.Since{1,...,N}G{s1}∪···∪{s T},this implies that z is a coordinatewise minimum point of f.Since f is regular at z,then z is in fact a stationary point of f.(c)Suppose that f(x1,...,x N)has at most one minimum in x k for k G s2,...,s T A1.This holds under the assumption(c),with{x r}r∈R being any convergent subsequence of{x r}r≡(N A1)mod N.For each j G2,...,T A1,sinceJOTA:VOL.109,NO.3,JUNE 2001483(7)and (8)hold,then the functiond s j >f (z j C (0,...,d s j ,...,0))attains its minimum at both d s j G 0and d s j G z j A 1s j A z j s j .By assumption,the minimum point is unique,implying 0G z j A 1s j A z j s j ,or equivalently,z j A 1Gz j .Thus,z 1G z 2G ···G z T A 1G z and (7)yields that (4)holds for k G s 1,...,s T A 1.Since z T A 1G z and (8)holds (in particular,for j G T ),then (4)holds for k G s T also.Since{1,...,N }G {s 1}∪···∪{s T },this implies that z is a coordinatewise minimum point of f .If f is regular at z ,then z is also a stationary point of f .ᮀNotice that,if f is pseudoconvex,then f is pseudoconvex in (x k ,x i )for every i ,k ∈{1,...,N };if f is quasiconvex and hemivariate in x k ,then f has at most one minimum in x k .The converses do not hold.For example,the 2-variable Rosenbrock function has a unique minimum point but is not quasiconvex.The following 3-variable quadratic functionf (x 1,x 2,x 3)G (1͞2)x 21C (1͞2)x 22C (1͞2)x 23C x 1x 3C x 2x 3A x 1x 2is convex in every pair of variables,but is not pseudoconvex.In particular,for x G (0,0,1͞2)and d G (1,1,−1),we have f ′(x ;d )G 1͞2¤0,while f (x C d )G −7͞8F f (x )G 1͞8.This example generalizes to any quadratic functionf (x )G 〈x ,Qx 〉.where Q ∈R N B N is symmetric,not positive semide finite,but whose 2B 2principal submatrices are positie semide finite.Then,for any d satisfying 〈d ,Qd 〉F 0and any x satisfying0⁄〈x ,Qd 〉F −(1͞2)〈d ,Qd 〉,we have thatf ′(x ;d )¤0,while f (x C d )F f (x ).Thus,parts (a)and (c)of Theorem 4.1may be viewed as extensions of two results of Grippo and Sciandrone (Ref.27,Propositions 5.2,5.3)for the case of f 0being continuously differentiable and each f k being the indicator function of some closed convex set.In turn,the first of these results extended a result of Zadeh (Ref.26)for which f k ≡0for all k .Part (b)makes a less restrictive assumption on f than part (a),though its assumption on the BCD method is more restrictive.Part (b)is sharp in the sense that it is false if instead we assume that f is convex in every coordinate block.This484JOTA:VOL.109,NO.3,JUNE2001is because the Powell3-variable example(Ref.28)is convex in each variable; see Ref.27,Section6for further discussions of the example.We will see an application(Example6.4)in which part(b)applies but not part(a)nor(c).5.Convergence Analysis:IIThe convergence analysis of the previous section assumes f to be con-tinuous on a bounded level set and makes no use of the special structure(1) of f.In this section,we show that this assumption can be relaxed by exploiting the special structure(1),provided that f is quasiconvex and hemi-variate in each coordinate block.More precisely,we will make the following assumptions on f,f0,f1,...,f N:(B1)f0is continuous on dom f0.(B2)For each k∈{1,...,N}and(x j)j≠k,the function x k> f(x1,...,x N)is quasiconvex and hemivariate.(B3)f0,f1,...,f N are lsc.We will see some applications(Ref.6,Section3.4.3and Examples6.1–6.3)for which f satisfies this weaker assumption although it is not strictly convex.In addition,we will make one of the following technical assump-tions on f0:(C1)dom f0is open and f0tends to S at every boundary point of dom f0.(C2)dom f0G Y1B···B Y N,for some Y k⊆R n k,k G1,...,N.In contrast to Assumption C1,Assumption C2allows f0to have afinite value on bdry(dom f).We will see in Example6.2a nonseparable function f0that satisfies Assumptions B1–B3and C2,but not C1.We show below that Assumptions B1–B3,together with either Assumption C1or C2,ensure that every cluster point of the iterates generated by the BCD method is a coordinate minimum point of f.The proof of this result is patterned after an argument given by Bertsekas and Tsitsiklis(Ref.6,pp.220–221;also see Ref.27),but is complicated by the fact that f is not necessarily differentiable (or even continuous)on its effective domain.Proposition5.1.Suppose that f,f0,f1,...,f N satisfy Assumptions B1–B3and that f0satisfies either Assumption C1or C2.Also,assume that the sequence{x r G(x r1,...,x r N)}r G0,1,...generated by the BCD method using the essentially cyclic rule is defined.Then,either{f(x r)}↓−S,or else every cluster point z G(z1,...,z N)is a coordinatewise minimum point of f.Proof.Since f(x0)FS and f(x r C1)⁄f(x r)for all r,then either {f(x r)}↓−S,or else{f(x r)}converges to some limit and{f(x r C1)A f(x r)}→0.Consider the latter case and let z be any cluster point of{x r}. Since f is lsc by Assumption B3,we havef(z)⁄limr→Sf(x r)FS,so z∈dom f.We show below that z satisfies(4)for k G1,...,N.First,we claim that,for any infinite subsequence{x r}r∈R→z,(10) with R⊆{0,1,...},there holds that(x r C1}r∈R→z.(11) We prove this by contradiction.Suppose that this were not true.Then,there exists an infinite subsequence R′of R and a scalar(H0such that ͉͉x r C1A x r͉͉¤(,for all r∈R′.By further passing to a subsequence,if necessary,we can assume that there is some nonzero vector d for which{(x r C1A x r)͉͉͞x r C1A x r͉͉}r∈R′→d,(12) and that the same coordinate block,say x s,is chosen t the(r C1)st iteration for all r∈R′.Moreover,(10)implies that{f0(x r)}r∈R and{f k(x r k)}r∈R,k G 1,...,N,are bounded from below,which together with the convergence of{f(x r)}G{f0(x r)C∑Nk G1f k(x r k)}implies that{f0(x r)}r∈R and{f k(x r k)}r∈R,k G1,...,N,are bounded.Hence,by further passing to a subsequence,if necessary,we can assume that there is some scalarθfor which{f0(x r)C f s(x r s)}r∈R′→θ.(13) Fix anyλ∈[0,(].LetzˆG z Cλd,(14) and for each r∈R′,letxˆr G x r Cλ(x r C1A x r)͉͉͞x r C1A x r͉͉.(15) Then,by(10),(12),and(14),{xˆr}r∈R′→zˆ.(16) For each r∈R′,we see from(2)that x r C1is obtained from x r by minimizing f with respect to x s,while the other coordinates are heldfixed.Sinceλ͉͉͞x r C1A x r͉͉⁄λ͞(⁄1,so xˆr lies on the line segment joining x r with x r C1,this together with f(x r C1)⁄f(x r)and the quasiconvexity of x s>f(x r1,...,x r s A1,x s, x r s C1,...,x r N)impliesf(xˆr)⁄f(x r),∀r∈R′.Since f is lsc,this and(16)imply zˆ∈dom f.Also,this and(1)and the obser-vation that x r and xˆr differ only in their s th coordinate block imply f0(xˆr)C f s(xˆr s)⁄f0(x r)C f s(x r s),∀r∈R′.This combined with(13)yieldslimr→S,r∈R′sup{f0(xˆr)C f s(xˆr s)}⁄θ.(17) Also,since{f(x r C1)A f(x r)}r∈R′→0,we have equivalently that{f0(x r C1)C f s(x r C1s)A f0(x r)A f s(x r s)}r∈R′→0,so(13)implies{f0(x r C1)C f s(x r C1s)}r∈R′→θ.(18) LetδG f0(zˆ)C f s(zˆs)Aθ.Since f0and f s are lsc,we have from(16),(17)thatδ⁄0.We claim that in factδG0.Suppose that this were not true,so thatδH0.By(16)and the observation that,for all r∈R′,xˆr and x r differ in only their s th coordinate block,we have{(x r1,...,x r s A1,zˆs,x r s C1,...,x r N)}r∈R′→zˆ.(19) Moreover,the vector on the left-hand side of(19)is in dom f0for all r∈R′sufficiently large.Since zˆ∈dom f0,this is certainly true under Assumption C1;under Assumption C2,this is also true because x r∈dom f0for all r and dom f0has a product structure corresponding to the coordinate blocks. Then,(18)together with(19)and the continuity of f0on dom f0implies that,for all r∈R′sufficiently large,there holds thatf0(x r1,...,x r s A1,zˆs,x r s C1,...,x r N)C f s(zˆs)⁄f0(x r C1)C f s(x r C1s)Cδ͞2,or equivalently[via(1)and the observation that x r and x r C1differ in only their s th coordinate block],f(x r1,...,x r s A1,zˆs,x r s C1,...,x r N)⁄f(x r C1)Cδ͞2,a contradiction to the fact that x r C1is obtained from x r by minimizing f with respect to the s th coordinate block,while the other coordinates are heldfixed.Hence,δG0and thereforef0(zˆ)C f s(zˆs)Gθ.Since the choice ofλwas arbitrary,we obtain[also using(14)]f0(z Cλd)C f s(z s Cλd s)Gθ,∀λ∈[0,(],where d s denotes the s th coordinate block of d.Since x r and x r C1differ in only their s th coordinate block for all r∈R′,then all coordinate blocks of d,except d s,are zero[see(12)],and the above relation,together with(1), shows that f(z Cλd)is constant(andfinite)for allλ∈[0,(],a contradiction to Assumption B2,namely,that f is hemivariate in the s th coordinate block. Hence,(11)holds.Since(11)holds for any subsequence{x r}r∈R of{x r}converging to z, we can apply(11)to the subsequence{x r C1}r∈R to conclude that {x r C2}r∈R→z and so on,yielding{x r C j}r∈R→z,∀j G0,1,...,T,(20) where T is the bound specified in the essentially cyclic rule.We claim that(20),together with Assumption C1or C2,implies f0(z)C f k(z k)⁄f0(z1,...,z k A1,x k,z k C1,...,z N)C f k(x k),(21) for all x k and all k∈{1,...,N}.To see this,fix any k∈{1,...,N}.Since the coordinate blocks are chosen according to the essentially cyclic rule, there exists some j∈{1,...,T}and an infinite subsequence R′⊆R such that the coordinate block x k is chosen at the(r C j)th iteration for all r∈R′.Then,for each r∈R′,x r C j k minimizes f0(x r C j1,...,x r C j k A1,x k, x r C j k C1,...,x r C j N)C f k(x k)over all x k[see(1),(2),(3)],so thatf0(x r C j)C f k(x r C j k)(x r C j1,...,x r C j k A1,x k,x r C j k C1,...,x r C j N)C f k(x k),∀x k.(22)⁄fFix any x k∈dom f k such that(z1,...,z k A1,x k,z k C1,...,z N)∈dom f0. Suppose that Assumption C1holds,so dom f0is open.Since z∈dom f0,then(20)implies that(x r C j1,...,x r C j k A1,x k,x r C j k C1,...,x r C j N)∈dom f0,for all r∈R′sufficiently large. Passing to the limit as r→S,r∈R′,and using the lsc property of f k and the continuity of f0on the open set dom f0,we obtain from(20) and(22)that(21)holds.Suppose instead that Assumption C2holds,sodom f0G Y1B···B Y N,for some Y1⊆ℜn1,...,Y N⊆ℜn N.Then,thefirst quantity on the right-hand side of(22)isfinite for all r∈ℜ′.Passing to the limit as r→S,r∈ℜ′,and using the lsc property of f k and the continuity of f0on dom f0,we obtain from(20)and(22)that (21)holds.If x k∉dom f k or(z1,...,z k A1,x k,z k C1,...,z N)∉dom f0,then the right-hand side of(21)has the extended value S,so(21)holds trivially.Since the above choice of k was arbitrary,this shows that(21) holds for all x k and all k∈{1,...,N}.Then,it follows from(1)that(4) holds for all k G1,...,N.ᮀProposition5.1extends a result of Grippo and Sciandrone(Ref.27, Proposition5.1)for the special case where each f k is the indicator func-tion for some closed convex set and f0is continuously differentiable and (block)coordinatewise strictly pseudoconvex.In turn,the latter result is an extension of a result of Bertsekas and Tsitsiklis(Ref.6,Proposition 3.9in Section3.3.5),which assumes further f0to be convex.As a cor-ollary of Proposition5.1,we obtain the following convergence result for the BCD method.Theorem5.1.Suppose that f,f0,f1,...,f N satisfy Assumptions B1–B3and that f0satisfies either Assumption C1or C2.Also,assume that {x:f(x)⁄f(x0)}is bounded.Then,the sequence{x r}generated by the BCD method using the essentially cyclic rule is defined,bounded,and every cluster point is a coordinatewise minimum point of f.Theorem5.1extends a result of Auslender[see Theorem1.2(a)in Ref. 4,p.95]for the special case where f k is convex for all k,dom f0G Y1B···B Y N for some closed convex sets Y k⊆ℜn k,k G1,...,N,and f0is strongly convex and continuous on dom f0.6.ApplicationsWe describe four interesting applications of the BCD method below. In all applications,the objective function f is not necessarily strictly convex nor differentiable everywhere on its effective domain.Example6.1.Proximal Minimization Algorithm.Letψ:ℜn>ℜ∪{S}be a proper(i.e.,ψ͞≡S)lsc function.Fix any scalar c H0,and consider the proper lsc function f defined byf(x,y)G c͉͉x A y͉͉2Cψ(x).Clearly,this function has the form(1)withf0(x,y)G c͉͉x A y͉͉2,f1Gψ,f2≡0.Applying the BCD method to f yields a method whereby f(x,y)is alter-nately minimized with respect to x and y.This method has the form x r C1G arg minc͉͉x A x r͉͉2Cψ(x),r G0,1,...,xwhich is the proximal minimization algorithm withfixed parameter c for minimizingψ;see Ref.6,Section3.4.3and Refs.36–37and references therein.It is easily seen that f,f0,f1,f2satisfy Assumptions B1–B3and that f0 satisfies Assumptions A1and C1.Moreover,f is regular everywhere on dom f.Then,by Proposition5.1,ifψis bounded below(so,f is bounded below),then every cluster point z of the iterates generated by the above proximal minimization algorithm is a stationary point ofψ,i.e.,ψ′(z;d)¤0,for all d.Notice that Theorem4.1is not applicable here,since f need not be continu-ous on its level sets.Example6.2.Arimoto–Blahut Algorithm.Let P ij,i G1,...,n,j G 1,...,m,be given nonnegative scalars satisfyingP ij G1,for all i.∑jThe P ij may be viewed as probabilities.Consider the proper lsc function f defined byf(x,y)G f0(x,y)C f1(x)C f2(y),。