神经-符号系统
大脑的奥秘:神经科学导论-网课答案
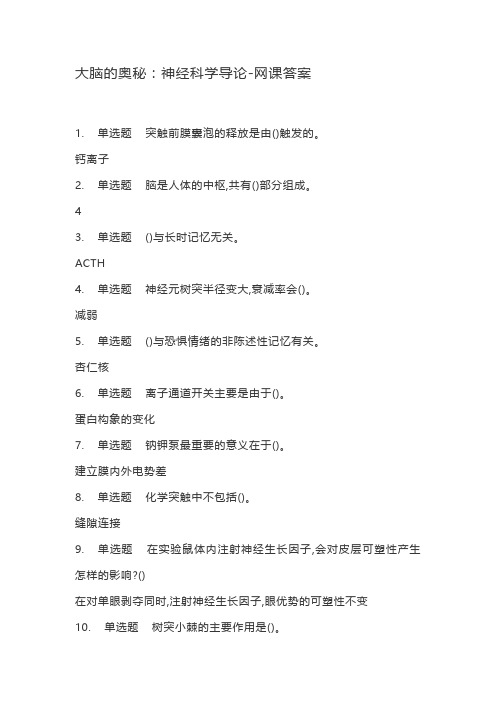
大脑的奥秘:神经科学导论-网课答案1. 单选题突触前膜囊泡的释放是由()触发的。
钙离子2. 单选题脑是人体的中枢,共有()部分组成。
43. 单选题 ()与长时记忆无关。
ACTH4. 单选题神经元树突半径变大,衰减率会()。
减弱5. 单选题 ()与恐惧情绪的非陈述性记忆有关。
杏仁核6. 单选题离子通道开关主要是由于()。
蛋白构象的变化7. 单选题钠钾泵最重要的意义在于()。
建立膜内外电势差8. 单选题化学突触中不包括()。
缝隙连接9. 单选题在实验鼠体内注射神经生长因子,会对皮层可塑性产生怎样的影响?()在对单眼剥夺同时,注射神经生长因子,眼优势的可塑性不变10. 单选题树突小棘的主要作用是()。
接受其它神经元的输入11. 单选题海兔的缩腮反射是研究习惯化极好的模型,缩腮反射习惯化产生的原因是()。
感觉神经元和运动神经元之间的突触发生了变化12. 单选题运动系统是由()种器官组成。
313. 单选题关于情景记忆,不正确的说法是()。
前额叶受损仅对老人的源头记忆有影响,而对小孩则不会产生影响14. 单选题以下需要意识介入的活动是()。
内心演练15. 单选题 LGN细胞的动作电位都可以表现为()种方式。
216. 单选题陈述性记忆的长时程增强效应(LTP)不正确的是()。
LTP是记忆改变的唯一神经机制17. 单选题在短时间内进行连续数学心算属于()。
工作记忆18. 单选题小脑皮层区域包括()层。
319. 单选题神经元的细胞体大小为()。
10微米20. 单选题当脑干受损时可能发生的现象是()。
咀嚼功能受阻21. 单选题突显的生命特征,是神经系统在()层次上产生认知、情绪、语言、意识的原因。
高级22. 单选题小动物在幼年关键期内将一只眼遮挡,几天时间内视觉皮层会发生()。
剥夺眼对应的神经元反应下降23. 单选题关于经典条件反射正确的是()。
经典条件反射包括延缓条件反射和痕迹条件反射24. 单选题运动系统共有()个功能。
基于神经符号系统的数学推理
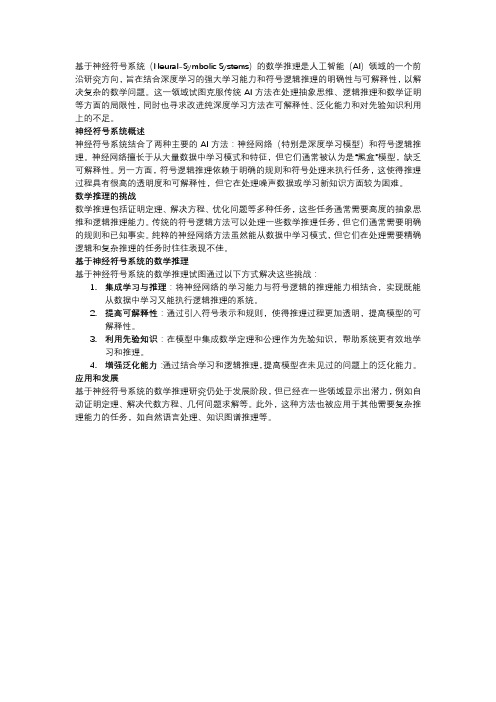
基于神经符号系统(Neural-Symbolic Systems)的数学推理是人工智能(AI)领域的一个前沿研究方向,旨在结合深度学习的强大学习能力和符号逻辑推理的明确性与可解释性,以解决复杂的数学问题。
这一领域试图克服传统AI方法在处理抽象思维、逻辑推理和数学证明等方面的局限性,同时也寻求改进纯深度学习方法在可解释性、泛化能力和对先验知识利用上的不足。
神经符号系统概述神经符号系统结合了两种主要的AI方法:神经网络(特别是深度学习模型)和符号逻辑推理。
神经网络擅长于从大量数据中学习模式和特征,但它们通常被认为是“黑盒”模型,缺乏可解释性。
另一方面,符号逻辑推理依赖于明确的规则和符号处理来执行任务,这使得推理过程具有很高的透明度和可解释性,但它在处理噪声数据或学习新知识方面较为困难。
数学推理的挑战数学推理包括证明定理、解决方程、优化问题等多种任务,这些任务通常需要高度的抽象思维和逻辑推理能力。
传统的符号逻辑方法可以处理一些数学推理任务,但它们通常需要明确的规则和已知事实。
纯粹的神经网络方法虽然能从数据中学习模式,但它们在处理需要精确逻辑和复杂推理的任务时往往表现不佳。
基于神经符号系统的数学推理基于神经符号系统的数学推理试图通过以下方式解决这些挑战:1.集成学习与推理:将神经网络的学习能力与符号逻辑的推理能力相结合,实现既能从数据中学习又能执行逻辑推理的系统。
2.提高可解释性:通过引入符号表示和规则,使得推理过程更加透明,提高模型的可解释性。
3.利用先验知识:在模型中集成数学定理和公理作为先验知识,帮助系统更有效地学习和推理。
4.增强泛化能力:通过结合学习和逻辑推理,提高模型在未见过的问题上的泛化能力。
应用和发展基于神经符号系统的数学推理研究仍处于发展阶段,但已经在一些领域显示出潜力,例如自动证明定理、解决代数方程、几何问题求解等。
此外,这种方法也被应用于其他需要复杂推理能力的任务,如自然语言处理、知识图谱推理等。
神经科学中的认知计算模型研究
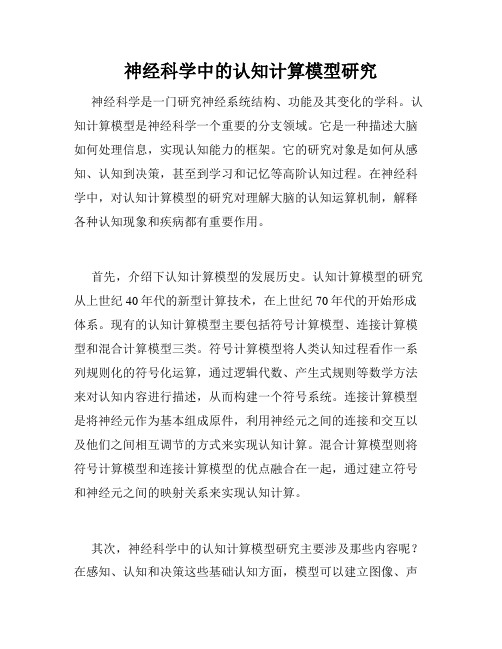
神经科学中的认知计算模型研究神经科学是一门研究神经系统结构、功能及其变化的学科。
认知计算模型是神经科学一个重要的分支领域。
它是一种描述大脑如何处理信息,实现认知能力的框架。
它的研究对象是如何从感知、认知到决策,甚至到学习和记忆等高阶认知过程。
在神经科学中,对认知计算模型的研究对理解大脑的认知运算机制,解释各种认知现象和疾病都有重要作用。
首先,介绍下认知计算模型的发展历史。
认知计算模型的研究从上世纪40年代的新型计算技术,在上世纪70年代的开始形成体系。
现有的认知计算模型主要包括符号计算模型、连接计算模型和混合计算模型三类。
符号计算模型将人类认知过程看作一系列规则化的符号化运算,通过逻辑代数、产生式规则等数学方法来对认知内容进行描述,从而构建一个符号系统。
连接计算模型是将神经元作为基本组成原件,利用神经元之间的连接和交互以及他们之间相互调节的方式来实现认知计算。
混合计算模型则将符号计算模型和连接计算模型的优点融合在一起,通过建立符号和神经元之间的映射关系来实现认知计算。
其次,神经科学中的认知计算模型研究主要涉及那些内容呢?在感知、认知和决策这些基础认知方面,模型可以建立图像、声音、语言等信息的形式化表示,探究基础认知过程如何抽象、处理信息,构建知识结构。
在学习和记忆等高阶认知方面,模型可研究人类的长期记忆、工作记忆等工作机制,阐释人类记忆被遗忘和重新学习的现象。
在疾病治疗方面,可利用认知计算模型来了解和治疗认知障碍、失语等疾病。
在人工智能领域,认知计算模型可以为智能机器人、循环神经网络等技术提供算法支持。
最后,介绍下认知计算模型研究的应用领域。
认知计算模型研究成果广泛应用于诸多领域。
在人机交互应用方面,可以通过用户行为与反馈信息对应的方式,提高人工智能的智能化水平,实现更为精准、自然、高效的人机交互。
在医疗信息学应用方面,可以扩展医疗域的知识和概念,支持临床决策和远程医疗。
在智能交通和智能制造等应用领域中,可以提高智能型设备的自主性和交互性,实现有物联网性质的智能交通和智能制造。
人脑认知体系结构及其计算模型
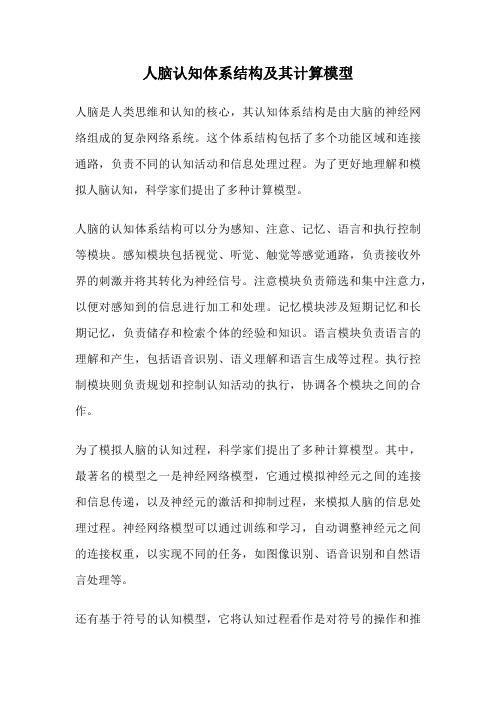
人脑认知体系结构及其计算模型人脑是人类思维和认知的核心,其认知体系结构是由大脑的神经网络组成的复杂网络系统。
这个体系结构包括了多个功能区域和连接通路,负责不同的认知活动和信息处理过程。
为了更好地理解和模拟人脑认知,科学家们提出了多种计算模型。
人脑的认知体系结构可以分为感知、注意、记忆、语言和执行控制等模块。
感知模块包括视觉、听觉、触觉等感觉通路,负责接收外界的刺激并将其转化为神经信号。
注意模块负责筛选和集中注意力,以便对感知到的信息进行加工和处理。
记忆模块涉及短期记忆和长期记忆,负责储存和检索个体的经验和知识。
语言模块负责语言的理解和产生,包括语音识别、语义理解和语言生成等过程。
执行控制模块则负责规划和控制认知活动的执行,协调各个模块之间的合作。
为了模拟人脑的认知过程,科学家们提出了多种计算模型。
其中,最著名的模型之一是神经网络模型,它通过模拟神经元之间的连接和信息传递,以及神经元的激活和抑制过程,来模拟人脑的信息处理过程。
神经网络模型可以通过训练和学习,自动调整神经元之间的连接权重,以实现不同的任务,如图像识别、语音识别和自然语言处理等。
还有基于符号的认知模型,它将认知过程看作是对符号的操作和推理。
在这种模型中,符号表示了不同的概念和知识,通过对符号的操作和推理,来模拟人脑的思维过程。
符号系统模型在逻辑推理、问题解决和专家系统等领域有广泛应用。
还有基于统计的认知模型,它通过统计分析大量的数据,来发现数据中的模式和规律。
这种模型主要应用于机器学习和数据挖掘等领域,可以实现自动分类、预测和决策等任务。
除了以上模型,还有许多其他的认知模型,如混合模型、连接主义模型和群体智能模型等。
这些模型从不同的角度和层次,对人脑的认知过程进行了建模和解释。
人脑的认知体系结构是由多个功能区域和连接通路组成的复杂网络系统,负责感知、注意、记忆、语言和执行控制等认知活动。
为了模拟和理解人脑的认知过程,科学家们提出了多种计算模型,如神经网络模型、符号系统模型和统计模型等。
人工智能的学科派别
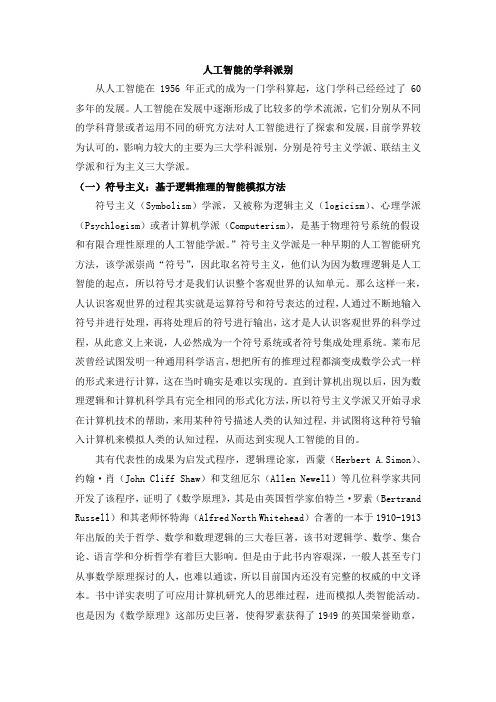
人工智能的学科派别从人工智能在1956年正式的成为一门学科算起,这门学科已经经过了60多年的发展。
人工智能在发展中逐渐形成了比较多的学术流派,它们分别从不同的学科背景或者运用不同的研究方法对人工智能进行了探索和发展,目前学界较为认可的,影响力较大的主要为三大学科派别,分别是符号主义学派、联结主义学派和行为主义三大学派。
(一)符号主义:基于逻辑推理的智能模拟方法符号主义(Symbolism)学派,又被称为逻辑主义(logicism)、心理学派(Psychlogism)或者计算机学派(Computerism),是基于物理符号系统的假设和有限合理性原理的人工智能学派。
”符号主义学派是一种早期的人工智能研究方法,该学派崇尚“符号”,因此取名符号主义,他们认为因为数理逻辑是人工智能的起点,所以符号才是我们认识整个客观世界的认知单元。
那么这样一来,人认识客观世界的过程其实就是运算符号和符号表达的过程,人通过不断地输入符号并进行处理,再将处理后的符号进行输出,这才是人认识客观世界的科学过程,从此意义上来说,人必然成为一个符号系统或者符号集成处理系统。
莱布尼茨曾经试图发明一种通用科学语言,想把所有的推理过程都演变成数学公式一样的形式来进行计算,这在当时确实是难以实现的。
直到计算机出现以后,因为数理逻辑和计算机科学具有完全相同的形式化方法,所以符号主义学派又开始寻求在计算机技术的帮助,来用某种符号描述人类的认知过程,并试图将这种符号输入计算机来模拟人类的认知过程,从而达到实现人工智能的目的。
其有代表性的成果为启发式程序,逻辑理论家,西蒙(Herbert A.Simon)、约翰·肖(John Cliff Shaw)和艾纽厄尔(Allen Newell)等几位科学家共同开发了该程序,证明了《数学原理》,其是由英国哲学家伯特兰·罗素(Bertrand Russell)和其老师怀特海(Alfred North Whitehead)合著的一本于1910-1913年出版的关于哲学、数学和数理逻辑的三大卷巨著,该书对逻辑学、数学、集合论、语言学和分析哲学有着巨大影响。
符号学的概念

符号学的概念什么是符号学符号学是一门研究符号系统的学科,它关注符号的构成、使用和意义。
符号学可以应用于语言学、心理学、社会学等多个领域,帮助人们理解符号系统在人类文化和认知中的重要作用。
符号的定义符号是一种代表或表达某种概念、概念群体或其他东西的记录或表征。
符号可以是文字、图像、声音或其他感官形式。
符号的使用基于共同的约定,即符号与所代表的对象之间存在一种公认的联系。
符号的三个要素符号包含三个要素:符号形式、符号内容和符号使用。
符号形式是符号的外在表现形式,比如文字的字母、图像的形状等。
符号内容是符号所代表的具体概念、对象或意义。
符号使用涉及到符号的生成、传播和解释。
符号的分类符号可以按照不同的维度进行分类。
常见的分类方式包括:1. 语言符号 vs. 非语言符号语言符号是通过语言系统进行表达的符号,包括文字、口语和手语等。
非语言符号则是通过图像、手势、音乐等非语言形式进行表达的符号。
2. 指示性符号 vs. 表示性符号指示性符号直接指向所代表的对象或概念,比如路牌指示前方的道路或地点。
表示性符号则是通过某种规定的约定与所代表的对象或概念相关联,比如红色在某些文化中代表危险或警示。
3. 存在性符号 vs. 表示性符号存在性符号是直接存在于所代表的对象上的符号,比如动物的外貌和行为特征。
表示性符号则是用来表达对象的符号,比如动物形象的图像或文字描述。
4. 表征符号 vs. 规约符号表征符号直接表示所代表的对象或概念的特征和属性,比如天平和正负号。
规约符号是一种约定俗成的符号系统,比如音符和文字字母,它们本身没有直接的表征意义,而是在符号系统中与其他符号形成关联从而表达意义。
符号学在社会科学中的应用符号学在社会科学中有着广泛的应用。
以下是一些符号学在不同领域中的具体应用示例:1. 文化研究符号学可以帮助研究者理解不同文化中的符号系统和其背后的意义。
通过研究符号在文化中的传播和解释方式,可以揭示出文化差异和共通性,帮助人们更好地理解不同文化之间的交流和互动。
神经学知识点

神经学知识点神经系统是人类身体中一个十分复杂而又神奇的系统,它控制着我们的感觉、运动、思维等各项生理活动。
神经学作为研究神经系统的学科,涉及到许多重要的知识点,下面将详细介绍一些常见的神经学知识点。
一、神经系统的组成神经系统包括中枢神经系统和周围神经系统两个部分。
中枢神经系统由大脑和脊髓组成,控制着身体的感觉和运动功能。
周围神经系统则由脑神经和脊神经组成,负责传递信息和指挥身体各部位的活动。
二、神经元的结构和功能神经元是神经系统的基本单位,它具有细胞体、轴突和树突等部分。
神经元的主要功能是传递神经冲动,通过突触将信号传递给其他神经元或靶器官,实现信息传递和神经调控的作用。
三、神经传导神经传导是指神经冲动在神经元内外传递的过程。
神经冲动在神经元内部的传导是通过神经元的轴突和树突进行的,而在神经元之间的传导则通过突触实现。
神经传导的快慢和稳定性对神经系统功能的正常运行至关重要。
四、神经调节神经调节是指神经系统对机体内部环境和外部刺激进行调节的过程。
通过神经元之间的连接和神经递质的释放,神经系统可以调节生理活动的速度和强度,维持机体内稳定的内环境。
五、神经系统疾病神经系统疾病是指神经系统发生的各种疾病和异常,常见的有中风、帕金森病、脑瘤等。
这些疾病会影响到神经系统的正常功能,导致感觉、运动、认知等方面的障碍。
结语神经学知识点涉及到神经系统的组成、神经元的结构和功能、神经传导、神经调节和神经系统疾病等多方面内容。
了解这些知识点不仅有助于我们更好地理解神经系统的运行机制,还能帮助我们更好地认识和预防神经系统疾病的发生。
希望以上内容能为您对神经学知识点有一个全面的了解。
多元智能理论之父-霍华德.加德纳PPT课件

主要内容
霍华德.加德纳近影 霍华德.加德纳的主要成就和声望 霍华德.加德纳成长与学术背景 霍华德.加德纳之前有关智能的研究 多元智能理论的诞生背景 多元智能理论简介 多元智能理论的进一步发展 多元智能理论对教育的启示 多元智能理论的评价 致谢
霍华德.加德纳近影
人类智能的标准。根据上述新的智能定义,加德纳提出了关 于智能结构的新理论──多元智能理论。这一理论认为,就 其基本结构来说,智能是多元的──不是一种能力而是一组 能力,而且,这组能力中的各种能力不是以整合的形式存在 而是以相对独立的形式存在。
从上述有关智能的概念可以看出,加德纳认为,智能是在某种社 会或文化环境的价值标准下,个体用以解决自己遇到的真正难题或生产 及创造出有效产品所需要的能力。通过对智能概念的分析,可以发现, 智能的本质可从以下几个方面进行理解:
➢ 智能的多元性 多元智能理论认为每一个个体有着相对独立的多种智能,这是 有其生物基础的。并且不同文化对需要解决的问题或制造的产品有着不同的 要求,而且解决实际问题和创造产品的能力不只局限于语言和逻辑能力,因 此不同文化下的不同个体在智能的发展方向和程度上存在差异,智能的表现 千差万别。
➢ 智能的发展性 与传统上认为智能是遗传因素决定的观点不同,加德纳认为
智能可以在任何年龄阶段发展,或任何能力层次的人都可以通过学习让自己 在各方面都变得很聪明。于是在《智能的结构》一书中,加德纳首次提出并 着重论述了多元智能理论的基本结构。加德纳认为,支撑多元智能理论的是 个体身上相对独立存在着的、与特定的认知领域或知识范畴相联系的八种智 能。
多元智能理论简介
为了能够达到他所提出的智能种类,加德纳和他的同事在几个领域内查阅了大量的文 献,即正常人的认知能力发展,在各种器官病变下的认知能力障碍,“特殊人群”,如神 童、孤独症儿童、白痴学者以及学习困难儿童等人群中能力的存在;在不同种类中存在 的智能形式,在不同文化中被珍视的智能形式;经过几千年的认知的进化,以及两种形式 的心理学证据,一种是对人类认知能力的因素分析研究的结果,另一种是对迁移和概括化 研究的结果。在这些迥然不同的文献中重复出现的候选能力才能组成暂时的智能,而在不 同文献中只出现一、二次的能力则不予考虑其作为候选智能。
人工智能三大流派
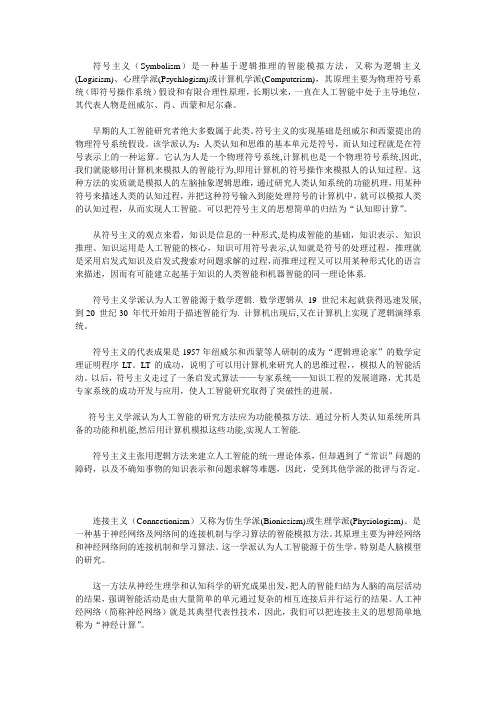
符号主义(Symbolism)是一种基于逻辑推理的智能模拟方法,又称为逻辑主义(Logicism)、心理学派(Psychlogism)或计算机学派(Computerism),其原理主要为物理符号系统(即符号操作系统)假设和有限合理性原理,长期以来,一直在人工智能中处于主导地位,其代表人物是纽威尔、肖、西蒙和尼尔森。
早期的人工智能研究者绝大多数属于此类。
符号主义的实现基础是纽威尔和西蒙提出的物理符号系统假设。
该学派认为:人类认知和思维的基本单元是符号,而认知过程就是在符号表示上的一种运算。
它认为人是一个物理符号系统,计算机也是一个物理符号系统,因此,我们就能够用计算机来模拟人的智能行为,即用计算机的符号操作来模拟人的认知过程。
这种方法的实质就是模拟人的左脑抽象逻辑思维,通过研究人类认知系统的功能机理,用某种符号来描述人类的认知过程,并把这种符号输入到能处理符号的计算机中,就可以模拟人类的认知过程,从而实现人工智能。
可以把符号主义的思想简单的归结为“认知即计算”。
从符号主义的观点来看,知识是信息的一种形式,是构成智能的基础,知识表示、知识推理、知识运用是人工智能的核心,知识可用符号表示,认知就是符号的处理过程,推理就是采用启发式知识及启发式搜索对问题求解的过程,而推理过程又可以用某种形式化的语言来描述,因而有可能建立起基于知识的人类智能和机器智能的同一理论体系.符号主义学派认为人工智能源于数学逻辑. 数学逻辑从19 世纪末起就获得迅速发展,到20 世纪30 年代开始用于描述智能行为. 计算机出现后,又在计算机上实现了逻辑演绎系统。
符号主义的代表成果是1957年纽威尔和西蒙等人研制的成为“逻辑理论家”的数学定理证明程序LT。
LT的成功,说明了可以用计算机来研究人的思维过程,,模拟人的智能活动。
以后,符号主义走过了一条启发式算法——专家系统——知识工程的发展道路,尤其是专家系统的成功开发与应用,使人工智能研究取得了突破性的进展。
认知语言学主要内容

认知语言学主要内容1内省法语言内省是指语言使用者对认知中凸现的语言的某些方面进行有意识的注意。
认知语言学在开创之初,为了构建该学派的理论框架,主要都采用的是内省法的思辨性研究,即研究者通过观察、内省、分析、推理手段等对语言现象形成规律性的认识。
内省研究方法可以追溯到古希腊时期。
柏拉图是纯粹的心智主义者。
他认为人类先天具有认知世界的能力,可用内省法进行逻辑推理,采用演绎法认识世界,并获得关于世界的知识。
但是,内省法研究语言存在一些天然的缺陷。
首先,主要参照既有的研究文献或语料进行研究,虽然该方法在逻辑上具有演绎的性质,但主观性太强;其次,不同实验主体用内省法得出的实验结果仅适用于解释特定的实验,缺乏普遍的解释力;最后,人类的某些认知功能无法被感觉经验所感知,等等。
2语料库法语料库法是用来记录自然发生的言语的一种方法。
它主要是对书写文本中的片段进行记录。
因此,语料库法的优势在于它可以给研究人员提供大量的文本信息。
从本质上看,语料库是研究者对于某一语言现象内省的验证。
它能利用计算机存储量大和检索迅速的优势。
近年来,该方法在认知隐喻理论、构式理论及词义理论等方面获得了广泛应用,为认知语言学的众多理论假设提供了实际的语料支撑,从使用频率上对语言现象的集中趋势进行验证。
语料库法的优点还反映在以下几个方面。
首先,语料库法可以通过频率充分反映某一个语言现象的特征,从而阐明该语言现象在人类心智过程中的无意识性。
这种无意识性往往很难通过有意识的内省法阐明出。
其次,可从语料分析上观察某一语言现象的历时演变过程。
另外,语料的频率统计数据还可以协助研究人员辨认出词语的共现特征。
而在这一点上其他研究方法,尤其就是内省法很难努力做到。
语料库法还能帮助研究人员发现那些与某一语词(表述)意义相近的表述。
这样,就会形成一个有关某情境的表达式集合,如同义词词典。
而内省法很难完成这样的任务,说明人的认知能力不具备这样的信息提取能力。
语料库法最小的弊端是它受到研究者个人主观推论影响。
第一章04神经系统
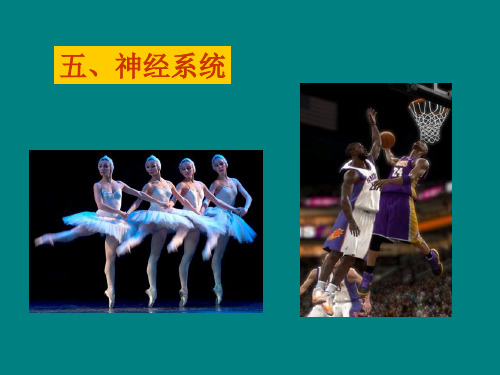
3、动力定型(习惯成自然)
大脑皮质的活动有它的规律的,若 一系列的刺激总是按照一定的时间、 顺序,先后出现,重复多次后,这种 顺序和时间就在大脑皮质上“固定” 下来,每到一定时间大脑就“知道” 下面该做什么了,提前做好准备。这 种大脑皮质活动的特性就叫动力定型。
如:作息制度习惯的形成,动物的驯化
4、保护性抑制—睡眠
异相睡眠中醒来,常发现自已在做梦。 会睡的孩子更聪明
(二)儿童神经系统的特点
1、神经系统发育迅速 (1)脑细胞数目的增长 妊娠三个月后,胎儿的神经系统已 基本形成。一岁前是脑细胞数目不再 增加,而后是由短变长,细胞像一棵 小树苗,逐渐长成枝繁叶茂的大树。
(2)脑重量的变化
出生350克 1岁950克 成人1500克 6岁1200克
大 脑,是脑与躯干、内脏之间的 联系通路
脊髓里的神经中枢,是受大脑控制的,人能有 意识地控制排便和排尿就是一个例证。 幼儿因大脑的发育尚未完善,对排尿的抑制能 力较弱,所以排尿次数多,而且容易发生夜间遗尿 现象。
3、周围神经系统
由脊髓发 出的神经叫脊 神经,分布在 躯干、四肢的 皮肤和肌肉里。 由脑发出的神 经叫脑神经, 大都分布到头 部的感觉器官、 皮肤、肌肉等 处。脑和脊髓 还有通向内脏 器官的神经
周围神经系统
脑神经:12对,来自脑部,主
要支配头部和颈部的各个器官
的感受和运动。 脊神经:31对,来自脊椎,主 要支配身体的颈部、四肢及内 脏的感觉和运动。分布在内脏 等处的自主神经分为交感神经
和副交感神经,两者为交互抑
制。
交感神经和副交感神经的比较
4、神经元
神经元即神经细胞,是神经系统结构和功能的 基本单位。
产生反应(肌肉收缩 或腺体分泌)
人工智能三大流派

符号主义(Symbolism)是一种基于逻辑推理的智能模拟方法,又称为逻辑主义(Logicism)、心理学派(Psychlogism)或计算机学派(Computerism),其原理主要为物理符号系统(即符号操作系统)假设和有限合理性原理,长期以来,一直在人工智能中处于主导地位,其代表人物是纽威尔、肖、西蒙和尼尔森。
早期的人工智能研究者绝大多数属于此类。
符号主义的实现基础是纽威尔和西蒙提出的物理符号系统假设。
该学派认为:人类认知和思维的基本单元是符号,而认知过程就是在符号表示上的一种运算。
它认为人是一个物理符号系统,计算机也是一个物理符号系统,因此,我们就能够用计算机来模拟人的智能行为,即用计算机的符号操作来模拟人的认知过程。
这种方法的实质就是模拟人的左脑抽象逻辑思维,通过研究人类认知系统的功能机理,用某种符号来描述人类的认知过程,并把这种符号输入到能处理符号的计算机中,就可以模拟人类的认知过程,从而实现人工智能。
可以把符号主义的思想简单的归结为“认知即计算”。
从符号主义的观点来看,知识是信息的一种形式,是构成智能的基础,知识表示、知识推理、知识运用是人工智能的核心,知识可用符号表示,认知就是符号的处理过程,推理就是采用启发式知识及启发式搜索对问题求解的过程,而推理过程又可以用某种形式化的语言来描述,因而有可能建立起基于知识的人类智能和机器智能的同一理论体系.符号主义学派认为人工智能源于数学逻辑. 数学逻辑从19 世纪末起就获得迅速发展,到20 世纪30 年代开始用于描述智能行为. 计算机出现后,又在计算机上实现了逻辑演绎系统。
符号主义的代表成果是1957年纽威尔和西蒙等人研制的成为“逻辑理论家”的数学定理证明程序LT。
LT的成功,说明了可以用计算机来研究人的思维过程,,模拟人的智能活动。
以后,符号主义走过了一条启发式算法——专家系统——知识工程的发展道路,尤其是专家系统的成功开发与应用,使人工智能研究取得了突破性的进展。
神经符号原理 system 1
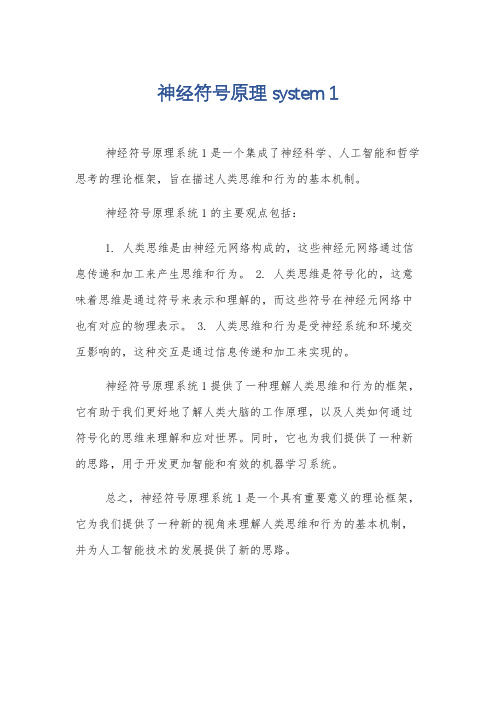
神经符号原理 system 1
神经符号原理系统1是一个集成了神经科学、人工智能和哲学思考的理论框架,旨在描述人类思维和行为的基本机制。
神经符号原理系统1的主要观点包括:
1. 人类思维是由神经元网络构成的,这些神经元网络通过信息传递和加工来产生思维和行为。
2. 人类思维是符号化的,这意味着思维是通过符号来表示和理解的,而这些符号在神经元网络中也有对应的物理表示。
3. 人类思维和行为是受神经系统和环境交互影响的,这种交互是通过信息传递和加工来实现的。
神经符号原理系统1提供了一种理解人类思维和行为的框架,它有助于我们更好地了解人类大脑的工作原理,以及人类如何通过符号化的思维来理解和应对世界。
同时,它也为我们提供了一种新的思路,用于开发更加智能和有效的机器学习系统。
总之,神经符号原理系统1是一个具有重要意义的理论框架,它为我们提供了一种新的视角来理解人类思维和行为的基本机制,并为人工智能技术的发展提供了新的思路。
卡西尔符号形式哲学探析
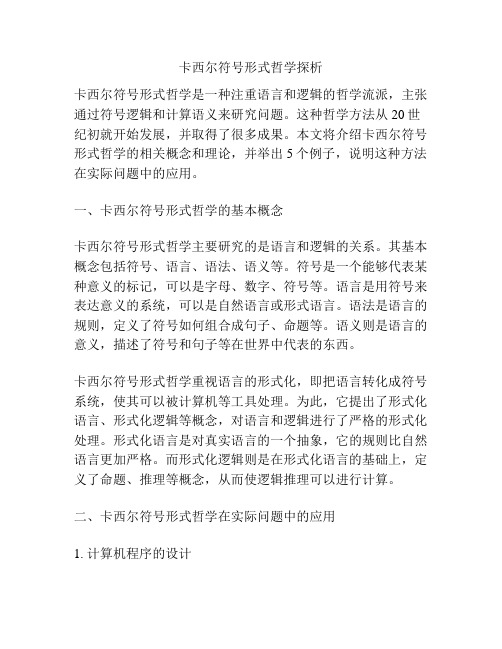
卡西尔符号形式哲学探析卡西尔符号形式哲学是一种注重语言和逻辑的哲学流派,主张通过符号逻辑和计算语义来研究问题。
这种哲学方法从20世纪初就开始发展,并取得了很多成果。
本文将介绍卡西尔符号形式哲学的相关概念和理论,并举出5个例子,说明这种方法在实际问题中的应用。
一、卡西尔符号形式哲学的基本概念卡西尔符号形式哲学主要研究的是语言和逻辑的关系。
其基本概念包括符号、语言、语法、语义等。
符号是一个能够代表某种意义的标记,可以是字母、数字、符号等。
语言是用符号来表达意义的系统,可以是自然语言或形式语言。
语法是语言的规则,定义了符号如何组合成句子、命题等。
语义则是语言的意义,描述了符号和句子等在世界中代表的东西。
卡西尔符号形式哲学重视语言的形式化,即把语言转化成符号系统,使其可以被计算机等工具处理。
为此,它提出了形式化语言、形式化逻辑等概念,对语言和逻辑进行了严格的形式化处理。
形式化语言是对真实语言的一个抽象,它的规则比自然语言更加严格。
而形式化逻辑则是在形式化语言的基础上,定义了命题、推理等概念,从而使逻辑推理可以进行计算。
二、卡西尔符号形式哲学在实际问题中的应用1. 计算机程序的设计计算机是一个符号处理系统,卡西尔符号形式哲学能够为计算机程序的设计提供基础。
例如,程序设计语言就是一种形式化语言,它利用符号来表达程序的逻辑、控制流等信息。
程序逻辑的正确性也可以用形式化逻辑来证明,避免了程序中的各种bug和错误。
2. 语言学研究语言学研究也是卡西尔符号形式哲学的应用领域之一。
它利用形式化语言和语义模型研究语言结构、语义关系等问题。
例如,人们可以将自然语言转换成形式化语言,然后利用其中的规则和算法分析语法和语义等问题。
3. 哲学知识表示卡西尔符号形式哲学也可以用于哲学知识表示和推理。
哲学中有很多概念、命题等需要进行精确的定义和推理,卡西尔符号形式哲学正是为这一目的而设计的。
通过形式化逻辑,哲学家可以更清晰地理解和表达概念,并推导出正确的结论。
神经符号方法

神经符号方法神经符号方法是一种将符号逻辑与神经科学相结合的计算模型,它可以用于处理语言、知识表示和推理等问题。
本文将介绍神经符号方法的基本原理、模型结构、应用领域以及未来发展方向。
一、基本原理1.1 符号逻辑符号逻辑是一种基于符号的推理系统,它使用形式化的语言描述命题和关系,并通过规则推导出新的命题和关系。
符号逻辑包括命题逻辑、谓词逻辑和模态逻辑等不同类型。
其中,命题逻辑主要研究命题之间的关系,谓词逻辑则研究对象之间的关系,而模态逻辑则研究命题在不同情境下的真值。
1.2 神经科学神经科学是研究神经系统结构和功能的学科,它涉及生物学、物理学、化学等多个领域。
神经科学主要研究大脑中神经元之间的信息传递和处理方式,包括突触传递、电信号传导等过程。
1.3 神经符号方法神经符号方法是将符号逻辑与神经科学相结合的计算模型,它基于符号逻辑的推理规则和神经元之间的信息传递方式,实现了符号和神经元之间的映射。
神经符号方法可以将符号逻辑中的命题和关系转化为神经元之间的连接,从而实现了对符号逻辑中命题和关系的处理。
二、模型结构2.1 神经网络神经网络是一种类似于大脑神经系统结构的计算模型,它由多个神经元组成,并通过连接权重进行信息传递。
神经网络可以分为前馈网络、循环网络、卷积网络等不同类型。
其中,前馈网络是最常见的一种类型,它由输入层、隐藏层和输出层组成。
2.2 神经符号网络神经符号网络是一种将符号逻辑与神经网络相结合的计算模型,它将符号逻辑中的命题和关系转化为神经元之间的连接权重,从而实现了对符号逻辑中命题和关系的处理。
神经符号网络包括输入层、隐藏层和输出层三个部分。
其中,输入层接收外部输入信号,并将其转化为一组向量表示;隐藏层通过连接权重实现了对符号逻辑中命题和关系的处理;输出层将隐藏层的结果转化为符号逻辑表示。
三、应用领域3.1 语言处理神经符号方法可以用于自然语言处理,包括文本分类、情感分析、机器翻译等任务。
幼儿高级神经活动的特点
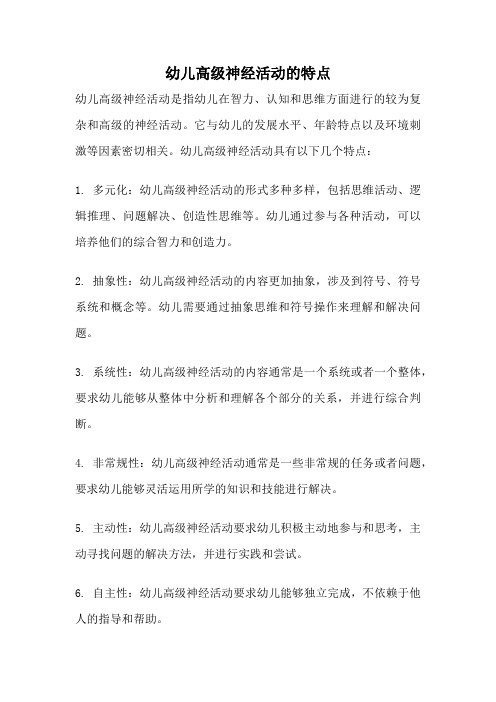
幼儿高级神经活动的特点幼儿高级神经活动是指幼儿在智力、认知和思维方面进行的较为复杂和高级的神经活动。
它与幼儿的发展水平、年龄特点以及环境刺激等因素密切相关。
幼儿高级神经活动具有以下几个特点:1. 多元化:幼儿高级神经活动的形式多种多样,包括思维活动、逻辑推理、问题解决、创造性思维等。
幼儿通过参与各种活动,可以培养他们的综合智力和创造力。
2. 抽象性:幼儿高级神经活动的内容更加抽象,涉及到符号、符号系统和概念等。
幼儿需要通过抽象思维和符号操作来理解和解决问题。
3. 系统性:幼儿高级神经活动的内容通常是一个系统或者一个整体,要求幼儿能够从整体中分析和理解各个部分的关系,并进行综合判断。
4. 非常规性:幼儿高级神经活动通常是一些非常规的任务或者问题,要求幼儿能够灵活运用所学的知识和技能进行解决。
5. 主动性:幼儿高级神经活动要求幼儿积极主动地参与和思考,主动寻找问题的解决方法,并进行实践和尝试。
6. 自主性:幼儿高级神经活动要求幼儿能够独立完成,不依赖于他人的指导和帮助。
7. 创造性:幼儿高级神经活动要求幼儿能够发挥自己的创造力,提出新颖的观点和解决问题的方法。
幼儿高级神经活动的特点与幼儿的认知发展密切相关。
幼儿的认知发展经历了从感知到运动、操作、形象思维到抽象思维的过程。
在幼儿的认知发展初期,他们主要通过感官和运动经验来认识世界,进行简单的认知活动。
随着年龄的增长,幼儿的认知能力逐渐发展,他们开始具备一定的逻辑思维能力和符号操作能力,能够进行更加复杂和高级的神经活动。
幼儿高级神经活动的特点也与幼儿的环境刺激密切相关。
幼儿在家庭和学校等环境中接受到丰富的刺激,这些刺激可以促进幼儿的认知和思维发展。
家长和教师可以通过提供丰富多样的学习材料和活动,激发幼儿的兴趣,引导他们进行高级神经活动。
幼儿高级神经活动是幼儿认知和思维发展的重要表现形式,具有多元化、抽象性、系统性、非常规性、主动性、自主性和创造性等特点。
认知科学和人工智能的发展
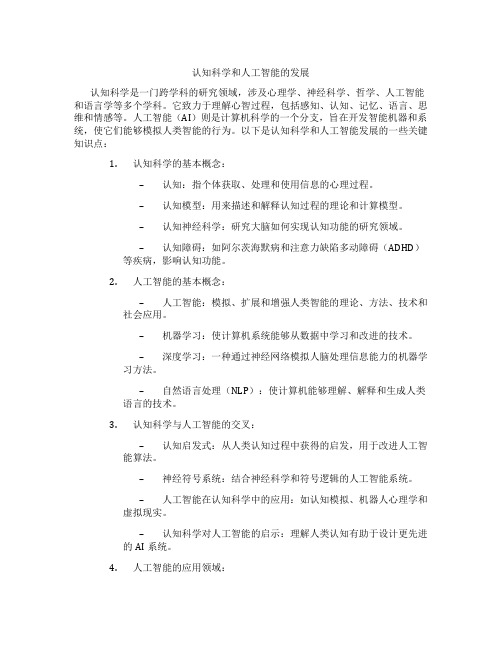
认知科学和人工智能的发展认知科学是一门跨学科的研究领域,涉及心理学、神经科学、哲学、人工智能和语言学等多个学科。
它致力于理解心智过程,包括感知、认知、记忆、语言、思维和情感等。
人工智能(AI)则是计算机科学的一个分支,旨在开发智能机器和系统,使它们能够模拟人类智能的行为。
以下是认知科学和人工智能发展的一些关键知识点:1.认知科学的基本概念:–认知:指个体获取、处理和使用信息的心理过程。
–认知模型:用来描述和解释认知过程的理论和计算模型。
–认知神经科学:研究大脑如何实现认知功能的研究领域。
–认知障碍:如阿尔茨海默病和注意力缺陷多动障碍(ADHD)等疾病,影响认知功能。
2.人工智能的基本概念:–人工智能:模拟、扩展和增强人类智能的理论、方法、技术和社会应用。
–机器学习:使计算机系统能够从数据中学习和改进的技术。
–深度学习:一种通过神经网络模拟人脑处理信息能力的机器学习方法。
–自然语言处理(NLP):使计算机能够理解、解释和生成人类语言的技术。
3.认知科学与人工智能的交叉:–认知启发式:从人类认知过程中获得的启发,用于改进人工智能算法。
–神经符号系统:结合神经科学和符号逻辑的人工智能系统。
–人工智能在认知科学中的应用:如认知模拟、机器人心理学和虚拟现实。
–认知科学对人工智能的启示:理解人类认知有助于设计更先进的AI系统。
4.人工智能的应用领域:–医疗:AI辅助诊断、个性化治疗计划和药物研发。
–教育:个性化学习、智能辅导系统和教育游戏。
–工业:自动化、智能制造和供应链优化。
–交通:自动驾驶汽车和智能交通管理系统。
5.人工智能的伦理和社会问题:–隐私和数据安全:AI系统处理个人数据时涉及的隐私保护问题。
–就业影响:AI技术对劳动力市场和职业结构的影响。
–偏见和公平性:确保AI系统决策过程的公正性和无偏见性。
–责任归属:当AI系统导致错误或伤害时,责任的归属问题。
6.人工智能的发展趋势:–增强人工智能:通过集成人类专家知识和经验来增强AI系统能力。
符号主义与联结主义的深度融合
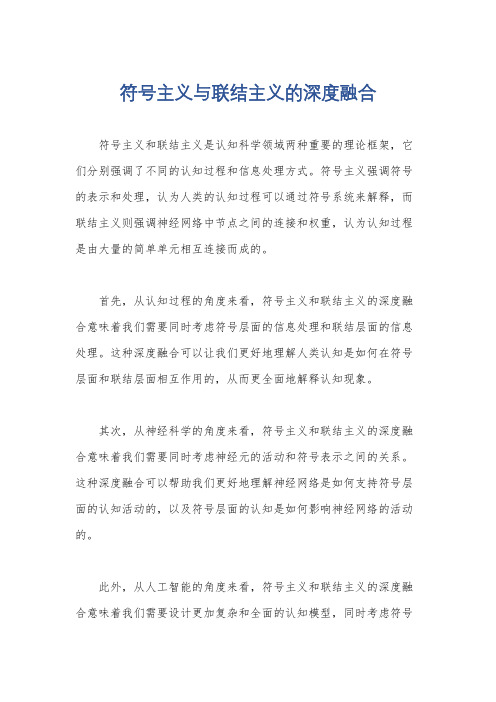
符号主义与联结主义的深度融合
符号主义和联结主义是认知科学领域两种重要的理论框架,它们分别强调了不同的认知过程和信息处理方式。
符号主义强调符号的表示和处理,认为人类的认知过程可以通过符号系统来解释,而联结主义则强调神经网络中节点之间的连接和权重,认为认知过程是由大量的简单单元相互连接而成的。
首先,从认知过程的角度来看,符号主义和联结主义的深度融合意味着我们需要同时考虑符号层面的信息处理和联结层面的信息处理。
这种深度融合可以让我们更好地理解人类认知是如何在符号层面和联结层面相互作用的,从而更全面地解释认知现象。
其次,从神经科学的角度来看,符号主义和联结主义的深度融合意味着我们需要同时考虑神经元的活动和符号表示之间的关系。
这种深度融合可以帮助我们更好地理解神经网络是如何支持符号层面的认知活动的,以及符号层面的认知是如何影响神经网络的活动的。
此外,从人工智能的角度来看,符号主义和联结主义的深度融合意味着我们需要设计更加复杂和全面的认知模型,同时考虑符号
处理和联结学习的机制。
这种深度融合可以帮助我们构建更加强大
和智能的人工智能系统,能够更好地模拟人类的认知能力。
总之,符号主义与联结主义的深度融合将为我们提供更加全面
和深入的认知科学理论框架,帮助我们更好地理解人类认知的本质,推动神经科学和人工智能领域的发展。
- 1、下载文档前请自行甄别文档内容的完整性,平台不提供额外的编辑、内容补充、找答案等附加服务。
- 2、"仅部分预览"的文档,不可在线预览部分如存在完整性等问题,可反馈申请退款(可完整预览的文档不适用该条件!)。
- 3、如文档侵犯您的权益,请联系客服反馈,我们会尽快为您处理(人工客服工作时间:9:00-18:30)。
60Garcez and Zaveruchaemployed in symbolic machine learning is tofind hy-potheses that are consistent with a background knowl-edge to explain a given set of examples.In general, these hypotheses are definitions of concepts described in some logical language.The examples are descrip-tions of instances and non-instances of the concept to be learned,and the background knowledge provides additional information about the examples and the con-cepts’domain knowledge[1].In contrast to symbolic learning systems,neural net-works’learning implicitly encodes patterns and their generalizations in the networks’weights,so reflect-ing the statistical properties of the trained data[5].It has been indicated that neural networks can outper-form symbolic learning systems,especially when data are noisy[3].This result,due also to the massively parallel architecture of neural networks,contributed decisively to the growing interest in combining,and possibly integrating,neural and symbolic learning sys-tems(see[6]for a clarifying treatment on the suitability of neural networks for the representation of symbolic knowledge).Pinkas[7,8]and Holldobler[9]have made important contributions to the subject of neural-symbolic integra-tion,showing the capabilities and limitations of neu-ral networks for performing logical inference.Pinkas defined a bi-directional mapping between symmetric neural networks and mathematical logic[10].He pre-sented a theorem showing a weak equivalence between the problem of satisfiability of propositional logic and minimizing energy functions;in the sense that for every well-formed formula(wff)a quadratic energy function can efficiently be found,and for every energy func-tion there exists a wff(inefficiently found),such that the global minima of the function are exactly equal to the satisfying models of the formula.Holldobler pre-sented a parallel unification algorithm and an auto-mated reasoning system forfirst order Horn clauses, implemented in a feedforward neural network,called the Connectionist Horn Clause Logic(CHCL)System. In[11],Holldobler and Kalinke presented a method for inserting propositional general logic programs[12] into three-layer feedforward artificial neural networks. They have shown that for each program P,there exists a three-layer feedforward neural network N with binary threshold units that computes T P,the program’sfixed point operator.If N is transformed into a recurrent network by linking the units in the output layer to the corresponding units in the input layer,it always settles down in a unique stable state when P is an acceptable program1[13,14].This stable state is the leastfixed point of T P,that is identical to the unique stable model of P,so providing a declarative semantics for P(see [15]for the stable model semantics of general logic programs).In[16],Towell and Shavlik presented KBANN (Knowledge-based Artificial Neural Network),a sys-tem for rules’insertion,refinement and extraction from neural networks.They have empirically shown that knowledge-based neural networks’training based on the backpropagation learning algorithm[17]is a very efficient way to learn from examples and back-ground knowledge.They have done so by comparing KBANN’s performance with other hybrid,neural and purely symbolic inductive learning systems’(see[1] and[18]for a comprehensive description of symbolic inductive learning systems,including Inductive Logic Programming).The Connectionist Inductive Learning and Logic Programming(C-IL2P)system is a massively parallel computational model based on a feedforward artificial neural network that integrates inductive learning from examples and background knowledge,with deductive learning from Logic Programming.Starting with the background knowledge represented by a propositional general logic program,a translation algorithm is ap-plied(see Fig.1(1))generating a neural network that can be trained with examples(2).Furthermore,that neural network computes the stable model of the pro-gram inserted in it or learned with the examples,so functioning as a massively parallel system for Logic Programming(3).The result of refining the background knowledge with the training examples can be explained by extracting a revised logic program from the network (4).Finally,the knowledge extracted can be more eas-ily analyzed by an expert that decides if it should feed the system once more,closing the learning cycle(5). The extraction step of C-IL2P(4)is beyond the scope of this paper,and the interested reader is referred to [19].In Section2,we present a new translation algorithm from general logic programs(P)to artificial neural networks(N)with bipolar semi-linear neurons.The algorithm is based on Holldobler and Kalinke’s trans-lation algorithm from general logic programs to neu-ral networks with binary threshold neurons[11].We also present a theorem showing that N computes the fixed-point operator(T P)of P.The theorem ensures that the translation algorithm is sound.In Section3, we show that the result obtained in[11]still holds,i.e.,Connectionist Inductive Learning and Logic61Figure1.The connectionist inductive learning and logic programming system.N is a massively parallel model for Logic Program-ming.However,N can also perform inductive learning from examples efficiently,assuming P as background knowledge and using the standard backpropagation learning algorithm as in[16].We outline the steps for performing both deduction and induction in the neu-ral network.In Section4,we successfully apply the system to two real-world problems of DNA classifi-cation,which have become benchmark data sets for testing machine learning systems’accuracy.We com-pare the results with other neural,symbolic,and hybrid inductive learning systems.Briefly,C-IL2P’s test-set performance is at least as good as KBANN’s and better than any other system investigated,while its training-set performance is considerably better than KBANN’s. In Section5,we conclude and discuss directions for future work.2.From Logic Programs to Neural NetworksIt has been suggested that the merging of theory(back-ground knowledge)and data learning(learning from examples)in neural networks may provide a more ef-fective learning system[16,20].In order to achieve this objective,one mightfirst translate the background knowledge into a neural network initial architecture, and then train it with examples using some neural learning algorithm like backpropagation.To do so,the C-IL2P system provides a translation algorithm from propositional(or grounded)general logic programs to feedforward neural networks with semi-linear neurons.A theorem then shows that the network obtained is equivalent to the original program,in the sense that what is computed by the program is computed by the network and vice-versa.Definition1.A general clause is a rule of the form A←L1,...,L k,where A is an atom and L i(1≤i≤k)is a literal(an atom or the negation of an atom).A general logic program is afinite set of general clauses.To insert the background knowledge,described by a general logic program(P),in the neural network(N), we use an approach similar to Holldobler and Kalinke’s [11].Each general clause(C l)of P is mapped from the input layer to the output layer of N through one neuron (N l)in the single hidden layer of N.Intuitively,the translation algorithm from P to N has to implement the following conditions:(1)The input potential of a hidden neuron(N l)can only exceed N l’s threshold (θl),activating N l,when all the positive antecedents of C l are assigned the truth-value“true”while all the negative antecedents of C l are assigned“false”;and(2) The input potential of an output neuron(A)can only exceed A’s threshold(θA),activating A,when at least one hidden neuron N l that is connected to A is activated.Example2.Consider the logic program P={A←B,C,not D;A←E,F;B←}.The translation al-gorithm should derive the network N of Fig.2,setting weights(W s)and thresholds(θ s)in such a way that conditions(1)and(2)above are satisfied.Note that, if N ought to be fully-connected,any other link(not shown in Fig.2)should receive weight zero initially.Note that,in Example2,we have labelled each input and output neuron as an atom appearing,respectively, in the body and in the head of a clause of P.This allows us to refer to neurons and propositional vari-ables interchangeably and to regard each network’s in-put vector i=(i1,...,i m)(i j(1≤j≤m)∈[−1,1])as62Garcez andZaveruchaFigure 2.Sketch of a neural network for the above logic prog-ram P .an interpretation for P .2If i j ∈[A min ,1]then the propositional variable associated to the jth neuron in the network’s input layer is assigned “true ”,while i j ∈[−1,−A min ]means that it is assigned “false ”,where A min ∈(0,1)is a predefined value as shown in the notation below.Note also that each hidden neuron N l corresponds to a clause C l of P .The following notation will be used in our translationalgorithm.Notation :Given a general logic program P ,let q de-note the number of clauses C l (1≤l ≤q )occurringin P ;m ,the number of literals occurring in P ;A min the minimum activation for a neuron to beconsidered “active”(or “true ”),0<A min <1;A max ,the maximum activation for a neuron to be con-sidered “not active”(or “false ”),−1<A max <0;h (x )=21+e −βx −1,the bipolar semi-linear activation function,where βis the steepness parameter (thatdefines the slope of h (x ));g (x )=x ,the standard linear activation function;W (resp.−W ),the weight of connections associatedwith positive (resp.negative)literals;θl ,the threshold of hidden neuron N l associated withclause C l ;θA ,the threshold of output neuron A ,where A is thehead of clause C l ;k l ,the number of literals in the body of clause C l ;p l ,the number of positive literals in the body ofclause C l ;n l ,the number of negative literals in the body of clause C l ;µl ,the number of clauses in P with the same atom in the head for each clause C l ;max C l (k l ,µl ),the greater element among k l and µl for clause C l ,max P (k 1,...,k q ,µ1,...,µq ),the greatest element among all k ’s and µ’s of P .For instance,for the program P of Example 2,q =3,m =6,k 1=3,k 2=2,k 3=0,p 1=2,p 2=2,p 3=0,n 1=1,n 2=0,n 3=0,µ1=2,µ2=2,µ3=1,max C 1(k 1,µ1)=3,max C 2(k 2,µ2)=2,max C 3(k 3,µ3)=1,and max P (k 1,k 2,k 3,µ1,µ2,µ3)=3.In the translation algorithm below,we define A min =ξ1(k ,µ),W =ξ2(h (x ),k ,µ,A min ),θl =ξ3(k ,A min ,W ),and θA =ξ4(µ,A min ,W )such that condi-tions (1)and (2)are satisfied,as we will see later in the proof of Theorem 3.Given a general logic program P ,consider that the literals of P are numbered from 1to m such that the input and output layers of N are vectors of maximum length m ,where the i th neuron represents the i th lite-ral of P .Assume,for mathematical convenience and without loss of generality,that A max =−A min .1.Calculate max P (k 1,...,k q ,µ1,...,µq )of P ;2.Calculate A min >max P (k 1,...,k q ,µ1,...,µq )−1max P (k 1,...,k q ,µ1,...,µq )+1;3.Calculate W ≥2β·ln (1+A min )−ln (1−A min )max P (k 1,...,k q ,µ1,...,µq )(A min −1)+A min +1;4.For each clause C l of P of the form A ←L 1,...,L k (k ≥0):(a)Add a neuron N l to the hidden layer of N ;(b)Connect each neuron L i (1≤i ≤k )in the input layer to the neuron N l in the hidden layer.If L i is a positive literal then set the connec-tion weight to W ;otherwise,set the connection weight to −W ;(c)Connect the neuron N l in the hidden layer to the neuron A in the output layer and set the connection weight to W ;(d)Define the threshold (θl )of the neuron N l in the hidden layer as θl =(1+A min )(k l −1)W (e)Define the threshold (θA )of the neuron A in the output layer as θA =(1+A min )(1−µl )W .5.Set g (x )as the activation function of the neurons in the input layer of N .In this way,the activation of the neurons in the input layer of N ,given by each input vector i ,will represent an interpretation for P .Connectionist Inductive Learning and Logic636.Set h(x)as the activation function of the neurons inthe hidden and output layers of N.In this way,agradient descent learning algorithm,such as back-propagation,can be applied on N efficiently.7.If N ought to be fully-connected,set all other con-nections to zero.Since N contains a bipolar semi-linear(differen-tiable)activation function h(x),instead of a binarythreshold non-linear activation function,the network’soutput neurons activations are real numbers in the range[−1,1].Therefore,we say that an output within therange[A min,1]represents the truth-value“true”,while an output within[−1,−A min]represents“false”.Wewill see later in the proof of Theorem3that the abovedefined weights and thresholds do not allow the net-work to present activations in the range(−A min,A min). Note that the translation of facts of P into N,for instance B←in Example2,is done by simply tak-ing k=0in the above algorithm.Alternatively,each fact of the form A←may be converted to a rule of the form A←T that is inserted in N using k=1, where T denotes“true”,and is an extra neuron that is always active in the input layer of N,i.e.,T has input datafixed at“1”.From the point of view of the computation of P by N,there is absolutely no differ-ence between the above two ways of inserting facts of P into N.However,considering the subsequent pro-cess of inductive learning,regarding P as background knowledge,if A←is inserted in N then the set of examples to be learned afterwards can defeat that fact by changing weights and/or establishing new connec-tions in N.On the other hand,if A←T is inserted in N then A can not be defeated by the set of examples since the neuron T is clamped in N.Defeasible and nondefeasible knowledge can therefore be respectively inserted in the network by defining variable andfixed weights.The above translation algorithm is based upon theone presented in[11],where N is defined with bi-nary threshold neurons.It is known that such networkshave limited ability to learn.Here,in order to performinductive learning efficiently,N is defined using theactivation function h(x).An immediate result is that N can also perform inductive learning from examples and background knowledge as in[16].Moreover,the restriction imposed over W in[11],where it is shown that N computes T P for W=1,is weakened here, since the weights must be able to change during train-ing.Nevertheless,in[16],and more clearly in[21],thebackground knowledge must have a“sufficiently small”number of rules as well as a“sufficiently small”numberof antecedents in each rule3in order to be accuratelyencoded in the neural network.Unfortunately,theserestrictions become quite strong or even unfeasible if,for instance,A max=1as in([16],Section5:Empiri-cal Tests of KBANN).Consequently,an interpretationthat does not satisfy a clause can wrongly activate aneuron in the output layer of N.This results fromthe use of the standard(unipolar)semi-linear activa-tion function,where each neuron’s activation is in therange[0,1].Hence,in[16]both“false”and“true”arerepresented by positive numbers in the ranges[0,A max]and[A min,1]respectively.For example,if A min=0.7 and k=2,an interpretation that assigns“false”to positive literals in the input layer of N can generate a positive input potential greater than the hidden neu-ron’s threshold,wrongly activating the neuron in the output layer of N.In order to solve this problem we use bipolar ac-tivation functions,where each neuron’s activation isin the range[−1,1].Now,an interpretation that doesnot satisfy a clause contributes negatively to the hiddenneuron’s input potential,since“false”is represented bya number in[−1,−A min],while an interpretation thatdoes satisfy a clause contributes positively to the in-put potential,because“true”is in[A min,1].Theorem3 will show that the choice of a bipolar activation function is sufficient to solve the above problem.Furthermore, the choice of−1instead of zero to represent“false”will lead to faster convergence in almost all cases.The rea-son is that the update of a weight connected to an input variable will be zero when the corresponding variable is zero in the training pattern[5,22].Thus making use of bipolar semi-linear activationfunction h(x),let us see how we have obtained thevalues for the hidden and output neurons’thresholds θl andθA.To confer symmetric mathematical results, without loss of generality,assume that A max=−A min. From the input to the hidden layer of N(L1,...,L k⇒N l),if an interpretation satisfies L1,...,L k then the contribution of L1,...,L k to the input potential of N l is greater than I+=k A min W.If,conversely,an interpre-tation does not satisfy L1,...,L k then the contribution of L1,...,L k to the input potential of N l is smaller than I−=(p−1)W−A min W+nW.Therefore,we define θl=I++I−2=(1+A min)(k−1)2W(translation algorithm,step4d).From the hidden to the output layer of N(N l⇒A),if an interpretation satisfies N l then the64Garcez and Zaveruchacontribution of Nl to the input potential of A is greaterthan I +=A min W −(µ−1)W .If,conversely,aninterpretation does not satisfy N l then the contribu-tion of N l to the input potential of A is smallerthan I −=−µA min W .Similarly,we define θA =I ++I −=(1+A min )(1−µ)2W (translation algorithm,step 4e).Obvi-ously,I +>I −should be satisfied in both cases above.Therefore,A min >k l −1l +1and A min >µl −1µl +1must ified and,more generally,the condition imposed overA min in the translation algorithm (step 2).Finally,givenA min ,the value of W (translation algorithm (step 3))re-sults from the proof of Theorem 3below.In what follows,we show that the theorem presentedin [11],where N with binary threshold neurons com-putes the fixed point operator T P of the program P ,still holds for N with semi-linear neurons.The fol-lowing theorem ensures that our translation algorithmis sound.The function TP mapping interpretations tointerpretations is defined as follows.Let i be an inter-pretation and A an atom.TP (i )(A )=“true ”iff thereexists A ←L 1,...,L k in P s .t . k i =1i (L i )=“true ”.Theorem 3.For each propositional general logicprogram P ,there exists a feedforward artificial neuralnetwork N with exactly one hidden layer and semi-linear neurons ,obtained by the above “TranslationAlgorithm ”,such that N computes T P .Proof:We have to show that there exists W >0suchthat N computes TP .In order to do so we need to provethat given an input vector i ,each neuron A in the outputlayer of N is “active”if and only if there exists a clauseof P of the form A ←L 1,...,L k s.t.L 1,...,L k aresatisfied by interpretation i .The proof takes advantageof the monotonically non-decreasing property of thebipolar semi-linear activation function h (x ),which al-lows the analysis to focus on the boundary cases.Asbefore,we assume that A max =−A min without loss ofgenerality.(←)A ≥A min if L 1,...,L k is satisfied by i .As-sume that the p positive literals in L 1,...,L k are“true”,while the n negative literals in L 1,...,L k are“false”.Consider the mapping from the input layerto the hidden layer of N .The input potential (I l )ofN l is minimum when all the neurons associated with apositive literal in L 1,...,L k are at A min ,while all the neurons associated with a negative literal in L 1,...,L kare at −A min .Thus,I l ≥p A min W +n A min W −θland assuming θl =(1+A min )(k −1)2W ,I l ≥p A min W+n A min W −(1+A min )(k −1)2W .If h (I l )≥A min ,i.e.,I l ≥−1βln (1−A min min ),then N l is active.Therefore,Eq.(1)must be satisfied.p A min W +n A min W −(1+A min )(k −1)2W ≥−1βln 1−A min 1+A min (1)Solving Eq.(1)for the connection weight (W )yields Eqs.(2)and (3),given that W >0.W ≥−2β·ln (1−A min )−ln (1+A min )k (A min −1)+A min +1(2)A min >k −1k +1(3)Consider now the mapping from the hidden layer to the output layer of N .By Eqs.(2)and (3)at least one neuron N l that is connected to A is “active”.The input potential (I l )of A is minimum when N l is at A min ,while the other µ−1neurons connected to A are at −1.Thus,I l ≥A min W −(µ−1)W −θl and assuming θl =(1+A min )(1−µ)2W ,I l ≥A min W −(µ−1)W −(1+A min )(1−µ)W .If h (I l )≥A min ,i.e.,I l ≥−1βln (1−A min 1+A min ),then A is active.Therefore,Eq.(4)must be satisfied.A min W −(µ−1)W −(1+A min )(1−µ)2W ≥−1βln 1−A min 1+A min (4)Solving Eq.(4)for the connection weight W yields Eqs.(5)and (6),given that W >0.W ≥−2β·ln (1−A min )−ln (1+A min )µ(A min −1)+A min +1(5)A min >µ−1µ+1(6)(→)A ≤−A min if L 1,...,L k is not satisfied by i .Assume that at least one of the p positive literals in L 1,...,L k is “false”or one of the n negative literals in L 1,...,L k is “true”.Consider the mapping from the input layer to the hidden layer of N .The input potential (I l )of N l is maximum when only one neuron associ-ated to a positive literal in L 1,...,L k is at −A min or when only one neuron associated to a negative literal in L 1,...,L k is at A min .Thus,I l ≤(p −1)W −A min W +nW −θl or I l ≤(n −1)W −A min W +pW阈值只是区分了两种情况的界限丆至于输出的是多大电势还取决于输出函数Connectionist Inductive Learning and Logic 65−θl ,respectively,and assumingθl =(1+A min )(k −1)2W ,I l ≤(k −1−A min )W −(1+A min )(k −1)2W .If −A min ≥h (I l ),i.e.,−A min ≥2−β(I l )−1,then I l ≤−1βln (1+A min 1−A min ),and so N l is not active.Therefore,Eq.(7)must be satisfied.(k −1−A min )W −(1+A min )(k −1)2W ≤−1ln 1−A min 1+A min(7)Solving Eq.(7)for the connection weight W yields Eqs.(8)and (9),given that W >0.W ≥2·ln (1+A min )−ln (1−A min )k (A min min (8)A min >k −1k +1(9)Consider now the mapping from the hidden layer to the output layer of N .By Eqs.(8)and (9),all neu-rons N l that are connected to A are “not active”.The input potential (I l )of A is maximum when all the neurons connected to A are at −Amin .Thus,I l ≤−µA min W −θl andassuming θl =(1+A min)(1−µ)2W ,I l ≤−µA min W −(1+A min )(1−µ)2W .If −A min ≥h (I l ),i.e.,−A min ≥2−β(Il )−1,then I l ≤−1βln (1+A minmin ),and so A is not active.Therefore,Eq.(10)must be satisfied.−µA min W −(1+A min )(1−µ)2W ≤−1ln 1+A min1−A min(10)Solving Eq.(10)for the connection weight W yields Eqs.(11)and (12),given that W >0.W ≥2β·ln (1+A min )−ln (1−A min )µ(A min −1)+A min +1(11)A min >µ−1(12)Notice that Eqs.(2)and (5)are equivalent to Eqs.(8)and (11),respectively.Hence,the above theorem holds if for each clause C l in P Eqs.(2)and (3)are satisfied by W and A min from the input to the hidden layer of N ,while Eqs.(5)and (6)are satisfied by W and A min from the hidden to the output layer of N .In order to unify the weights in N for each clause C l of P given the definition of max C l (k l ,µl ),it is sufficient that Eqs.(13)and (14)below are satisfied by W and A min ,respectively.W ≥2β·ln (1+A min )−ln (1−A min )max C l (k l ,µl )(A min −1)+A min +1(13)A min >max C l (k l ,µl )−1max C l (k l ,µl )+1(14)Finally,in order to unify all the weights in N for a program P given the definition of max P (k 1,...,k q ,µ1,...,µq ),it is sufficient that Eqs.(15)and (16)are satisfied by W and A min ,res-pectively.W ≥2β·ln (1+A min )−ln (1−A min )max P (k 1,...,k q ,µ1,...,µq )(A min −1)+A min +1(15)A min >max P (k 1,...,k q ,µ1,...,µq )−1max P (k 1,...,k q ,µ1,...,µq )+1(16)As a result,if Eqs.(15)and (16)are satisfied by W and A min ,respectively,then N computes T P .2Example 4.Consider the program P ={A ←B ,C ,not D ;A ←E ,F ;B ←}.Converting fact B ←to rule B ←T and applying the Translation Algorithm ,we obtain the neural network N of Fig.3.Firstly,we Figure 3.The neural network N obtained by the translation overP .Connections with weight zero are not shown.66Garcez and Zaveruchacalculate max P(k1,...,k n,µ1,...,µn)=3(step1), and A min>0.5(step2).Then,suppose A min=0.6, we obtain W≥6.931/β(step3).Alternatively,sup-pose A min=0.7,then W≥4.336/β.Let us take A min=0.7and h(x)as the standard bipolar semi-linear activation function(β=1),then if W=4.5,N computes the operator T P of P.4In the above example,the neuron B appears at both the input and the output layers of N.This indicates that there are at least two clauses of P that are link-ed through B(in the example:A←B,C,not D and B←),defining a dependency chain[23].We represent that chain in the network using the recurrent connec-tion W r=1to denote that the output of B must feed the input of B in the next learning or recall step.In this way,regardless of the length of the dependency chains in P,N always contains a single hidden lay-er,thus obtaining a better learning performance.5We will explain in detail the use of recurrent connections in Section3.In Section4we will compare the learning results of C-IL2P with KBANN’s,where the number of hidden layers is equal to the length of the greatest dependency chain in the background knowledge.Remark1.Analogously to[11],for any logic pro-gram P,the time needed to compute T P(i)in the network is constant;equal to two time steps(one to compute the activations from the input to the hidden neurons and another from the hidden to the output neu-rons).A parallel computational model requiring p(n) processors and t(n)time to solve a problem of size n is optimal if p(n)×t(n)=O(T(n)),where T(n) is the best sequential time to solve the problem[24]. The number of neurons and connections in the network that corresponds to a program P is given respectively by O(q+r)and O(q·r),where q is the number of clauses and r is the number of propositional vari-ables(atoms)occurring in P.The sequential time to compute T P(i)is bound to O(q·r),and so the above parallel computational model is optimal.3.Massively Parallel Deductionand Inductive LearningThe neural network N can perform deduction and in-duction.In order to perform deduction,N is trans-formed into a partially recurrent network N r by connecting each neuron in the output layer to its corres-pondent neuron in the input layer with weight W r=1,Figure4.The recurrent neural network N r.as shown in Fig.4.In this way,N r is used to iterate T Pin parallel,because its output vector becomes its inputvector in the next computation of T P.Let us now show that as in[11],if P is an accept-able program then N r always settles down in a stablestate that yields the uniquefixed point of T P,since N rcomputes the upward powers(T m P(i))of T P.A simi-lar result could also be easily proved for the class oflocally stratified programs(see[12]).Definition5[13,23].Let B P denote the Herbrandbase of P,i.e.,the set of propositional variables(atoms)occurring in P.A level mapping for a program P is afunction||:B P→ℵof ground atoms to natural num-bers.For A∈B P,|A|is called the level of A and |not A|=|A|.Definition6[13,23].Let P be a program,||a level mapping for P,and i a model of P.P is called accept-able w.r.t||and i if for every clause A←L1,...,L k in P the following implication holds for1≤i≤k:if i|=i−1j=1L j then|A|>|L j|.Theorem7[14].For each acceptable general pro-gram P,the T P has a uniquefixed-point.The sequence of all T m P(i),m∈ℵ,converges to thisfixed-point T P(i)(which is identical to the stable model of P[15]),for each i⊆B P.Recall that,since N r has semi-linear neurons,for each real value o i in the output vector(o)of N r,if o i≥A min then the corresponding i th atom in P is。