Policy Learning by GA using Importance Sampling
基于ga-elm模型的我国天然气进口预测
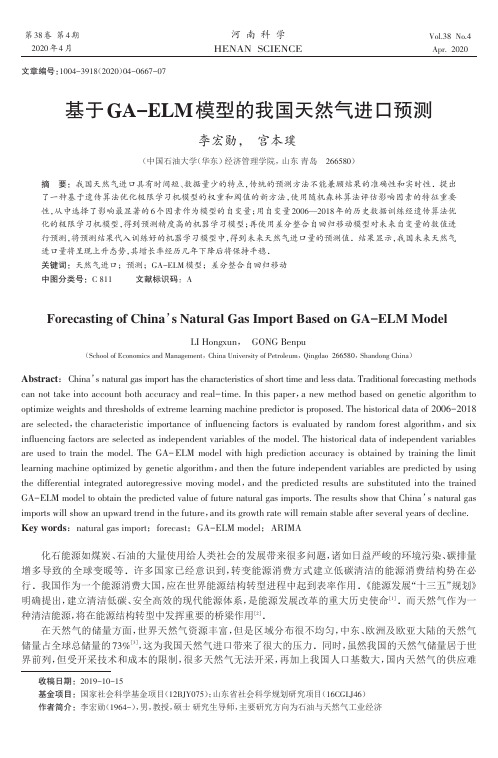
收稿日期:2019-10-15基金项目:国家社会科学基金项目(12BJY075);山东省社会科学规划研究项目(16CGLJ46)作者简介:李宏勋(1964-),男,教授,硕士研究生导师,主要研究方向为石油与天然气工业经济基于GA-ELM 模型的我国天然气进口预测李宏勋,宫本璞(中国石油大学(华东)经济管理学院,山东青岛266580)摘要:我国天然气进口具有时间短、数据量少的特点,传统的预测方法不能兼顾结果的准确性和实时性.提出了一种基于遗传算法优化极限学习机模型的权重和阈值的新方法,使用随机森林算法评估影响因素的特征重要性,从中选择了影响最显著的6个因素作为模型的自变量;用自变量2006—2018年的历史数据训练经遗传算法优化的极限学习机模型,得到预测精度高的机器学习模型;再使用差分整合自回归移动模型对未来自变量的数值进行预测,将预测结果代入训练好的机器学习模型中,得到未来天然气进口量的预测值.结果显示,我国未来天然气进口量将呈现上升态势,其增长率经历几年下降后将保持平稳.关键词:天然气进口;预测;GA-ELM 模型;差分整合自回归移动中图分类号:C 811文献标识码:AForecasting of China ’s Natural Gas Import Based on GA-ELM ModelLI Hongxun ,GONG Benpu(School of Economics and Management ,China University of Petroleum ,Qingdao 266580,Shandong China )Abstract :China ’s natural gas import has the characteristics of short time and less data.Traditional forecasting methods can not take into account both accuracy and real-time.In this paper ,a new method based on genetic algorithm to optimize weights and thresholds of extreme learning machine predictor is proposed.The historical data of 2006-2018are selected ,the characteristic importance of influencing factors is evaluated by random forest algorithm ,and six influencing factors are selected as independent variables of the model.The historical data of independent variables are used to train the model.The GA-ELM model with high prediction accuracy is obtained by training the limit learning machine optimized by genetic algorithm ,and then the future independent variables are predicted by using the differential integrated autoregressive moving model ,and the predicted results are substituted into the trainedGA-ELM model to obtain the predicted value of future natural gas imports.The results show that China ’s natural gasimports will show an upward trend in the future ,and its growth rate will remain stable after several years of decline.Key words :natural gas import ;forecast ;GA-ELM model ;ARIMA化石能源如煤炭、石油的大量使用给人类社会的发展带来很多问题,诸如日益严峻的环境污染、碳排量增多导致的全球变暖等.许多国家已经意识到,转变能源消费方式建立低碳清洁的能源消费结构势在必行.我国作为一个能源消费大国,应在世界能源结构转型进程中起到表率作用.《能源发展“十三五”规划》明确提出,建立清洁低碳、安全高效的现代能源体系,是能源发展改革的重大历史使命[1].而天然气作为一种清洁能源,将在能源结构转型中发挥重要的桥梁作用[2].在天然气的储量方面,世界天然气资源丰富,但是区域分布很不均匀,中东、欧洲及欧亚大陆的天然气储量占全球总储量的73%[3],这为我国天然气进口带来了很大的压力.同时,虽然我国的天然气储量居于世界前列,但受开采技术和成本的限制,很多天然气无法开采,再加上我国人口基数大,国内天然气的供应难第38卷第4期河南科学2020年4月以满足天然气的需求.在天然气进口量方面,2010年我国天然气进口量为165亿m 3,2018年增长到1215亿m 3,是2010年的7倍多;天然气进口依存度也从2010年的15.3%增长到2018年的42.9%[4].国际能源署(IEA )发布的《2018天然气分析及预测报告》对未来五年全球天然气市场的发展进行了预测,预计2019年中国将成为世界最大的天然气进口国[5].在天然气的能源消费占比方面,2018年中国天然气消费占一次能源消费的比例为7.4%,与世界平均水平(24%)相比我国天然气占一次能源消费的比例还很低,未来天然气的国内需求还有很大的增长潜力[4].综上所述,进口天然气对于满足国内天然气的需求就显得至关重要.1文献回顾通过查阅现有相关文献资料,笔者从天然气预测指标、天然气预测方法和天然气进口研究现状三个方面对现有文献进行梳理.天然气预测指标体系的构建是一个不断丰富发展的过程.在完善的预测指标体系尚未建立时,Li 等运用情景分析的方法分析了中国各部门天然气的消费现状和趋势变化情况,认为GDP 和人口数量是天然气消费的主要影响因素[6],认为GDP 和总人口是中国天然气消费量的核心影响因素,在对天然气消费量进行系统的分析时必须考虑这两个因素的变化.李君臣等的研究为天然气预测指标的后续发展奠定了重要基础,后来的研究也都将经济发展水平和人口作为天然气需求的重要影响因素[7].Zhang 等认为影响中国天然气消费的因素包括GDP 、城镇化率、能源效率、能源消费结构、产业结构和商品的出口贸易[8].Chai 等通过实证研究,确定了天然气消费与影响因素之间的关系,使用LMDI 分解影响因素,将影响因素分为经济发展指标和清洁指标[9].目前为止,天然气的预测指标主要可以划分为四类:经济发展指标、人口指标、工业指标和能源消费指标.传统的天然气预测采用的方法以灰色模型、Hubbert 模型和OLS 模型等方法为主[10~12].比如,Lifeng 等使用一种新型灰色系统模型,基于不完整的信息,通过对较近的数据分配较高的权重来提升灰色系统模型的预测精度,并使用这种改进灰色模型预测了2014—2018年中国的天然气消费量[13];Zhang 等认为贝叶斯模型平均法可以计算后验概率,能够解决模型的不确定性问题,且比灰色预测模型和人工神经网络等常用模型有更好的预测效果,并使用贝叶斯模型平均法预测了中国天然气的消费量[8];Shaikh 等通过对数几率回归分析预测了中国天然气需求[14].这些传统的预测方法能够较好地解释变量与结果之间的关系,在以往的天然气预测研究中得到了广泛的应用.传统的预测方法对变量之间以及变量和结果之间的关系具有较好的解释性,但是预测的精度较低,并且需要大量的数据样本作为支撑.随着人工智能的兴起,越来越多的智能算法被用在天然气预测的研究中.智能算法与传统的预测方法相比,具有预测精度高、自适应和动态学习的优点,更加适用于动态的能源需求预测.在智能算法用于天然气预测的初期,使用的模型大多是BP 神经网络模型或支持向量机模型等单一算法模型,如Szoplik 等使用神经网络模型[15]、Bai 等使用支持向量机[16]分别建立了天然气消费预测模型来预测短期内天然气的消费量;随着智能算法在预测领域应用的日益广泛,国内外学者们发现将不同的算法之间进行组合优化能够得到更精确的预测结果,如Deyun 等构建了基于粒子群优化算法和小波神经网络(PSO-WNN )的混合预测模型,利用PSO 算法优化初始权重和小波参数,并通过更新动态学习率提高训练速度和预测准确性,并减少了WNN 波动,分析了影响我国天然气消费的主要因素[17];Karadede 等使用种群遗传算法和基于非线性回归的种子混合算法的模拟退火算法,以非常小的误差预测了天然气消费量[18];De 等对传统的AdaBoost 算法进行改进,使用PSO 算法对ELM 模型的输入权值和阈值进行优化,并以此作为AdaBoost的弱预测器,构建AdaBoost-PSO-ELM 模型,预测了未来十年我国天然气需求量[19].在天然气进口研究方面,近几年进口天然气在我国天然气消费中占有很高的比重,目前针对我国天然气进口业务的研究大多集中在天然气进口的空间布局、价格研究以及供应安全等方面.例如,孙聆轩等综合运用多个空间布局指标,定量分析了我国天然气进口空间格局的演进及优化情况,发现我国天然气进口空--668引用格式:李宏勋,宫本璞.基于GA-ELM 模型的我国天然气进口预测[J ].河南科学,2020,38(4):667-673.间布局的很多方面与日本、韩国还存在差距,从比较优势、治理安全的角度提出了中国天然气进口空间格局优化的方向[20].对于进口价格的研究有很多不同的角度,邹莉娜等建立了能综合考虑天然气储备对进口量和国内价格水平影响的模型,探讨了出口国垄断能力和进口国的供应中断风险厌恶程度对最优稳态储备规模、进口规模、国内天然气价格水平的影响[21];殷建平等通过建立静态和动态计量经济学模型,对我国液化天然气(LNG )进口价格与国际原油价格之间的关系进行实证研究,得出了“二者在长期内存在稳定均衡关系”的结论,并对我国天然气定价机制及相关进口贸易提出了一些合理的建议[22].除此之外,还有学者从原油价格波动、出口国偏好等角度分析对进口价格进行研究.此外,供应安全问题也是天然气进口的一大研究热点,近年来中国天然气对外依存度快速增长,在天然气进口的过程中会面临各种风险,董秀成等以供应链为基础,构建了中国天然气进口风险指标体系,使用熵权法计算指标权重,计算了中国2011—2014年从不同国家进口天然气的风险,认为我国天然气进口风险总体呈上升趋势,应从优化进口来源、加强运输通道安全、扩大海外开发投资、培养自给能力等四个方面着手,降低进口的风险,从而保证天然气供应的持续性和稳定性[23].不难看出,天然气预测领域的研究十分丰富.起初,研究主要集中在探索天然气需求的影响因素,研究的重点是确定天然气需求的核心影响因素,构建起科学合理的天然气预测指标体系;后来随着研究进程的逐渐深入,研究的重点逐渐转移到对天然气需求量的预测,试图寻找到一种可以准确预测天然气需求量的方法.然而过往的预测研究主要集中在天然气需求和消费方面,针对天然气进口量预测的研究较少.随着我国天然气消费的逐年增长,天然气进口对外依存度逐年攀升,进口天然气在我国的天然气消费中占有很高的比重,对天然气进口量进行准确预测,对于保证我国天然气供应安全、满足国内天然气消费需求有着重要的意义.2模型构建2.1随机森林模型随机森林是一种应用非常广泛的数据挖掘算法,随机森林算法具有评估变量特征重要性的功能,也是本文使用的一种功能.变量的特征重要性数值越高,则表明该变量对结果的影响越显著.用随机森林进行变量特征重要性评估的思想比较简单,主要是看每个特征在随机森林中的每棵树上做了多大的贡献,计算它们的基尼指数然后取平均值,比较不同特征之间的贡献大小.具体步骤如下.步骤1计算不纯度减少量.i (N )=∑i =1c (n iW i)2∑i =1cn iWi,(1)∆i =i (N L )-i (N R ),(2)其中:N 表示未分离的节点;N L 和N R 表示分离后的左侧节点和右侧节点;W i 为样本的类权重;n i 表示节点内各类样本的数量;∆i 是不纯度减少量,该值越大表明分离点的分离效果越好.步骤2通过计算基尼值评价各个变量的特征重要性.GI m =1-∑k =1||K p 2mk .(3)得到每个变量在各个节点上的基尼值取平均值,结果可作为变量的重要性评分,然后对各个变量进行重要性排序.2.2ARIMA 模型ARIMA 模型(Autoregressive Integrated Moving Average model ),即差分整合移动平均自回归模型,又称整合移动平均自回归模型,是时间序列预测分析方法之一.ARIMA (p ,d ,q )中,AR 是“自回归”,p 为自回归项--669第38卷第4期河南科学2020年4月数,MA 为“滑动平均”,q 为滑动平均项数,d 为使之成为平稳序列所做的差分次数(阶数).ARIMA (p ,d ,q )模型是ARMA (p ,q )模型的扩展.ARIMA (p ,d ,q )模型可以表示为:æèçöø÷1-∑i =1p φi L i (1-L )d X t =æèçöø÷1+∑i =1qθi L i εt ,(4)其中L 是滞后算子,d ∈Z ,d >0.使用ARIMA 模型的流程如下所示.步骤1根据时间序列的散点图、自相关函数和偏自相关函数图识别其平稳性.步骤2对非平稳的时间序列数据进行平稳化处理.直到处理后的自相关函数和偏自相关函数的数值非显著非零.步骤3根据所识别出来的特征建立相应的时间序列模型.平稳化处理后,若偏自相关函数是截尾的,而自相关函数是拖尾的,则建立AR 模型;若偏自相关函数是拖尾的,而自相关函数是截尾的,则建立MA 模型;若偏自相关函数和自相关函数均是拖尾的,则序列适合ARMA 模型.步骤4参数估计,检验是否具有统计意义.步骤5假设检验,判断(诊断)残差序列是否为白噪声序列.步骤6利用已通过检验的模型进行预测.2.3GA-ELM 算法由于我国天然气进口时间短、数据量少,使用简单的极限学习机(ELM )模型并不能取得很好的预测效果,我们使用遗传算法(GA ,Genetic Algorithm )对极限学习机的隐藏层节点数、输入权值和隐藏层偏差优化,能够加快ELM 模型收敛的速度,从而能够在数据样本少的情况下提高训练速度和预测精度.使用遗传算法优化ELM 的基本步骤如下.步骤1确定神经网络的拓扑结构,对神经网络的权值和阈值编码,得到初始种群.Q γ=[w γ11,⋯,w γ1j ,⋯,w γi 1,⋯,w γij ,b γ1,b γ2,⋯,b γj ],(5)式中:Q γ为种群中第γ个个体,1≤γ≤k ;w ij 、b j 在区间[-1,1]中随机取值.步骤2解码得到权值和阈值,将权值和阈值赋给新建的ELM 网络,使用训练和测试样本训练和测试网络.对网络设置目标函数为:obj =1n ∑k =1n()y i (k )-y *i (k )2,(6)式中:n 为预测时刻点的个数;y i (k )为k 时刻的真实值;y *i (k )为k 时刻的预测值.步骤3确定适应度函数、种群规模k 以及进化代数P .适应度函数用于评价个体的优劣程度,适应度函数采用排序方式的适应度分配函数:Fitness -V =ranking (obj ),其中obj 为目标函数的输出.步骤4局部求解最优适应度函数Fitness -best .进化代数θ及种群个体γ的初始值设为0,逐个求解每个个体对应的适应度函数,直到y =k 时结束循环,求得Fitness -best 值,即从中选出最优个体.步骤5全局求解最优适应度函数Fitness -best .每进行一轮局部求解最优适应度函数后,利用交叉、变异对种群进行进化,并检查进化代数θ值.当θ不大于P 时,将γ值初始化为0,返回步骤4,直到θ>P 时结束运算,此时计算出的Fitness -best 即为最优适应度函数,根据其对应的参数并解码,即可得到最佳神经网络的权值和阈值,进而确定优化的ELM 模型.3我国天然气进口预测3.1确定天然气进口的影响因素到目前为止,完善的天然气进口预测指标体系尚未构建完成.本文在借鉴了De G 等构建的天然气需求--670预测指标体系基础上,考虑了地缘政治[24]、进口依存度[25]对天然气进口的影响,选取了GDP 、城市人口数量、人均生活消费(人均每年用于各项生活消费上的支出)、结构(第二产业增加值占GDP 的比重)、家庭平均能源消费(家庭平均每年用于能源消费的支出)、对外依存度、地缘政治作为天然气进口的影响因素,其中地缘政治因素指标的确定借鉴了ECN (2004)提出的一种香农多样性指数的计算方法[26],使用随机森林算法[27]评估上述八个因素的特征重要性,指标的筛选标准是特征重要性大于0.1,图1中前六个指标的特征重要性大于0.1,可以作为模型的自变量.分别是地缘政治(x 1)、能源消费结构(x 2)、人均生活消费(x 3)、对外依存度(x 4)、工业结构(x 5)、GDP (x 6).本文数据来源于《BP 世界能源统计》和《中国能源统计年鉴》,具体数据如表1所示.表12006—2018年影响因素的历史数据Tab.1Historical data of influencing factors during 2006-2018年份2006200720082009201020112012201320142015201620172018x 10.000.270.700.940.880.880.850.840.830.850.860.820.79x 275.5375.6275.0274.9072.7473.4472.2371.3169.8064.1062.7060.4059.00x 36399755386859491108921310214663161501773219349211662284124516x 41.605.805.908.5315.3121.5628.8931.6032.4032.2034.3838.7745.30x 547.646.946.945.946.446.445.344.043.140.939.940.540.7x 6219439270232319516349081413030489301540367595244643974689052743586827122900309由于官方尚未公布2018年人均生活消费的具体数值,本文根据2016、2017年的数据对2018年的数据进行估计.通过均值插补法计算得到2018年的人均生活消费的预测值.3.2自变量预测将自变量数据代入不同的ARIMA 模型中进行拟合,选取拟合效果最好的模型对各个自变量2019—2028年的数值进行预测,结果如表2所示.根据表2的预测结果,地缘政治因素在未来十年内呈波动上升的态势;能源消费结构逐年下降;人均生活消费逐年增加;天然气对外依存度逐年上升,预计在2020年之后天然气进口对外依存度超过50%;第二产业增加值占GDP 的比重逐年减少,工业结构指标逐年下降;GDP 总量预计逐年增长,但是增长速度会逐年放慢.3.3预测结果及分析为了避免各参数取值范围差异较大给预测结果带来影响,本文在预测时对所有数据进行归一化处理.仿真实验在PC 上进行,运行环境为:Intel (R )Core (TM )i5-42102.4GHz 处理器,8GB 内存,Win864位操作系统,运行平台为Matlab 2018b .选取自变量2006—2015年的数据作为模型的训练集,2016—2018年的数据作为测试集.为了增强实验结果的说服力,本文对模型训练了30次,并计算出30次对测试集预测结果的误差的平均值.误差分析结果如表3所示.图1天然气进口影响因素的特征重要性Fig.1Characteristic importance of influencing factors on natural gas import特征重要性影响因素引用格式:李宏勋,宫本璞.基于GA-ELM 模型的我国天然气进口预测[J ].河南科学,2020,38(4):667-673.--671第38卷第4期河南科学2020年4月表22019—2028年影响因素的预测值Tab.2Predicted values of influencing factors during 2019-2028年份2019202020212022202320242025202620272028ARIMA (3,0,3)0.871.041.211.321.371.351.311.281.281.33ARIMA (0,1,1)57.6256.2554.8453.552.1250.7549.3747.9946.6245.24ARIMA (0,1,0)26407283583036832436345633674938994412984366046081ARIMA (1,0,1)49.8752.7156.0059.4763.0265.6168.2070.8171.4172.01ARIMA (1,0,1)39.9539.0638.3237.6336.9636.3035.6434.9834.3233.66GDP/亿元ARIMA (1,0,1)94085798748710424591098176115395912097491265539132132913771191432909表3结果误差Tab.3Result error模型ELMR 20.7005MSE 25703.61MAE 119.96MAPE 14.28模型GA-ELMR 20.9994MSE 20.24MAE 3.63MAPE 0.51不难看出,虽然受到数据样本数量的限制,但经过遗传算法优化后的极限学习机模型的样本拟合度和精确度相比单纯的极限学习机模型提高了很多,模型拟合优度R 2达到0.999以上,MSE (均方误差)、MAE (平均绝对误差)、MAPE (平均绝对百分比误差)等误差指标大幅度降低,说明遗传算法对极限学习机(ELM )模型的优化效果十分明显.将自变量的预测值代入到训练好的GA-ELM 模型中后,我们得到了2019—2028年中国天然气进口量的预测值,如表4所示.表4未来我国天然气进口量预测值Tab.4Predicted value of China ’s natural gas import in the future年份20192020202120222023预测值/(亿m 3)1583.671816.732059.852298.152572.61增长量/(亿m 3)306.02233.06243.12238.30274.46增长率/%23.9514.7213.3411.5711.94年份20242025202620272028预测值/(亿m 3)2895.903266.463604.064020.694408.18增长量/(亿m 3)323.29370.56337.6416.63387.49增长率/%12.5712.8010.3411.569.64从表4预测出的我国天然气未来的进口趋势可以看出,未来十年内我国天然气进口量还将持续增长,进口量在经历了最初几年的波动之后,呈逐年上升的趋势.天然气进口的增长速度在2019年最快,随后会逐年下降,2022年以后,天然气进口增长速度趋于平稳.4结论本文提出了一种基于GA-ELM 集成学习的天然气进口预测模型,该模型使用遗传算法优化了ELM 隐藏层的节点数、输入权重和阈值.使用随机森林算法提取了中国天然气进口的核心影响因素并作为预测模型的自变量,使用ARIMA 模型对自变量未来的变化趋势进行预测.得到如下结论.1)使用随机森林算法计算各要素的特征重要性,选取特征重要性最高的六个影响因素作为预测模型中的自变量.通过计算特征重要性,我们发现地缘政治因素对天然气进口量的影响最大,主要原因可能是天然气进口协议是在国家层面签订的,而国家之间的地缘政治关系影响着天然气进口协议的签订和履行.其次是能源消费结构,我国能源消费总量大,能源结构向着低碳化、清洁化的方向调整,会对天然气进口需求产生相应的影响.此外,对外依存度、工业结构和经济发展水平等因素对天然气进口量也存在显著影响.--672引用格式:李宏勋,宫本璞.基于GA-ELM模型的我国天然气进口预测[J].河南科学,2020,38(4):667-673.2)使用遗传算法优化ELM模型的阈值和隐藏层权重可以加快收敛速度、减少训练样本和训练次数,可以有效提高模型的预测性能.比较GA-ELM和ELM模型的R2、MSE、MAE、MAPE值,证明了遗传算法对ELM模型的优化效果十分明显,使用GA-ELM模型以及ARIMA模型对2019—2028年中国天然气进口量以及核心影响因素的变化进一步预测.结果表明,本文提出的GA-ELM集成学习模型对于天然气进口量的预测是有效的,能以较高的精度预测未来天然气进口量的变化,为后续相关研究提供参考.3)预测结果表明未来十年内我国天然气进口量还将持续增长,天然气进口的增长速度在2019年达到顶峰,随后几年里快速下降,并在2022年之后趋于平稳.天然气进口量的变化是复杂多样的,本文使用的预测方法并不一定能准确预测实际的天然气进口量,但有可能掌握天然气进口的增长趋势,为我国天然气进口计划以及天然气产业相关政策的制定提供参考,帮助政府提前对天然气市场中可能出现的问题进行干预,以平衡市场中可能出现的矛盾,为天然气产业的健康发展提供理论依据.参考文献:[1]中华人民共和国国家发展和改革委员会.能源发展“十三五”规划[EB/OL].(2016-12-24)[2017-02-25].http://www.ndrc./zcfb/zcfbtz/201701/W020170117335278192779.html.[2]刘澎涛,刘刚,韩金良,等.中国能源转型中天然气的角色[J].科技和产业,2014,14(6):136-139.[3]范照伟.全球天然气发展格局及我国天然气发展方向分析[J].中国矿业,2018,27(4):11-16,22.[4]BP能源公司.BP世界能源统计年鉴[EB/OL].(2018-06-13)[2019-04-15].http:///zh_cn/china/reports-and-publications.html.[5]国家能源局石油天然气司,国务院发展研究中心资源与环境政策研究所与国土资源部油气资源战略研究中心.中国天然气发展报告[M].北京:石油工业出版社,2017.[6]LI J,DONG X,SHANGGUAN J,et al.Forecasting the growth of Chinese natural gas consumption[J].Fuel&Energy Abstracts,2011,36(3):1380-1385.[7]李君臣,董秀成,高建.我国天然气消费的系统动力学预测与分析[J].天然气工业,2010(4):127-129.[8]ZHANG W,YANG J.Forecasting natural gas consumption in China by Bayesian Model Averaging[J].Energy Reports,2015,1:216-220.[9]CHAI J,LIANG T,LAI K K,et al.The future natural gas consumption in China:Based on the LMDI-STIRPAT-PLSR framework and scenario analysis[J].Energy Policy,2018,119:215-225.[10]王兵,田丹,刘书文,等.天然气消费量的灰色模型预测[J].管道技术与设备,2008(5):3-5.[11]袁树明,赖建波,张德坤,等.城市燃气管网长期规划用气量的预测[J].煤气与热力,2009,29(5):24-27.[12]WANG J,JIANG H,ZHOU Q,et al.China’s natural gas production and consumption analysis based on the multicycle Hubbert model and rolling Grey model[J].Renewable&Sustainable Energy Reviews,2016,53:1149-1167.[13]WU L,LIU S,CHEN H,et ing a novel grey system model to forecast natural gas consumption in China[J].Mathematical Problems in Engineering,2015,2015(pt.1):686501.1-686501.7.[14]SHAIKH F,JI Q.Forecasting natural gas demand in China:Logistic modelling analysis[J].International Journal of Electrical Power&Energy Systems,2016,77:25-32.[15]SZOPLIK,JOLANTA.Forecasting of natural gas consumption with artificial neural networks[J].Deyun W Energy,2015,85:208-220.[16]BAI Y,LI C.Daily natural gas consumption forecasting based on a structure-calibrated support vector regression approach[J].Energy and Buildings,2016,127:571-579.[17]DEYUN W,YANLING L,ZENG W,et al.Scenario analysis of natural gas consumption in China based on wavelet neural network optimized by particle swarm optimization algorithm[J].Energies,2018,11(4):825.[18]KARADEDE Y,OZDEMIR G,AYDEMIR E.Breeder hybrid algorithm approach for natural gas demand forecasting model[J].Energy,2017,141(PT.1):1269-1284.[19]DE G,GAO W.Forecasting China’s natural gas consumption based on adaboost-particle swarm optimization-extreme learning machine integrated learning method[J].Energies,2018,11(11):1-20.[20]孙聆轩,吴晓明,李建平,等.中国天然气进口空间格局演进及优化路径[J].天然气工业,2016,36(2):125-130.[21]邹莉娜,张荣,任庆忠.天然气储备对天然气进口及国内价格的动态影响[J].中国管理科学,2017,25(6):132-142.[22]殷建平,黄志健,张云凌.我国液化天然气进口价格与国际原油价格相关性实证分析[J].价格月刊,2012(10):57-60.[23]董秀成,孔朝阳.基于供应链角度的中国天然气进口风险研究[J].天然气工业,2017,37(5):113-118.[24]CABALU H.Indicators of security of natural gas supply in Asia[J].Energy Policy,2010,38(1):218-225.[25]Donald B P,Andrew T W.Spectral analysis for physical applications[M].Cambridge:Cambridge University Press,1993.[26]JANSEN J C,VAN ARKEL W G,BOOTS M G.Designing indicators of long-term energy supply security[R].Amsterdam:Energy Reseach Center of the Netherlands,2004:14-15.[27]CUTLER A,CUTLER D R,STEVENS J R.Random forests[J].Machine Learning,2011,45(1):157-176.(编辑康艳)-673-。
人工智能英文课件

Supervised learning is a type of machine learning where the algorithm is provided with labeled training data The goal is to learn a function that maps input data to desired outputs based on the provided labels Common examples include classification and regression tasks
Deep learning is a type of machine learning that uses neural networks with multiple layers of hidden units to learn complex patterns and representations from data It is based on biomimetic neural networks and self-organizing mapping networks.
Machine translation is the process of automatically translating text or speech from one language to another using computer algorithms and language data banks This technology has identified the need for human translators in many scenarios
Some challenges associated with deep learning include the requirement for large amounts of labeled data, the complexity of explaining the learned patterns or representations, and the potential for overflow or poor generalization to unseen data
麦肯锡奖——精选推荐
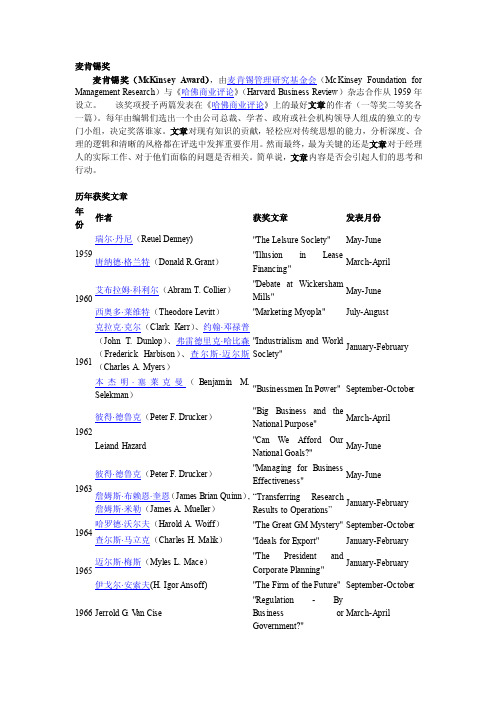
麦肯锡奖麦肯锡奖(McKinsey Award),由麦肯锡管理研究基金会(Mc Kinsey Foundation for Management Research)与《哈佛商业评论》(Harvard Business Review)杂志合作从1959年设立。
该奖项授予两篇发表在《哈佛商业评论》上的最好文章的作者(一等奖二等奖各一篇)。
每年由编辑们选出一个由公司总裁、学者、政府或社会机构领导人组成的独立的专门小组,决定奖落谁家。
文章对现有知识的贡献,轻松应对传统思想的能力,分析深度、合理的逻辑和清晰的风格都在评选中发挥重要作用。
然而最终,最为关键的还是文章对于经理人的实际工作、对于他们面临的问题是否相关。
简单说,文章内容是否会引起人们的思考和行动。
历年获奖文章年份作者获奖文章发表月份1959 瑞尔·丹尼(Reuel Denney) "The Lelsure Soclety" May-June 唐纳德·格兰特(Donald R.Grant)"Illusion in LeaseFinancing"March-April1960 艾布拉姆·科利尔(Abram T. Collier)"Debate at WickershamMills"May-June 西奥多·莱维特(Theodore Levitt)"Marketing Myopla" July-August1961 克拉克·克尔(Clark Kerr)、约翰·邓禄普(John T. Dunlop)、弗雷德里克·哈比森(Frederick Harbison)、查尔斯·迈尔斯(Charles A. Myers)"Industrialism and WorldSoclety"January-February本杰明·塞莱克曼(Benjamin M.Selekman)"Businessmen In Power" September-October1962 彼得·德鲁克(Peter F. Drucker)"Big Business and theNational Purpose"March-April Leiand Hazard"Can We Afford OurNational Goals?"May-June1963 彼得·德鲁克(Peter F. Drucker)"Managing for BusinessEffectiveness"May-June詹姆斯·布赖恩·奎恩(James Brian Quinn),詹姆斯·米勒(James A. Mueller)“Transferring ResearchResults to Operations”January-February1964 哈罗德·沃尔夫(Harold A. Woiff)"The Great GM Mystery" September-October 查尔斯·马立克(Charles H. Malik)"Ideals for Export" January-February1965 迈尔斯·梅斯(Myles L. Mace)"The President andCorporate Planning"January-February 伊戈尔·安索夫(H. Igor Ansoff) "The Firm of the Future" September-October1966 Jerrold G. V an Cise "Regulation - ByBusiness or Government?"March-April西奥多·莱维特(Theodore Levitt)"Innovative Imitation" September-October1967 爱德华·莱普(H. Edward Wrapp)"Good Managers Don'tMake Policy Decisions"September-October 詹姆斯·布赖恩·奎恩(James Brian Quinn)"TechnologicalForecasting"March-April1968 西奥多·莱维特(Theodore Levitt)"Why Business AlwaysLoses"March-April西奥多·珀塞尔(Theodore V. Purcell)"Break Down Y ourEmployment Barriers"July-August吉恩·布拉德利(Gene E. Bradley)"What BusinessmenNeed to Know About theStudent Left"September-October1969 梅尔文·安申(Melvin Anshen)"The Management ofIdeas"July-August 哈里·莱文森(Harry Levinson)"On Being aMiddle-Aged Manager"July-August1970 乔治·洛奇(George Cabot Lodge)"Top Priority:Renovating OurIdeology"September-October塞缪尔·卡尔伯特(Samuel A. Culbert)、詹姆斯·埃尔登(James M. Elden)"An Anatomy ofActivism for Executives"November-December1971 利文斯顿·斯特林(J. Sterling Livingston)"Myth of theWell-Educated Manager"January-February 彼得·德鲁克(Peter F. Drucker)"What We Can LearnFrom JapaneseManagement"March-April1972 西奥多·莱维特(Theodore Levitt)"Production-LineApproach to Service"September-October 迈尔斯·梅斯(Myles L. Mace)"The President and theBoard of Directors"March-April1973 小杰克逊·格雷森(C. Jackson Grayson, Jr.)"Let's Get Back to theCompetitive MarketSystem"November-December 布鲁斯·斯科特(Bruce R. Scott)"The Industrial State: OldMyths and NewRealities"March-April1974 乔治·洛奇(George Cabot Lodge)"Business and theChanging Society"March-April彼得·德鲁克(Peter F. Drucker)"New Templates forToday's Organizations"January-February1975 亨利·明茨伯格(Henry Mintzberg) "The Manager's Job: July-AugustFolklore and Fact"马丁·费尔德斯坦(Martin S. Feldstein)"UnemploymentInsurance: Time for Reform"March-April1976 戴维·麦克利兰(David C. McClelland)、戴维·伯纳姆(David H. Burnham)"Power is the GreatMotivator"March-April罗伯特·安东尼(Robert N. Anthony)"A Case for HistoricalCosts"November-December1977 亚伯拉罕·扎莱兹尼克(AbrahamZaleznik)"Managers and Leaders:Are They Different?"May-June托马斯·弗林(Thomas D. Flynn)"Why We ShouldAccount for Inflation"September-October1978 阿尔弗雷德·尼尔(Alfred C. Neal)"Immolation of BusinessCapital"March-April 理查德·坦纳·帕斯卡尔(Richard TannerPascale)"Zen and the Art ofManagement"March-April1979 迈克尔·波特(Michael Porter)"How CompetitiveForces Shape Strategy"March-April 罗莎贝斯·莫斯·坎特(Rosabeth MossKanter)"Power Failure inManagement Circuits"July-August1980 罗伯特·海斯(Robert H. Hayes)、威廉·艾伯纳西(William J. Abernathy)"Managing Our Way toEconomic Decline"July-August安德烈·贝纳德(Andre Benard)"World Oil and ColdReality"November-December 约翰·加巴罗(John J. Gabarro)、约翰·科特(John P. Kotter)"Managing Y our Boss" January-February1981 威廉·艾伯纳西(William J. Abernathy),金·克拉克(Kim B. Clark), and Alan M.Kantrow"The New IndustrialCompetition"September-October 彼得·德鲁克(Peter F. Drucker)"Behind Japan's Success" J anuary-February1982 罗伯特·海斯(Robert H. Hayes)、戴维·加文(David A. Garvin)"Managing as ifTomorrow Mattered"May-June布鲁斯·斯科特(Bruce R. Scott)"Can Industry Survivethe Welfare State?"September-October1983 戴维·加文(David A. Garvin)"Quality on the Line" September-October 罗伯特·杰克考尔(Robert Jackall)"Moral Mazes:Bureaucracy andManagerial Work"September-October1984 理查德·伯耶尔(Richard J. Boyle)"Wrestling withJellyfish"January-February罗伯特·卡普兰(Robert S. Kaplan)"Yesterday's Accounting Undermines Production"July-August1986 威克汉姆·斯金纳(Wickham Skinner)"The ProductivityParadox"July-August William H. Peace"I Thought I Knew WhatGood Management Was"March-April1987 迈克尔·波特(Michael Porter)"From CompetitiveAdvantage to CorporateStrategy"May-June 亨利·明茨伯格(Henry Mintzberg) "Crafting Strategy" July-August1985 詹姆斯·布赖恩·奎恩(James Brian Quinn)"Managing Innovation:Controlled Chaos"May-June皮埃尔·瓦克(Pierre Wack)"Scenarios: UnchartedWaters Ahead"September-October1988 小乔治·斯托克(George Stalk, Jr.)"Time - The Next Sourceof CompetitiveAdvantage"July-August 克里斯托弗·哈特(Christopher W.L. Hart)"The Power ofUnconditional ServiceGuarantees"July-August1989 加里·哈默尔(Gary Hamel)and 普拉哈拉德(C.K.Prahalad)"Strategic Intent" May-June菲利斯·斯沃兹(Felice N. Schwartz)"Management Womenand the New Facts ofLife"January-February 迈克尔·詹森(Michael C. Jensen)"Eclipse of the PublicCorporation"September-October1990 William Wiggenhorn"Motorola U: WhenTraining Becomes anEducation"July-August 查尔斯·弗格森(Charles H. Ferguson)"Computers and theComing of the U.S.Keiretsu"July-August普拉哈拉德(C.K.Prahalad)、加里·哈默尔(Gary Hamel)"The Core Competenceof the Corporation"May-June1991 安德鲁·拉帕波特(Andrew S. Rappaport)、斯缪尔·哈勒维(Shmuel Halevi)"The ComputerlessComputer Company"July-August约翰·希利·布朗(John Seely Brown)"Research ThatReinvents theCorporation"January-February1992 查尔斯·汉迪(Charles Handy)"Balancing Corporate November-DecemberPower: A New Federalist Paper"查尔斯·奈特(Charles F. Knight)"Emerson Electric:Consistent Profits, Consistently"January-February1993 詹姆斯·摩尔(James Moore)"Predators and Prey: ANew Ecology ofCompetition"May-June 戴维·加文(David A. Garvin)"Building a LearningOrganization"July-August1994 克里斯·阿吉里斯(Chris Argyris)"Good CommunicationThat Blocks Learning"July-August彼得·德鲁克(Peter F. Drucker)"The Theory of theBusiness"September-October1995 约瑟夫·鲍尔(Joseph L. Bower)、克莱顿·克里斯滕森(Clayton M. Christensen)"DisruptiveTechnologies: Catchingthe Wave"January-February 查尔斯·汉迪(Charles Handy)"Trust and the V irtualOrganization"May-June1996 加里·哈默尔(Gary Hamel)"Strategy as Revolution" July-August迈克尔·波特(Michael Porter)"What Is Strategy?" November-December1997 斯图尔特·哈特(Stuart L. Hart)"Beyond Greening:Strategies for aSustainable World"January-February 阿里·德赫斯(Arie de Geus)"The Living Company" March-April菲利普·埃文斯(Philip B. Evans)、托马斯·沃斯特(Thomas S. Wurster)"Strategy and the NewEconomics ofInformation"September-October1998 琼·玛格丽塔(Joan Magretta)"The Power of V irtualIntegration: An Interviewwith Dell Computer'sMichael Dell"March-April提摩西·鲁曼(Timothy A. Luehrman)"Strategy as a Portfolioof Real Options"September-October 普拉哈拉德(C.K.Prahalad)、李侃如(Kenneth Lieberthal)"The End of CorporateImperialism"July-August1999 约翰·哈格尔三世(John Hagel III)、马克·辛格(Marc Singer)"Unbundling theCorporation"March-April加里·哈默尔(Gary Hamel)"Bringing Silicon V alleyInside"September-October2000 罗伯特·戈费(Robert Goffee)、加雷斯·琼"Why Should Anyone Be September-October斯(Gareth Jones)Led by Y ou?"迈克尔·麦考比(Michael Maccoby)"Narcissistic Leaders:The Incredible Pros, theInevitable Cons"January-February胡安·恩里克斯(Juan Enriquez)、雷·戈德伯格(Ray A. Goldberg)"Transforming Life, Transforming Business:The Life-Science Revolution"March-April2001 迈克尔·波特(Michael Porter)"Strategy and theInternet"March约翰·哈格尔(John Hagel)、约翰·希利·布朗(John Seely Brown)"Y our Next IT Strategy" October 克莱顿·克里斯滕森(ClaytonChristensen)、迈克尔·雷纳(MichaelRaynor)、马修·韦尔兰登(Matthew C.V erlinden)"Skate to Where theMoney Will Be"November2002 理查德·法森(Richard Farson)、拉尔夫·凯伊斯(Ralph Keyes)"The Failure TolerantLeader"August迈克尔·波特(Michael Porter)、马克·克莱默(Mark R. Kramer)"The CompetitiveAdvantage of CorporatePhilanthropy"December2003 悉尼·罗森(Sydney Rosen)、乔纳森·西蒙(Jonathan Simon)、杰弗里·文森特(Jeffery R. V incent)、威廉·麦克劳德(William MacLeod)、马修·福克斯(Matthew Fox)、唐纳德·西娅(DonaldM. Thea)"AIDS Is Y our Business" F ebruary 罗德里克·克莱默(Roderick M. Kramer)"The Harder They Fall" October2004 彼得·德鲁克(Peter F. Drucker)"What Makes anEffective Executive"June罗伯特·莫里森(Robert Morison)、塔玛拉·埃里克森(Tamara Erickson)、肯·迪赫特沃德(Ken Dychtwald)"It's Time to RetireRetirement"March 李效良(Hau L. Lee)"The Triple-A SupplyChain"October2005 潘卡基·格玛沃特(Pankaj Ghemawat)"Regional Strategies forGlobal Leadership"December 斯蒂文·斯比尔(Steven J. Spear)"Fixing Health Care fromthe Inside, Today"。
you ga 口语
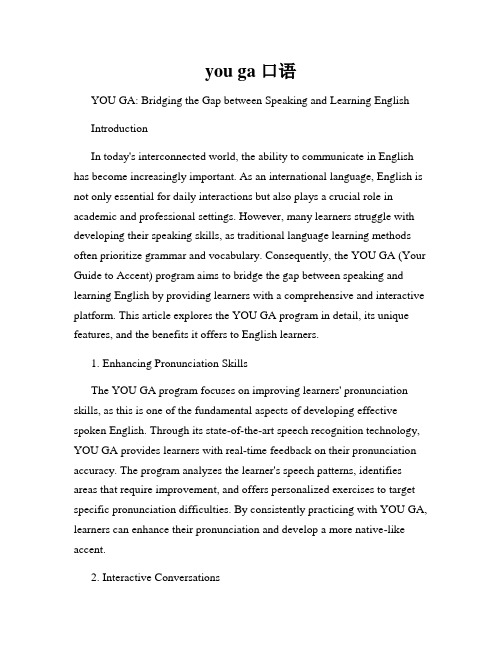
you ga 口语YOU GA: Bridging the Gap between Speaking and Learning EnglishIntroductionIn today's interconnected world, the ability to communicate in English has become increasingly important. As an international language, English is not only essential for daily interactions but also plays a crucial role in academic and professional settings. However, many learners struggle with developing their speaking skills, as traditional language learning methods often prioritize grammar and vocabulary. Consequently, the YOU GA (Your Guide to Accent) program aims to bridge the gap between speaking and learning English by providing learners with a comprehensive and interactive platform. This article explores the YOU GA program in detail, its unique features, and the benefits it offers to English learners.1. Enhancing Pronunciation SkillsThe YOU GA program focuses on improving learners' pronunciation skills, as this is one of the fundamental aspects of developing effective spoken English. Through its state-of-the-art speech recognition technology, YOU GA provides learners with real-time feedback on their pronunciation accuracy. The program analyzes the learner's speech patterns, identifies areas that require improvement, and offers personalized exercises to target specific pronunciation difficulties. By consistently practicing with YOU GA, learners can enhance their pronunciation and develop a more native-like accent.2. Interactive ConversationsOne of the key features of the YOU GA program is its interactive conversation modules. Traditional language learning methods often lack opportunities for learners to engage in real-life conversations, leading to a significant gap between classroom learning and practical application. YOU GA addresses this issue by providing a wide range of conversational topics and scenarios. Learners can engage in dialogue with virtual characters, simulating real-life conversations. This immersive experience not only enhances speaking skills but also boosts learners' confidence in using English in various social and professional contexts.3. Cultural ContextualizationUnderstanding cultural nuances is crucial for effective communication in any language. YOU GA acknowledges the importance of cultural contextualization by integrating cultural elements into its language learning modules. Learners are exposed to authentic materials such as videos, articles, and interviews that reflect different aspects of English-speaking cultures. By immersing themselves in these materials, learners not only develop their language skills but also gain insights into cultural practices, customs, and norms. This holistic approach contributes to a deeper understanding of English as a global language.4. Vocabulary ExpansionWhile vocabulary is often considered a component of traditional language learning, YOU GA takes a creative approach to vocabulary expansion. Instead of simply memorizing word lists, learners are exposed to contextualized vocabulary exercises. These exercises help learners understand how words are used in different contexts, promoting morenatural and fluent communication. YOU GA also incorporates gamification elements into vocabulary learning, making it more engaging and motivating for learners. Through these innovative techniques, learners can expand their vocabulary while enjoying the learning process.5. Peer InteractionYOU GA recognizes the importance of peer interaction in language learning. The program facilitates learners' engagement with their peers through various interactive features, such as discussion forums and virtual group activities. Learners can practice their speaking skills by engaging in conversations with fellow learners from around the world. This not only provides an opportunity for language practice but also promotes cultural exchange and global understanding. Collaborative learning experiences foster a supportive and inclusive learning community, encouraging learners to actively participate and grow together.6. Progress Tracking and AssessmentTo ensure learners' progress, YOU GA offers comprehensive progress tracking and assessment systems. Learners can monitor their improvement through detailed performance reports, which include feedback on pronunciation, vocabulary usage, and conversational proficiency. Additionally, periodic assessments and quizzes allow learners to evaluate their understanding of different language aspects. This data-driven approach enables learners to identify areas for improvement and tailor their learning accordingly, resulting in effective and efficient English language acquisition.ConclusionThe YOU GA program revolutionizes the way English learners develop their speaking skills. By combining advanced technology, interactive conversations, cultural contextualization, vocabulary expansion, peer interaction, and comprehensive assessment, YOU GA offers a comprehensive and engaging language learning experience. With its user-friendly interface and personalized approach, YOU GA provides learners with the tools necessary to bridge the gap between speaking and learning English. Start your journey with YOU GA today and unlock the full potential of your English oral proficiency.。
学习方法对英语学习的重要性 英语作文
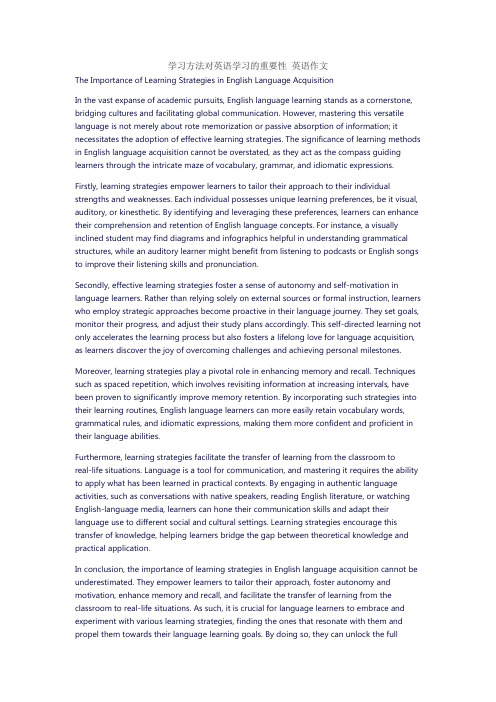
学习方法对英语学习的重要性英语作文The Importance of Learning Strategies in English Language AcquisitionIn the vast expanse of academic pursuits, English language learning stands as a cornerstone, bridging cultures and facilitating global communication. However, mastering this versatile language is not merely about rote memorization or passive absorption of information; it necessitates the adoption of effective learning strategies. The significance of learning methods in English language acquisition cannot be overstated, as they act as the compass guiding learners through the intricate maze of vocabulary, grammar, and idiomatic expressions.Firstly, learning strategies empower learners to tailor their approach to their individual strengths and weaknesses. Each individual possesses unique learning preferences, be it visual, auditory, or kinesthetic. By identifying and leveraging these preferences, learners can enhance their comprehension and retention of English language concepts. For instance, a visually inclined student may find diagrams and infographics helpful in understanding grammatical structures, while an auditory learner might benefit from listening to podcasts or English songs to improve their listening skills and pronunciation.Secondly, effective learning strategies foster a sense of autonomy and self-motivation in language learners. Rather than relying solely on external sources or formal instruction, learners who employ strategic approaches become proactive in their language journey. They set goals, monitor their progress, and adjust their study plans accordingly. This self-directed learning not only accelerates the learning process but also fosters a lifelong love for language acquisition, as learners discover the joy of overcoming challenges and achieving personal milestones. Moreover, learning strategies play a pivotal role in enhancing memory and recall. Techniques such as spaced repetition, which involves revisiting information at increasing intervals, have been proven to significantly improve memory retention. By incorporating such strategies into their learning routines, English language learners can more easily retain vocabulary words, grammatical rules, and idiomatic expressions, making them more confident and proficient in their language abilities.Furthermore, learning strategies facilitate the transfer of learning from the classroom toreal-life situations. Language is a tool for communication, and mastering it requires the ability to apply what has been learned in practical contexts. By engaging in authentic language activities, such as conversations with native speakers, reading English literature, or watching English-language media, learners can hone their communication skills and adapt their language use to different social and cultural settings. Learning strategies encourage this transfer of knowledge, helping learners bridge the gap between theoretical knowledge and practical application.In conclusion, the importance of learning strategies in English language acquisition cannot be underestimated. They empower learners to tailor their approach, foster autonomy and motivation, enhance memory and recall, and facilitate the transfer of learning from the classroom to real-life situations. As such, it is crucial for language learners to embrace and experiment with various learning strategies, finding the ones that resonate with them and propel them towards their language learning goals. By doing so, they can unlock the fullpotential of the English language, using it as a key to open doors to new cultures, ideas, and opportunities.。
(完整版)人工智能原理MOOC习题集及答案北京大学王文敏课件
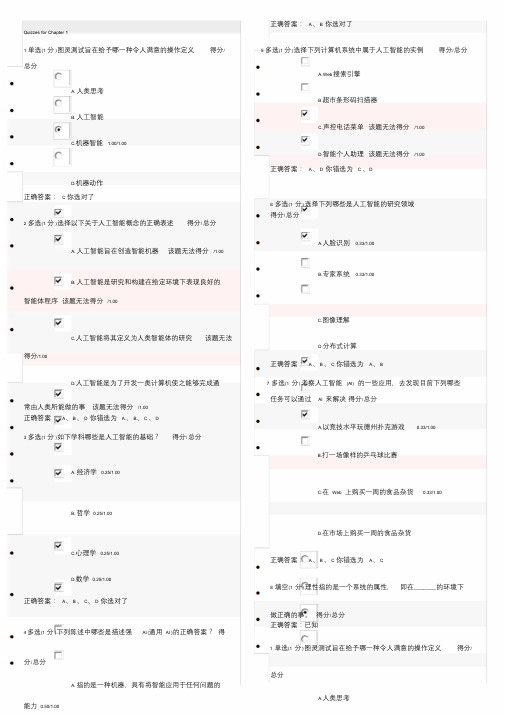
正确答案:A、B 你选对了Quizzes for Chapter 11 单选(1 分)图灵测试旨在给予哪一种令人满意的操作定义得分/ 5 多选(1 分)选择下列计算机系统中属于人工智能的实例得分/总分总分A. W eb搜索引擎A. 人类思考B.超市条形码扫描器B. 人工智能C.声控电话菜单该题无法得分/1.00C.机器智能 1.00/1.00D.智能个人助理该题无法得分/1.00正确答案:A、D 你错选为C、DD.机器动作正确答案: C 你选对了6 多选(1 分)选择下列哪些是人工智能的研究领域得分/总分2 多选(1 分)选择以下关于人工智能概念的正确表述得分/总分A.人脸识别0.33/1.00A. 人工智能旨在创造智能机器该题无法得分/1.00B.专家系统0.33/1.00B. 人工智能是研究和构建在给定环境下表现良好的智能体程序该题无法得分/1.00C.图像理解C.人工智能将其定义为人类智能体的研究该题无法D.分布式计算得分/1.00正确答案:A、B、C 你错选为A、BD.人工智能是为了开发一类计算机使之能够完成通7 多选(1 分)考察人工智能(AI) 的一些应用,去发现目前下列哪些任务可以通过AI 来解决得分/总分常由人类所能做的事该题无法得分/1.00正确答案:A、B、D 你错选为A、B、C、DA.以竞技水平玩德州扑克游戏0.33/1.003 多选(1 分)如下学科哪些是人工智能的基础?得分/总分B.打一场像样的乒乓球比赛A. 经济学0.25/1.00C.在Web 上购买一周的食品杂货0.33/1.00B. 哲学0.25/1.00D.在市场上购买一周的食品杂货C.心理学0.25/1.00正确答案:A、B、C 你错选为A、CD.数学0.25/1.008 填空(1 分)理性指的是一个系统的属性,即在_________的环境下正确答案:A、B、C、D 你选对了做正确的事。
得分/总分正确答案:已知4 多选(1 分)下列陈述中哪些是描述强AI (通用AI )的正确答案?得1 单选(1 分)图灵测试旨在给予哪一种令人满意的操作定义得分/ 分/总分总分A. 指的是一种机器,具有将智能应用于任何问题的A.人类思考能力0.50/1.00B.人工智能B. 是经过适当编程的具有正确输入和输出的计算机,因此有与人类同样判断力的头脑0.50/1.00C.机器智能 1.00/1.00C.指的是一种机器,仅针对一个具体问题D.机器动作正确答案: C 你选对了D.其定义为无知觉的计算机智能,或专注于一个狭2 多选(1 分)选择以下关于人工智能概念的正确表述得分/总分窄任务的AIA. 人工智能旨在创造智能机器该题无法得分/1.00B.专家系统0.33/1.00B. 人工智能是研究和构建在给定环境下表现良好的C.图像理解智能体程序该题无法得分/1.00D.分布式计算C.人工智能将其定义为人类智能体的研究该题无法正确答案:A、B、C 你错选为A、B得分/1.00 7 多选(1 分)考察人工智能(AI) 的一些应用,去发现目前下列哪些任务可以通过AI 来解决得分/总分D.人工智能是为了开发一类计算机使之能够完成通A.以竞技水平玩德州扑克游戏0.33/1.00常由人类所能做的事该题无法得分/1.00正确答案:A、B、D 你错选为A、B、C、DB.打一场像样的乒乓球比赛3 多选(1 分)如下学科哪些是人工智能的基础?得分/总分C.在Web 上购买一周的食品杂货0.33/1.00A. 经济学0.25/1.00D.在市场上购买一周的食品杂货B. 哲学0.25/1.00正确答案:A、B、C 你错选为A、CC.心理学0.25/1.008 填空(1 分)理性指的是一个系统的属性,即在_________的环境下D.数学0.25/1.00 做正确的事。
The importance of learning a second language
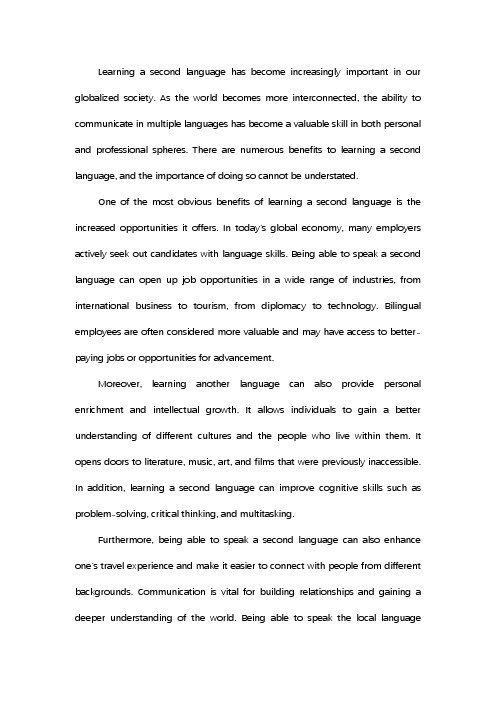
Learning a second language has become increasingly important in our globalized society. As the world becomes more interconnected, the ability to communicate in multiple languages has become a valuable skill in both personal and professional spheres. There are numerous benefits to learning a second language, and the importance of doing so cannot be understated.One of the most obvious benefits of learning a second language is the increased opportunities it offers. In today's global economy, many employers actively seek out candidates with language skills. Being able to speak a second language can open up job opportunities in a wide range of industries, from international business to tourism, from diplomacy to technology. Bilingual employees are often considered more valuable and may have access to better-paying jobs or opportunities for advancement.Moreover, learning another language can also provide personal enrichment and intellectual growth. It allows individuals to gain a better understanding of different cultures and the people who live within them. It opens doors to literature, music, art, and films that were previously inaccessible. In addition, learning a second language can improve cognitive skills such as problem-solving, critical thinking, and multitasking.Furthermore, being able to speak a second language can also enhance one's travel experience and make it easier to connect with people from different backgrounds. Communication is vital for building relationships and gaining a deeper understanding of the world. Being able to speak the local languagewhen traveling can also make it easier to navigate unfamiliar places, understand local customs, and form meaningful connections with the people one encounters.For those living in multicultural societies, knowing more than one language can also promote social inclusion and understanding. It can bridge the gap between different communities and foster a sense of unity. By learning the language of a minority group, one can gain insight into their experiences and struggles, as well as better understand their culture and traditions.The importance of learning a second language is also reflected in its impact on mental health. Studies have shown that bilingualism can delay the onset of dementia and Alzheimer's disease. The brain of a bilingual person is constantly juggling between languages, which exercises and strengthens cognitive functions. This cognitive flexibility can lead to better problem-solving skills and a higher capacity for creative thinking.In conclusion, there are numerous benefits and reasons for learning a second language. It not only provides practical advantages in the job market, but also fosters personal growth, cultural understanding, and mental agility. As the world becomes more interconnected, the ability to speak multiple languages is becoming an essential skill. Therefore, learning a second language is a valuable investment that can significantly enrich one's personal and professional life. Whether for career advancement, personal development, or global citizenship, the importance of learning a second language cannot be overstated.It is a skill that opens doors, enriches lives, and connects people across borders.。
Bipedal Walking on Rough Terrain Using Manifold Control
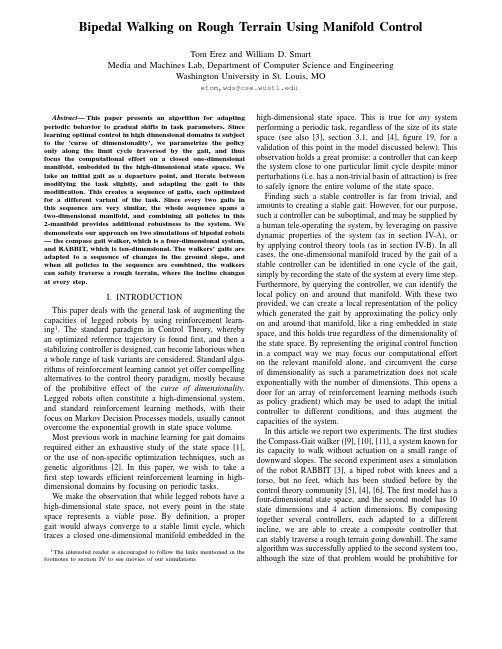
Bipedal Walking on Rough Terrain Using Manifold ControlTom Erez and William D.SmartMedia and Machines Lab,Department of Computer Science and EngineeringWashington University in St.Louis,MOetom,wds@Abstract—This paper presents an algorithm for adapting periodic behavior to gradual shifts in task parameters.Since learning optimal control in high dimensional domains is subject to the’curse of dimensionality’,we parametrize the policy only along the limit cycle traversed by the gait,and thus focus the computational effort on a closed one-dimensional manifold,embedded in the high-dimensional state space.We take an initial gait as a departure point,and iterate between modifying the task slightly,and adapting the gait to this modification.This creates a sequence of gaits,each optimized for a different variant of the task.Since every two gaits in this sequence are very similar,the whole sequence spans a two-dimensional manifold,and combining all policies in this 2-manifold provides additional robustness to the system.We demonstrate our approach on two simulations of bipedal robots —the compass gait walker,which is a four-dimensional system, and RABBIT,which is ten-dimensional.The walkers’gaits are adapted to a sequence of changes in the ground slope,and when all policies in the sequence are combined,the walkers can safely traverse a rough terrain,where the incline changes at every step.I.INTRODUCTIONThis paper deals with the general task of augmenting the capacities of legged robots by using reinforcement learn-ing1.The standard paradigm in Control Theory,whereby an optimized reference trajectory is foundfirst,and then a stabilizing controller is designed,can become laborious when a whole range of task variants are considered.Standard algo-rithms of reinforcement learning cannot yet offer compelling alternatives to the control theory paradigm,mostly because of the prohibitive effect of the curse of dimensionality. Legged robots often constitute a high-dimensional system, and standard reinforcement learning methods,with their focus on Markov Decision Processes models,usually cannot overcome the exponential growth in state space volume. Most previous work in machine learning for gait domains required either an exhaustive study of the state space[1], or the use of non-specific optimization techniques,such as genetic algorithms[2].In this paper,we wish to take a first step towards efficient reinforcement learning in high-dimensional domains by focusing on periodic tasks.We make the observation that while legged robots have a high-dimensional state space,not every point in the state space represents a viable pose.By definition,a proper gait would always converge to a stable limit cycle,which traces a closed one-dimensional manifold embedded in the 1The interested reader is encouraged to follow the links mentioned in the footnotes to section IV to see movies of our simulations high-dimensional state space.This is true for any system performing a periodic task,regardless of the size of its state space(see also[3],section3.1,and[4],figure19,for a validation of this point in the model discussed below).This observation holds a great promise:a controller that can keep the system close to one particular limit cycle despite minor perturbations(i.e.has a non-trivial basin of attraction)is free to safely ignore the entire volume of the state space. Finding such a stable controller is far from trivial,and amounts to creating a stable gait.However,for our purpose, such a controller can be suboptimal,and may be supplied by a human tele-operating the system,by leveraging on passive dynamic properties of the system(as in section IV-A),or by applying control theory tools(as in section IV-B).In all cases,the one-dimensional manifold traced by the gait of a stable controller can be identified in one cycle of the gait, simply by recording the state of the system at every time step. Furthermore,by querying the controller,we can identify the local policy on and around that manifold.With these two provided,we can create a local representation of the policy which generated the gait by approximating the policy only on and around that manifold,like a ring embedded in state space,and this holds true regardless of the dimensionality of the state space.By representing the original control function in a compact way we may focus our computational effort on the relevant manifold alone,and circumvent the curse of dimensionality as such a parametrization does not scale exponentially with the number of dimensions.This opens a door for an array of reinforcement learning methods(such as policy gradient)which may be used to adapt the initial controller to different conditions,and thus augment the capacities of the system.In this article we report two experiments.Thefirst studies the Compass-Gait walker([9],[10],[11],a system known for its capacity to walk without actuation on a small range of downward slopes.The second experiment uses a simulation of the robot RABBIT[3],a biped robot with knees and a torso,but no feet,which has been studied before by the control theory community[5],[4],[6].Thefirst model has a four-dimensional state space,and the second model has10 state dimensions and4action dimensions.By composing together several controllers,each adapted to a different incline,we are able to create a composite controller that can stably traverse a rough terrain going downhill.The same algorithm was successfully applied to the second system too, although the size of that problem would be prohibitive formost reinforcement learning algorithms.In the following wefirst give a short review of previous work in machine learning,and then explain the technical aspects of constructing a manifold controller,as well as the learning algorithms used.We then demonstrate the effec-tiveness of Manifold Control by showing how it is used to augment the capacities of existing controllers in two different models of bipedal walk.We conclude by discussing the potential of our approach,and offer directions for future work.II.P REVIOUS W ORKThe generalfield of gait design has been at the focus of mechanical engineering for many years,and recent years saw an increase in the contribution from the domain of machine learning.For example,Stilman et al.[7]studied an eight-dimensional system of a biped robot with knees, similar to the one studied below.They showed that in their particular case the dimensionality can be reduced through some specific approximations during different phases.Then, they partitioned the entire volume of the reduced state space into a grid,and performed Q-learning using a simulation model of the system’s dynamics.The result was a robot walker that can handle a range of slopes around the level horizontal plane.In addition,there is a growing interest in recent years in gaits that can effectively take advantage of the passive dynamics(see the review by Collins et al.[8]for a thorough coverage).Tedrake[9]discusses several versions the com-pass gait walker which were built and analyzed.Controllers for the compass gait based on an analytical treatment of the system equations wasfirst suggested by Goswami et al.[10],who used both hip and ankle actuation.Further results were described by Spong and Bhatia[11],where the case of uneven terrain was also discussed.Ramamoorthy and Kuipers[12]suggested hybrid control of walking over irregular terrain by seeking inspiration from human walking.III.M ANIFOLD C ONTROLA.The Basic Control SchemeThe basic idea in manifold control is to focus the com-putational effort on the limit cycle.Therefore,the policy is approximated using locally activated processing elements (PEs),positioned along the manifold spanned by the limit cycle.Each PE defines a local policy,linear in the position of the state relative to that PE.When the policy is queried with a given state x,the local policy of each PE is calculated as:µi(x)=[1(x−c i)T M T]G i(1) where c i is the location of element i,M is a diagonal matrix which determines the scale of each dimension,and G i is an (n+1)-by-m matrix,where m is the action dimension and n is the number of state space dimensions.G i is made of m columns,one for each action dimension,and each column is an(n+1)-sized gain vector.Thefinal policy u(x)is calculated by mixing the local policies of each PEaccordingFig.1.Illustrations of the models used.On the left,the compass-gait walker:the system’s state is defined by the two legs’angles from the vertical direction and the associated angular velocities,for a total of four dimensions. Thisfigure also depicts the incline of the sloped ground.On the right, RABBIT:the system’s state is defined bt the angle of the torso form the vertical direction,the angles between the thighs and the torso,and the knee angles between the shank and the thigh.This model of RABBIT has ten state dimensions,where at every moment one leg isfixed to the ground, and the other leg is free to swing.to a normalized Gaussian activation function,usingσas a scalar bandwidth term:w i=exp(−(x−c i)T M TσM(x−c i)),(2)u(x)= n i=1w iµitraverse a path of higher value(i.e.collect more rewards,or less cost)along its modified limit cycle.1)Defining the Value Function:In the present work we consider a standard nondiscounted reinforcement learning formulation with afinite time horizon and no terminal costs. More accurately,we define the Value Vπ(x0)of a given state x0under afixed policyπ(x)as:Vπ(x0)= T0r(x t,π(x t))dt(4) where r(x,u)is the reward determined by the current state and the selected action,T is the time horizon,and x t is the solution of the time invariant ordinary differential equation ˙x=f(x,π(x))with the initial condition x=x0,so thatx t= t0f(xτ,π(xτ))dτ.(5) 2)Approximating the Policy Gradient:With C being the locations of the processing elements,and G being the set of their local gains,we make use of a method,due to[14],of piecewise estimation of the gradient of the value function at a given initial state x0with respect to the parameter set G. As Munos showed in[14],from(4)we can write∂V∂G,(6) and for the general form r=r(x,u)we can decompose∂r/∂G as∂r∂u ∂u∂x∂xFig.2.The path used to test the compass gait walker,and an overlay of the walker traversing this path.Note how the step length adapts to the changing incline.the forward swing,but will undergo an inelastic collision with thefloor during the backward swing.At this point it will become the stance leg,and the other leg will be set free to swing.The entire system is placed on a plane that is inclined at a variable angleφfrom the horizon.In the interests of brevity,we omit a complete description of the system dynamics here,referring the interested reader to the relevant literature[15],[10],[11].Although previous work considered actuation both at the hip and the ankle,we chose to study the case of actuation at the hip only.The learning phase in this system was done using simple stochastic gradient ascent,rather than the elaborate policy gradient estimation described in section III-B.2.The initial manifold was sampled at an incline ofφ=−0.05(the initial policy is the zero policy,so there were no approximation errors involved).One shaping iteration consisted of the following:first,G was modified to G tent=G+ηδG, withη=0.1andδG drawn at random from a multinormal distribution with unit covariance.The new policy’s perfor-mance was measured as a sum of all the rewards along20 steps.If the value of this new policy was bigger than the present one,it was adopted,otherwise it was rejected.Then, a newδG was drawn,and the process repeated itself.After 3successful adoptions,the shaping iterations step concluded with a resampling of the new controller,and the incline was decreased by0.01.After10shaping iteration steps,we had controllers that could handle inclines up toφ=−0.14.After another10 iteration steps with the incline increasing by0.005,we had controllers that could handle inclines up toφ=0.0025(a slight uphill).This range is approxmately double the limit of the passive walker[15].Finally,we combined the various controllers into one composite controller.This new controller used1500charts to span a two-dimensional manifold embedded in the four-dimensional state space.The performance of the composite controller was tested on an uneven terrain where the incline was gradually changed fromφ=0toφ=0.15radians, made of“tiles”of variable length whose inclines were0.01 radians apart.figure III-B.2shows an overlay of the walker’s downhill path.A movie of this march is available online.2B.The Uphill-Walking RABBIT RobotWe applied manifold control also to simulations of the legged robot RABBIT,using code from Prof.Jessy Grizzle that is freely available online[16].RABBIT is a biped robot with a torso,two knees and no feet(seefigure1b.),and is actuated at four places:both hip joins(where thighs are actuated against the torso),and both knees(where shanks are actuated against the thighs).The simulation assumes a stance leg with no slippage,and a swing leg that is free to move at all directions until it collides inelastically with the floor,and becomes the stance leg,freeing the other leg to swing.This robot too is modeled as a nonlinear system with impulse effects.Again,we are forced to omit a complete reconstruction of the model’s details,and refer the interested reader to[4],equation8.This model was studied extensively by the control theory community.In particular,an optimal desired signal was derived in[6],and a controller that successfully realizes this signal was presented in[4].However,all the efforts were focused on RABBIT walking on even terrain.We sought a way to augment the capacities of the RABBIT model,and allow it to traverse a rough,uneven terrain.We found that the controller suggested by[4]can easily handle negative (downhill)incline of0.2radiand and more,but cannot handle positive(uphill)inclines.3.Learning started by approximating the policy from[4]as a manifold controller,using400processing elements with a mean distance of about0.03state space length units.The performance of the manifold controller was indistinguishable to the naked eye from the original controller,and perfor-mance,as measured by the performance criterion C3in[6] (the same used by[4]),was only1%worse,probably due to minor approximation errors.The policy gradient was estimated using(6),according to a simple reward model:r(x,u)=10v x hip−1Fig.3.The rough terrain traversed by RABBIT.Since this model has knees,it can walk both uphill and downhill.Note how the step length adapts to the changing terrain.The movie of this parade can be seen at tt http:/2b8sdm,which is a shortcut to the YouTube website.where v xhip is the velocity of the hip joint(where the thighand the torso meet)in the positive direction of the X-axis, and u max is a scalar parameter(in our case,chosen to be 120)that tunes the nonlinear action penalty and promotes energetic efficiency.After the initial manifold controller was created,the system followed a fully automated shaping protocol for 20iterations:at every iteration,∂V/∂G was estimated, andηwasfixed to0.1%of|G|.This small learning rate ensured that we don’t modify the policy too much and lose stability.The modified policy,assumed to be slightly better, was then tested on a slightly bigger incline(the veryfirst manifold controller was tried on an incline of0rad.,and in every iteration we increased the incline in0.003rad.).This small modification to the model parameters ensured that the controller can still walk stably on the incline.If stability was not lost(as was the case in all our iterations),we resampled u(·;C,G new)so that C adj overlapped the limit cycle of the modified system(with the new policy and new incline),and the whole process repeated.This procedure allowed a gradual increase in the system’s maximal stable incline.Figure4depicts the evolution of the stability margins of every ring along the shaping iteration:for every iteration we present an upper(and lower)bound on the incline for which the controller can maintain stability.Thiswas tested by setting a test incline,and allowing the system to run for10 seconds.If no collapse happened by this time,the test incline was raised(lowered),until an incline was found for which the system can no longer maintain stability.As this picture shows,our automated shaping protocol does not maintain a tight control on the stability margins-for most iterations,a modest improvement is recorded.The system’s nonlinearity is well illustrated by the curious case of iteration9,where the same magnitude ofδG causes a massive improvement, despite the fact that the control manifold itself didn’t change dramatically(seefigure5).The converse is also true for some iterations(such as17and18)there is a decrease in the stability margins,but this is not harming the overall effectiveness,since these iterations are using training data obtained at an incline that is very far from the stability Fig.4.Thisfigure shows the inclines for which each iteration could maintain a stable gait on the RABBIT model.The diagonal line shows the incline for which each iteration was trained.Iteration0is the original controller.The initial manifold control approximation degrades most of the stability margin of the original control,but this is quickly regained through adaptation.Note that both the learning rate and the incline change rate were held constant through the entire process.The big jump in iteration 9exemplifies the nonlinearity of the system,as small changes may have unpredictable results,in this case,for the best.margin.Finally,three iterations were composed together,and the resulting controller successfully traversed a rough terrain that included inclines from−0.05to0.15radians.Figure3 shows an overlay image of the rough path.V.C ONCLUSION AND F UTURE W ORKIn this paper we present a compact representation of the policy for periodic tasks,and apply a trajectory-based policy gradient algorithm to it.Most importantly,the methods we present do not scale exponentially with the number of dimensions,and hence allow us to circumvent the curse of dimensionality in the particular case of periodic tasks.By following a gradual shaping process,we are able to create robust controllers that augment the capacities of existing systems in a consistent way.33.54−1.5−0.2anglea n g . v e l.anglea n g . v el.anglea n g . v e l.anglea n g . v el.anglea n g . v e l.anglea n g . v el.anglea n g . v e l.anglea n g . v el.anglea n g . v e l.anglea n g . v el.anglea n g . v e l.anglea n g . v el.anglea n g . v e l.anglea n g . v e l.Fig.5.A projection of the manifold of several stages of the shaping process for the RABBIT model.The top row shows the angle and angular velocity between the torso and the stance thigh,and the bottom row shows the angle and angular velocity of the knee of the swing leg.Every two consecutive iterations are only slightly different from each other.Throughout the entire shaping process,changes accumulate,and new solutions emerge.Manifold control may also be used when the initial con-troller is profoundly suboptimal 4.It is also important to note that the rough terrain was traversed without informing the walker of the current terrain.We may say that the walkers walked blindly on their rough path.This demonstrates how stable a composite manifold controller can be.However,in some practical applications it could be beneficial to represent this important piece of information explicitly,and select the most appropriate ring at every step.We believe that the combination of local learning and careful shaping holds a great promise to many applications of periodic tasks,and hope to demonstrate it through future work on even higher-dimensional systems.Future research directions could include methods that allow second-order convergence,and learning a model of the plant.R EFERENCES[1]M.Stilman,C.G.Atkeson,J.J.Kuffner,and G.Zeglin,“Dynamicprogramming in reduced dimensional spaces:Dynamic planning for robust biped locomotion,”in Proceedings of the 2005IEEE Interna-tional Conference on Robotics and Automation (ICRA 2005),2005,pp.2399–2404.[2]J.Buchli, F.Iida,and A.Ijspeert,“Finding resonance:Adaptivefrequency oscillators for dynamic legged locomotion,”in Proceedings of the IEEE/RSJ International Conference on Intelligent Robots and Systems (IROS).IEEE,2006,pp.3903–3909.[3] C.Chevallereau and P.Sardain,“Design and actuation optimization ofa 4-axes biped robot for walking and running,”in Proceedings of the IEEE International Conference on Robotics and Automation (ICRA),2000.[4] F.Plestan,J.W.Grizzle,E.Westervelt,and G.Abba,“Stable walkingof a 7-dof biped robot,”IEEE Trans.Robot.Automat.,vol.19,no.4,pp.653–668,Aug.2003.4theinterested reader is welcome to see other results of manifold learning on a 14-dimensional system at /2h3qny and /2462j7.[5] C.Sabourin,O.Bruneau,and G.Buche,“Experimental validation ofa robust control strategy for the robot rabbit,”in Proceedings of the IEEE International Conference on Robotics and Automation (ICRA),2005.[6] C.Chevallereau and Y .Aoustin,“Optimal reference trajectories forwalking and running of a biped robot,”Robotica ,vol.19,no.5,pp.557–569,2001.[7]M.Stilman,C.G.Atkeson,J.J.Kuffner,and G.Zeglin,“Dynamicprogramming in reduced dimensional spaces:Dynamic planning for robust biped locomotion,”in Proceedings of the 2005IEEE Interna-tional Conference on Robotics and Automation (ICRA 2005),2005,pp.2399–2404.[8]S.H.Collins,A.Ruina,R.Tedrake,,and M.Wisse,“Efficient bipedalrobots based on passive-dynamic walkers,”Science ,pp.307:1082–1085,February 2005.[9]R.L.Tedrake,“Applied optimal control for dynamically stable leggedlocomotion,”Ph.D.dissertation,Massachusetts Institute of Technol-ogy,August 2004.[10] A.Goswami, B.Espiau,and A.Keramane,“Limit cycles in apassive compass gait biped and passivity-mimicking control laws,”Autonomous Robots ,vol.4,no.3,pp.273–286,1997.[11]M.W.Spong and G.Bhatia,“Further results on the control of the com-pass gait biped,”in Proceedings of the 2003IEEE/RSJ International Conference on Intelligent Robots and Systems (IROS 2003),vol.2,2003,pp.1933–1938.[12]S.Ramamoorthy and B.Kuipers,“Qualitative hybrid control ofdynamic bipedal walking,”in Robotics :Science and Systems II ,G.S.Sukhatme,S.Schaal,W.Burgard,and D.Fox,Eds.MIT Press,2007.[13]S.Schaal and C.Atkeson,“constructive incremental learning fromonly local information,”neural computation ,no.8,pp.2047–2084,1998.[14]R.Munos,“Policy gradient in continuous time,”Journal of MachineLearning Research ,vol.7,pp.771–791,2006.[15] A.Goswami,B.Thuilot,and B.Espiau,“Compass-like biped robotpart i:Stability and bifurcation of passive gaits,”INRIA,Tech.Rep.2996,October 1996.[16] E.Westervelt, B.Morris,and J.Grizzle.(2003)Five link walker.IEEE-CDC Workshop:Feedback Control of Biped Walking Robots.[Online].Available:/2znlz2。
参考文献(人工智能)
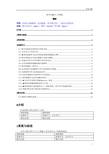
参考文献(人工智能)曹晖目的:对参考文献整理(包括摘要、读书笔记等),方便以后的使用。
分类:粗分为论文(paper)、教程(tutorial)和文摘(digest)。
0介绍 (1)1系统与综述 (1)2神经网络 (2)3机器学习 (2)3.1联合训练的有效性和可用性分析 (2)3.2文本学习工作的引导 (2)3.3★采用机器学习技术来构造受限领域搜索引擎 (3)3.4联合训练来合并标识数据与未标识数据 (5)3.5在超文本学习中应用统计和关系方法 (5)3.6在关系领域发现测试集合规律性 (6)3.7网页挖掘的一阶学习 (6)3.8从多语种文本数据库中学习单语种语言模型 (6)3.9从因特网中学习以构造知识库 (7)3.10未标识数据在有指导学习中的角色 (8)3.11使用增强学习来有效爬行网页 (8)3.12★文本学习和相关智能A GENTS:综述 (9)3.13★新事件检测和跟踪的学习方法 (15)3.14★信息检索中的机器学习——神经网络,符号学习和遗传算法 (15)3.15用NLP来对用户特征进行机器学习 (15)4模式识别 (16)4.1JA VA中的模式处理 (16)0介绍1系统与综述2神经网络3机器学习3.1 联合训练的有效性和可用性分析标题:Analyzing the Effectiveness and Applicability of Co-training链接:Papers 论文集\AI 人工智能\Machine Learning 机器学习\Analyzing the Effectiveness and Applicability of Co-training.ps作者:Kamal Nigam, Rayid Ghani备注:Kamal Nigam (School of Computer Science, Carnegie Mellon University, Pittsburgh, PA 15213, knigam@)Rayid Ghani (School of Computer Science, Carnegie Mellon University, Pittsburgh, PA 15213 rayid@)摘要:Recently there has been significant interest in supervised learning algorithms that combine labeled and unlabeled data for text learning tasks. The co-training setting [1] applies todatasets that have a natural separation of their features into two disjoint sets. We demonstrate that when learning from labeled and unlabeled data, algorithms explicitly leveraging a natural independent split of the features outperform algorithms that do not. When a natural split does not exist, co-training algorithms that manufacture a feature split may out-perform algorithms not using a split. These results help explain why co-training algorithms are both discriminativein nature and robust to the assumptions of their embedded classifiers.3.2 文本学习工作的引导标题:Bootstrapping for Text Learning Tasks链接:Papers 论文集\AI 人工智能\Machine Learning 机器学习\Bootstrap for Text Learning Tasks.ps作者:Rosie Jones, Andrew McCallum, Kamal Nigam, Ellen Riloff备注:Rosie Jones (rosie@, 1 School of Computer Science, Carnegie Mellon University, Pittsburgh, PA 15213)Andrew McCallum (mccallum@, 2 Just Research, 4616 Henry Street, Pittsburgh, PA 15213)Kamal Nigam (knigam@)Ellen Riloff (riloff@, Department of Computer Science, University of Utah, Salt Lake City, UT 84112)摘要:When applying text learning algorithms to complex tasks, it is tedious and expensive to hand-label the large amounts of training data necessary for good performance. This paper presents bootstrapping as an alternative approach to learning from large sets of labeled data. Instead of a large quantity of labeled data, this paper advocates using a small amount of seed information and alarge collection of easily-obtained unlabeled data. Bootstrapping initializes a learner with the seed information; it then iterates, applying the learner to calculate labels for the unlabeled data, and incorporating some of these labels into the training input for the learner. Two case studies of this approach are presented. Bootstrapping for information extraction provides 76% precision for a 250-word dictionary for extracting locations from web pages, when starting with just a few seed locations. Bootstrapping a text classifier from a few keywords per class and a class hierarchy provides accuracy of 66%, a level close to human agreement, when placing computer science research papers into a topic hierarchy. The success of these two examples argues for the strength of the general bootstrapping approach for text learning tasks.3.3 ★采用机器学习技术来构造受限领域搜索引擎标题:Building Domain-specific Search Engines with Machine Learning Techniques链接:Papers 论文集\AI 人工智能\Machine Learning 机器学习\Building Domain-Specific Search Engines with Machine Learning Techniques.ps作者:Andrew McCallum, Kamal Nigam, Jason Rennie, Kristie Seymore备注:Andrew McCallum (mccallum@ , Just Research, 4616 Henry Street Pittsburgh, PA 15213)Kamal Nigam (knigam@ , School of Computer Science, Carnegie Mellon University Pittsburgh, PA 15213)Jason Rennie (jr6b@)Kristie Seymore (kseymore@)摘要:Domain-specific search engines are growing in popularity because they offer increased accuracy and extra functionality not possible with the general, Web-wide search engines. For example, allows complex queries by age-group, size, location and cost over summer camps. Unfortunately these domain-specific search engines are difficult and time-consuming to maintain. This paper proposes the use of machine learning techniques to greatly automate the creation and maintenance of domain-specific search engines. We describe new research in reinforcement learning, information extraction and text classification that enables efficient spidering, identifying informative text segments, and populating topic hierarchies. Using these techniques, we have built a demonstration system: a search engine forcomputer science research papers. It already contains over 50,000 papers and is publicly available at ....采用多项Naive Bayes 文本分类模型。
翻译者要注意历史和文化上的差异

英语学习者一般愿做英译汉题目,怕做汉译英题目。
由于历史和文化上的差异,汉英词语之间存在着或显或隐的翻译上的陷阱,导致汉译英远比英译汉易于出错。
用鲁迅的话说,就是“词典不离手,冷汗不离身。
”这提醒译者必须对这些差异心中有数,然后再调和这些差异,“摆平”这些差异。
为此,要迈好下面八道坎儿,时刻要留心这八条“戒律”—只想为你擦亮满天星光,以免在熟路上“迷失方向”。
一、戒“从一而终”。
汉语言简意赅,句子灵活,往往是一个汉语词汇对应N个英语词汇,具体到在本句中应该采用那个意项,务必抓住精神实质,不可以不变应万变。
至于怎么应变,这就是显示译者功力的地方了。
比如:都是“问题”,下面的翻译各不相同:共同关心的问题questions of common interest解决问题solve a problem问题的关键the heart of the matter关键问题a key problem原则问题a question / matter of principle悬而未决的问题an outstanding issue没有什么问题Without any mishap摩托车有点问题。
Something is wrong with the motorcycle.问题不在这里。
That is not the point.最近揭发出相当严重的贪污、受贿和官僚主义问题。
Serious cases of embezzlement, bribery, and bureaucracy have been brought to light recently.译者要掌握这种汉英翻译中的“游击战术”,翻译家应是不同“文化王国”边境线上的“游击战略家”。
沙博里将《水浒传》译为:Outlaws of the Marsh(沼泽地上的亡命之徒)。
杨宪益译将屈原的《国殇》译为:For Those Fallen for Their Country,北外出版社将《儒林外史》译为:The Scholars。
词汇翻译练习知识讲解
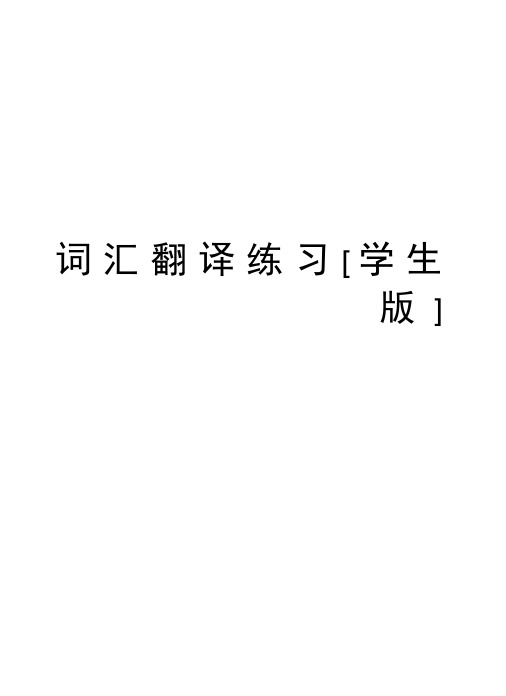
词汇翻译练习[ 学生版]Name & No.: Exercises for Bus in ess En glish Tran slati on练习之词义选择(1)1. Tha nk you for your letter refere nee DT/Zi No. 102, of 29th, March.感谢贵方3月29日编号为DT/Zi, No.102的来信。
2. With only one order from ABC Co., Ltd., we regret we cannot give you a referenee from long experie nee.我们仅接受ABC有限公司的一笔定货,很遗憾我们不能提供具有长期交往经历的资信情况。
3. Referenee is made to your Sales Confirmation No. 1529.现谈到贵方的第1529号销售确认书。
4. The Buyer asks for credit and has given the Bank of China, Beijing as a referenee.买方要求记账交易,并提出中国银行北京分行作为资信备询人。
5. The Sales Company is given the same power with referenee to apportioning the commissi on.关于佣金的分配问题,应授予销售公司同样的权利。
6. A referenee to your records will show that we have more than once asked you to establish L/C against S/C No. 2523.查阅你方记录可以看出,我方已多次催促,要求你方对第2523号销售确认书开立信用证7. The time limit for inspection and claim is 60 days after discharge of the cargo at the port of dest in ati on.检验与索赔的期限为货物卸至目的港后60天。
基于专家系统的CSE-UCLA评价BLCS模型(Bruner,Local.,Scaffolding)在数学教学中的有效性(IJEME-V7-N4-1)
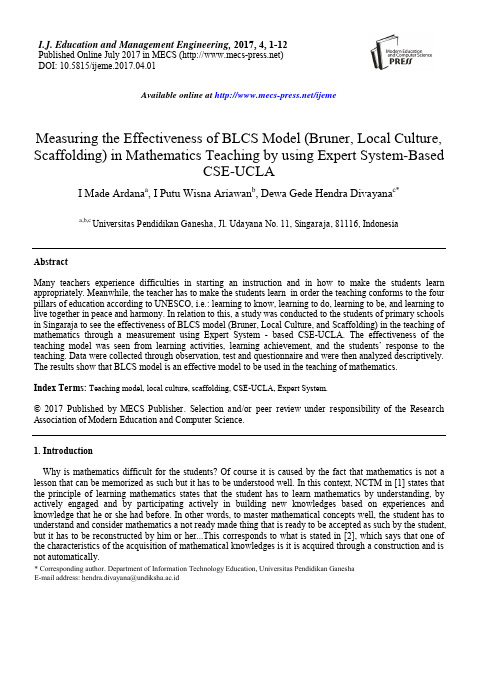
I.J. Education and Management Engineering, 2017, 4, 1-12Published Online July 2017 in MECS ()DOI: 10.5815/ijeme.2017.04.01Available online at /ijemeMeasuring the Effectiveness of BLCS Model (Bruner, Local Culture, Scaffolding) in Mathematics Teaching by using Expert System-BasedCSE-UCLAI Made Ardana a, I Putu Wisna Ariawan b, Dewa Gede Hendra Divayana c*a,b,c Universitas Pendidikan Ganesha, Jl. Udayana No. 11, Singaraja, 81116, IndonesiaAbstractMany teachers experience difficulties in starting an instruction and in how to make the students learn appropriately. Meanwhile, the teacher has to make the students learn in order the teaching conforms to the four pillars of education according to UNESCO, i.e.: learning to know, learning to do, learning to be, and learning to live together in peace and harmony. In relation to this, a study was conducted to the students of primary schools in Singaraja to see the effectiveness of BLCS model (Bruner, Local Culture, and Scaffolding) in the teaching of mathematics through a measurement using Expert System - based CSE-UCLA. The effectiveness of the teaching model was seen from learning activities, learning achievement, and the students‟ response to the teaching. Data were collected through observation, test and questionnaire and were then analyzed descriptively. The results show that BLCS model is an effective model to be used in the teaching of mathematics.Index Terms: Teaching model, local culture, scaffolding, CSE-UCLA, Expert System.© 2017 Published by MECS Publisher. Selection and/or peer review under responsibility of the Research Association of Modern Education and Computer Science.1.IntroductionWhy is mathematics difficult for the students? Of course it is caused by the fact that mathematics is not a lesson that can be memorized as such but it has to be understood well. In this context, NCTM in [1] states that the principle of learning mathematics states that the student has to learn mathematics by understanding, by actively engaged and by participating actively in building new knowledges based on experiences and knowledge that he or she had before. In other words, to master mathematical concepts well, the student has to understand and consider mathematics a not ready made thing that is ready to be accepted as such by the student, but it has to be reconstructed by him or her...This corresponds to what is stated in [2], which says that one of the characteristics of the acquisition of mathematical knowledges is it is acquired through a construction and is not automatically.* Corresponding author. Department of Information Technology Education, Universitas Pendidikan GaneshaE-mail address: hendra.divayana@undiksha.ac.idThe implication of the principle of learning mathematics is that the student has to participate actively in constructing the concepts being learned. That student can learn well depends very much on the learning experiences provided by the teacher daily in the classroom [3] states that to be able to provide learning that has a high quality, the teacher has: (1) to understand deeply the mathematics learning material being learned,; (2) to understand how the student learns mathematics, such as to understand such things as his or her attentiveness and misconceptions; and (3) to select tasks that make learning meaningful like when the student has been trained to think, to ask questions, to solve problems, and to discuss ideas, strategies, and solutions that he or she has acquired.In addition, Steffe in [4] states that: cognitive theory in mathematics learn ing makes the teacher‟s role not just as the one who transfers knowledge to the student, but as mediator and facilitator in learning. Thus the roles of the teacher are: (1) to facilitate the process of constructing knowledge by making information meaningful and relevant to the student (2) to give opportunities to the student to express or apply his or her own ideas and to guide him or her to consciously use his or her own learning strategies; (3) to develop and accept the student‟s autonomy and initiative; (4) to provide activities that can devel op the student‟s curiosity and help him or her to express ideas and communicate ideas to other people; (5) to write tasks by using cognitive terms i.e. to ask the student to classify, analyze, and predict; and (6) to provide the opportunities to the student to respond to the learning process, and the opportunities to think after giving a question.In this connection, a discussion will focus on how to combine Bruner‟s theory, local culture (the concept jengah)and scaffolding in BLCS model to make mathematics teaching process effective.2.Literature ReviewA. Bruner’s Theory in TeachingBruner assumes that a child‟s intellectual development occurs through three phases, i.e.: preoperational, concrete operational, and formal operational. Although Bruner‟s s tages refer to Piaget‟s cognitive development, but he does not agree that a child‟s cognitive development depends on his or her age.Bruner states that a child‟s cognitive development is ge nerally related to learning. In the preoperational stage, the child‟s mental activity is developing a relation between experience and action and problem solving is done through trial and error. At the concrete operational stage, the child can already solve problems both directly and indirectly. Direct problem solving is meant solving a problem through manipulating concrete objects. While the indirect problem solving is done by manipulating symbols that the child has in his or her mind. However, the child cannot solve the problem easily because he or she has no experience or the cannot describe the problem solving strategy that he or she uses.Based on the theory above, Bruner in [5] and [6] develops a learning theory that consists of three progressive stages, i.e.: (1) enactive - learning by doin g/acting; (2) Iconic - learning by means of images and pictures(learning through meanings and pictures, scheme, graphics, tables, diagrams, etc.); (3) symbolic - learning by means of words or numbers.The three modes have a hierarchical relation, which means that symbolic representational meaning is more abstract than iconic representation, and iconic representation is more abstract than enactive representation. In learning mathematics the three modes are very important. However, learning does not need to start from the lowest (enactive) but depends on the extent of the student‟s basic idea knowledge about what he or she will learn.The representation modes introduced by Bruner area very important in the implementation of BLCS model as what the researchers have done at the primary school in the course of a research particularly in the teaching to find a rule in addition and subtraction of numbers. For example, in finding a relation between the addition of a positive number and a positive number and the subtraction of a positive number by a negative number. Finding a rule through this model of teaching was done by helping the student learn through a transformationactivity from model, words, and symbols. In the model activity, the student was active through enactive mode. Then the student tried to transform it into every day words/ sentences (iconic) and finally he or she transformed it into a symbol (symbolic). Before the transformation process was done, first the teacher agreed with the students like: (i) Positive number means “go forward ”(ii) Negative number means “retreat”; (iii) added means “continued with” and (iv) Subtraction meaning “turn right”. This agreement was a modification of the agreement on addition and subtraction of round numbers as stated in [7] i.e., (i) Positive number means “move forward”; (ii) Negative number means “retreat”; (iii) Added means “go straight ahead”; and (iv) Subtracted means “turn right ”.B. Local Culture (The Concept of Jengah)It has already been known that teaching occurs in a cultural community and the result of learning will be applied to a certain cultural community and the result of learning will also be applied to a certain cultural community. In this context, teaching will be interesting and meaningful if it is done based on/oriented toward a culture. This corresponds to the view of Brooks & Brooks in [8] that state that a culture-based approach can give opportunities to the student to create meaning and to attain an integrated understanding of scientific information he or she acquired and to apply it in the context of his or her cultural community problems. BLCS model some Balinese cultural concepts as stated by Widja in [9] were used. Widja in [9] states that the basic concepts such as rwabhineda, tri-hita-karana, and desa-kala-patra can be related to the process of education to support development. Based on the opinion above, the main concepts need to be considered in the model of teaching: (1) the rwabhineda concept refers to the effort to keep a balance in this life through binary classification that has complementary elements (good and bad natures in a thing) This concept is very useful in this teaching model especially when the student learns in a cooperative group. BLCS model stresses mutual respect, respecting the opinion that is stated by every group member; (2) the concept of tri-hita-karana contains the elements of parahyangan(the relationship of human beings and God), pawongan (the relationship of human beings and themselves and their fellow human beings), and palemahan(the relationship of human beings and their environment). The application of this concept is generally seen in every activity which ought to be started with prayer. The same is true with a teaching using BLCS model. Besides, this concept is very useful in the learning activity especially in realizing a positive relationship among members of a group and its environment; (3) the concept of desa-kala-patra. The concept of desa-kala-patra according to [10] is the concept of space, time and human that basically contains the adaptation and harmony and ability to accept diversity in unity which is the same as the slogan of Bhineka Tunggal Ika. This concept provides a flexible and harmonious foundation in communication among group members without looking at strengthening differences that they have especially in their learning ability; (4) the concept of jengah in the cultural context has the connotation of enthusiasm. This concept needs to be developed in the student so that everything learned is relatively easy to understand. The concept jengah will be able to motivate it, he student in learning since the concept of jengah can become a motivator of every activity that will be done, can give a direction of the activity toward the objective to be attained, and is able to determine the actions to be done that are harmonious with the objective in order to achieve the objective.The concept of jengah is the foundation of BLCS model, beside other Balinese cultural concepts in a cooperative activity. In this case, the student is planned to interact in order that he or she, in an interaction, can respect others and others can respect him or her, does not sharpen the existing differences and help each other, and is motivated by the concept jengah through sentences containing suggestions uttered by the teacher. The sentences that contain suggestions include: “You must be able to do it”, “Others can and you surely can do it”, “No problems that cannot be solved” etc. The concepts above are concepts that support the implementation of teaching in BLCS model. Thus, these concepts, especially the concept of jengah are maximized in BLCS model with the purpose of preventing the emergence of the concept of nasib(fate) that can hinder learning process. This concept of nasib can extremely minimize the student‟s ability in learning, that can manifest as apassive attitude and easiness to surrender.C. ScaffoldingVygotsky in [11] defines Nearest Development Zone (ZPD) as the distance between actual level of development, that is determined through autonomous solution to a problem and child potential development level, that is determined through adult assisted solution to a problem or cooperation with peers. It can be thought that ZPD is a difference between what a child can do alone and what he or she can do with the help from adults (scaffolding). The location of ZPD stated [12] can be illustrated as shown in Fig. 1.IncreasingTaskDifficultyFig.1. Location of ZPD (adopted from Teaching in the Zone: An Introduction to Working within the Zone of Proximal Development (ZPD) to Drive Effective Early Childhood Instruction; 2012)Vygotsky believes that learning occurs at the time a child is working in his or her ZPD, since ZPD is an area between an actual level of development that is defined as ability to solve a problem by oneself and potential level of development that is defined as problem solving under adult‟s guidance or peers who are more able. Tasks are not things that a child can do alone but can be done with the help from peers or adults who are more competent in the form of scaffolding.Scaffolding refers to help given by the peers or more able adults in [11] it is stated that to provide scaffolding means to give to the child a great amount of support during the initial stages of learning and then minimize help and chance to the child to assume responsibility which is increasingly greater right after he or she can perform the task alone. In BLCS model, if the student experiences difficulty in constructing a concept, he or she is given a necessary support until he or she can actually orient himself or herself toward the concept being learned.D. CSE-UCLACSE-UCLA evaluation model developed by Alkin in [15] has five stages of evaluation, namely system assessment, program planning, program implementation, program improvement, and program certification.C SE-UCLA model is an evaluation model with five evaluation dimensions (system assessment, program planning, program implementation, program improvement, program certification) and are suitable to be used to evaluate service programs that help human life, such as: library program, bank, cooperative, e-government, e-learning, etc [16].CSE-UCLA model is a model evaluation has five dimensions of evaluation, among other systems assessmentthat provide information about the state of the system, the program planning which enables selection of the program particular to meet the needs of the program, program implementation that prepare information to introduce the program, program improvement that provides information of function/program performance, program certification which gives information about the benefit or usefulness of the program[17].On the basis of some views above, a synthesis can be made that in general CSE-UCLA model is an evaluation model that has five evaluation dimensions, which include system assessment, program planning, program implementation, program improvement, and program certification that is suitable to be used to evaluate service programs that help human life.E. Expert SystemIn [18], expert system is an artificial intelligence system that combines knowledge base with inference engine so that it can adopt the ability of the experts into a computer, so the computer can solve problems such as the often performed by experts.In [19], expert system is a computer application program that tries to imitate an expert‟s reasoning in solving problems specifically.In [20], expert system is an artificial intelligence program that combines knowledge base with inferential system or an effort to imitate an expert.In [21], an expert system is one branch of artificial intelligence to learn how to …adopt‟ an expert how to think and reason to solve a problem and make a decision or the conclusion of a number of facts.Based on some views above a synthesis can be generally made that CSE-UCLA model is an evaluation model that has five dimensions which include system assessment, program planning, program implementation, program improvement, and program certification that are suitable to be used to evaluate service programs that help human life.3.MethodologyA. Object and locations of the Study1)The object of the study was BLCS model (Bruner, Local Culture, and Scaffolding).2)The locations were primary schools in Singaraja, Bali.B. Subject of the StudyThe subjects of the study were 71 elementary school students in Singaraja Town.C. Type of DataThe data in this study were quantitative and qualitative data.D. Technique of data collectionThe data were collected through observation, test and questionnaire.E. Technique of Data AnalysisThe data of the study were analyzed descriptively.4.Result and DiscussionA. Result1.Student Learning ActivityResult that is related to student learning activity is presented as shown in Fig. 2.Fig.2. Student Activity in BLCSFig. 2 shows that for every question about student learning activity, only some students answered no (in red), and the rest answered yes (in blue). Through a computation the student learning activity average was 95.2%. This means that the students‟ activity in learning through BLCS model was very high.2.Learning AchievementThe student learning achievement in the implementation activity can be reported as shown in Fig. 3.Fig.3. Average, Absorption Rate, and Learning Mastery for Each MeetingFig. 3 shows average, absorption rate (DS); and learning mastery (LM) have exceeded the criterion specified, namely, Absorption Rate (AR) = 65%, and Learning Mastery (LM)= 85%. Through a further computation, generally, Average = 75.8, Absorption Rate = 75.80%; Absorption Rate = 75.80%; and Learning Mastery (LM) = 93.00%, thus it can be concluded that the students‟ learning achievement falls into good category.3.Student Response to BLCS Model ImplementationFig.4. Student Response to BLCS Model ImplementationFig. 4 shows that almost all students gave a positive response to each question in the questionnaire Student Response to BLCS model implementation and all exceeded 85% , thus it can be concluded that the studen ts‟ response was positive to each question in the questionnaire Student Response to BLCS model implementation.4.The result of the measurement of BLCS Model in Mathematics Teaching Using Expert System-BasedCSE-UCLAThe result of the measurement of the effectiveness of BLCS model in Mathematics teaching using expert-system-based CSE - UCLA in Mathematics teaching can be shown in Table 1 as follows.Table 1. Result of the Measurement of the Effectiveness of BLCS Teaching Model in Mathematics Teaching Using Expert System-Based CSE-UCLANo Evaluation Component Aspect to be EvaluatedModel 1 Model 2 Conventional CF BLCS Teaching CF1 System Assessment Learning activity 76.0 0.4 85.0 0.8 Learning achievement 75.0 0.2 87.2 0.8 Student response 70.0 0.2 81.0 0.8 Average 73.7 0.3 84.4 0.82 Program Planning Learning activity 72.5 0.2 84.0 0.6 Learning achievement 71.0 0.2 86.5 0.8 Student response 70.5 0.2 82.5 0.8 Average 71.3 0.2 84.3 0.73 Program Implementation Learning activity 72.0 0.2 90.0 0.8 Learning achievement 71.0 0.2 91.7 1.0 Student response 71.0 0.2 87.8 0.8 Average71.3 0.2 89.8 0.9No Evaluation Component Aspect to be EvaluatedModel 1 Model 2 Conventional CF BLCS Teaching CF4 Program Improvement Learning activity 76.0 0.4 85.2 0.8 Learning achievement 74.0 0.2 86.7 0.8 Student response 72.0 0.2 83.2 0.8 Average 74.0 0.3 85.0 0.85 Program Certification Learning activity 78.0 0,6 87.6 0.8 Learning achievement 77.5 0.4 88.2 0.8 Student response 73.0 0.4 85.5 0.8 Average 76.2 0.5 87.1 0.8Total Average73.3 0.3 86.1 0.8The result of the measurement can be made clearer through Fig.5. belowFig.5. Result of the Measurement of Effectiveness between Conventional Teaching Model and BLCS Teaching Model by using Expert System-Based CSE-UCLAB. DiscussionThe syntax of BLCS model consists of 4 stages, namely Stage 1, introdu ction; Stage 2, Use of Bruner‟s theory, local culture, and scaffolding; Stage 3, Presentation and conclusion drawing, and; Stage 4, Evaluation as presented in Fig. 6.Fig.6. Syntax of BLCS ModelArdana, in [3] states that the initial phase stresses on teaching process starting with the teacher leading the students to make a connection between the learning task that they are doing and their past experiences, in terms of academic, personal and cultural experiences. The aim is to involve the students in learning by activating their curiosity, attracting their attention to the problem they are facing, or asking some questions that make the students think. In addition, this phase gives the chance to the teacher and the students through evaluation activity to identify prior concepts that they have that are related to the new concepts that they are learning, do their prior concepts match with the new ones or not (misconceptions). In this phase the teacher identifies the strengths and weaknesses of every individual or group. This is meant for the teacher to facilitate the adjustment of the teaching activity being implemented with the learning experience of each individual or group in relation to the knowledge being learned. When the students have not been involved in learning, the teacher will dig the students‟ prior knowledges by giving them triggering questions that fit the students‟ abilities that enable them to think and at the same time activate their concept jengah through suggestion sentences: “I believe that you can...must b e able to do it”; “others can ... You / can too”; “All things can be solved.. if we try our best”, etc. With the possession of a good prior knowledge, the students are ready to communicate and will keep calm in following the lesson and their communication will be directed and will be prevented from over communication. This Activity in this phase needs to be done because Mathematics teaching can proceed well when the students‟ prior knowledges that are related to the material being learned are understood well so that they will facilitate assimilation in the students. In this regard, Piaget [1] states that assimilation is a process of integrating new information with the existing cognitive structure in the student‟s mind. It means that the student‟s prior knowledge is something that needs to be taken into consideration in teaching [13] states that one important factor that can influence the child‟s learning is what he or she has known. [2] state s that to be more exact, we need to focus our attention on the student‟s schemata in the ass imilation. [14] stresses that every teacher has to be aware first of what the students‟ preconcept ions and experiences look like and then he or she has to adjust the lesson and the method of teaching to the “pre”-knowledges themselves. This means that in order for the students‟ schemata can be built and developed, the teacher needs to relate their preconceptions with the new concept that they are going to learn.In the second phase, the teaching is conducted by grouping the students into cooperative groups with 4-5 members with different abilities and sexes. In this phase, the teacher facilitates the students at the time of investigating into the mathematical task they are doing, working to understand certain concepts and to acquire skill to solve problems and mathematical skill. In this phase, the teacher facilitates the students to learn in ZPD and makes use of Bruner‟s theory, local culture and provides assistance in the form of scaffolding. Bruner‟s theory (enactive, iconic, and symbolic) serve to facilitate learning at their pace. The students who are still learning in enactive and iconic levels can move on to representative level in the standard mathematical process.If the students get stuck at the time of investigation, the teacher will investigate it, the teacher will facilitate them by providing a help in the form of scaffolding and activating their concept of jengah by using suggestion sentences such as: “n othing is impossible to be done....”; “whatever is be lieved and tried with our best will be accomplished”; “nothing is impossible... as long as you want to strive to get it ...”. Thus in the end, they will be able to have a deep understanding in accordance with their potentials. Scaffolding in refers to the help provided by the more competent peers or adults. [11] states that to provide scaffolding means to provide some support during initial stages of teaching and then minimizing help, giving the chance to the children to assume increasingly greater responsibility after they are able to perform the task by themselves.The presentation and decision making activities in the third phase involve the students more than just reviewing what they have learned. During this phase, the teacher involves the students in activities and discussions that are challenging that can broaden their understanding of concepts and problem solving skill. The students apply what they have learned the students apply what they have learned about mathematical tasks and their experiences to develop, broaden and deepen their conceptual understanding. In this phase, the activation of the concept of jengah is done when the students have been able to communicate, no matter how simple it is done what they have accomplished.Evaluation in the middle in Fig. 6 means that in every phase, there is a need to do an evaluation as part of reflection on the activity in that phase. In every phase, from the beginning to the end, the teacher evaluates the students‟ progress and asks the students to evaluate themselves. The feedback can come from quizzes, discussion with the students, or the use of other techniques. The teacher uses feedback to reflect on the effectiveness of teaching that he or she has done, and to do some corrections during the lesson.. The students use feedback to reflect on what they have understood, what they still need to learn, and what they want to learn next. With such phases of teaching above, one can expect meaningful mathematical learning to happen.5.ConclusionsIn the light of the results and discussion above, it can be concluded that the use of the combination of Bruner‟s theory, local culture (the concept of jengah), and scaffolding in the BLCS teaching model is very effective to use in mathematics teaching.AcknowledgementsThe authors express their gratefulness to entire staff at Department of Mathematics Education and Information Technology Department at Universitas Pendidikan Ganesha, who have given us morale and material supports in this research.References[1]VandeWalle.J.dkk.Elementary and Middle School Mathematics, Teaching A.PearsonEducation, Inc; 2013.[2]Steffe, L.P & D‟Ambrosio Beatrizs. Toward APO Working Model of Constructivist Teaching; APOReaction To Simon. Journal for Research in Mathematics Education. Vol. 26. No. 2, (1995), 146-159. [3]Ardana. Pembelajaran Matematika Berkarakter. Makalah disajikan dalam rangka SemNas oleh FPMIPAIKIP PGRI BALIdi Grand Mutiara Ballroom Hotel Nikki, Denpasar Bali pada Tgl 13/1/2016; 2016. [4]Ardana. Standar Proses Matematika dan Zpd dalam Implementasi Kurikulum 2013. Makalah disajikandalam rangka seminar Regional Bali dengan tema “Pembelajaran Matematika yang Eksploratif dan Inovatif dalam Implementasi Kurikulum 2013” pada Tgl 1/3/2014; 2014.[5]Marpaung. Y. Pengelolaan Proses Belajar Mengejar Matematika. Jakarta. Depdiknas; 2002.[6]Raka Joni. T. Teori-teori Belajar. Jakarta. P2LPTK; 1988.[7]Soedjadi. Kiat Pendidikan Matematika di Indonesia. Jakarta. Dirjen DIKTI, Depdiknas; 2000.[8]Soetarno. Ragam Budaya Indonesia,Direktorat Pembinaan PendidikanTenaga Kependidikan danKetenagaan Perguruan Tinggi - Dirjen Dikti -Depdiknas, Jakarta; 2004.[9]Widja, I.G. Keserasian Transformasi Nilai dan Pembangunan Berwawasan Budaya dalamMasyarakatBali, Perspektif pendidikan. makalah disajikan dalam Seminar Nasional Keserasian Transformasi Nilai dan Pembangunan Berwawasan Budaya. Denpasar: Fakultas Sastra; 1990.[10]Ardhana, I.G.G, Sudharta, R.T. Keserasian Transformasi Nilai dan Pembangunan Berwawasan BudayaDalam Masyarakat Bali.Makalah Disajikan Dalam Seminar Nasional Keserasian Transformasi Nilai dan Pembangunan Berwawasan Budaya. Denpasar: Fakultas Sastra; 1990.[11]Slavin, R.E. Educational Psychology: Theory and Practice. Fourth Edition. Needham Heights: Allynand Bacon Publisher; 1997.[12]Lui, A. Teaching in the Zone: An Introduction to Working Within the Zone of Proximal Development(ZPD) to Drive Effective Early Childhood Instruction. Children‟s Progress; 2012.[13]Novak, J.D & Gown, D.B. Learning How To Learn. New York: Cambridge University Press; 1985.[14]Berg. Euwe Van Den (Edt). Miskonsepsi Fisika dan Remidiasi.Salatiga: Universitas Kristen SatyaWacana; 1991.[15]Tayibnapis, F.Y. Evaluasi Program. Jakarta: PT. Rineka Cipta; 2000.[16]Divayana, D.G.H. “Penggunaan Mod el CSE-UCLA Dalam Mengevaluasi Kualitas Program AplikasiSistem Pakar.” SNATIA 2015, (2015), 165-168.[17]Divayana, D.G.H., & Sugiharni, G.A.D. “Evaluasi Program Sertifikasi Komputer Pada UniversitasTeknologi Indonesia Menggunakan Model CSE-UCLA,”. Jurnal P endidikan Indonesia, Vol.5, No. 2, (2016), 865–872.[18]Divayana, D.G.H. “Development of Duck Diseases Expert System with Applying Alliance Method atBali Provincial Livestock Office,” International Journal of Advanced Computer Science and Applications, Vol. 5, No. 8, (2014), 48-54.[19]Hamdani, “Sistem Pakar Untuk Diagnosa Penyakit Mata Pada Manusia,” Jurnal InformatikaMulawarman, Vol. 5, No. 1, (2010), 13-21.[20]Eviyanti, A. “Aplikasi Sistem Pakar Untuk Mendiagnosis Gangguan Pencernaan Pada Orang Dewasa,”TEKNOLOJIA, Vol. 5, No.1, (2012), 1-10.[21]Sugiharni, G.A.D., & Divayana, D.G.H. “Pemanfaatan Metode Forward Chaining DalamPengembangan Sistem Pakar Pendiagnosa Kerusakan Televisi Berwarna,” Jurnal Nasional Pendidikan Teknik Informatika (JANAPATI), Vol. 6, No.1, (2017), 20-29.Authors’ ProfilesProf. Dr. I Made Ardana, M.Pd. was born in Badung, August 27th 1962. He was receivedDoctor in Mathematics Education from Universitas Negeri Surabaya, Indonesia. He workedas Lecturer of Statistics, Algebra, in Department of Mathematics Education at UniversitasPendidikan Ganesha.。
培养数据能力的重要性英语作文

培养数据能力的重要性英语作文The Importance of Cultivating Data Capabilities in the Modern EraIn the ever-evolving landscape of the 21st century, the significance of data capabilities has grown exponentially, becoming a vital asset for individuals, organizations, and entire economies. As the world continues to digitize and rely more heavily on data-driven decision-making, it is imperative to understand and appreciate the profound importance of cultivating data capabilities.Firstly, let's delve into the fundamental nature of data and its pervasive influence. Data is the backbone of modern society, informing every aspect of our lives, from the mundane tasks of daily routine to the complexstrategies of global enterprises. It is a resource that, when harnessed correctly, can yield insights that transform businesses, streamline operations, and enhance the quality of life for individuals.The importance of cultivating data capabilities begins with its impact on decision-making. In a data-driven world,the ability to collect, analyze, and interpret data is crucial for making informed decisions. Whether it's a business choosing a new market to expand into or a government formulating a policy, data provides an objective and quantifiable basis for decision-making, reducing the risk of bias and enhancing the chances of success.Moreover, data capabilities are essential for innovation and competitiveness. In a globalized economy where competition is fierce, organizations that possess strong data capabilities are able to stay ahead of the curve, identifying new opportunities and developing innovative solutions. They can leverage data to understand consumer trends, optimize product development, and improve customer service, ultimately gaining a competitive edge in their respective industries.However, the benefits of data capabilities are not limited to the corporate world. They are also vital for individuals seeking to enhance their employability and personal development. In today's job market, data literacy and proficiency in data analysis tools are increasingly becoming prerequisites for many positions. By cultivatingthese capabilities, individuals can demonstrate their value and contribute more effectively to their organizations, leading to better career opportunities and personal growth. Moreover, data capabilities also play a crucial role in the field of social sciences and policy-making. They enable researchers to conduct more rigorous and informed studies, leading to more effective policies that address complex societal issues. Whether it's reducing poverty, improving healthcare, or tackling climate change, data capabilities provide the foundation for informed and impactful policy interventions.To cultivate data capabilities effectively, a multi-faceted approach is required. Firstly, education systems must prioritize the teaching of data literacy andanalytical skills, ensuring that students are equipped with the necessary tools and knowledge to navigate the data-driven world. Secondly, organizations should invest in training and development programs that enhance their employees' data capabilities, enabling them to make better use of data in their daily work. Additionally, policies and incentives should be created to encourage the developmentand adoption of data-driven solutions, fostering a culture of innovation and collaboration.It's worth noting that the importance of data capabilities is not just a passing trend. As technology continues to advance and data becomes even more ubiquitous, the need for individuals and organizations to possess strong data capabilities will only increase. Therefore, it is essential to invest in cultivating these capabilities now, ensuring that we are prepared to harness the full potential of data in the future.In conclusion, the importance of cultivating data capabilities cannot be overstated. They are crucial for informed decision-making, competitiveness, personal development, and social progress. By prioritizing the teaching and development of data capabilities, we can ensure that individuals and organizations are equipped to navigate the data-driven world effectively, driving innovation and creating a better future for all.。
模拟ai英文面试题目及答案
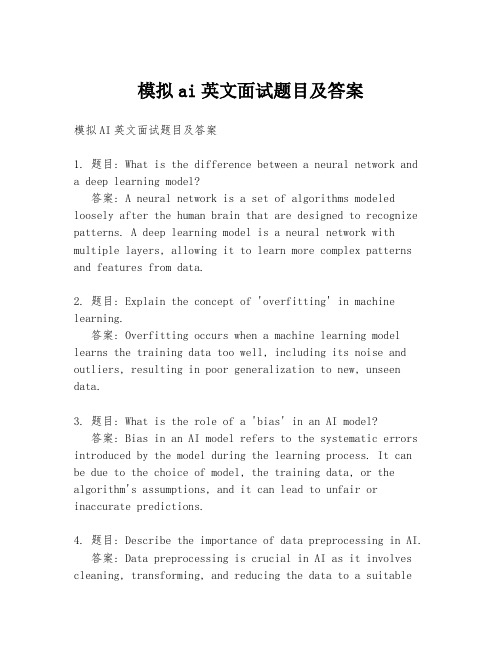
模拟ai英文面试题目及答案模拟AI英文面试题目及答案1. 题目: What is the difference between a neural network anda deep learning model?答案: A neural network is a set of algorithms modeled loosely after the human brain that are designed to recognize patterns. A deep learning model is a neural network with multiple layers, allowing it to learn more complex patterns and features from data.2. 题目: Explain the concept of 'overfitting' in machine learning.答案: Overfitting occurs when a machine learning model learns the training data too well, including its noise and outliers, resulting in poor generalization to new, unseen data.3. 题目: What is the role of a 'bias' in an AI model?答案: Bias in an AI model refers to the systematic errors introduced by the model during the learning process. It can be due to the choice of model, the training data, or the algorithm's assumptions, and it can lead to unfair or inaccurate predictions.4. 题目: Describe the importance of data preprocessing in AI.答案: Data preprocessing is crucial in AI as it involves cleaning, transforming, and reducing the data to a suitableformat for the model to learn effectively. Proper preprocessing can significantly improve the performance of AI models by ensuring that the input data is relevant, accurate, and free from noise.5. 题目: How does reinforcement learning differ from supervised learning?答案: Reinforcement learning is a type of machine learning where an agent learns to make decisions by performing actions in an environment to maximize a reward signal. It differs from supervised learning, where the model learns from labeled data to predict outcomes based on input features.6. 题目: What is the purpose of a 'convolutional neural network' (CNN)?答案: A convolutional neural network (CNN) is a type of deep learning model that is particularly effective for processing data with a grid-like topology, such as images. CNNs use convolutional layers to automatically and adaptively learn spatial hierarchies of features from input images.7. 题目: Explain the concept of 'feature extraction' in AI.答案: Feature extraction in AI is the process of identifying and extracting relevant pieces of information from the raw data. It is a crucial step in many machine learning algorithms, as it helps to reduce the dimensionality of the data and to focus on the most informative aspects that can be used to make predictions or classifications.8. 题目: What is the significance of 'gradient descent' in training AI models?答案: Gradient descent is an optimization algorithm used to minimize a function by iteratively moving in the direction of steepest descent as defined by the negative of the gradient. In the context of AI, it is used to minimize the loss function of a model, thus refining the model's parameters to improve its accuracy.9. 题目: How does 'transfer learning' work in AI?答案: Transfer learning is a technique where a pre-trained model is used as the starting point for learning a new task. It leverages the knowledge gained from one problem to improve performance on a different but related problem, reducing the need for large amounts of labeled data and computational resources.10. 题目: What is the role of 'regularization' in preventing overfitting?答案: Regularization is a technique used to prevent overfitting by adding a penalty term to the loss function, which discourages overly complex models. It helps to control the model's capacity, forcing it to generalize better to new data by not fitting too closely to the training data.。
2023年高考英语2卷阅读第二节
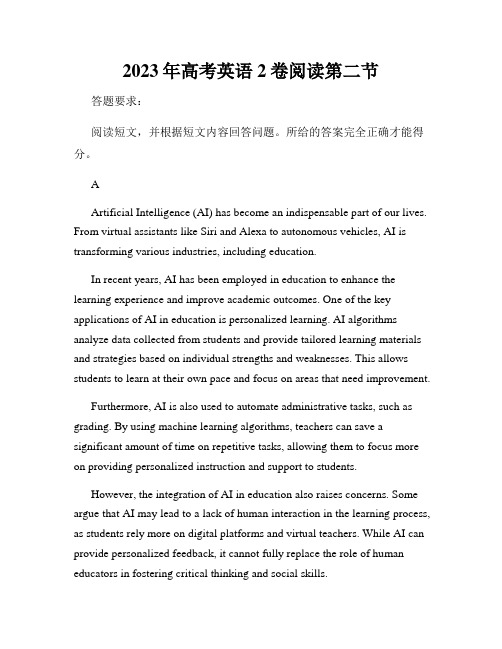
2023年高考英语2卷阅读第二节答题要求:阅读短文,并根据短文内容回答问题。
所给的答案完全正确才能得分。
AArtificial Intelligence (AI) has become an indispensable part of our lives. From virtual assistants like Siri and Alexa to autonomous vehicles, AI is transforming various industries, including education.In recent years, AI has been employed in education to enhance the learning experience and improve academic outcomes. One of the key applications of AI in education is personalized learning. AI algorithms analyze data collected from students and provide tailored learning materials and strategies based on individual strengths and weaknesses. This allows students to learn at their own pace and focus on areas that need improvement.Furthermore, AI is also used to automate administrative tasks, such as grading. By using machine learning algorithms, teachers can save a significant amount of time on repetitive tasks, allowing them to focus more on providing personalized instruction and support to students.However, the integration of AI in education also raises concerns. Some argue that AI may lead to a lack of human interaction in the learning process, as students rely more on digital platforms and virtual teachers. While AI can provide personalized feedback, it cannot fully replace the role of human educators in fostering critical thinking and social skills.Another concern is the potential bias in AI algorithms. AI systems learn from data, and if the data used to train the algorithms is biased, it can perpetuate existing biases in educational systems. For example, if the algorithm is trained on historical data that reflects gender or racial bias, it may unintentionally reinforce those biases in the personalized learning recommendations it provides.In conclusion, AI has great potential in revolutionizing education by providing personalized learning experiences and automating administrative tasks. However, it is important to strike a balance between the benefits of AI and the preservation of human interaction and critical thinking skills in education. Additionally, efforts should be made to ensure the fairness and ethical use of AI algorithms in educational settings.B1. What is one of the key applications of AI in education?Personalized learning is one of the key applications of AI in education. AI algorithms analyze data collected from students and provide tailored learning materials and strategies based on individual strengths and weaknesses.2. How does AI help save time for teachers?AI helps save time for teachers by automating administrative tasks, such as grading. By using machine learning algorithms, teachers can focus more on providing personalized instruction and support to students.3. What concerns are raised regarding the integration of AI in education?Two concerns raised regarding the integration of AI in education are the lack of human interaction in the learning process and the potential bias in AI algorithms.4. How can the potential bias in AI algorithms be addressed?Efforts should be made to ensure the fairness and ethical use of AI algorithms in educational settings. This includes carefully selecting and monitoring the data used to train the algorithms to avoid perpetuating existing biases.5. What is the importance of striking a balance between AI and human interaction in education?While AI can provide personalized feedback, it cannot fully replace the role of human educators in fostering critical thinking and social skills. Striking a balance ensures that students benefit from both the advantages of AI and the essential human aspect of education.。
仁爱英语九年级上册单词表含音标

仁爱英语九年级上册单词表Unit1第1单元Topic1Ourcountryhasdevelopedrapidly. 话题1我们的国家飞速发展。
proper['pr?p?]['prɑp?]adj.恰当的,合适的;真正的bytheway顺便说volunteer[v?l?n'ti?][vɑl?n't?r]n.义务工作者;志愿者belln.钟(铃)声;铃,钟;钟状物grandpa['gr?ndpɑ:]n.爷爷;外公chairwoman['t???wum?n]n.女主席,女会长;女议长grandson['gr?nds?n]n.(外)孙子disabled[dis'eibld]adj.残疾的,残废的shut[??t]v.关上,封闭;禁闭;合拢rope[r?up]n.绳子,绳索teenager['ti:neid??]n.(13~19岁的)青少年,十几岁的少年granny['gr?ni]n.祖母,外婆;老奶奶describe[di'skraib]v.描写,叙述indetail['di:teil]详细地education[,edjukei?n]n.教育;培养childhood['t?aild,hud]n.童年,幼年时代support[s?'p?:t]v.&n.供养,抚养;支持,赞助laborer['leib?r?]n.(尤指户外的)体力劳动者,劳工,工人develop[di'vel?p]v.(使)发展;(使)发达;开发rapidly['r?pidli]adv.快地,迅速地luckily['l?kili]adv.幸运的development[di'vel?pm?nt]n.发展;发达;开发narrow['n?r?u]adj.狭窄的communication[k?,mju:ni'kei??n]n.交流;交往;通讯quick[kwik]adj.快的;敏捷的;急剧的;adv.快地;敏捷地;急剧地leisure['li:??]n.空闲,闲暇,业余时间keepintouchwith跟……保持联系relative['rel?tiv]n.亲属,亲戚faraway遥远的mainly['meinli]adv.主要地,总体上,大致telegram['teligr?m]n.电报;电文reformandopening-up改革开放sort[s?:t]n.种类,类别;v.把……分类;拣选fax[f?ks]n.传真;传真机rapid['r?pid]adj.快的,迅速的progress['pr?ugres]n.进步;进展;v.进展;逐步发展makeprogress取得进展;取得进步already[?:l'redi]adv.已经succeed[s?k'si:d]v.成功organization[,?:g?nai'zei??n]n.组织,机构war[w?:]n.战争tugofwarn.拔河note[n?ut]n.便条;笔记;注释;钞票,纸币;v.记下,记录;注意,留意composition[,k?mp?'zi??n]n.作文;作曲consider[k?n'sid?]v.考虑drawup拟定,起草tool[tu:l]n.工具,器具thanksto幸亏,由于Unit1第1单元Topic2Chinahasthelargestpoipulation. 话题2中国拥有最庞大的人口。
学习应用的重要性英语作文
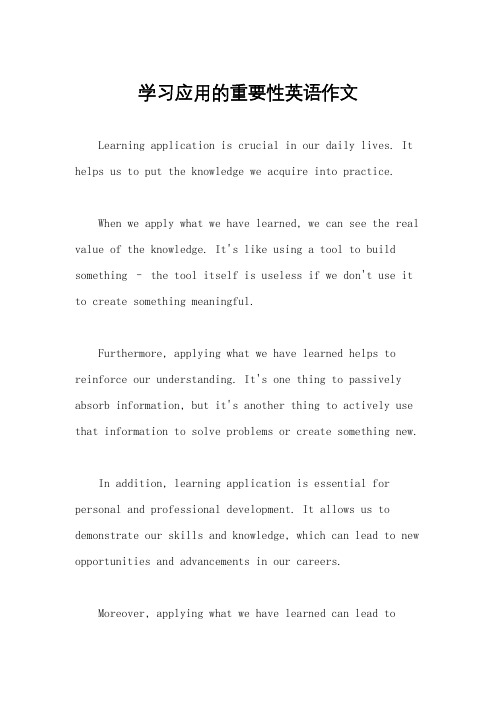
学习应用的重要性英语作文Learning application is crucial in our daily lives. It helps us to put the knowledge we acquire into practice.When we apply what we have learned, we can see the real value of the knowledge. It's like using a tool to build something – the tool itself is useless if we don't use it to create something meaningful.Furthermore, applying what we have learned helps to reinforce our understanding. It's one thing to passively absorb information, but it's another thing to actively use that information to solve problems or create something new.In addition, learning application is essential for personal and professional development. It allows us to demonstrate our skills and knowledge, which can lead to new opportunities and advancements in our careers.Moreover, applying what we have learned can lead toinnovation and progress. When we take what we know and apply it in new and creative ways, we can make breakthroughs and advancements in various fields.In conclusion, learning application is vital for turning knowledge into action, reinforcing understanding, personal and professional development, and fostering innovation and progress. It's not enough to just learn –we must also apply what we have learned in order to truly benefit from it.。
人工智能专业重要词汇表
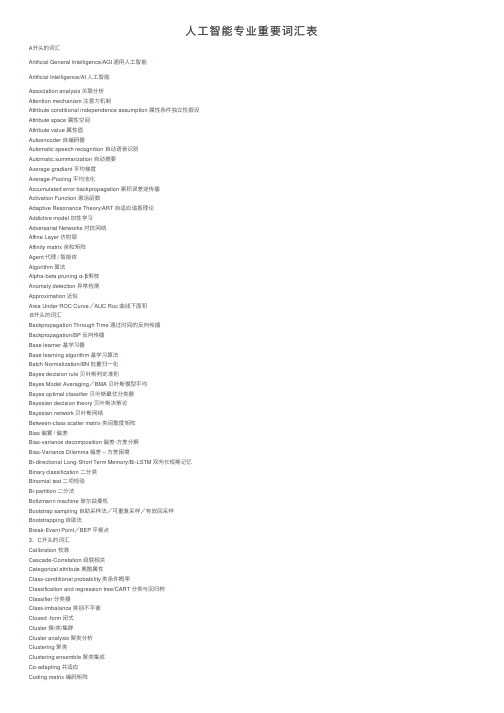
⼈⼯智能专业重要词汇表A开头的词汇Artificial General Intelligence/AGI 通⽤⼈⼯智能Artificial Intelligence/AI ⼈⼯智能Association analysis 关联分析Attention mechanism 注意⼒机制Attribute conditional independence assumption 属性条件独⽴性假设Attribute space 属性空间Attribute value 属性值Autoencoder ⾃编码器Automatic speech recognition ⾃动语⾳识别Automatic summarization ⾃动摘要Average gradient 平均梯度Average-Pooling 平均池化Accumulated error backpropagation 累积误差逆传播Activation Function 激活函数Adaptive Resonance Theory/ART ⾃适应谐振理论Addictive model 加性学习Adversarial Networks 对抗⽹络Affine Layer 仿射层Affinity matrix 亲和矩阵Agent 代理 / 智能体Algorithm 算法Alpha-beta pruning α-β剪枝Anomaly detection 异常检测Approximation 近似Area Under ROC Curve/AUC Roc 曲线下⾯积B开头的词汇Backpropagation Through Time 通过时间的反向传播Backpropagation/BP 反向传播Base learner 基学习器Base learning algorithm 基学习算法Batch Normalization/BN 批量归⼀化Bayes decision rule 贝叶斯判定准则Bayes Model Averaging/BMA 贝叶斯模型平均Bayes optimal classifier 贝叶斯最优分类器Bayesian decision theory 贝叶斯决策论Bayesian network 贝叶斯⽹络Between-class scatter matrix 类间散度矩阵Bias 偏置 / 偏差Bias-variance decomposition 偏差-⽅差分解Bias-Variance Dilemma 偏差 – ⽅差困境Bi-directional Long-Short Term Memory/Bi-LSTM 双向长短期记忆Binary classification ⼆分类Binomial test ⼆项检验Bi-partition ⼆分法Boltzmann machine 玻尔兹曼机Bootstrap sampling ⾃助采样法/可重复采样/有放回采样Bootstrapping ⾃助法Break-Event Point/BEP 平衡点3、C开头的词汇Calibration 校准Cascade-Correlation 级联相关Categorical attribute 离散属性Class-conditional probability 类条件概率Classification and regression tree/CART 分类与回归树Classifier 分类器Class-imbalance 类别不平衡Closed -form 闭式Cluster 簇/类/集群Cluster analysis 聚类分析Clustering 聚类Clustering ensemble 聚类集成Co-adapting 共适应Coding matrix 编码矩阵COLT 国际学习理论会议Committee-based learning 基于委员会的学习Competitive learning 竞争型学习Component learner 组件学习器Comprehensibility 可解释性Computation Cost 计算成本Computational Linguistics 计算语⾔学Computer vision 计算机视觉Concept drift 概念漂移Concept Learning System /CLS 概念学习系统Conditional entropy 条件熵Conditional mutual information 条件互信息Conditional Probability Table/CPT 条件概率表Conditional random field/CRF 条件随机场Conditional risk 条件风险Confidence 置信度Confusion matrix 混淆矩阵Connection weight 连接权Connectionism 连结主义Consistency ⼀致性/相合性Contingency table 列联表Continuous attribute 连续属性Convergence 收敛Conversational agent 会话智能体Convex quadratic programming 凸⼆次规划Convexity 凸性Convolutional neural network/CNN 卷积神经⽹络Co-occurrence 同现Correlation coefficient 相关系数Cosine similarity 余弦相似度Cost curve 成本曲线Cost Function 成本函数Cost matrix 成本矩阵Cost-sensitive 成本敏感Cross entropy 交叉熵Cross validation 交叉验证Crowdsourcing 众包Curse of dimensionality 维数灾难Cut point 截断点Cutting plane algorithm 割平⾯法4、D开头的词汇Data mining 数据挖掘Data set 数据集Decision Boundary 决策边界Decision stump 决策树桩Decision tree 决策树/判定树Deduction 演绎Deep Belief Network 深度信念⽹络Deep Convolutional Generative Adversarial Network/DCGAN 深度卷积⽣成对抗⽹络Deep learning 深度学习Deep neural network/DNN 深度神经⽹络Deep Q-Learning 深度 Q 学习Deep Q-Network 深度 Q ⽹络Density estimation 密度估计Density-based clustering 密度聚类Differentiable neural computer 可微分神经计算机Dimensionality reduction algorithm 降维算法Directed edge 有向边Disagreement measure 不合度量Discriminative model 判别模型Discriminator 判别器Distance measure 距离度量Distance metric learning 距离度量学习Distribution 分布Divergence 散度Diversity measure 多样性度量/差异性度量Domain adaption 领域⾃适应Downsampling 下采样D-separation (Directed separation)有向分离Dual problem 对偶问题Dummy node 哑结点Dynamic Fusion 动态融合Dynamic programming 动态规划5、E开头的词汇Eigenvalue decomposition 特征值分解Embedding 嵌⼊Emotional analysis 情绪分析Empirical conditional entropy 经验条件熵Empirical entropy 经验熵Empirical error 经验误差Empirical risk 经验风险End-to-End 端到端Energy-based model 基于能量的模型Ensemble learning 集成学习Ensemble pruning 集成修剪Error Correcting Output Codes/ECOC 纠错输出码Error rate 错误率Error-ambiguity decomposition 误差-分歧分解Euclidean distance 欧⽒距离Evolutionary computation 演化计算Expectation-Maximization 期望最⼤化Expected loss 期望损失Exploding Gradient Problem 梯度爆炸问题Exponential loss function 指数损失函数Extreme Learning Machine/ELM 超限学习机6、F开头的词汇Factorization 因⼦分解False negative 假负类False positive 假正类False Positive Rate/FPR 假正例率Feature engineering 特征⼯程Feature selection 特征选择Feature vector 特征向量Featured Learning 特征学习Feedforward Neural Networks/FNN 前馈神经⽹络Fine-tuning 微调Flipping output 翻转法Fluctuation 震荡Forward stagewise algorithm 前向分步算法Frequentist 频率主义学派Full-rank matrix 满秩矩阵Functional neuron 功能神经元7、G开头的词汇Gain ratio 增益率Game theory 博弈论Gaussian kernel function ⾼斯核函数Gaussian Mixture Model ⾼斯混合模型General Problem Solving 通⽤问题求解Generalization 泛化Generalization error 泛化误差Generalization error bound 泛化误差上界Generalized Lagrange function ⼴义拉格朗⽇函数Generalized linear model ⼴义线性模型Generalized Rayleigh quotient ⼴义瑞利商Generative Adversarial Networks/GAN ⽣成对抗⽹络Generative Model ⽣成模型Generator ⽣成器Genetic Algorithm/GA 遗传算法Gibbs sampling 吉布斯采样Gini index 基尼指数Global minimum 全局最⼩Global Optimization 全局优化Gradient boosting 梯度提升Gradient Descent 梯度下降Graph theory 图论Ground-truth 真相/真实8、H开头的词汇Hard margin 硬间隔Hard voting 硬投票Harmonic mean 调和平均Hesse matrix 海塞矩阵Hidden dynamic model 隐动态模型Hidden layer 隐藏层Hidden Markov Model/HMM 隐马尔可夫模型Hierarchical clustering 层次聚类Hilbert space 希尔伯特空间Hinge loss function 合页损失函数Hold-out 留出法Homogeneous 同质Hybrid computing 混合计算Hyperparameter 超参数Hypothesis 假设Hypothesis test 假设验证9、I开头的词汇ICML 国际机器学习会议Improved iterative scaling/IIS 改进的迭代尺度法Incremental learning 增量学习Independent and identically distributed/i.i.d. 独⽴同分布Independent Component Analysis/ICA 独⽴成分分析Indicator function 指⽰函数Individual learner 个体学习器Induction 归纳Inductive bias 归纳偏好Inductive learning 归纳学习Inductive Logic Programming/ILP 归纳逻辑程序设计Information entropy 信息熵Information gain 信息增益Input layer 输⼊层Insensitive loss 不敏感损失Inter-cluster similarity 簇间相似度International Conference for Machine Learning/ICML 国际机器学习⼤会Intra-cluster similarity 簇内相似度Intrinsic value 固有值Isometric Mapping/Isomap 等度量映射Isotonic regression 等分回归Iterative Dichotomiser 迭代⼆分器10、K开头的词汇Kernel method 核⽅法Kernel trick 核技巧Kernelized Linear Discriminant Analysis/KLDA 核线性判别分析K-fold cross validation k 折交叉验证/k 倍交叉验证K-Means Clustering K – 均值聚类K-Nearest Neighbours Algorithm/KNN K近邻算法Knowledge base 知识库Knowledge Representation 知识表征11、L开头的词汇Label space 标记空间Lagrange duality 拉格朗⽇对偶性Lagrange multiplier 拉格朗⽇乘⼦Laplace smoothing 拉普拉斯平滑Laplacian correction 拉普拉斯修正Latent Dirichlet Allocation 隐狄利克雷分布Latent semantic analysis 潜在语义分析Latent variable 隐变量Lazy learning 懒惰学习Learner 学习器Learning by analogy 类⽐学习Learning rate 学习率Learning Vector Quantization/LVQ 学习向量量化Least squares regression tree 最⼩⼆乘回归树Leave-One-Out/LOO 留⼀法linear chain conditional random field 线性链条件随机场Linear Discriminant Analysis/LDA 线性判别分析Linear model 线性模型Linear Regression 线性回归Link function 联系函数Local Markov property 局部马尔可夫性Local minimum 局部最⼩Log likelihood 对数似然Log odds/logit 对数⼏率Logistic Regression Logistic 回归Log-likelihood 对数似然Log-linear regression 对数线性回归Long-Short Term Memory/LSTM 长短期记忆Loss function 损失函数12、M开头的词汇Machine translation/MT 机器翻译Macron-P 宏查准率Macron-R 宏查全率Majority voting 绝对多数投票法Manifold assumption 流形假设Manifold learning 流形学习Margin theory 间隔理论Marginal distribution 边际分布Marginal independence 边际独⽴性Marginalization 边际化Markov Chain Monte Carlo/MCMC 马尔可夫链蒙特卡罗⽅法Markov Random Field 马尔可夫随机场Maximal clique 最⼤团Maximum Likelihood Estimation/MLE 极⼤似然估计/极⼤似然法Maximum margin 最⼤间隔Maximum weighted spanning tree 最⼤带权⽣成树Max-Pooling 最⼤池化Mean squared error 均⽅误差Meta-learner 元学习器Metric learning 度量学习Micro-P 微查准率Micro-R 微查全率Minimal Description Length/MDL 最⼩描述长度Minimax game 极⼩极⼤博弈Misclassification cost 误分类成本Mixture of experts 混合专家Momentum 动量Moral graph 道德图/端正图Multi-class classification 多分类Multi-document summarization 多⽂档摘要Multi-layer feedforward neural networks 多层前馈神经⽹络Multilayer Perceptron/MLP 多层感知器Multimodal learning 多模态学习Multiple Dimensional Scaling 多维缩放Multiple linear regression 多元线性回归Multi-response Linear Regression /MLR 多响应线性回归Mutual information 互信息13、N开头的词汇Naive bayes 朴素贝叶斯Naive Bayes Classifier 朴素贝叶斯分类器Named entity recognition 命名实体识别Nash equilibrium 纳什均衡Natural language generation/NLG ⾃然语⾔⽣成Natural language processing ⾃然语⾔处理Negative class 负类Negative correlation 负相关法Negative Log Likelihood 负对数似然Neighbourhood Component Analysis/NCA 近邻成分分析Neural Machine Translation 神经机器翻译Neural Turing Machine 神经图灵机Newton method ⽜顿法NIPS 国际神经信息处理系统会议No Free Lunch Theorem/NFL 没有免费的午餐定理Noise-contrastive estimation 噪⾳对⽐估计Nominal attribute 列名属性Non-convex optimization ⾮凸优化Nonlinear model ⾮线性模型Non-metric distance ⾮度量距离Non-negative matrix factorization ⾮负矩阵分解Non-ordinal attribute ⽆序属性Non-Saturating Game ⾮饱和博弈Norm 范数Normalization 归⼀化Nuclear norm 核范数Numerical attribute 数值属性14、O开头的词汇Objective function ⽬标函数Oblique decision tree 斜决策树Occam’s razor 奥卡姆剃⼑Odds ⼏率Off-Policy 离策略One shot learning ⼀次性学习One-Dependent Estimator/ODE 独依赖估计On-Policy 在策略Ordinal attribute 有序属性Out-of-bag estimate 包外估计Output layer 输出层Output smearing 输出调制法Overfitting 过拟合/过配Oversampling 过采样15、P开头的词汇Paired t-test 成对 t 检验Pairwise 成对型Pairwise Markov property 成对马尔可夫性Parameter 参数Parameter estimation 参数估计Parameter tuning 调参Parse tree 解析树Particle Swarm Optimization/PSO 粒⼦群优化算法Part-of-speech tagging 词性标注Perceptron 感知机Performance measure 性能度量Plug and Play Generative Network 即插即⽤⽣成⽹络Plurality voting 相对多数投票法Polarity detection 极性检测Polynomial kernel function 多项式核函数Pooling 池化Positive class 正类Positive definite matrix 正定矩阵Post-hoc test 后续检验Post-pruning 后剪枝potential function 势函数Precision 查准率/准确率Prepruning 预剪枝Principal component analysis/PCA 主成分分析Principle of multiple explanations 多释原则Prior 先验Probability Graphical Model 概率图模型Proximal Gradient Descent/PGD 近端梯度下降Pruning 剪枝Pseudo-label 伪标记16、Q开头的词汇Quantized Neural Network 量⼦化神经⽹络Quantum computer 量⼦计算机Quantum Computing 量⼦计算Quasi Newton method 拟⽜顿法17、R开头的词汇Radial Basis Function/RBF 径向基函数Random Forest Algorithm 随机森林算法Random walk 随机漫步Recall 查全率/召回率Receiver Operating Characteristic/ROC 受试者⼯作特征Rectified Linear Unit/ReLU 线性修正单元Recurrent Neural Network 循环神经⽹络Recursive neural network 递归神经⽹络Reference model 参考模型Regression 回归Regularization 正则化Reinforcement learning/RL 强化学习Representation learning 表征学习Representer theorem 表⽰定理reproducing kernel Hilbert space/RKHS 再⽣核希尔伯特空间Re-sampling 重采样法Rescaling 再缩放Residual Mapping 残差映射Residual Network 残差⽹络Restricted Boltzmann Machine/RBM 受限玻尔兹曼机Restricted Isometry Property/RIP 限定等距性Re-weighting 重赋权法Robustness 稳健性/鲁棒性Root node 根结点Rule Engine 规则引擎Rule learning 规则学习18、S开头的词汇Saddle point 鞍点Sample space 样本空间Sampling 采样Score function 评分函数Self-Driving ⾃动驾驶Self-Organizing Map/SOM ⾃组织映射Semi-naive Bayes classifiers 半朴素贝叶斯分类器Semi-Supervised Learning 半监督学习semi-Supervised Support Vector Machine 半监督⽀持向量机Sentiment analysis 情感分析Separating hyperplane 分离超平⾯Sigmoid function Sigmoid 函数Similarity measure 相似度度量Simulated annealing 模拟退⽕Simultaneous localization and mapping 同步定位与地图构建Singular Value Decomposition 奇异值分解Slack variables 松弛变量Smoothing 平滑Soft margin 软间隔Soft margin maximization 软间隔最⼤化Soft voting 软投票Sparse representation 稀疏表征Sparsity 稀疏性Specialization 特化Spectral Clustering 谱聚类Speech Recognition 语⾳识别Splitting variable 切分变量Squashing function 挤压函数Stability-plasticity dilemma 可塑性-稳定性困境Statistical learning 统计学习Status feature function 状态特征函Stochastic gradient descent 随机梯度下降Stratified sampling 分层采样Structural risk 结构风险Structural risk minimization/SRM 结构风险最⼩化Subspace ⼦空间Supervised learning 监督学习/有导师学习support vector expansion ⽀持向量展式Support Vector Machine/SVM ⽀持向量机Surrogat loss 替代损失Surrogate function 替代函数Symbolic learning 符号学习Symbolism 符号主义Synset 同义词集19、T开头的词汇T-Distribution Stochastic Neighbour Embedding/t-SNE T – 分布随机近邻嵌⼊Tensor 张量Tensor Processing Units/TPU 张量处理单元The least square method 最⼩⼆乘法Threshold 阈值Threshold logic unit 阈值逻辑单元Threshold-moving 阈值移动Time Step 时间步骤Tokenization 标记化Training error 训练误差Training instance 训练⽰例/训练例Transductive learning 直推学习Transfer learning 迁移学习Treebank 树库Tria-by-error 试错法True negative 真负类True positive 真正类True Positive Rate/TPR 真正例率Turing Machine 图灵机Twice-learning ⼆次学习20、U开头的词汇Underfitting ⽋拟合/⽋配Undersampling ⽋采样Understandability 可理解性Unequal cost ⾮均等代价Unit-step function 单位阶跃函数Univariate decision tree 单变量决策树Unsupervised learning ⽆监督学习/⽆导师学习Unsupervised layer-wise training ⽆监督逐层训练Upsampling 上采样21、V开头的词汇Vanishing Gradient Problem 梯度消失问题Variational inference 变分推断VC Theory VC维理论Version space 版本空间Viterbi algorithm 维特⽐算法Von Neumann architecture 冯 · 诺伊曼架构22、W开头的词汇Wasserstein GAN/WGAN Wasserstein⽣成对抗⽹络Weak learner 弱学习器Weight 权重Weight sharing 权共享Weighted voting 加权投票法Within-class scatter matrix 类内散度矩阵Word embedding 词嵌⼊Word sense disambiguation 词义消歧23、Z开头的词汇Zero-data learning 零数据学习Zero-shot learning 零次学习。
中考英语外研版 提升作业教材系统复习 八年级(下)书面表达专练

At homAe,twheosmhouel,dwlievesihnopuealcde lwiviteh oinurppeaarecnetsw. Witehshoouurldptaalkreanbotsu.t oWur edrsehamous lwditthatlhkemabaonduttaolkurabdourteaoumr strwouibtlhestihnetmimea. nLdet tpaalrkents kanbowouevteoryuthrintgroaubobulteuss.inWittihmthee.irLseuptppoartr, ewnetwsilkl ngeotwtwice the result weivtherhayltfhthine geffaorbto. Autttuhes.saWmeitthimteh, weiershsouupldpaolrsot,hwelpeowurilplagreentts do stowmeicheoutsheeworreksuanldt wleairtnhshomaleflitfhe eskeilflsf.oIrf ty.oAu atrtehine asabmademtoiomd,ey,ou cwanelisthenoutoldsomalesmouhsieclopr odousrompaetrheinngtysodu olikseome housework . and learn some life skills. If you are in a bad mood, you can listen to some music or do something you like.
We alWl haevealdlrehaamvjeobdsr. Aeas mfor jmoeb, sI .dAresamfotorbme ae,scIiedntriesta. Imhatvoe ableways
高中英语词汇教学的有效方法
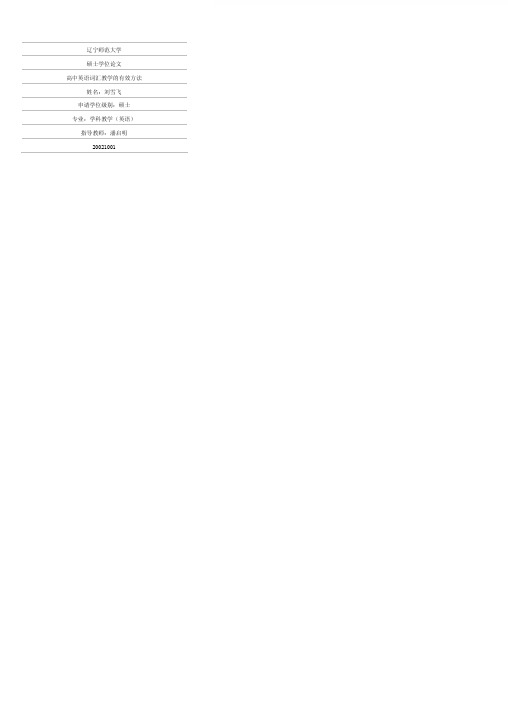
辽宁师范大学硕士学位论文高中英语词汇教学的有效方法姓名:***申请学位级别:硕士专业:学科教学(英语)指导教师:***20021001高中英语词汇教学的有效方法刘雪飞摘要:厂/过三关(语音关。
词汇关,语法关),靳五将(昕,说,读,L一写,译)。
对于外语学习者来说部是非常重要的。
相对来说,掌握英语单词是最难过的一关。
众所周知,语言离不开词汇,外语教学离不开词汇教学,不掌握一定董的词汇,断,说,读,写,译,都无法顺利进行,正如语言学家威尔金斯在《语言学与语言教学》中所说的:“我们不能接受语法比词汇更重要的说法。
事实是没有语法就只能传递有限的信息。
然而,如果没有词汇。
则\、什么也传达不了了。
”2~,,H’,pv目前,随着英语在全世界的广泛应用,中国入世,英语学习已经成为我们日常学习、工作、生活的一部分了。
英语学习不再是为了满足考试的需要,而是进行交流、发展经济、了解落界的必要工具。
只有掌握一定的词汇量,才能使这些活动有实现的可能。
越来越多的学生似乎认为,既然英语教学中阅读理解占的比例出现上升的趋势,没有必要在词忙上花时间。
不少老师也持这一观点。
在教学实践中,花在词汇教学上的时间很少。
印使在教授词汇中,也显的缺乏足够的理论和令人信服的有效的词汇教学方法。
这种现象令人担忧。
随着阅读理解题的增加。
词汇在夕卜语教学中的地位只能交终更重要。
如何教授词汇就显得非常重要。
本文结合高中教材及教学实践,研究如何使用合理的词汇教学方法,及教学策略,对学生进行必要的学习指导,进而使学生找到适合自己的有效学习策略,提高词汇的记忆效率。
培养学生终身学习能力。
而不是仅仅是死记硬背。
我们在大连十一中的词汇教学中运用和总结出许多行之有效的教学方法,如关键词记忆法教学,借助偶然词’汇学习,遇过大萋阙读,增加语言输入,扩大词汇量。
本文从教学实际出发,紧密结合教材,抓住英语单词的特点,利用圊义词。
反义词,一词多义,同音异义词,同形异义词,同形异音词,同形同音异义词以及一些固定搭配,开展词汇教学。
- 1、下载文档前请自行甄别文档内容的完整性,平台不提供额外的编辑、内容补充、找答案等附加服务。
- 2、"仅部分预览"的文档,不可在线预览部分如存在完整性等问题,可反馈申请退款(可完整预览的文档不适用该条件!)。
- 3、如文档侵犯您的权益,请联系客服反馈,我们会尽快为您处理(人工客服工作时间:9:00-18:30)。
Policy Learning by GA using ImportanceSamplingChikao Tsuchiya,Hajime Kimura,Shigenobu KobayashiTokyo Institute of Technology,4259Nagatsuta,Midori,Yokohama,Kanagawa Japan Abstract.The most difficult problem of applying GA to a policy learning is that in-teractions with the environment require much time to evaluate the individuals.In thispaper,we propose a new approach to estimate the individual’s value using importancesampling.Importance sampling reuses the experiences obtained by some policy to es-timate values of the other policies.The proposed technique cuts down the interactionswith the environment in evaluating children,it can speed up optimization.In particular,it is effective in case GA is applied to a real robot’s policy learning,because the load tothe hardware accompanying trial and error can be mitigated.The proposed techniquewas implemented to the crawling robot,it was applied to obtain the control rules sothat the robot is to walk.The experimental results show the strong affinity betweenGA and importance sampling,and also mean that GA using importance sampling canbe a powerful tool for policy learning.1IntroductionReinforcement learning(RL)learns the policy to maximize the average reward through inter-actions with the environment.That is,the problem is to search for mapping from the state space to the action space.However,because RL is basically a local search,it may lapse into a local minimum when applied to multimodal optimization problems.On the other hand,GA isa global search and thus it can deal with them.However,in the policy learning which requires interactions with the environment,it has been a serious obstacle in practical use that many trial and error are called for.In recent years,the usefulness of importance sampling that reuses the data of the state transition series obtained by a certain policy for learning another policy,attracts attention[9][6][7]. In the domain of RL,several researchers use importance sampling to estimate Q-values for MDP.On the other hand,in the domain of GA,there is still little research on policy learning, and there is almost no research which sets the focus to importance sampling.In this paper,in order to accelerate policy learning by GA,we introduce importance sam-pling.The policy learning by the naive GA requires many interactions with the environmentto evaluate the children generated by crossover or mutation,and this is a practical obstacle. We evaluate the children by reusing the population’s experiences using importance sampling.It is expected that our technique considerably cuts down the number of interactions with the environment.In the proposed method,the agent can use plural policies held in the population,acts in the environment according to those policies,and accumulates new experiences.After some pe-riod,many children are generated by crossover between the parents chosen randomly from thepopulation.Processing the accumulated experiences using importance sampling,the rewards obtained by population are transformed into the children’s rewards.Then let those rewards be their values.Because the children’s policies aren’t performed in the environment,the time to evaluate children turns into only processing time of importance sampling.Therefore,the op-timization process is accelerated.We applied the proposed technique to the crawling robot to obtain control rules so that the robot is to walk.As the result,the usefulness of the proposed technique is verified.2Problem Formulation2.1Target ProblemThe target problem is a reinforcement learning task in a Markov decision process(MDP). Even if it is partial observable Markov decision process(POMDP),the proposed technique can be applied.MDP is shown in the following.Let S denote state space,A be action space,R be a set of real number.At each discrete time t,the agent observes state s t∈S,selects action a t∈A,and then receives an instantaneous reward r t∈R resulting from state transition in theenvironment.In general,the reward and the next state may be random,but their probability distributions are assumed to depend only on s t and a t in MDP.In MDP,the next state s t+1 is chosen according to the transition probability Pr(s t+1|s t,a t),and the reward r t is given randomly according to the expectation r(s t,a).The learning agent does not know Pr(s t+1|s t,a t)and r(s t,a)ahead of time.The objective of RL is to construct a policy that maximizes the agent’s performance.A natural performance measure for a given task is the average reward per episode:V=1MMi=1r i2.2Policy Learning by Real Coded GAWe use UNDX[5]which is the well-known crossover operator in the real coded GAs,and MGG[8]which is one of the generation-alternation models excellent in diversity mainte-nance.The policy learning by the naive GA usually acquires the values of generated policies. Figure1shows the framework of policy learning by the naive GA.Although interactions with the environment are required for evaluating the generated chil-dren,the efficiency of learning is improvable if the experiences of population are reusable. 3Estimation of Policy’s Value using Importance Sampling3.1Estimation using Importance SamplingWe assume that the policies can be parameterized by a vectorθ.If we know the similarity between some policyθand anothor policyθ ,the value of policyθ can be calculated by applying some corrections proportional to it.In the policy parameterized byθ,letπ(s,a;θ)be the probability an agent selects the action a in the state s.Generation ofChildren byFigure 1:The framework of the policy learning by the naive GA.The probability that an episode h ={s 1,a 1,r 1,s 2,a 2,r 2,...,s M ,a M ,r M ,s M +1}occurs is described as follows:Pr(h |θ)=Pr(s 1)Φ(h )Ψ(h )=Pr(s 1)Mi =1π(s i ,a i ;θ)Pr(s i +1|s i ,a i )where let Φ(h )be the action selection probability,let Ψ(h )be the state transition probability,let Pr(s 1)be the probability the initial state is s 1.Using importance sampling,the value of the policy parameterized by θ can be estimated as follows[6]:ˆV (θ)=1N N i =1R i Pr(h i |θ )Pr(h i |θ)=1N N i =1R i Pr(s 1)Φ (h i )Ψ(h )Pr(s 1)Φ(h i )Ψ(h )=1N N i =1R i Φ (h i )Φ(h i )=1N N i =1R i M j =1π(s j ,a j |θ )π(s j ,a j |θ)where let R i be the sum of rewards on the episode i ,let N be the number of episodes.This formula means that the values can be calculated using only the ratio of the action selection probability in each policy.Moreover,Precup et al.[6]show the weighted importance sampling.It has a lower vari-ance estimate at cost of adding bias.It is described as follows:ˆV (θ )=1 N i =1Φ (h i )Φ(h i )N i =1R i Φ (h i )Φ(h i )=1 N i =1 M j =1π(s j ,a j |θ )π(s j ,a j |θ)N i =1R i M j =1π(s j ,a j |θ )π(s j ,a j |θ)3.2Estimate the Value of ChildrenImportance sampling described in the previous section estimates the values of the other poli-cies using the experience obtained by one policy.GA generates many children from two or more parents.Shelton et al.[9]proposed weighted importance sampling using the experiences obtained by policies θ1,θ2,...,θN .According to it,the value can be estimated as follows:ˆV (θ )=1 N i =1Pr(h i |θ )N j =1Pr(h i |θj ) Ni =1R i Pr(h i |θ ) N j =1Pr(h i |θj )=1 N i =1M k =1π(s k ,a k |θ )N j =1M k =1π(s k ,a k |θj ) N i =1R i M k =1π(s k ,a k |θ ) N j =1 M k =1π(s k ,a k |θj )Using this technique,the children’s values can be estimated from experiences and policies held within the population.3.3Algorithm of Policy Learning using Importance SamplingThe algorithm of the proposed technique is described as follows:1.Generate N policies as an initial population,and obtain the experiences by these policies.2.Choose 2+1parents from N policies by random sampling,and generate C children by UNDX.3.Estimate all children’s values using importance sampling.4.Choose two individuals from the family containing the parents and their children:one is the best individual and the other is selected from C +1individuals other than the best one by the rank-based roulette wheel selection[1].Replace the two parents with those two children.And,do away with the experiences obtained by the parents’policies.5.Obtain the experiences by interactions with the environment using the policies of two newly added individuals.And,repeat the above procedures from step 2.Figure 2shows the framework of the policy learning by the proposed technique.Generation ofChildren bySelection forFigure2:The framework of the policy learning by the proposed technique.4Application to the Crawling Robots4.1Crawling RobotsIn this paper,we use the crawling robot shown in Figure3.The crawling robot has one arm controlled by two servo motors and the touch sensor which investigates whether the tip of the arm is touching the ground or not.We aim at obtaining control rules so that the robot is to walk through trial and error.How-ever it is difficult to execute sufficient experiments using real robots for comparing several algorithms.We consider an imaginary crawling robot shown in Figure4,and we evaluate the proposed technique from the experiments.The robot has bounded continuous and discrete state variables.Continuous state variables are angular-position of the two joints,and discrete state variable represents for a touch sen-sor for the arm.The agent observes these state variables.The action the agent selects is an objective angular-position of two joint-motors.That is the same dimension of the continuous state.When the agent selects an action,the robot moves the motors towards the commanded positions.When the joint-angles move to the commanded position or the touch sensor’s state changes,the reward is given as the result of the transition,and time step proceeds to the next step.The crawling robot’s action stops when the motors reach to the objective angular-position or the touch sensor’s state changes.That is,while an arm keeps contacting the ground or separating from the ground,the arm can move to the objective angular-position.Therefore, when the case of sensor variables changing in the way of moving joint-motors,the angular-position would not correspond to the selected objective position.Fot this reason,there existsuncertainty of the state transition.The reward signal reflects achievement of the given task.We want the robot to go forward,the immediate reward is defined as the speed of the body at each step.Figure 3:Crawling robot.The arm is controlled by two servo motors that react to angular-position commands.Figure 4:Imaginary crawling robot.4.2Implementation for the RobotThe action space has two dimensions,each element has range[0,1].The state space has two dimensions for angular-position of the joints (each element has range[0,1]),one dimension for touch sensor (an element has 0or 1).Therefore,the state space has a three dimensional vector X =(x 1,x 2,x 3).The policy is represented as follows.We construct a 7dimensional feature vector F =(x 1,x 2,x 3,x 4(=1−x 1),x 5(=1−x 2),x 6(=1−x 3),1)based on X =(x 1,x 2,x 3).The seventh element is always set to ing weight vector Θ=(θ1,i ,θ2,i ,θ3,i ,θ4,i ,θ5,i ,θ6,i ,θ7,i ),the action at i -th dimension is selected from the normal distribution of the average of µi =1/(1+exp(−6 k =1θk,i x k ))and the standard deviation of σi =1/(1+exp(−θ7,i ))+0.1.If a selected action is out of range,it is resampled[3].The number of policy parameters is 14(=7x2),and the GA’s search space has 14dimensions.4.3Configuration of Experiment In this experiment,the proposed method(GA-IS )holds 30policies(N =30).At each episode,the agent performs 20steps(M =20).In the generation-alternation,10children are generated by UNDX(C =10).The parameters of UNDX is based on Kita[4],Ono[5].To verify the usefulness of the proposed technique,we compare the proposed method with the naive GA (naive-GA-1,naive-GA-2)and Stochastic Gradient Ascent(SGA )[2].Both naive-GA-1and naive-GA-2don’t use importance sampling.Naive-GA-1’s configuration is basically same to GA-IS ’s(N =30,M =20,C =10).However,because the naive GA don’t estimate the chidlren’s values,the evaluation of children requires interac-tions with the environment using each child’s policy.In this configuration,naive-GA-1re-quires 5(=C/2)times as many interactions as GA-IS does.On the other hand,naive-GA-2performs 600steps at each episode(N =30,M =600,C =10).This configuration corre-sponds to that GA-IS uses the imaginary experiences of 600steps.SGA is a RL approach which is based on gradient descent.But it has probable character so that a hill climbing search can be made.4.4Experimental ResultGA-IS ,naive-GA-1,naive-GA-2and SGA are performed at 30000steps.Figure 5shows these performances.a v e r a g e r e w a r d step Figure 5:The perfoemance of learned policy averaged over 10trials.GA-IS is the proposed method,naive-GA-1and naive-GA-2are the naive GA,SGA is the Stochastic Gradient Ascent for comparison.5DiscussionAccording to Figure 5,the number of steps taken for average reward to reach 5.0is about 10000by GA-IS ,30000by the naive-GA-1.Therefore,the proposed technique made policy learning about three times as faster as the naive GA in this experiment.Consideringthe number of interactions is reduced to 1/5by the proposed technique,GA-IS should learn the policy five times as faster as the naive GA theoretically.We think that one of cause of the gap between theory and practice is the period to accu-mulate experiences in first time.In the proposed method,all the individuals in a population need to hold the experiences interacting with the environment.But each individual holds no experiences in first time,so they need to interact with the environment.The episodes of the number of individuals are needed.In this configuration,it takes MN =600step to accumu-late experiences.If we ignore this period,GA-IS can make policy learning about four times as faster as the naive-GA-1.Moreover,we think that another cause of the gap between theory and practice is that the estimation by importance sampling is not completely correct.We investigated the estimation accuracy of importance sampling in this task.Figure 6shows the children’s values estimated by importance sampling and by maximum likelihood estimate method after 3000steps;here,as to the maximum likelihood estimate method,the policies are actually performed in the environment.Because the proposed method uses experiences of 30episodes of the parents to estimate the values,maximum likelihood estimate method also uses 30episodes.e s t i m a t e d v a l u e children Figure 6:The children’s values estimated by importance sampling and by maximum likelihood estimate method.The error-bars show the average rewards and the max/min rewards in 30episodes,the boxes show the rewards estimated by importance sampling.According to Figure 6,because the values estimated by importance sampling are between the max/min of maximum likelihood estimates and importance sampling can be said to esti-mate the true value well.In the proposed method,the rank-based roulette wheel selection is used in selecting for survival.Therefore,it is thought that there is no remarkable performance decrement of the proposed method resulting from the estimation accuracy of importance sam-pling even if some little errors are included in it.Because naive-GA-2evaluates the children from their experience of 600steps,its accuracy is equal or better than GA-IS .However,according to Figure 5,it turns out that naive-GA-2cannot converge within 30000steps.Finally,naive-GA-2is converged at about 1300000steps.This requires about 130times as much time as the proposed method does.Because SGA is basically a gradient descent method,it tends to lapse into local optima. Indeed,it lapsed into local optima whose value are about3.0in several trials.Contrarily, GA-IS can search globally,so it is stronger for multimodality than SGA.Moreover,SGA is sensitive to their parameters.To obtain a good performance stably,it needs hand tuning. On the other hand,because GA-IS has fewer parameters,a user need not worry about this. However,in speed of learning,a gradient descent method is generally more advantageous than the direct search method like GA.Also in this experiment,SGA’s performance is better than GA-IS’s until4000step.There exist trade-offs between the speed of gradient descent method and the global search capability of the proposed technique.Then,we consider the population size(N),the number of generated children(C),and the length of episode(M).Because N is related to the number of samples used for importance sampling,it influences the estimation accuracy.The larger it becomes,the better the estima-tion accuracy of importance sampling improves.C is related to the search capability of GA.The larger it becomes,the larger the number of individuals which must be estimated by importance sampling becomes.However,that pro-cessing is possible with only numerical computation;the interactions with the environment are not required.This means the strong affinity between the framework of MGG generates many children and the estimation by importance sampling.M is related to the estimation accuracy of values of parents.In general,the culculation of the probability that an episode occurs becomes unstable if it is too long.Actually,we comfirmed that the limit of the length of an episode was about30steps by experiments.To deal with the longer episode is the future work.6ConclusionIn this paper,we proposed a method reduces the number of interactions with the environment, using importance sampling in policy learning by GA.There is a strong affinity between GA and importance sampling for policy learning.The experimental result shows the proposed method can learn policies about three times faster than the naive GA.This means that GA can be a powerful tool for policy learning.The future work is to deal with the longer episode tasks and the higher dimensional tasks.References[1]Goldberg,D.E.:Genetic Algorithms in Search,Optimization,and Machine Learning,AddisonWsley Pub-lishing Company Inc.(1989).[2]Kimura,H.and Kobayashi,S.:Reinforcement Learning for Continuous Action using Stochastic GradientAscent,Intelligent Autonomous Systems(IAS-5),pp.288–295(1998).[3]Kimura,H.,Yamashita,T.and Kobayashi,S.:Reinforcement Learning of Walking Behavior for a Four-Legged Robot,40th IEEE Conference on Decision and Control(CDC2001),pp.411–416(2001).[4]Kita,H.,Ono,I.and Kobayashi,S.:Theoretical Analysis of the Unimodal Normal Distribution Crossoverfor Real-coded Genetic Algorithms,Proc.1998IEEE ICEC,pp.529–534(1998).[5]Ono,I.and Kobayashi,S.:A Real-coded Genetic Algorithm for Function Optimization Using UnimodalNormal Distribution Crossover,in Proc.7th ICGA,pp.246–253(1997).[6]Precup,D.,Sutton,R.S.,and Singh,S.:Eligibility Traces for Off-Policy Policy Evaluation,Proc.17thInternational Conf.on Machine Learning(ICML2000),pp.759–766(2000).[7]Precup,D.,Sutton,R.S.,and Dasgupta,S.:Off-Policy Temporal-Difference Learning with Function Ap-proximation,Proc.18th International Conf.on Machine Learning(ICML2001),pp.417–424(2001). [8]Satoh,H.,Yamamura,M.and Kobayashi,S.:Minimal Generation Gap Model for GAs considering BothExploration and Exploitation,Proceedings of IIZUKA’96,pp.494–497(1996).[9]Shelton,C.R.:Policy Improvement for POMDPs using Normalized Importance Sampling,Proceedings ofthe17th Conference on Uncertainty in Artificial Intelligence(UAI2001),pp.496–503(2001).。