英语印地语新闻语篇重用的语言模式类型(IJITCS-V8-N8-9)
新闻语篇互文性视角下英文报刊的“十字型阅读法”

新闻语篇互文性视角下英文报刊的“十字型阅读法”
李淼
【期刊名称】《经贸实践》
【年(卷),期】2015(0)6X
【摘要】新闻语篇是一种互文性极强的文体,它最大限度的与社会现实和历史融合,同时也承载着检验社会动态,批判社会意识,引导社会舆论的工具和武器的功能。
本文在Kristeva、巴赫金、费尔克拉夫等人的原有理论基础上,对新闻语篇的水平互文性和垂直互文性的向度进行具体化,对其定义和内涵进行调整、扩充、延展和丰富;同时借鉴辛斌教授的新闻语篇研究的部分理论,用于解读新闻语篇特性,从而形成独创性的英语新闻"十字型阅读法"。
【总页数】2页(P148-148)
【作者】李淼
【作者单位】华北科技学院外国语学院英语系
【正文语种】中文
【中图分类】H315
【相关文献】
1.互文性视角下的英语新闻语篇转述话语的批评性分析
2.互文性视角下的英语新闻语篇转述话语的批评性分析
3.互文性视角下英汉新闻语篇的解读——基于民族通婚报道的批评性话语分析
4.互文性视角下新闻语篇的批评性话语分析--以BBC上的报道为例
5.互文性视角下新闻语篇的批评性分析
因版权原因,仅展示原文概要,查看原文内容请购买。
纯英语EBMT法在印地语句子翻译系统中的应用(IJMECS-V6-N7-1)
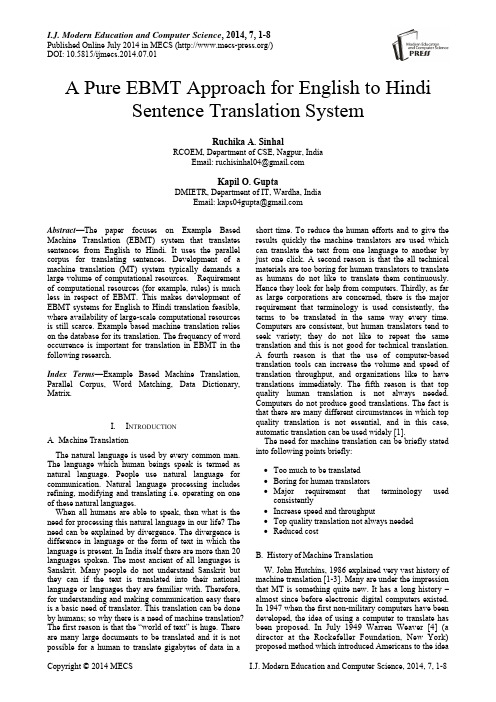
I.J. Modern Education and Computer Science, 2014, 7, 1-8Published Online July 2014 in MECS (/)DOI: 10.5815/ijmecs.2014.07.01A Pure EBMT Approach for English to HindiSentence Translation SystemRuchika A. SinhalRCOEM, Department of CSE, Nagpur, IndiaEmail: ruchisinhal04@Kapil O. GuptaDMIETR, Department of IT, Wardha, IndiaEmail: kaps04gupta@Abstract—The paper focuses on Example Based Machine Translation (EBMT) system that translates sentences from English to Hindi. It uses the parallel corpus for translating sentences. Development of a machine translation (MT) system typically demands a large volume of computational resources. Requirement of computational resources (for example, rules) is much less in respect of EBMT. This makes development of EBMT systems for English to Hindi translation feasible, where availability of large-scale computational resources is still scarce. Example based machine translation relies on the database for its translation. The frequency of word occurrence is important for translation in EBMT in the following research.Index Terms—Example Based Machine Translation, Parallel Corpus, Word Matching, Data Dictionary, Matrix.I.I NTRODUCTIONA.Machine TranslationThe natural language is used by every common man. The language which human beings speak is termed as natural language. People use natural language for communication. Natural language processing includes refining, modifying and translating i.e. operating on one of these natural languages.When all humans are able to speak, then what is the need for processing this natural language in our life? The need can be explained by divergence. The divergence is difference in language or the form of text in which the language is present. In India itself there are more than 20 languages spoken. The most ancient of all languages is Sanskrit. Many people do not understand Sanskrit but they can if the text is translated into their national language or languages they are familiar with. Therefore, for understanding and making communication easy there is a basic need of translator. This translation can be done by humans; so why there is a need of machine translation? The first reason is that the “world of text” is huge. There are many large documents to be translated and it is not possible for a human to translate gigabytes of data in a short time. To reduce the human efforts and to give the results quickly the machine translators are used which can translate the text from one language to another by just one click. A second reason is that the all technical materials are too boring for human translators to translate as humans do not like to translate them continuously. Hence they look for help from computers. Thirdly, as far as large corporations are concerned, there is the major requirement that terminology is used consistently, the terms to be translated in the same way every time. Computers are consistent, but human translators tend to seek variety; they do not like to repeat the same translation and this is not good for technical translation.A fourth reason is that the use of computer-based translation tools can increase the volume and speed of translation throughput, and organizations like to have translations immediately. The fifth reason is that top quality human translation is not always needed. Computers do not produce good translations. The fact is that there are many different circumstances in which top quality translation is not essential, and in this case, automatic translation can be used widely [1].The need for machine translation can be briefly stated into following points briefly:∙Too much to be translated∙Boring for human translators∙Major requirement that terminology used consistently∙Increase speed and throughput∙Top quality translation not always needed∙Reduced costB.History of Machine TranslationW. John Hutchins, 1986 explained very vast history of machine translation [1-3]. Many are under the impression that MT is something quite new. It has a long history –almost since before electronic digital computers existed. In 1947 when the first non-military computers have been developed, the idea of using a computer to translate has been proposed. In July 1949 Warren Weaver [4] (a director at the Rockefeller Foundation, New York) proposed method which introduced Americans to the ideaof using computers for translation. From this time on, the idea spread quickly, and in fact machine translation has been to become the first non-numerical application of computers. The first conference on MT was held in 1952 [5]. Just two years later, there has been the first demonstration of a translation system in January 1954 [6]. Unfortunately it has been the wrong kind of attention as many readers thought that machine translation has been just around the corner and that not only would translators be out of a job but everybody would be able to translate everything and anything at the touch of a button. It gave quite a false impression. However, it has been not too long before the first systems have been in operation, even though the quality of their output has been quite poor. In 1959 a system has been installed by IBM at the Foreign Technology Division of the US Air Force [7], and in 1963 and 1964 Georgetown University, one of the largest research projects at the time, installed systems at Euratom and at the US Atomic Energy Agency. But in 1966 there appeared a rather damning report for MT from a committee set up by most of the major sponsors of MT research in the United States. It found that the results being produced have been just too poor to justify the continuation of governmental support and it recommended the end of MT research in the USA altogether. It advocated the development of computer aids for translators. Consequently, most of the US projects – the main ones in the world at that time – came to an end. The Russians, who had also started to do MT research in the mid 1950‟s, concluded that if the Americans were not going to do it any more than they would not either, because their computers have not been as powerful as the American ones. However, MT did continue in fact, and in 1970 the Systran system has been installed at the US Air Force (replacing the old IBM system), and that system for Russian to English translation continues in use to this day [8]. The year 1976 is one of the turning points for MT. In this year, the Météo system for translating weather forecasts has been installed in Canada and became the first general public use of a MT system [9]. The European Commission decided to purchase the Systran system and from that date its translation service has been developed and installed versions for a large number of language pairs for use within the Commission. Subsequently, the Commission decided to support the development of a system designed to be better than Systran, which at that time has been producing poor quality output, and began support for the Eurotra project – which did not produce a system in the end. During the 1970‟s other systems began to be installed in large corporations [10]. In 1981, came the first translation software for the newly introduced personal computers, and gradually MT came into more widespread use [11]. In the 1980‟s there had been a revival of research, Japanese companies began the production of commercial systems, and computerized translation aids became more familiar to professional translators. Then in 1990, the first translator workstations came to the market [12]. In the last decade MT has become an online service on the Internet [13-14].The term machine translation (MT) is translation of one language to another. The ideal aim of machine translation system is to produce the best possible translation without human assistance. Basically every machine translation system requires automated programs for translation, dictionaries and grammars to support translation [15].The work in this paper is organized in five parts. First part discusses the review of literature on machine translators and the related work in development of example based machine translators. The further discussion focuses on the proposed methodology and the algorithm implemented in translation and training of the system. The implementation and working of the system is discussed later followed by drawn conclusion, result and the future scope for the translation system.II.L ITERATURE R EVIEWA.Example Based Machine TranslationEBMT is a corpus based machine translation, which requires parallel-aligned three machine-readable corpora. Here, the already translated example serves as knowledge to the system. This approach derives the information from the corpora for analysis, transfer and generation of translation. These systems take the source text and find the most analogous examples from the source examples in the corpora. The next step is to retrieve corresponding translations. And the final step is to recombine the retrieved translations into the final translation.EBMT is best suited for sub-language phenomena like –phrasal verbs; weather forecasting, technical manuals, air travel queries, appointment scheduling, etc. Since, building a generalized corpus is a difficult task, the translation work requires annotated corpus, and annotating the corpus in general is a very complicated task.Nagao (1984) has been the first to introduce the idea of translation by analogy and claimed that the linguistic data are more reliable than linguistic theories [16]. In EBMT, instead of using explicit mapping rules for translating sentences from one language to another, the translation process is basically a procedure for matching the input sentence against the stored translated examples. Fig. 1 shows the architecture of a pure EBMT [17].The basic tasks of an EBMT system are –- Building Parallel Corpora- Matching and Retrieval- Adaptation and RecombinationFig. 1 EBMT ArchitectureThe knowledge base, parallel aligned corpora consist of two sections, one for the source language examples and the other for the target language examples. Each example in the source section has one to one mapping in the target language section. The corpus may be annotated in accordance with the domain. The annotation may be semantic (like name, place and organization) or syntactic (like noun, verb, preposition) or both. For example, in the case of phrasal verb as the sub-language the annotations could be subject, object, preposition and indirect object governed by the preposition. In the matching and retrieving phase, the input text is parsed into segments of certain granularity. Each segment of the input text is matched with the segments from the source section of the corpora at the same level of granularity. The matching process may be syntactic or semantic level or both, depending upon the domain. On syntactic level, matching can be done by the structural matching of the phrase or the sentence. In semantic matching, the semantic distance is found out between the phrases and the words. The semantic distance can be calculated by using a hierarchy of terms and concepts, as in WordNet. The corresponding translated segments of the target language are retrieved from the second section of the corpora. In the final phase of translation, the retrieved target segments are adapted and recombined to obtain the translation. It identifies the discrepancy between the retrieved target segments with the input sent ences‟ tense, voice, gender, etc. The divergence is removed from the retrieved segments by adapting the segments according to the input sentence‟s features.B.Machine TranslatorsThe table 1, describes the Example Based Machine Translator Implementation. These translators have different domain of their working. These translators used different computational resources for their operation.Table 1: Example Based Machine Translators∙Anubharti-II TechnologyR.M.K Sinha in 2004, has proposed a system with an approach for machine aided translation having the combination of example-based and corpus based approaches and some elementary grammatical analysis. In ANUBHARTI the traditional EBMT approach has been modified to reduce the requirement ofa large example base. ANUBHARTI-II uses Hindi as a source language for translation to other Indian language [18].∙VAASAANUBAADA A Bilingual Bengali Vijayanand Kommaluri, Sirajul Islam Choudhury and Pranab Ratna in 2002 proposed Assamese automatic MT system for translating the news texts by using the Example Based Machine Translation (EBMT) technique. In this the translation is done at sentence level. Some preprocessing and post processing work has to be done for the translation. The longer sentences were fragmented at punctuation, which gives high quality translations. Backtracking is used when exact match does not occur at the sentence level, which results in further fragmentation of the sentence [19].∙Shiva and ShaktiIn 2004 two MT systems were developed jointly by IIIT Hyderabad, IIS Bangalore and Carnegie Mellon University USA for translation from English to Hindi. The Shiva by Sivaji Bandyopadhyay[20-21], system uses example based approach and the Shakti by R. Moona Bharati, P. Reddy, B. Sankar, D.M. Sharma, R. Sangal[20], system uses rule-based approach with statistical approach for MT. The Shakti system is working for three target languages Hindi, Marathi and Telugu.∙ANUBAD Hybrid Machine Translation SystemSivaji Bandyopadhyay in 2004, proposed a MT system for translating English news headlines to Bengali at Jadavpur University Kolkata[22]. Saha Gautam Kumar in 2005 developed EB-ANUBAD [23], system for translating English to Bengali language and it showed 98% correct results although the output has been in English language. The approach taken for the translation has been the hybrid approach of rule based and transfer based with a parser for both morphological as well as for the Lexical parsing of the text. A brief description of the various machine translation systems is given above in the table 1. ∙IBM-English-Hindi Machine Translation SystemD. Gupta, N. Chatterjee [24] and Raghavendra Udupa, Tanveer A. Faruquie [25] in 2006 proposed to develop a MT based on Example Based approach and later on shifted to the Statistical approach for machine translation from English to Indian Languages in IBM India Research Lab.∙English to {Hindi, Kannada, and Tamil} and Kannada to Tamil language pair EBMTB. K.Murthy, W. R.Deshpande in 2006 [24, 26] developed a MT system based on bilingual dictionary of sentences, phrases, words and phonetic dictionary. Each dictionary contains parallel corpora for the language pair based on EBMT. EBMT has a set of 75000 commonly used sentences from English and translated into the target Indian Languages.III.P ROPOSED M ETHODOLOGYThere are two objectives in the presented research. Proposed algorithms for the two objectives are explained below in stepwise manner. The training corpus is the parallel database containing 677 sentences. The corpus generated is not preprocessed. The examples contained in training corpus are newspaper headlines.The database trained forms the matrix. This matrix can be stored in mat file for later use. Once the system is trained, there is no need to train the system later till there is some modification made in the database.A.Training AlgorithmThe first objective for the system designed is to train the system for translation. The training algorithm stated below is designed to create matrix data structures later used in the translation phase.Step 1: Initialize the Similarity Matrix, Training Matrix and TagMatrix as zero.Step 2: Read first sentence form database.Step 3: Tokenize the string.Step 4: Read sentence from database to compare with the first.Step 5: Tokenize the string. Step 6: Compare two tokens if match found, then update similarity matrix and increment counter for wordelse compare for next word.Step 7: Store the counter for the training matrix.Step 8: Check current variable if less than size ofdatabase, then increment counter and go to Step 4. Step 9: Check current variable if less than size ofdatabase, then increment counter and go to Step 2to reinitialize the counter for the occurrence ofword.The training module forms the matrix; this matrix is used later for matching in translation phase. The indexes of sentence containing the corresponding word are matched to find the common Hindi string which is combined to form the output. The training matrix gives the number of occurrence of the word in the corpus. The system uses these databases as input in translation phase. These data structures are loaded each time.B.Translation AlgorithmThe algorithm for translation of sentence is presented below. The translator performs word based translation. Step 1: Train the database for finding the matrices.Step 2: Read the input string.Step 3: Divide the string into tokens.Step 4: Parse the database for the first word i.e. token. Step 5: If the word is present only once in database, use the dictionary to search for the translation else goto Step 6.Step 6: If the word is present twice in database, then it finds the common string in the Hindi language tofind the match else go to Step 7.Step 7: The corresponding Hindi sentences are taken and the intersection of sentences is found and thecommon string is found.The sentence entered by user is broken into tokens using user defined function. The sentence indexes for these tokens are matched for translation one-by-one from the corpus by user defined String Cmp function. The matched output is then combined to generate the output translated string. The input sentence is not preprocessed to remove stop words e.g. the, is, as etc.IV.I MPLEMENTATIONThe research mainly has two objectives training and translation. Training of database train the database for translation and translator converts the sentence from English to Hindi. These two objectives are explained more in detail below.A.TrainingThe training system objective further is divided in three modules. These modules develop the data structures while training which have been used in later objective. The training is performed using the parallel corpus. Theparallel corpus containing English-Hindi sentence pair is generated. These modules are described in following parts:-∙Development of Similarity MatrixThe sentence is correct with meaningful alignment of the words. The corpus used for training is made of 677 parallel English-Hindi sentence pairs. Similarity matrix is generated using training corpus to find the existence of each word from sentence in the corpus. Each word is checked for its match in entire corpus of English sentences. If the match is found, the value in the matrix is changed to a non-zero value i.e. 1. The non-zero value indicates the presence of a word in database. If the word is present in database the system compute the matrix by placing 1 in place of existence of word. This check is performed for each sentence and each word present in thecorpus. The Similarity Matrix is then stored in .mat format for later use.The values in table 2 show the similarity matrix for first 10 sentences in the corpus. This similarity matrix is calculated for ten English sentences in the corpus.Table 2: Similarity Matrix for 10 sentences∙Development of training MatrixThe training matrix is used to find the occurrence of the word in the entire corpus i.e. the count of the each word in the database. The occurrence gives the frequency of word in the database. The word present in the corpus and its corresponding match can be retrieved using translation algorithm. If the frequency of the word is more, then the accuracy of translation for that word will be more.The values in table 3 are the occurrence for the corresponding word in the sentence.Table 3: Training Matrix for 10 sentencesThe occurrence of the first word for example “Uganda” is 1, similarly for second word “using” is 2. The index of the occurrence in which sentence is found from the Tagmatrix is explained below.∙Development of Tagging Matrix (TagM)The tagmatrix stores the sentence number of the occurrence of the word. The sentences are then compared and the intersection is found for the comparison. The common string for the tag matrix is found for the output. The tag matrix for the first 12 words is shown in the table 4 below the word for corresponding tag is shown in first column. The sentence index is stored in preceding columns. The matching is found with the match using the index of the sentences.Table 4: Tag Matrix for 12 wordsB.Translation of SentenceThis objective translates the sentence from English to Hindi. Word based translation is performed by comparing tokens of the input sentences. The input sentence is divided in word strings called tokens. The space is used as delimiter. The English sentence has SVO sentence structure and Hindi has SOV structure. The correct translation is formed by alignment of text in proper structured format i.e. if sentence is translated from English-Hindi the Subject-Verb-Object is aligned as Subject-Object-Verb in translated output. The translator presented in research-work does not align the text in SOV format for generated Hindi translation. Let us summarize the modules in detail.∙Finding the occurrence of word in databaseThe word to be translated is checked for its occurrence in the corpus. The occurrence of the word is found from the training matrix generated in training phase. TagM matrix gives the indexes of the English sentence in which the word to be translated is present. These indexes are used to retrieve the corresponding Hindi sentences from the corpus. These Hindi sentences are compared to find the common string. The string common in the sentences is the corresponding translation for the word.Matching the word in sentenceThe matching of a word in sentence depends on occurrence of the word in the corpus. The retrieved Hindi sentences are tokenized before matching. The matching for the word has been implemented in three cases.a.Word occurs only once in databaseThe occurrence of the word is found from the training matrix. If the word exists only once in the database, then comparison of two Hindi sentences is not possible. In such case the dictionary is used. The dictionary containing one-to-one translation of English-Hindi words is used. The word is checked in English words corpus. If the word is present, its corresponding match is extracted from the Hindi words corpus.b.Word occurs twice in databaseThe word if present in two English sentences, the corresponding Hindi sentence is retrieved from the corpus. These Hindi strings are passed into user defined function StringCmp( ) which finds the common string comparing both the Hindi sentences.c.Word occurs thrice in databaseThe word if present in more than two sentences that is, if its frequency is greater than or equal to three the sentences, it is intersected using intersect( ) the inbuilt Matlab function. The Hindi sentences are passed as input to the function and the common output string is found. The example explained below describes the processing in detail.For example, the sentence “Kerala state beautiful” is to be translated using the translator, it performs the following steps while translation. The tokens are generated from the input sentence. The sentence is divided in tokens considering space as the delimiter. Therefore the sentence tokens are “Kerala”, “state” and “beautiful”. In the next step, each token is to be processed for translation one-by-one. The occurrence of these tokens is checked from training matrix. The sentences containing these words are found from the tagmatrix. Through the tag of sentences in tagmatrix the corresponding translation is searched for match and translation is extracted from the corpus. Thus the output retrieved is word to word translation.The example is elaborated in following description. The text to be compared is “Kerala”. It is compared in the corpus and the tag matrix the tag matrix is displayed below.TagM = 255 259i.e. word kerala is present in sentence 255 and 259. These two strings are compared using a Stringcmp function. S1= 'bljkbyh' ';qxy' 'fuokZlu' '%' 'dsjy' 'mPp' 'U;k;ky;' 'vnkyr' 'us' 'yxkbZ' 'jksd'S2= 'dsjy' 'esa' 'vkjih,Q' 'la[;k' 'Åij' 'tk' 'ldrh' 'gS'The common string of the two sentences S1 and S2 is found which is …dsjy‟ i.e. translation for “Kerala”. The same procedure is followed for the rest of the tokens in the sentences. If the word occurs in matrix three times, the intersection of sentences found from the tag matrix is found for example “state”. The tag matrix for state is:TagM= 14 98 246i.e. word state is in sentence 14, 98 and 246. These three strings are intersected using intersect function in MATLAB as,S1= 'MkDVj' 'gMrky' 'ij' '%' 'ekSr' 'okyksa' 'dh' 'Vksy' 'la[;k' 'jkT;' 'ds' 'vLirkyks' 'es' 'cMh'S2= 'jkT;' 'ljdkj' 'dks' 'vnkyr' '%' 'D;k' 'flaxwj' ',DV' 'vko‟;d' 'Fkk\\'S3= 'vtsZaVhuk' 'ihfMr' 'jkT;' 'vkradokn' 'ds' 'muds' 'ne' 'ij' 'vc' 'fldkj'The intersection of these three sentences is …jkT;‟ which is concatenated with the previous matched output. Thus the sentences index is used for finding the common string to find the right translation.V.R ESULT A NALYSIS &D ISCUSSIONThe non parallel testing database of 150 English sentences is created to test the precision and word-strength of translator. The precision is the mathematical measure which gives the quantity up to which the output generated is correct. Word-strength gives the mathematical quantity for the exact word translation for the keywords in the sentence.Precision is the measure to check the quality of translation. It is given by the following mathematical formula [27]. The precision for the translator presented in the research work is computed by varying the size of corpus used for translation. The table 5 below shows the precision and word-strength of the output generated through the translator. The quantities make it clear as the size of database increases, the precision of the system also increases. The graph is drawn for the tabulated quantities in Fig. 2.The formula for precision is:-Precision=(1)Precision for 10 sentences = 3 / (3+7)= 0.3Precision for 50 sentences = 6 / (6+4)= 0.6Precision for 677 sentences = 126 / (126+20)= 0.86Table 5: Figures Showing Precision for Translation of the SystemThe graph for the translation precision is drawn below in Fig. 2:Fig. 2 Graph showing the precision of translatorThe database on which testing is performed is also checked for the word strength for the translation generated by the research. The table below gives the details of the word strength in detail.Word Strength= Correct Word Translation / (CorrectWord Translated + Missing Word Translated) (2)Word Strength for 10 sentences = 20/ (20+7)= 0.74Word Strength for 50 sentences = 34/ (34+2)= 0.94Word Strength 677 sentences = 489/ (489+20)= 0.96Table 6: Figures Showing Word Strength for Translation of the SystemFig. 3 Graph showing the word strength of translatorVI. C ONCLUSION AND F UTURE S COPEThis research focuses on simple way of comparing sentence to extract the translation. The research concludes the translator gives the proper expected output to some extent by comparing sentences. The research can be taken to next level by using preprocessed database. The translator presented in the research work does not structure the output sentence in SOV form. Thus the alignment of translation can be done. There is no approach to reduce divergence in generated output, therefore algorithm can be written for reducing the divergence in future.R EFERENCES[1] Hutchins W. John and Harold L. Somers, (1992). AnIntroduction to Machine Translation . London: Academic Press.[2] D. Arnold, L. Balkan, S. Meijer, L.L. Humphreys, L.Sadler: Machine Translation: an Introductory Guide . Blackwells-NCC, London, Great Britain, 1994.[3] Hutchins 95 J. Hutchins: Reflections on the history andpresent state of machine translation. In Proc. of Machine Translation Summit V , pp. 89–96, Luxembourg, July 1995. [4] W.Weaver. Translation. In W.N. Locke, A.D. Booth,editors, Machine Translation of Languages: fourteen essays , pp. 15–23. MIT Press, Cambridge, MA, 1955.[5] John Hutchins, Milestones in machine translation No.4:The first machine translation conference , June 1952 Language Today, no. 13, October 1998, pp.12-13 [Online].20406080100120140Correct Translation Sentences Tested。
试论英语新闻模糊语言的语用分析

试论英语新闻模糊语言的语用分析作者:段钰来源:《西部学刊》2020年第19期摘要:英语新闻属于特殊语篇中的一类,想要增强英语新闻信息传递的质量和效果,不单单需要在英语新闻语言准确方面加以关注,还需要关注和研究英语新闻上存在的模糊语言,使其可以在英语新闻报道当中发挥出关键作用。
“模糊语言”指的是外界不明确、不清晰的,内涵没有指定的特殊性语言,同时具备灵活性和概括性的特点。
在英语新闻中使用模糊语言不仅可以满足新闻受众的阅读心理,顺应新闻报道的特点,而且还能使得新闻报道更加生动,以免新闻报道太过绝对,便于新闻记者对新闻信息量展开动态化调整。
关键词:英语新闻;模糊语言;语用分析中图分类号:H313 文献标识码:A 文章编号:2095-6916(2020)19-0039-03英语新闻属于特殊的一种文体形式,其主要目的是进行客观、精准以及实时的传播各大社会事件[1]。
但必须要看到的一点,就是新闻在具备准确性的同时还有模糊性,这二者属于辩证统一的整体。
这是因为在新闻特性当中,准确性和时效性之间本就潜存着无法调和的矛盾:首先,新闻受众希望可以及时了解到新闻事件;其次,快速进行新闻发布很难确保实际数据,从而达到新闻准确性的要求[2]。
因此,为了最有效地避免新闻报道的风险,在大部分的英语新闻当中都会采用到模糊语言,由此不但可以更好地使受众需求得到满足,也可以为保障新闻时效性奠定较好的基础。
所以,在国内外的英语新闻中对于模糊语言的使用越来越多,其作用也日渐显现,本文将针对英语新闻中的模糊语言展开深入的语用分析。
一、模糊语言的概念、特征以及存在原因(一)模糊语言的概念和特征美国著名的数学家——Zadeh在1965年期间第一次在数学领域引入“模糊”的概念,进而开辟了一个全新的学科,模糊理论就此出现[3]。
在此后的几十年中,模糊理论在不同领域有了更深入地运用,不管是社会还是自然科学领域,都对模糊理论体系有很大程度的研究和借鉴[4]。
新闻英语语篇修辞研究
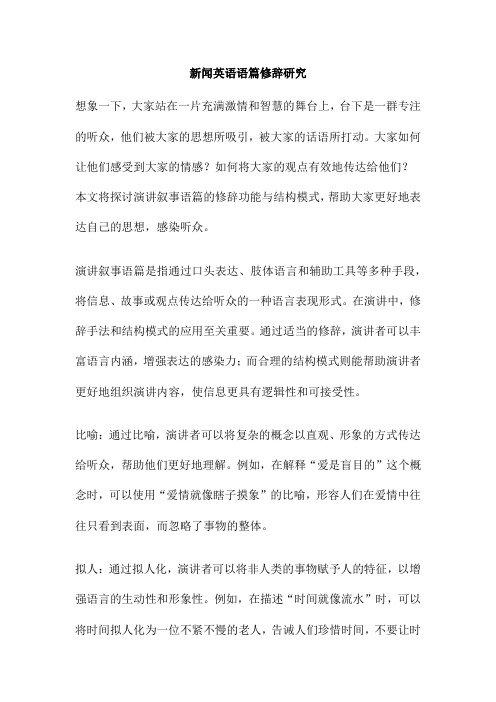
新闻英语语篇修辞研究想象一下,大家站在一片充满激情和智慧的舞台上,台下是一群专注的听众,他们被大家的思想所吸引,被大家的话语所打动。
大家如何让他们感受到大家的情感?如何将大家的观点有效地传达给他们?本文将探讨演讲叙事语篇的修辞功能与结构模式,帮助大家更好地表达自己的思想,感染听众。
演讲叙事语篇是指通过口头表达、肢体语言和辅助工具等多种手段,将信息、故事或观点传达给听众的一种语言表现形式。
在演讲中,修辞手法和结构模式的应用至关重要。
通过适当的修辞,演讲者可以丰富语言内涵,增强表达的感染力;而合理的结构模式则能帮助演讲者更好地组织演讲内容,使信息更具有逻辑性和可接受性。
比喻:通过比喻,演讲者可以将复杂的概念以直观、形象的方式传达给听众,帮助他们更好地理解。
例如,在解释“爱是盲目的”这个概念时,可以使用“爱情就像瞎子摸象”的比喻,形容人们在爱情中往往只看到表面,而忽略了事物的整体。
拟人:通过拟人化,演讲者可以将非人类的事物赋予人的特征,以增强语言的生动性和形象性。
例如,在描述“时间就像流水”时,可以将时间拟人化为一位不紧不慢的老人,告诫人们珍惜时间,不要让时间从指间流逝。
排比:通过排比,演讲者可以突出重点,增强语势,使语言更具表现力和节奏感。
例如,在表达对某事物的强烈情感时,可以使用“我爱……我爱……我爱……”的排比句式,让听众感受到演讲者真挚的情感。
开头:演讲的开头应具有吸引力,能迅速抓住听众的注意力。
常用的开头方式包括设置悬念、引用名言、讲述故事等。
例如,可以通过讲述一个与主题相关的小故事来引出演讲的主题,激发听众的好奇心。
中间过渡:在演讲过程中,如何从一个问题转移到另一个问题,或者从一个观点转移到另一个观点,是考验演讲者功力的关键。
过渡得好,演讲就会如行云流水般自然;过渡得不好,听众可能会感到迷茫。
例如,可以通过“此外”、“而且”等连接词来连接不同的观点或段落。
结尾:演讲的结尾应起到总结和点题的作用。
英语广播新闻话语中的末尾加重句式初探
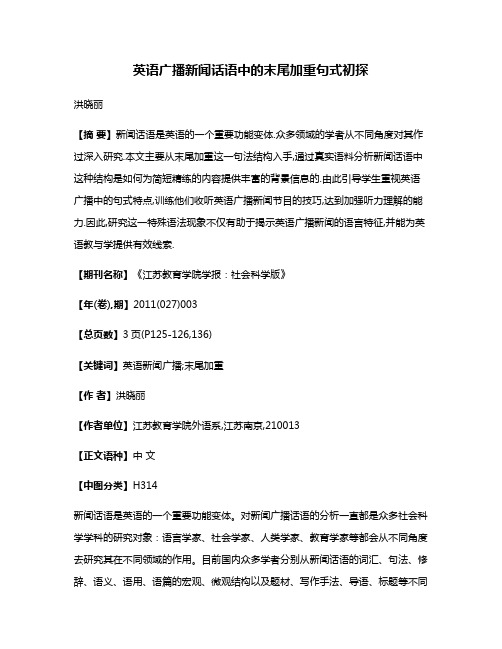
英语广播新闻话语中的末尾加重句式初探洪晓丽【摘要】新闻话语是英语的一个重要功能变体.众多领域的学者从不同角度对其作过深入研究.本文主要从末尾加重这一句法结构入手,通过真实语料分析新闻话语中这种结构是如何为简短精练的内容提供丰富的背景信息的.由此引导学生重视英语广播中的句式特点,训练他们收听英语广播新闻节目的技巧,达到加强听力理解的能力.因此,研究这一特殊语法现象不仅有助于揭示英语广播新闻的语言特征,并能为英语教与学提供有效线索.【期刊名称】《江苏教育学院学报:社会科学版》【年(卷),期】2011(027)003【总页数】3页(P125-126,136)【关键词】英语新闻广播;末尾加重【作者】洪晓丽【作者单位】江苏教育学院外语系,江苏南京,210013【正文语种】中文【中图分类】H314新闻话语是英语的一个重要功能变体。
对新闻广播话语的分析一直都是众多社会科学学科的研究对象:语言学家、社会学家、人类学家、教育学家等都会从不同角度去研究其在不同领域的作用。
目前国内众多学者分别从新闻话语的词汇、句法、修辞、语义、语用、语篇的宏观、微观结构以及题材、写作手法、导语、标题等不同方面将广播新闻与话语分析结合起来进行研究。
本文从末尾加重这一语法特点的角度描述、分析英语广播新闻话语。
如果能在教学中引导学生重视英语广播中的句式特点,将有利于培养他们听英语新闻节目的技巧,加强听力理解的能力。
因此,研究这一特殊语法现象不仅有助于揭示英语广播新闻的语言特征,也能为英语教与学提供有效线索。
新闻报道与大众生活密切相关,因此众学者对于新闻的理解较为一致。
其中王佐良等认为“新闻报道文体指报纸、杂志、广播电台、电视台等大众传媒工具在信息报道中使用的文体”[1](P.244),那么其中报道的内容就是新闻话语了。
《牛津现代高级英汉双解词典》把新闻定义为“新的或新鲜的信息;对于最近发生的事情的报道”。
[2](P.758)李悦娥等将话语定义为:话语是语义和语用上连贯的,用来实现一定的交际目的的一种交际行为的体现,它既可以是书面体也可以是口语体。
新闻英语语法特点与翻译
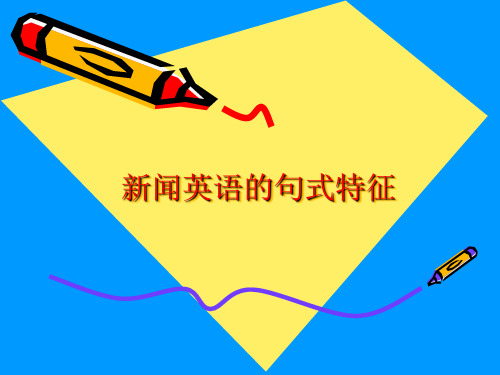
出现在新闻报道标题中,使之简明、清晰、 生动。
• 例如: Center targets kids’ illness. ( China Daily) • (中心致力于儿童疾病的研究。)
• • • • 对比: Co-operatives profit farmers more. (合作经营更有益于农民。) Co-operatives bring more profits to farmers
5. 根据内容灵活运用时态
• 新闻报道的绝大多数是刚刚发生或正在发生的 事情,因此记者常使用一般现在时态和现在完 成时态,以示“新”和“快”;为了把消息绘声 绘色地传达给听众,进行时态使用的也比较普 遍。 • 如新闻标题:
• WARSAW — A plane carrying the
Polish president and dozens of the country’s top political and military leaders to the site of a Soviet massacre of Polish officers in World War II crashed in western Russia on Saturday, killing everyone on board.
2、双重属性词汇大量作为动词使用。
• 既可作为名词又可作为动词使用的双重属性词 汇大量作为动词使用 (如“voice”, “target”, “brief”等词) ,从而使新闻英语生动简明,这是 新闻英语的基本特征。
• The Bush administration had no objection to a trip to China by former president Bill Clinton who was briefed by senior officials. • (据高级官员透露,前总统比尔· 克林顿将出访中国, 布什政府对此未予以反对。)
新闻英语(语言特色)

lead 新闻导语的五个“W”和一个“H” 有时导语过于简洁,记者会在第二段里交待 相对次要的内容,即副导语。 标题是对导语的浓缩和提炼。导语是标题的 扩展
Game together
Tehran– an Iranian journalist, Simon Farzami, has been executed in Tehran for spying for the u.s. embassy and working for late shah’s secret police, friends said Friday. New Delhi, India– close on the heels of a missile test by neighboring Pakistan, India on Friday tested its most sophisticated surface-to-air missile from a remote testing range on the country’s eastern coast, a news agency said.
Headline 新闻标题是新闻不可分割的重要组成部分, 也是学 习者阅读报刊英语的第一步,很多读者首先碰到的难 题是标题。报刊的标题要高度概括新闻的基本内容, 而且要吸引读者的眼球,所以要制作的标新立异和 富有色彩。 背景文化的原因,也会给读者造成理解上的困难。 遣词造句又一套独特的语法体系和标题词汇。
省略动词 Beef Prices Up Again Tube Strikes Off Arafat to Reagan: “We Are Still Here.” Troops Out
省略连词、代词 Woman Kills Husband, Self Anne and Baby Are Well Kings, Sheik Rap US, USSR Volunteer, Terrorist Killed in an Ambush
英语新闻用词特征及翻译方法
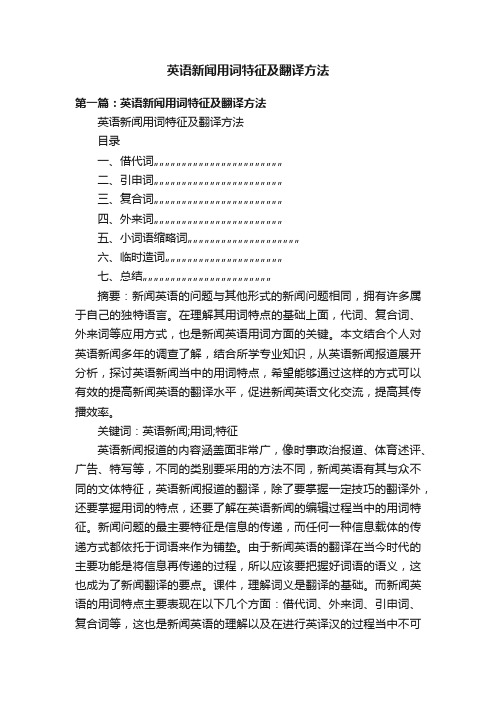
英语新闻用词特征及翻译方法第一篇:英语新闻用词特征及翻译方法英语新闻用词特征及翻译方法目录一、借代词……………………………………………………………二、引申词……………………………………………………………三、复合词……………………………………………………………四、外来词……………………………………………………………五、小词语缩略词……………………………………………………六、临时造词………………………………………………………七、总结……………………………………………………………摘要:新闻英语的问题与其他形式的新闻问题相同,拥有许多属于自己的独特语言。
在理解其用词特点的基础上面,代词、复合词、外来词等应用方式,也是新闻英语用词方面的关键。
本文结合个人对英语新闻多年的调查了解,结合所学专业知识,从英语新闻报道展开分析,探讨英语新闻当中的用词特点,希望能够通过这样的方式可以有效的提高新闻英语的翻译水平,促进新闻英语文化交流,提高其传播效率。
关键词:英语新闻;用词;特征英语新闻报道的内容涵盖面非常广,像时事政治报道、体育述评、广告、特写等,不同的类别要采用的方法不同,新闻英语有其与众不同的文体特征,英语新闻报道的翻译,除了要掌握一定技巧的翻译外,还要掌握用词的特点,还要了解在英语新闻的编辑过程当中的用词特征。
新闻问题的最主要特征是信息的传递,而任何一种信息载体的传递方式都依托于词语来作为铺垫。
由于新闻英语的翻译在当今时代的主要功能是将信息再传递的过程,所以应该要把握好词语的语义,这也成为了新闻翻译的要点。
课件,理解词义是翻译的基础。
而新闻英语的用词特点主要表现在以下几个方面:借代词、外来词、引申词、复合词等,这也是新闻英语的理解以及在进行英译汉的过程当中不可或缺的组成部分。
一、借代词德国新闻学教授法特曾经说过,新闻报道指的是在最短的时间和空间的距离内,将现实进行高度概括的形式,并将它连续介绍给每一个公众。
正由于新闻英语接触者的范围非常广泛,因此他们在理解和阅读的水平上面也是各有差异的,在这个时候就需要记者尽可能的使用形象生动的词语来满足不同公众的阅读和理解需求。
英语新闻的写作形式和语法特点
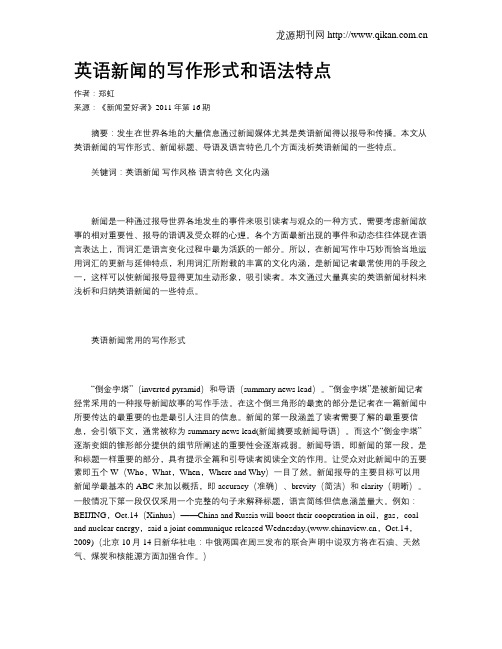
英语新闻的写作形式和语法特点作者:郑虹来源:《新闻爱好者》2011年第16期摘要:发生在世界各地的大量信息通过新闻媒体尤其是英语新闻得以报导和传播。
本文从英语新闻的写作形式、新闻标题、导语及语言特色几个方面浅析英语新闻的一些特点。
关键词:英语新闻写作风格语言特色文化内涵新闻是一种通过报导世界各地发生的事件来吸引读者与观众的一种方式,需要考虑新闻故事的相对重要性、报导的语调及受众群的心理。
各个方面最新出现的事件和动态往往体现在语言表达上,而词汇是语言变化过程中最为活跃的一部分。
所以,在新闻写作中巧妙而恰当地运用词汇的更新与延伸特点,利用词汇所附载的丰富的文化内涵,是新闻记者最常使用的手段之一,这样可以使新闻报导显得更加生动形象,吸引读者。
本文通过大量真实的英语新闻材料来浅析和归纳英语新闻的一些特点。
英语新闻常用的写作形式“倒金字塔”(inverted pyramid)和导语(summary news lead)。
“倒金字塔”是被新闻记者经常采用的一种报导新闻故事的写作手法。
在这个倒三角形的最宽的部分是记者在一篇新闻中所要传达的最重要的也是最引人注目的信息。
新闻的第一段涵盖了读者需要了解的最重要信息,会引领下文,通常被称为summary news lead(新闻摘要或新闻导语)。
而这个“倒金字塔”逐渐变细的锥形部分提供的细节所阐述的重要性会逐渐减弱。
新闻导语,即新闻的第一段,是和标题一样重要的部分,具有提示全篇和引导读者阅读全文的作用。
让受众对此新闻中的五要素即五个W(Who,What,When,Where and Why)一目了然。
新闻报导的主要目标可以用新闻学最基本的ABC来加以概括,即accuracy(准确)、brevity(简洁)和clarity(明晰)。
一般情况下第一段仅仅采用一个完整的句子来解释标题,语言简练但信息涵盖量大。
例如:BEIJING,Oct.14(Xinhua)——China and Russia will boost their cooperation in oil,gas,coal and nuclear energy,said a joint communique released Wednesday.(,Oct.14,2009)(北京10月14日新华社电:中俄两国在周三发布的联合声明中说双方将在石油、天然气、煤炭和核能源方面加强合作。
0507高三英语英语新闻阅读与语言应用
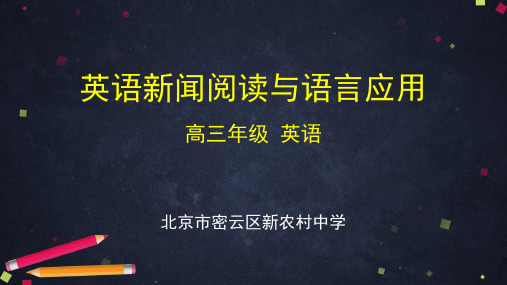
1. is 2. say
3. according to
解读标题,感知新闻内容——分析标点
1. Cat lives alone for two months, gives birth and
2. Travelers use festival for visits, sightseeing
解读标题,感知新闻内容——分析标点
英语新闻的结构
倒金字塔型结构
英语新闻的结构
倒金字塔型结构 金字塔型结构
菱形结构 总分结构 并列结构
二、英语新闻的阅读方法
解读标题,感知新闻内容 细读导语,获取新闻要义 研读主体,理解新闻实质
解读标题,感知新闻内容——划分意群
意群的英语名称为“sense group”,是指相邻的单个字 词在意义、语法之间的联系。在意思上具有相对的完整性,因 此在理解上不可断开。意群可以是一个词、一个短语、或一个 简短的句子。
细读导语,获取新闻要义——读取六要素
American singer, Christine Welch, has followed her love of Chinese poetry, literature and philosophy and forged a path to musical success in China, Chen Nan reports.
2022北京冬奥会中英文报道中转述型言据标记对比研究

2022北京冬奥会中英文报道中转述型言据标记对比研究2022北京冬奥会中英文报道中转述型言据标记对比研究引言:2022年北京冬奥会作为中国举办的第一个冬季奥运会,备受全球关注。
作为重大体育赛事,不同国家和地区的媒体对于报道语言的运用有着不同的风格和习惯,中英文报道的语言差异尤为显著。
本文旨在通过对比研究中英文报道的中转述型言据标记,深入了解两种语言报道的特点和风格。
一、中文报道的特点和风格中文报道注重表达思想的连贯性和完整性,采用较为复杂的推论和总结,并且通过辞藻华丽的修饰让读者产生强烈的感受。
中文报道常使用修辞手法,如比喻、夸张、排比、反问等,以增加情感的表达和文章的吸引力。
另外,中文报道在选择言据时,会尽量使用句子结构完整的言据,以确保句子的通顺和流畅。
这些特点使得中文报道显得丰富而华丽。
二、英文报道的特点和风格英文报道注重表达事实的准确性和简洁性,采用直接陈述和明了的论证。
英文报道常使用简洁明了的句子结构,避免冗长和累赘的修辞。
此外,英文报道在选择言据时,注重事实的证据性和客观性,通常使用简单的、干练的句子来传递信息。
这些特点使得英文报道显得简洁而明了。
三、中英文报道的中转述型言据标记对比1. 直接引用直接引用是中英文报道中最为常见的中转述型言据标记,用于直接引用相关人士的话语、言论或评论。
中文报道常会使用辞藻华丽的修辞手法来增加引用语的表达力,如:“我们坚信,冰雪运动的魅力将汇聚全球,点燃北京冬奥场馆的激情。
”(中文报道)而英文报道则更加注重简洁直接的表达:“We firmly believe that the charm of winter sports will gather the world and ignite the passion of Beijing Winter Olympics venues.”(英文报道)2. 间接引用间接引用是通过报道者对相关信息进行转述后再传递给读者。
英语新闻常用句子特征例析

英语新闻常用句子特征例析英语新闻常用句子特征例析新闻报道文体属于较正式的文体,语言结构比较复杂。
但它并不晦涩难懂,而是简洁明确, 主题突出,语言客观,显示出自己的独特风格。
新闻分书面和口头两大类,分别以报刊与广播为代表,二者在写作风格和技巧上大同小异。
除了语篇和词汇外,新闻的行文风格和技巧亦表现在句子及其使用上。
新闻报道语句是构成新闻语篇的独立的基本语义单位,既受制于新闻语篇的要求,又服务于新闻语篇。
因此,新闻语句的结构和句式要符合新闻报道的结构需要和行文规范。
新闻报道语篇一般由标题、导语、正文、背景和结尾组成,其用语要求具体、准确、简明、通俗和生动,所以句子也要反映这些语言要求,与之相辅相成,发挥出支持新闻语篇的作用。
因此,在新闻撰写中,习惯采用许多特定结构的语句,如新闻体语句、扩展的简单句、主从复合句、解释性语句等,这些常用句子或多或少显示新闻文体色彩、结构简洁紧凑、内容丰富明确、修辞手段多样和口语化倾向等特征。
(一)文体色彩较浓一文体色彩较浓在报道中,出现最多最明显的是新闻体词语( journalistic expressions) 。
新闻体词语是指媒体出于行文需要,使用频率较高的涉及新闻事件、人物和机构等的词语,即新闻的“套话”、“行话”, 包括报道套语、文头惯用语、分类常用语等, 它们在报道中单独成句或形成含有新闻体词语的惯用句式。
具有语义简明准确、位置相对固定, 使用快捷方便等特点。
虽然有些新闻体词语由于用得太多太久给人以陈旧呆板之感, 但还是不时出现在新闻的各个部分。
可以说,各种新闻体词语在报道里随处可见, 作用不一,如: as 话; 匿1. 表示信息来源出处 quote ? 引用? who spoke on condition of anonymity 以? 名为条件; a spokesman says 发言人说officials / sources/ critics/ analysts/observers / skeptics say 政府官员们/有关人士/评论家/分析家/观察家/怀疑者说; declined to comment on /elaborate o n /give one’s name /be named 谢绝评论/提供详细情况/披露本人姓名; Witnesses said? 目击者说; Reports from ? 来自? say 的报道说; opinion polls show 民意调查显示;studies show 研究显示。
中英新闻语篇转述引语对比分析——从批评话语分析的角度

中英新闻语篇转述引语对比分析——从批评话语分析的角度
当前,协调通过英语和中文沟通的非母语人士的数量正在不断增加。
英文和中文作为两个
最大的语言系统之间的新沟通模式正在诞生。
英语和中文之间的转述引语在批评话语分析
方面是一种重要的研究,其目的在于探究中英新闻语篇转述引语在实际使用中有什么样的
不同。
首先,从差异分析的角度来看,英语与汉语在口语表达方面存在很大的差异。
当英文报纸
翻译成汉语时,往往会被翻译成更加具象、甚至有节奏的表达形式,而英文原文往往会更
加直接、简洁、明了的表达出一个概念。
翻译时要注意翻译的表达连贯性。
英语中和中文
中的语篇结构也会有很大的差异,英语句式更紧凑,而中文多数词语更长篇幅,会更具有
表达思想的强度。
再者,从文化习惯角度来看,英语更偏向于抽象的表达形式,而中文更
具有具体的文化内涵的表达。
只有当这两种不同的文化习惯完美结合时,才能表达出原文
中最深层的文化含义。
从以上分析可以看出,中英新闻语篇转述引语的差异性很大,有必要采取一些有效的补救
措施以避免翻译中存在的风险。
例如,更加重视汉语表达方式的文化差异,把握翻译过程
中准确理解和表达本质内容所必要的技能,抓住字面意义与隐喻之间的联系等。
只有这样,才能真正做到在表达上的真实性和可操作性之间取得最佳的平衡。
英语新闻中的习语使用

古典文学名著和诗歌是成语来源之 一
1.西方文化源头是古希腊和由之脱胎而来的古罗马神话,如: Achilles’ heel 可乘之机 出自希腊神话 between Scylla and Charybdis 进退维谷 出自希腊神话 fish in troubed waters 浑水摸鱼 典出《伊索寓言· 渔夫》 2.汉语成语也和本国寓言故事,神话 传说有关: ”一鸣惊人“,”愚公移山“ 出自古代寓言 ”精卫填海“,”画龙点睛“ 出自神话传说
不同名族语言反映了本民族的历史 背景
许多成语是历史经验的总结,因而有其鲜明的名族特色 如:英语成语 burn one’s boat 和汉语成语“破釜沉舟” 意 义相同,但来自不同的历史背景 Burn one’s boat 反映的是公元49年,古罗马凯撒等将士赌 过卢比根河时,下令烧毁船只的情况。士兵后腿无路,只 有勇往直前,战胜敌人。 汉语的“破釜沉舟”出自《史记· 项羽本记》
English Idioms Vs Chinese Idioms in the News
英汉成语在性质上有其共性,它们是人们长期使用过程中 形成的固定的词组 各民族文化背景虽然不尽相同,但人类的思维却有着共同 的轨辙。因此,在英汉成语使用中存在着一些意义和文化 含义相同或相似的成语,例如: Plain sailing 一帆风顺 A drop in the ocean 沧海一粟 Walls have ears 隔墙有耳 as far as anyone knows 众所周知
Some news sentences including idioms
1.They have certainly been successful in killing two birds with one stone. 一箭双雕 2.”All of us are being asked to shed sweat and tears now.” 卧薪尝胆
英语新闻导语

▪ It sounds like the plot of a Hollywood thriller but it’s true: Just nine days before this year’ s Academy Awards,Oscar has disappeared, the FBI is hunting for him and a reward of 50,000 has been offered for his return.
▪ 一切就好像是好莱坞编造出来的惊险故事 一样,但一切又都是真真切切发生的事实: 就在离举行奥斯卡仪式还剩9天时,奥斯卡 金像突然不翼而飞。为了侦破这起神秘的 盗窃案,联邦调查局被请来了,悬赏破案 的赏金是5万美元。
▪ 导语中只交待了“事件”这一个新闻要素, 时间要素虽然交待了,但也是间接交待的 “离举行奥斯卡仪式还剩90”,其余要素根 本就没交待了。而其语言却极富文学色彩, 使趣味性和可读性很强,读者的胃口被吊了 起来,让人欲罢不能,接着去读主体新闻, 完成了导语的功能,达到了导语之目的。
▪ 上月,GeraldoAsamoah终于用脚将自己 踢进了足球史。他成为穿着德国国家足球 队队服的第一位非洲裔黑人球员。
▪ 这条导语将整个新闻的主要事实,用概括 性的语言叙述了出来,读者读完导语便知 道整个新闻大概了,要想详细了解新闻背 景后的新闻,便必须继续进行阅读,读者 的胃口也被吊了起来。当然,其概括是对 具体新闻事实的概括,而非抽象空洞的概 述。
系统功能语法角度下新闻英语语篇特点分析
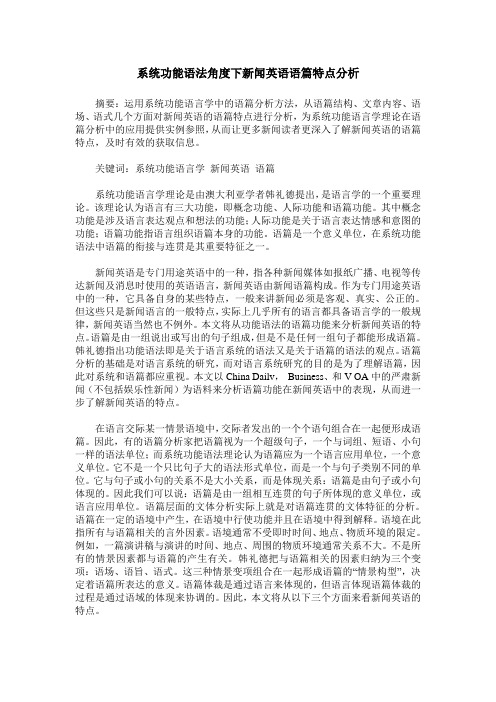
系统功能语法角度下新闻英语语篇特点分析摘要:运用系统功能语言学中的语篇分析方法,从语篇结构、文章内容、语场、语式几个方面对新闻英语的语篇特点进行分析,为系统功能语言学理论在语篇分析中的应用提供实例参照,从而让更多新闻读者更深入了解新闻英语的语篇特点,及时有效的获取信息。
关键词:系统功能语言学新闻英语语篇系统功能语言学理论是由澳大利亚学者韩礼德提出,是语言学的一个重要理论。
该理论认为语言有三大功能,即概念功能、人际功能和语篇功能。
其中概念功能是涉及语言表达观点和想法的功能;人际功能是关于语言表达情感和意图的功能;语篇功能指语言组织语篇本身的功能。
语篇是一个意义单位,在系统功能语法中语篇的衔接与连贯是其重要特征之一。
新闻英语是专门用途英语中的一种,指各种新闻媒体如报纸广播、电视等传达新闻及消息时使用的英语语言,新闻英语由新闻语篇构成。
作为专门用途英语中的一种,它具备自身的某些特点,一般来讲新闻必须是客观、真实、公正的。
但这些只是新闻语言的一般特点,实际上几乎所有的语言都具备语言学的一般规律,新闻英语当然也不例外。
本文将从功能语法的语篇功能来分析新闻英语的特点。
语篇是由一组说出或写出的句子组成,但是不是任何一组句子都能形成语篇。
韩礼德指出功能语法即是关于语言系统的语法又是关于语篇的语法的观点。
语篇分析的基础是对语言系统的研究,而对语言系统研究的目的是为了理解语篇,因此对系统和语篇都应重视。
本文以China Dailv,Business、和V OA中的严肃新闻(不包括娱乐性新闻)为语料来分析语篇功能在新闻英语中的表现,从而进一步了解新闻英语的特点。
在语言交际某一情景语境中,交际者发出的一个个语句组合在一起便形成语篇。
因此,有的语篇分析家把语篇视为一个超级句子,一个与词组、短语、小句一样的语法单位;而系统功能语法理论认为语篇应为一个语言应用单位,一个意义单位。
它不是一个只比句子大的语法形式单位,而是一个与句子类别不同的单位。
- 1、下载文档前请自行甄别文档内容的完整性,平台不提供额外的编辑、内容补充、找答案等附加服务。
- 2、"仅部分预览"的文档,不可在线预览部分如存在完整性等问题,可反馈申请退款(可完整预览的文档不适用该条件!)。
- 3、如文档侵犯您的权益,请联系客服反馈,我们会尽快为您处理(人工客服工作时间:9:00-18:30)。
I.J. Information Technology and Computer Science, 2016, 8, 75-86Published Online August 2016 in MECS (/) DOI: 10.5815/ijitcs.2016.08.09Typology for Linguistic Pattern in English-HindiJournalistic Text ReuseAarti KumarDepartment of Computer Applications, Maulana Azad National Institute of Technology, Bhopal-462003, IndiaE-mail: aartikumar01@, Mob: +919303132828Sujoy DasDepartment of Computer Applications, Maulana Azad National Institute of Technology, Bhopal-462003, IndiaE-mail: sujdas@, Mob: +919826345195Abstract —Linking and tracking news stories covering the same events written in different languages is a challenging task. In natural languages same information may be expressed in multiple ways and newspapers try to exploit this feature for making the news stories more appealing. It has been observed that the same news story is presented in same as well as in different language in different ways but normally the gist remains the same. Diversity of linguistic expressions presents a major challenge in identifying and tracking news stories covering the same events across languages , but doing so may provide rich and valuable resources as comparable and parallel corpora can be generated with this resource. In the case of Indian languages there exist limited language resources for Natural Language Processing and Information Retrieval tasks and identifying comparable and parallel documents would offer a potential source for deriving bilingual dictionaries and training statistical Machine Translation systems. Paraphrasing is the most common way of reproducing news stories and translated text is also a type of paraphrase. Prior to linking monolingual or bilingual news stories, these paraphrase types need to identified and classified to help researchers to devise techniques to solve these challenging problems. English-Hindi language pair not only differs in their scripts but also in their grammar and vocabulary. A number of paraphrase typologies have been built from the perspective of Natural Language Processing or for some or the other specific applications but as per the knowledge of the authors, no typology have been reported for English-Hindi cross language text reuse. In this paper a typology is formulated for cross lingual journalistic text reuse in English-Hindi. Typology unravels level of difficulties in English-Hindi mapping. It shall help in devising techniques for linking and tracking English-Hindi stories Index Terms —Paraphrasing, typology, linguistic transformation, lexical, cross-lingual, journalistic text reuse. I. I NTRODUCTIONNewspapers report events that are taking place in anypart of the world at more or less same time across different languages. Any news conveys same facts across language but news reporters try to incorporate their viewpoints according to their findings. Linking news stories covering the same events and with same content written in different languages may provide rich and valuable multilingual resources of both parallel and comparable text. Translation equivalents provides parallel fragments and paraphrases provides comparable fragments [2]. Guan and Yuan [29], while working with mislabeled data, have also emphasized on the importance of pattern classification in machine learning. In case of Indian languages there exist limited language resources for Natural Language Processing (NLP) and Information Retrieval (IR) tasks and identifying comparable and parallel documents would offer a potential source for deriving bilingual dictionaries and training statistical Machine Translation (MT) systems [2, 19]. Paraphrasing is the most common way of reproducing news stories. In paraphrasing, substitution for semantic equivalents and grammar, are performed over the text which make even similar contents difficult to identify. Although, linguistic transformations take place in the paraphrased sentence but meaning is still preserved. Translated text in a different language is also a type of special kind of paraphrasing [4]. In order to determine what paraphrasing types make text reuse detection harder to be revealed, analysis, identification and classification of the different types of paraphrasing strategies applied during the text re-use process is important. Typology is nothing but drawing boundaries among different paraphrase types, identifying their manifestations, going into depth to find their characterization and finally classifying them [4]. Building a Typology has been a tool for many NLP researchers to apprehend paraphrasing [24]. Knowledge of paraphrase typology will help in identifying and linking similar news stories by applying suitable techniques. It is also an important aspect in IR research which also deals with document representation languages and models, and finding similar matching contents from documents collections on the web [30].Therefore in this paper paraphrases are identified across English-Hindi language and a Typology for English-Hindi journalist text reuse has been proposed. It is apioneer work in context with English-Hindi journalist text reuse and unravels level of difficulties in English-Hindi mapping. The proposed typology has been built by considering other monolingual typologies given by different authors and comprises of previously defined categories in addition to many new categories to encompass the unique cases of English-Hindi cross-lingual journalistic reuse. The existing typologies have either been mapped in context to English Hindi language or are modified according to the intrinsic representation of the transformation across these two languages. The proposed typology may help in devising techniques for linking similar paraphrased contents in in English Hindi document pairs.The rest of the paper is structured as follows: In Section II various monolingual typologies given by different authors are discussed. In Section III proposed paraphrase typology for English-Hindi journalistic text reuse is discussed. Section IV presents discussion on the typology classes in context to empirical evidence and Section V presents the conclusion.II.C HRONOLOGICAL R ELATED W ORKEarly works on paraphrase typologies are by Culicover [9] in1968 and Honeck [17] in 1971. They divided paraphrase types into those classes which can either be formally mapped in natural language processing or cannot be.Culicover [9] logically grouped paraphrasing into five types and separated accessible paraphrase relationships from inaccessible ones.A taxonomy in the fields of Psychology was given by Honeck [17] which classified three types of paraphrases including transformational, lexical and formalexic.As reported by Vila et al.[23] in 2011 Apresjan (1973) mainly dealt with lexical paraphrases and Martin (1976) focused mainly on connotation, opposition and synonymy based paraphrases.An editing taxonomy has been given by Faigley & Witte [15] in 1981 which divides revisions into two major categories; surface changes and meaning changes, each of which have 2 subcategories finally culminating into 23 types at the lowest level.Dras [13] in 1999 studied syntactic paraphrases using Synchronous Tree Adjoining Grammars and classified paraphrasing types into classes based either on the formal change observed in the paraphrase pair or according to the paraphrase effect which makes them not mutually exclusive. The five classes of paraphrase that he identified are Change of Perspective, Change of Emphasis, Change of Relation, Deletion, and Clause Movement which are further divided into 51 sub-types. Barzilay et al. [5] in 1999, Dolan et al. [11] in 2004 and Dutrey et al. [14] in 2011 gave an NLP typology of the most frequent types in a corpus whereas Kozlowski et al. [18] in 2003, Dorr et al. [12] in 2004 and Boonthum [7] in 2004 concentrated on the paraphrases that NLP addresses. Rinaldi et al. [21] in 2003 focused on classic paraphrases with illustrative purposes.16 obfuscation types were reported by Clough [8] in 2003 in his paraphrase typology which dealt with text reuse.Conversives, non-literal language use and extended paraphrases were studied by Dorr et al. [12] in 2004 while dealing with paraphrases with equivalent meanings. He focused on the syntax, lexicon, and grammatical features of the paraphrases.Based on the type of linguistic units or the range of difference between the original and paraphrased sentences Shimohata [22] in 2004 has classified the paraphrase into three types only-Sentential, Phrasal and Lexical. Each paraphrasing type requires a different kind of knowledge to deal with. Sentential paraphrasing requires pragmatic knowledge, phrasal paraphrasing requires syntactic knowledge, and lexical paraphrasing requires lexical knowledgeFujita [16] in 2005 analyzed a variety of linguistic phenomena in Japanese and provided a more detailed classification of paraphrases than in Shimohata [22]. He classified them on the basis of their similarities and differences in syntactic characteristics. He presented a classification of lexical and structural paraphrases grouped into six classes including paraphrases of single content words, function-expressional paraphrases, paraphrases of compound expressions, clause-structural paraphrases, multi-clausal paraphrases, and paraphrases of idiosyncratic expressions. These where further subdivided into 24 types.Barreiro [3] in 2008 divided paraphrases into 5 classes- referential, lexical, phrasal, syntactic, lexical-syntactic and paraphrasing of multiword expression. The typology is based on the extent of paraphrasing within a sentence ranging from a single lexicon to a phrase to more than one phrase or more than one level of paraphrasing.Clough and Gaizauskas [25] in 2009 studied journalistic text reuse and gathered three recurrently applied operations which are analogous to some entries of their typology: deletion, lexical substitution, changes in syntax and summarization.A general typology of quasi-paraphrases together with their relative frequencies has been given by Bhagat [6] in 2009. The basis of classification of paraphrases i s lexical and each of the types of paraphrase is linked to the compositional alterations involved.Marta Vila et al. [23] in 2011 hypothesize that there exists a correlation between the differences in propositional content and the differences in wording on the one hand, and the degree of sameness of meaning or paraphrasability on the other, both being gradual properties. The typology they have presented classifies paraphrases according to the linguistic nature of their difference in wording and consists of a two-level typology of 2 paraphrasing types grouped into 5 classes. Paraphrasing types reflect a general paraphrase mechanis m and classes represent the level of language where this mechanism takes place.The paraphrase typology given by Barron et al. [4] in2012 relies on the paraphrase concept defined by Recasens and Vila [20] in 2010 and Vila et al. [23] in 2011. It consists of an upgraded version of the one presented in the latter. Their typology also consists of a two-level typology but of 20 paraphrase types instead of 9 there grouped into six classes instead of 5.Marta Vila et al. [24] in 2014 in their recent work refined their former typology and have given a new three level typology of 24 paraphrase types grouped in 5 classes.The paraphrase typologies and their basis are compiled in Fig. 1a and Fig. 1b.Although some work has been done towards finding text reuse or linking news stories in English-Hindi but as per the knowledge of the authors, no paraphrase typology for these two language pairs has been reported so far. Also, the work done in these two language pair is directly proportional to the tasks defined by FIRE since 2009.Fig.1a. Paraphrase TypologiesFig.1b. Paraphrase TypologiesEnglish and Hindi languages not only differ in scripts but also in their grammar and vocabulary. English stores the meaning of the words in positions whereas Hindi, in morphemes. Identifying equivalent translated text across language becomes a challenging task as this category of text can be treated as obfuscation as well as paraphrasing. Identifying parallel contents in cross language news becomes even more complex if too much of alternation has been done to the translated news stories.III.P ROPOSED T YPOLOGYAlthough a pioneer work in the field of English-Hindi language text reuse, the typology has been built by considering other monolingual typologies covered in the related work section. It aims to cover most of the phenomena described in these typologies. As the works of other authors, referred in this research paper, are primarily based on monolingual paraphrasing, therefore some classes that are not finding relevance across the language are dropped here. In the proposed typology, apart from inclusion of some of the previously defined categories, some new categories are introduced by us to signify their importance for cross language text reuse. The previously defined categories are followed by citations of the authors who have proposed them. Categories without any citation are the new categories proposed by us.The typology is strictly formulated for cross lingual news stories covering English-Hindi language. Cross Language Indian News Story Search (CLINSS) corpus1 of FIRE 2012 and 2013 with 50691 files, English newspaper Hindustan Times and Hindi newspaper Dainik Bhaskar has been used as the corpus for the study and for inferring a typology for cross language news story. The parallel stories have been extracted from these newspapers manually and have been retrieved from CLINSS corpus using relevance judgment file provided by them. Text alignment was done manually by the authors themselves. The categories are classified to be in isolation but some of them overlap i.e. two classes can co-exist. For example, if there is a sentence split, there is addition of words also. Any paraphrased parallel sentence in majority of the reported news is a combination of more than one such category. Still, while discussing any particular category of typology, only that category of paraphrasing is emphasized at that point.The classification has been done on the basis of extent of words in the sentences which are paraphrased and on the basis of difficulty in automatic identification of cross lingual news stories. Five difficulty levels have been identified and each level describes the extent of paraphrasing.The Hindi words/phrases/sentences which have been used as examples under each level also have their transliterated English versions following them, within brackets, for the ease of understanding by those who are not the native speakers of Hindi language.1http://users.dsic.upv.es/grupos/nle/clinss.htmlAs the following examples have been taken from original news stories, some names have been changed/hidden wherever found necessary, in view of keeping work purely for the purpose of research and not to hurt any sentiments.A. Level INews stories that are almost exact translations of their English counterpart fall under this category. 1(b) and 2(b) are nearly exact Hindi translation of 1(a) and 2(a). In such cases simple dictionary based cross language approach may be fruitful to retrieve same news story for text reuse1 a). Palson owner convicted in attempt-to-murder case 1 b). हत्माकीकोशििकसभेंऩारसनकभाशरकदोषी(hatya ki koshish mein palson ke maalik doshi)2 a). We wanted to know where all were the camps, who were in charge.2 b).हभजानना जाहतेथेकककऩ कहाॊ-कहाॊरगाएगएथेऔयउनकाइॊचाजजकौनथा।(hum janana chahte the ki camp kahan kahan lagaye gaye the aur unka incharge kaun tha)B. Level IIIn this level key content words in Hindi are unambiguously mapped from E1to H1 set but sentences may have a few additions/deletions of words or trivial modifications in one language or other. Linking Cross language news stories needs to map these words. Gist or meaning in this level is preserved. Categories identified under this level have also been reported in monolingual text reuse.B.1. Word Insertion/DeletionNew in formation is added to a sentence by adding or deleting words [4, 23], leading to a paraphrase at the time of cross language text reuse (3(a) & 3(b) and 4(a) & 4(b)). It may have minor syntactic transformation or lexical replacement. Robin [26] in 1994 introduced the term …Information adding‟ paraphrases for such type of paraphrase.3 a). The base price of $225-million remains the same.3 b). उन्होंनेकहाककटीभोंकाफेसप्राइस 22.5 कयोड़डॉरयमानीकयीफ10 अयफरुऩमेहीयहेगा(unhone kaha ki teamon ka base price 22.5 karod dollar yani kareeb 10 arab rupye hi rahega)4 a). This has remained secret until now.4 b). दस्तावेजोंकइसतयहनष्टमागभहोजानेकायाजआजतकफनाहआथा।(dastavejon ke is tarah nasht ya gum ho jaane ka raaj aaj tak bana hua tha)In 3(b) and 4(b) underlined lexical units are added but same information of 3 (a) and 4 (a) is communicated. In 3 (b) few lexical units are transliterated instead of translation such as “base price”is transliterated as “फेसप्राइस”.In case of deletion of lexical unit normally words in a sentence that are superfluous or peripheral in sentence are removed. The constituents deleted are: hedging verbs, relative pronouns etc. [13]. In 5 (b) “will completely” and “from circulation” are removed while translating 5 (a). This may be done to shorten the news stories.5 a). {..} will completely withdraw from circulation {..} 5 b). {..} वाऩसरेगा({..}wapas lega)B.2. Sentence Split/JoinThe information may be spread over more than one sentence or may be combined in single sentence. These types of paraphrases have two components text units and connective between clauses which is normally altered [13]. The sentence in 6 (a) has been split and translated into two sentences in Hindi 6 (b).6 a). Ramesh, 50, who was serving his life imprisonment, is survived by his wife and two children Rakesh and Karuna both of whom are college students.6 b). यभेिकऩरयवायभेंउसकीऩत्नीऔयदोफच्चेयाकितथाकरुणाहैं।दोनोंकॉरेजकववद्माथीहैं।(Ramesh ke parivar mein uski patni aur do bachche rakesh tatha karuna hain. Dono college ke vidyarthi hain) B.3. Change in ModalityThe modality of the sentence may also be changed (7(a) & 7(b) and 8(a) & 8(b)). Normally they may also be considered in discourse based change in which structure of the sentence is normally changed [4, 24].7 a). He won‟t be able to make {..} into {..}7 b). उनकीहशसमतनहीॊ{..}को{..}फनानेकी(unki haisiyat nahin{..} ko {..} banana ki)8 a). The meaning of {..} is 24 hours electricity8 b). जानतेहैं{..}फनानेकाभतरफ? {..}फनानेकाभतरफहोताह24 घॊटबफजरी.(jante hain {..} banane ka matlab? {..} banana ka matlab hota hai 24 ghante bijli) B.4. Passive vs. Active/Direct-Indirect Style Alteration/Voice Alternation/Change of Emphasis [4, 13,23, 24]It involves syntactic reorganization and contains those diathesis alternations where the meaning is preserved but the voice or style is changed at the time of translation (9(a) & 9(b) and 10 (a) & 10 (b)).9 a). Water released from the dam completely submerged the fields9 b). फाॊधसेजायीऩानीभेंखेतऩयीतयहजरभग्न(baandh se jaari paani mein khet poori tarah jalmagn) 10 a). "Of course I am disappointed. But it was thedecision of the governing council," he said10 b). आईऩीएरकशभश्नयनेफादभेंकहाककटेंडययद्दहोनेसेहाराॊककवहननयािहैंरेककनमहगवननिंगकाउॊशसरकापसराह।(IPL commissioner ne baad mein kaha ki tender radd hone se halanki who nirash hain lekin yeh governing council ka faisla hai)B.5. Representational ChangeMany times category of noun may be changed at the time of translation. In 12 (b) natives are represented by country at the time of translation from 12 (a).11 a). Indians showed great restraint after the last {..} attack11 b). वऩछरे{..}हभरेकफादबायतनेजफदजस्तधमकाऩरयचमददमा(pichhle {..} hamle ke baad bharat ne jabardast dhairya ka parichay diya)C. Level IIIIn this level, normally translated sentence contains few content lexical units that are not proper translation across the language. Linking such news stories is a challenging task as news stories communicate same information but words may not have direct mapping. Such cases may fall under the category of low obfuscation or lexical paraphrasing.C.1. Localization Related IssuesIn this class cross language text reuse is dominated by localization related issues. It is observed that such types of usages are among the most common ones in new story text reuse. [23] has considered this class as a case of change of format. Date (12 (a)) currency (12 (b) and 12(c)) are the most common examples of this class (Table1). Date can be written in any applicable format in English and gives rise to many permutations andcombinations of Hindi translations formats. Likewise in natural language, currency can be expressed in users own ways and may not always conform to the dictionary equivalents. Like in 12 (b) “ten million” is translated in Hindi as “दसराख”which is wrong, and not as “एककयोड” when given to a Machine Translation system. But a person can intrerpret it as“एककयोड”or as transliterated version of its English counterpart.Many such examples are present in FIRE corpus. Some of the examples are shown in Table 1Table 1. Localization related issues in English-Hindi Cross LanguageText ReuseC.2. Partial Improper TranslationIn this class linguistically the situation is communicated as per the syntax of the respective language but if one tries to map the text reuse then few lexical units may not map due to partial improper translation (13 (a) & 13 (b) and 14 (a) & 14 (b)). “Crashed on him” will never map to “टकयागएथे”and “amended” does not mean “कटौती”.13 a). {..} door crashed on him13 b). वेदयवाजेसेटकयागएथे.(ve darwaze se takra gaye the)14 a). {..} Can be amended.14 b). {..} कटौतीहोसकतीह(katauti ho sakti hai)Automatic mapping for text reuse under this clas s is quite challenging. In 15(a) “boy” the actor is referred as “व्मक्तत”in 15(b) which is not proper. Table 2 shows some of the words present in the new stories that shall never mapped properly.15 a). {..}an affair with a boy from a different community15 b). {..}ककसीऔयबफयादयीकव्मक्ततसेप्रेभ{..} ({..} kisi aur biradari ke vyakti se prem{..})Table 2. Partial Improper TranslationC.3. OOV words substitutionsSocio-cultural influence across globe results in acceptability of some of the lexical units that are normally treated as Out of Vocabulary (OOV) for native language. In such cases although the Hindi equivalents of the words are available, but instead of taking exact word translations, transliterated words are accepted at the time of news reporting because such transliterated versions are more in use than the translation equivalents. Hindi has adopted many such words in its day to day writings and conversations but such words do not find any place in the dictionary as Hindi meanings of English words. These words also create problems if we go for Dictionary based approaches for mapping these words. Some of the words of FIRE corpus are shown in Table 3.Table 3. OOV words substitutionsC.4. Role and Thought ShiftingOne news may depict thought and news in other language depicts other‟s role. 16 (a) shows what a person is thinking about himself and the person‟s own decision but its Hindi equivalent 16(b) leaves the deci sion on others.16 a). Will consider PM job if we win16 b). साॊसद चाहेंगतबी फनगा प्रधानभॊत्री (saansad chahenge tabhi banunga pradhanmantri)C.5. Syntax/Discourse Structure Changes [4, 23] While translating 17(a) interjection is converted to assertion in 17 (b) along with same polarity substitution. Whereas 17(a) expresses surprise, 17 (b) asserts that it can never be true.17 a). Kapoor {..} he didn‟t believe that the ministerwas capable of harming her.17 b).साथउन्होंने{..} कऩयउनकीभाॉकोिायीरयकनकसाननहीॊऩहचासकतेथे(saath unhone{..} kapoor unki maa ko sharirik nuksaan nahin pahuncha sakte the)C.6. Contextual Related WordThe contextual related word may be used in place of exact translation. Let word be e1, its exact translation be h1 and contextual words related to E in H be h c1, h c2, h c3. The contextual words h c1, h c2, h c3 may be used in place of h1(18(a) & 18(b)). In a simpler way, these are those translations, where an English lexicon can be represented by any of its Hindi synonyms.18 a) I told them that the bill could be amended to address their concerns in respect of OBC and Muslim women.18 b) भैंनउनसेकहाहककओफीसीऔय अल्ऩसॊख्मकभदहराओकोरेकयउनकीजोधचॊताह,उसेखत्भकयनेकशरएबफरभेंसॊिोधनककमाजासकताह।(maine unse kaha hai ki OBC aur alpsankhyak mahilaon ko lekar unki jo chinta hai, use khatm karne ke liye bill mein sanshidhan kiya ja sakta hai)C.7. Transliteration of SynonymIn this class lexical mapping across the language is present but one uses transliterated synonym of lexical unit at the time of news reporting (19 and 20). The synonyms for hired and plea are contract and appeal respectively and these English synonyms only have been transliterated for using in the Hindi stories.19). hired killer कॉन्रतटककरय (contract killer)20). plea अऩीर (appeal)C.8. Abbreviation vs. PolysemyIn this class abbreviation is either transliterated or its expanded form is translated or transliterated. There can also be more than one translation equivalents of the same word and it is difficult to map these words across language (Table 4). Fujita [16] and Barron et al. [4] referred this class as “Altering notational variants, abbreviations, and acronyms” and “Lexicon based spelling and format changes” respectiv ely.Table 4. Abbreviation vs. PolysemyC.9. Sentimental Outburst to Add SensationIn this category some phrase, idioms and words arousing emotional outburst may be added across the language (21 (a) & 21 (b)). Here “साभदहकदष्कभज(saamuhik dushkarm)” is not the exact translation of rape but has simply been used to arouse sensation.21 a). 12 rape girl on panchayat order21 b). ऩॊचामतकआदेिऩय१२रोगोनेककमासाभदहकदष्कभज(panchayat ke aadesh par 12 logon ne kiya saamuhik dushkarm)D. Level IVTranslations falling under this category come under pragmatic paraphrasing which have been dealt by several researchers. As special types of paraphrases it goes beyond pure semantic similarity to fall within the field of pragmatics [24]. Paraphrasing extends to a group of words. Linking and tracking news stories under this class becomes quite challenging.D.1. Action vs. ConsequenceThis category has been referred to as Textual Entailment [1, 16, 24]. In this class meaning of one expression can be inferred from the other [10]. Newspaper may report action or decision taken in one language, but its translation in other language may report the consequences of the action or decision (22 (a) & 22(b))22 a). All pre-2005 notes go out of currency22 b). वाऩसकयनेहोंगे2005 सेऩहरेकसबीनोट(wapas karne honge 2005 se pahle ke sabhi note)D.2. Change in ReferenceIn this category referencing of time period may be changed across language (23 (a) & 23(b)).23 a). Anybody who has such notes can get these exchanged in any bank after April 1.23 b). {..}कभताबफक30 जनतककोईबीव्मक्ततककतनाबीनोटफैंकभेंजाकयफदरसकताह({..} ke mutabik 30 june tak koi bhi vyakti kitna bhi note bank mein jakar badal sakta hai)D.3. Focus ShiftingThe focus may be shifted at the time of translation while preserving the gist of the sentence. In 24(a) the focus is on reason but in 24 (b) it is on relation.24 a). Shyam Gupta dies of brain hemorrhage24 b). याभगप्ताकबाईश्माभगप्ताकीभौत(ram gupta ke bhai shyam gupta ki maut)D.4. Actor/Action Substitution [6]Action may be replaced by actor to highlight actor in news stories (25 (a) & 25 (b)).25 a). {..} admitted to hospital here.25 b). {..}शसयऔयऩयभेंभाभरीचोटआमी. उऩचायकफादवेिदटॊगऩयरौटबीआमे.({..} sir aur pair mein maamuli chot aayi. Upchaar ke baad ve shooting par laut bhi aaye)D.5. Specification vs. GeneralizationGroup of lexical units i.e. noun phrase in one language may be replaced by some other word or by anaphora ((26 (a) & 26 (b) and 27 (a) & 27 (b) and 28 (a) & 28 (b)) [11]. Use of hyponyms and hypernyms represent this category.26 a). {..} two-day national conclave at Karla26 b). ऩणकऩासऩाटीकदोददनकसम्भेरन{..} (pune ke paas party ke do din ke sammelan{..})27 a). A combination of Guptas has emerged to oppose the Bill27 b). अभोर, भनीषऔयतषायगप्तानेबफरकाववयोधककमाह(amol, manish aur tushar gupta ne bill ka virodh kiya hai)28 a). This has remained secret until now.28 b). दस्तावेजोंकइसतयहनष्टमागभहोजानेकायाजआजतकफनाहआथा।(dastavezon ke is tarah nasht ya gum ho jaane ka raaj aaj tak bana hua tha)D.6. Lexicon based Opposite Polarity Substitution Marta vila et al. [23, 24] and Barron et al. [4] referred this class as Lexicon based opposite polarity substitution but few other authors has referred it as Inter-clausal negative-affirmative paraphrasing [16]. In this class polarity may be changed twice. In this lexical unit is changed by its antonym or complementary and then in order to maintain the meaning, another change of polarity occurs within the same sentence (29 (a) & 29 (b)).29 a). {..} “too strong” to commit suicide29 b). {..} इतनीकभजोयनहीॊथीककख़दकिीजसाकदभउठारे.({..} itni kamzor nahin thi ki khudkushi jaisa kadam utha le)D.7. Referential [16] or cognitive [27]The cross language news stories may comprise of co reference that needs attention as it may be difficult to identify reuse (30 (a) & 30 (b) and 31 (a) & 31(b)). Referential and cognitive are to be treated as co reference rather than paraphrase but for retrieving news stories co-reference i.e. referential and cognitive might be quite useful [16, 23].30 a).{..} photograph addressing a meeting in February 201130 b). {..} तीनसारऩयानीतस्वीय {..}({..} teen saal purani tasveer {..})31 a). He was released on 14-day parole on August 2, 1999。