A survey of techniques for Face Reconstruction
关于人脸识别的英语阅读理解
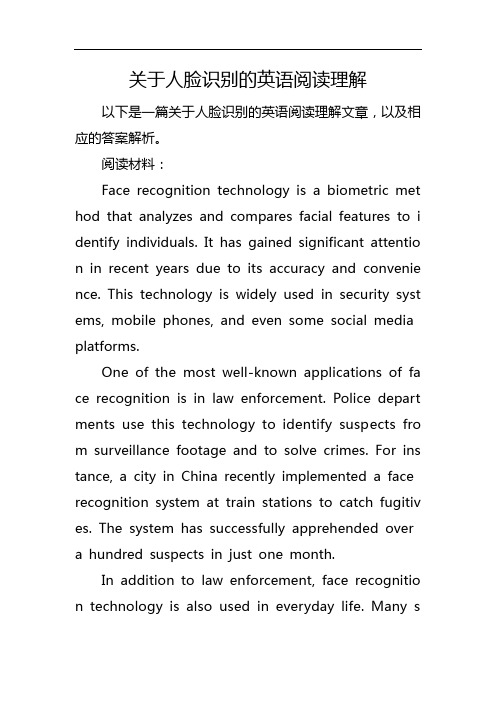
关于人脸识别的英语阅读理解以下是一篇关于人脸识别的英语阅读理解文章,以及相应的答案解析。
阅读材料:Face recognition technology is a biometric met hod that analyzes and compares facial features to i dentify individuals. It has gained significant attentio n in recent years due to its accuracy and convenie nce. This technology is widely used in security syst ems, mobile phones, and even some social media platforms.One of the most well-known applications of fa ce recognition is in law enforcement. Police depart ments use this technology to identify suspects fro m surveillance footage and to solve crimes. For ins tance, a city in China recently implemented a face recognition system at train stations to catch fugitiv es. The system has successfully apprehended over a hundred suspects in just one month.In addition to law enforcement, face recognitio n technology is also used in everyday life. Many smartphones now come with facial recognition soft ware, allowing users to unlock their phones simply by looking at them. This feature adds an extra lay er of security to the device.However, face recognition technology is not wi thout its challenges. Privacy concerns have been ra ised, as people worry about their personal informa tion being stored and used without their consent. There are also concerns about the accuracy of the technology, as it can sometimes mistake one perso n for another.Despite these challenges, face recognition tech nology continues to improve and expand its applic ations. It is now being used in airports, hotels, and even some retail stores. As the technology becom es more advanced, it is likely to play an even grea ter role in our lives.问题与答案解析:1. What is face recognition technology?Answer: Face recognition technology is a biom etric method that analyzes and compares facial fea tures to identify individuals.2. How is face recognition technology used in law enforcement?Answer: Police departments use face recognitio n technology to identify suspects from surveillance footage and to solve crimes.3. What are some everyday applications of face recognition technology?Answer: Everyday applications of face recogniti on technology include using it to unlock smartpho nes and improve security.4. What are some concerns about face recogni tion technology?Answer: Privacy concerns and accuracy issues a re two main concerns about face recognition techn ology.5. Despite the challenges, what is the future of face recognition technology likely to be?Answer: The future of face recognition technol ogy is expected to see continued improvement an d expansion of its applications.通过阅读这篇文章,读者可以了解到人脸识别技术的定义、应用领域、面临的挑战以及未来的发展趋势。
高三英语学术研究方法创新不断探索单选题30题

高三英语学术研究方法创新不断探索单选题30题1. In academic research, a hypothesis is a ______ that is tested through experiments and observations.A. predictionB. conclusionC. theoryD. assumption答案:D。
本题考查学术研究中“假说”相关的基本概念。
选项A“prediction”意为“预测”,通常是基于现有信息对未来的估计;选项B“conclusion”指“结论”,是在研究后得出的最终判断;选项C“theory”是“理论”,是经过大量研究和验证形成的体系;选项D“assumption”表示“假定、设想”,更符合“假说”的含义,即在研究初期未经充分验证的设想。
2. The main purpose of conducting academic research is to ______ new knowledge and understanding.A. discoverB. createC. inventD. produce答案:A。
此题考查学术研究目的相关的词汇。
选项A“discover”意思是“发现”,强调找到原本存在但未被知晓的事物;选项B“create”意为“创造”,侧重于从无到有地造出新的东西;选项C“invent”指“发明”,通常指创造出新的工具、设备等;选项D“produce”有“生产、产生”的意思,比较宽泛。
在学术研究中,主要是“发现”新知识和理解,所以选A。
3. A reliable academic research should be based on ______ data and methods.A. accurateB. preciseC. correctD. valid答案:D。
本题关于可靠学术研究的基础。
选项A“accurate”侧重于“准确无误”,强调与事实完全相符;选项B“precise”意为“精确的、明确的”,更强调细节的清晰和明确;选项C“correct”指“正确的”;选项D“valid”表示“有效的、有根据的”,强调数据和方法具有合理性和可靠性。
高三英语艺术批评方法科学严谨运用单选题30题
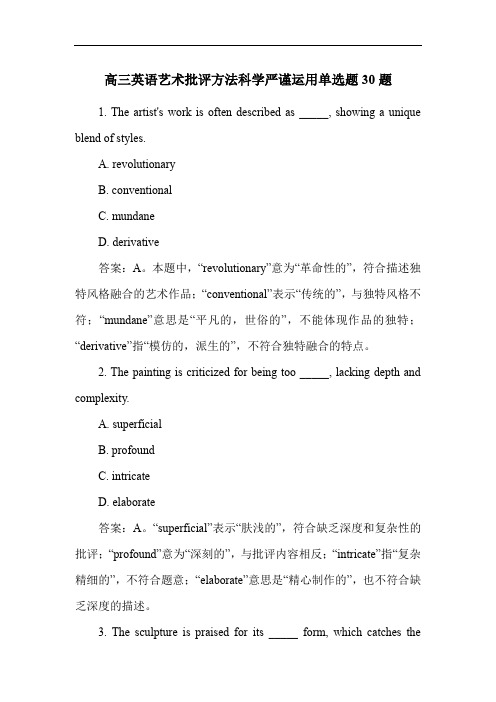
高三英语艺术批评方法科学严谨运用单选题30题1. The artist's work is often described as _____, showing a unique blend of styles.A. revolutionaryB. conventionalC. mundaneD. derivative答案:A。
本题中,“revolutionary”意为“革命性的”,符合描述独特风格融合的艺术作品;“conventional”表示“传统的”,与独特风格不符;“mundane”意思是“平凡的,世俗的”,不能体现作品的独特;“derivative”指“模仿的,派生的”,不符合独特融合的特点。
2. The painting is criticized for being too _____, lacking depth and complexity.A. superficialB. profoundC. intricateD. elaborate答案:A。
“superficial”表示“肤浅的”,符合缺乏深度和复杂性的批评;“profound”意为“深刻的”,与批评内容相反;“intricate”指“复杂精细的”,不符合题意;“elaborate”意思是“精心制作的”,也不符合缺乏深度的描述。
3. The sculpture is praised for its _____ form, which catches theviewer's eye immediately.A. amorphousB. symmetricalC. asymmetricalD. chaotic答案:B。
“symmetrical”表示“对称的”,能让人眼前一亮;“amorphous”意为“无定形的”,通常不具备吸引眼球的特点;“asymmetrical”指“不对称的”,可能不够直接吸引;“chaotic”意思是“混乱的”,不符合吸引人的描述。
托福阅读练习:人脸识别Facial Recognition
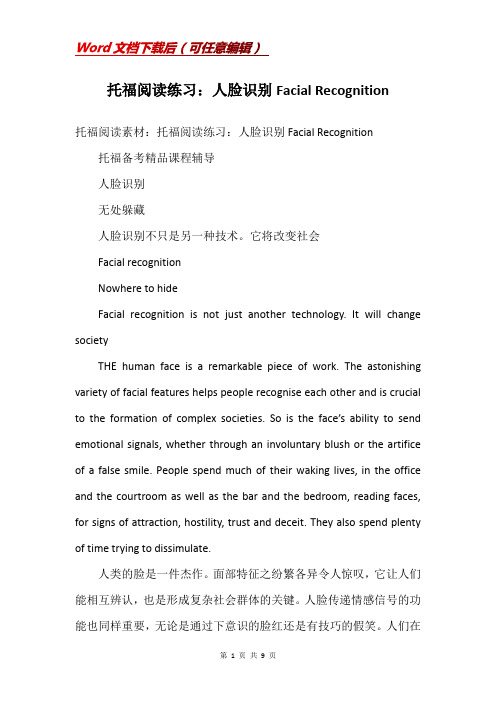
托福阅读练习:人脸识别Facial Recognition托福阅读素材:托福阅读练习:人脸识别Facial Recognition 托福备考精品课程辅导人脸识别无处躲藏人脸识别不只是另一种技术。
它将改变社会Facial recognitionNowhere to hideFacial recognition is not just another technology. It will change societyTHE human face is a remarkable piece of work. The astonishing variety of facial features helps people recognise each other and is crucial to the formation of complex societies. So is the face’s ability to send emotional signals, whether through an involuntary blush or the artifice of a false smile. People spend much of their waking lives, in the office and the courtroom as well as the bar and the bedroom, reading faces, for signs of attraction, hostility, trust and deceit. They also spend plenty of time trying to dissimulate.人类的脸是一件杰作。
面部特征之纷繁各异令人惊叹,它让人们能相互辨认,也是形成复杂社会群体的关键。
人脸传递情感信号的功能也同样重要,无论是通过下意识的脸红还是有技巧的假笑。
学术英语 理工 Unit 2练习答案
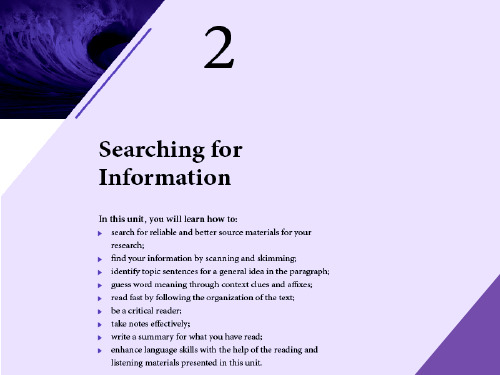
relationship of mutual reciprocity. Such an example also arises (这
种例子还出现) in other situations where social interaction occurs.
3 Identifying topic sentences for information
3 Identifying topic sentences for information
Enhancing your academic language
Complete the paragraph
Few social situations can be modeled by a single interaction. The strategy one uses to secure the best outcomes for himself (为自己 得到最好的结果) depends on his experience in previous interactions. To illustrate the point (为了说明这一点), the paper attempts to use (尝试运用) the example of a game. A player is likely to cooperate only if his opponent previously demonstrated willingness to cooperate, a process that leads to (这个过程就导致了) a
2 Scanning and skimming
Enhancing your academic language
高三英语学术研究方法创新不断单选题30题
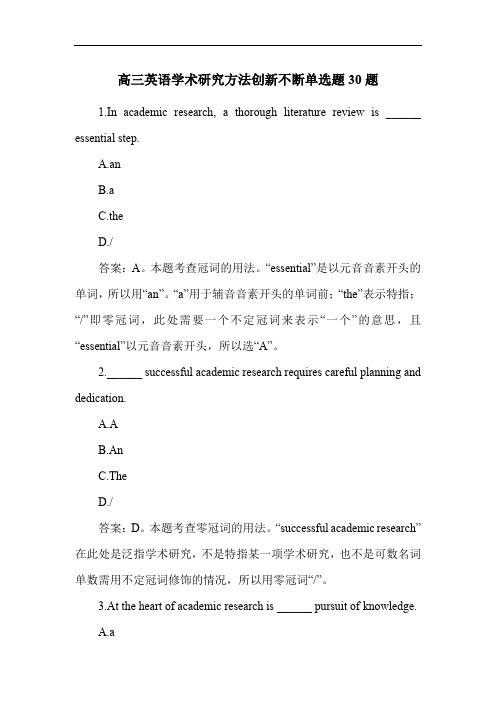
高三英语学术研究方法创新不断单选题30题1.In academic research, a thorough literature review is ______ essential step.A.anB.aC.theD./答案:A。
本题考查冠词的用法。
“essential”是以元音音素开头的单词,所以用“an”。
“a”用于辅音音素开头的单词前;“the”表示特指;“/”即零冠词,此处需要一个不定冠词来表示“一个”的意思,且“essential”以元音音素开头,所以选“A”。
2.______ successful academic research requires careful planning and dedication.A.AB.AnC.TheD./答案:D。
本题考查零冠词的用法。
“successful academic research”在此处是泛指学术研究,不是特指某一项学术研究,也不是可数名词单数需用不定冠词修饰的情况,所以用零冠词“/”。
3.At the heart of academic research is ______ pursuit of knowledge.A.aC.theD./答案:C。
本题考查定冠词的用法。
“the pursuit of knowledge”表示“对知识的追求”,是特指的概念,所以用“the”。
4.Researchers need ______ accurate data to draw valid conclusions.A.anB.aC.theD./答案:D。
本题考查零冠词的用法。
“data”在此处是不可数名词,且不是特指某一特定的数据,所以用零冠词“/”。
5.______ innovation is crucial in academic research.A.AnB.AC.TheD./答案:D。
本题考查零冠词的用法。
“innovation”在此处是泛指创新,不是特指某一个创新,也不是可数名词单数需用不定冠词修饰的情况,所以用零冠词“/”。
高中英语科技论文翻译单选题40题
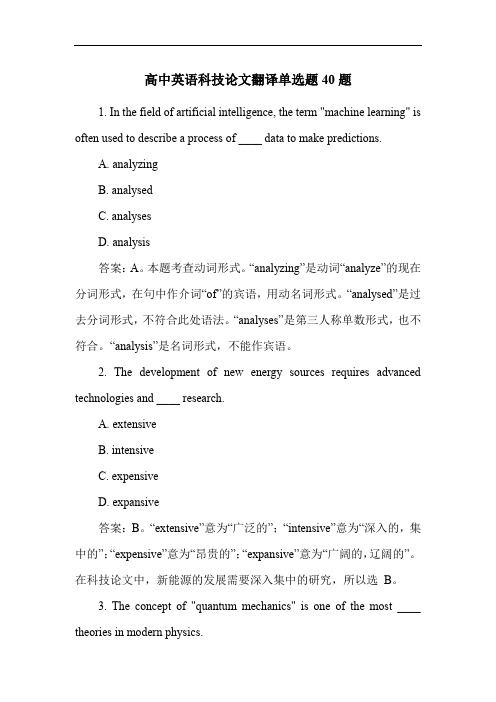
高中英语科技论文翻译单选题40题1. In the field of artificial intelligence, the term "machine learning" is often used to describe a process of ____ data to make predictions.A. analyzingB. analysedC. analysesD. analysis答案:A。
本题考查动词形式。
“analyzing”是动词“analyze”的现在分词形式,在句中作介词“of”的宾语,用动名词形式。
“analysed”是过去分词形式,不符合此处语法。
“analyses”是第三人称单数形式,也不符合。
“analysis”是名词形式,不能作宾语。
2. The development of new energy sources requires advanced technologies and ____ research.A. extensiveB. intensiveC. expensiveD. expansive答案:B。
“extensive”意为“广泛的”;“intensive”意为“深入的,集中的”;“expensive”意为“昂贵的”;“expansive”意为“广阔的,辽阔的”。
在科技论文中,新能源的发展需要深入集中的研究,所以选B。
3. The concept of "quantum mechanics" is one of the most ____ theories in modern physics.A. complexB. simpleC. easyD. common答案:A。
“complex”表示“复杂的”;“simple”表示“简单的”;“easy”表示“容易的”;“common”表示“常见的”。
量子力学的概念在现代物理学中是非常复杂的,故选A。
科学研究表明:脸部特征决定第一印象
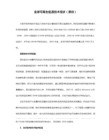
科学研究表明:脸部特征决定第一印象
It is the holy grail of politicians everywhere - how to win and keep the trust of voters.
Now researchers at the University of St Andrew's in Scotland say they may have the answer.
"Over the years, we have found that facial features affect the way many of us perceive others," says Elisabeth Cornwell, a psychology researcher at the university's Perception Laboratory.
“我们使用电脑程序改变他们的脸型和五官特征。”
“我们希望这有助于人们理解我们的成果。”
那么,我们是否可以期待在展览会上看到改头换面后的托尼·布莱尔,迈克尔·霍华德和查尔斯·肯尼迪赢得选民的青睐呢?
康威尔小姐说:“我想他们不会用这三个人的脸做实验。”
“在电视上不会成功的。我们都知道他们三个人长什么样子,我认为他们做这种试验实在是太幼稚了。”
"We are very good at processing these features quickly," says Ms Cornwell.
The researchers are putting their science to the test at the Royal Society's annual summer exhibition in London.
高三英语科学前沿展望单选题30题
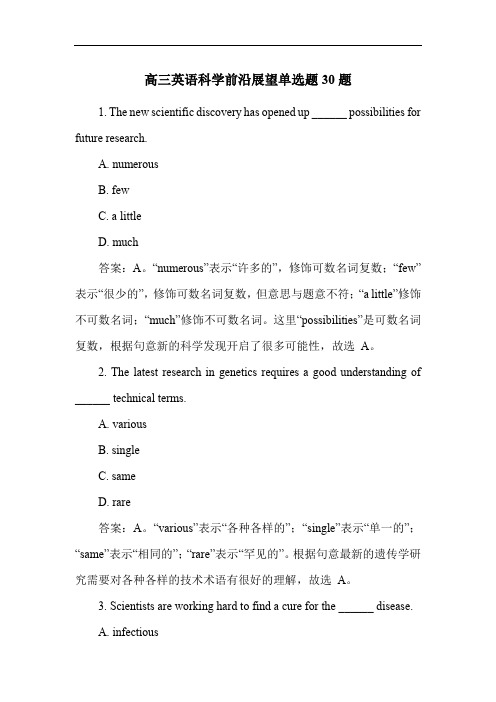
高三英语科学前沿展望单选题30题1. The new scientific discovery has opened up ______ possibilities for future research.A. numerousB. fewC. a littleD. much答案:A。
“numerous”表示“许多的”,修饰可数名词复数;“few”表示“很少的”,修饰可数名词复数,但意思与题意不符;“a little”修饰不可数名词;“much”修饰不可数名词。
这里“possibilities”是可数名词复数,根据句意新的科学发现开启了很多可能性,故选A。
2. The latest research in genetics requires a good understanding of ______ technical terms.A. variousB. singleC. sameD. rare答案:A。
“various”表示“各种各样的”;“single”表示“单一的”;“same”表示“相同的”;“rare”表示“罕见的”。
根据句意最新的遗传学研究需要对各种各样的技术术语有很好的理解,故选A。
3. Scientists are working hard to find a cure for the ______ disease.A. infectiousB. mentalC. chronicD. artificial答案:A。
“infectious”表示“传染性的”;“mental”表示“精神的”;“chronic”表示“慢性的”;“artificial”表示“人造的”。
根据常识,科学家努力寻找的通常是传染性疾病的治疗方法,故选A。
4. The breakthrough in quantum physics has brought ______ changes to the field of science.A. slightB. dramaticC. gradualD. temporary答案:B。
高三英语询问技术创新单选题50题
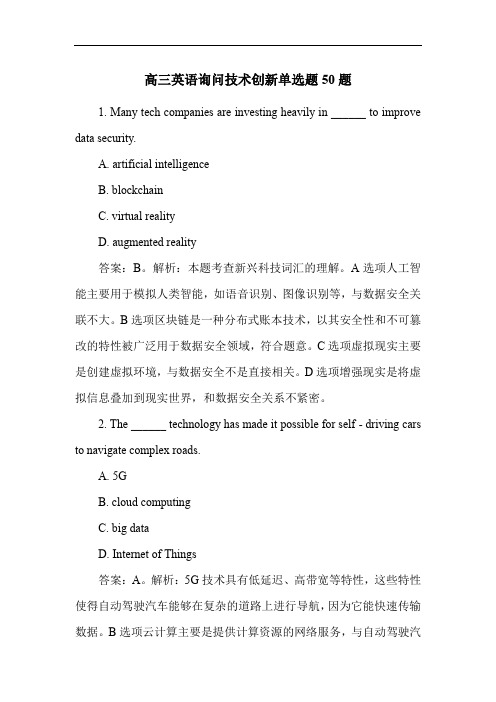
高三英语询问技术创新单选题50题1. Many tech companies are investing heavily in ______ to improve data security.A. artificial intelligenceB. blockchainC. virtual realityD. augmented reality答案:B。
解析:本题考查新兴科技词汇的理解。
A选项人工智能主要用于模拟人类智能,如语音识别、图像识别等,与数据安全关联不大。
B选项区块链是一种分布式账本技术,以其安全性和不可篡改的特性被广泛用于数据安全领域,符合题意。
C选项虚拟现实主要是创建虚拟环境,与数据安全不是直接相关。
D选项增强现实是将虚拟信息叠加到现实世界,和数据安全关系不紧密。
2. The ______ technology has made it possible for self - driving cars to navigate complex roads.A. 5GB. cloud computingC. big dataD. Internet of Things答案:A。
解析:5G技术具有低延迟、高带宽等特性,这些特性使得自动驾驶汽车能够在复杂的道路上进行导航,因为它能快速传输数据。
B选项云计算主要是提供计算资源的网络服务,与自动驾驶汽车导航关系不直接。
C选项大数据侧重于数据的收集、存储和分析,不是直接助力自动驾驶导航的关键。
D选项物联网强调设备之间的连接,并非自动驾驶汽车导航的最主要技术支持。
3. Tech startups are exploring the potential of ______ in the field of medical diagnosis.A. quantum computingB. gene editingC. nanotechnologyD. all of the above答案:D。
高中英语科技论文翻译单选题40题

高中英语科技论文翻译单选题40题1. The term "nanotechnology" is often translated as "_____".A. 纳米技术B. 微观技术C. 微观科学D. 纳米科学答案:A。
“nanotechnology”常见且准确的翻译就是“纳米技术”,B 选项“微观技术”通常用“microtechnology”,C 选项“微观科学”一般是“microscopic science”,D 选项“纳米科学”是“nanoscience”。
2. "Artificial intelligence" is best translated to "_____".A. 人工智慧B. 人造智能C. 人工智能D. 人工智力答案:C。
“Artificial intelligence”最准确和常用的翻译是“人工智能”,A 选项“人工智慧”不太符合常见表达,B 选项“人造智能”不够准确,D 选项“人工智力”不是常用的翻译。
3. The phrase "genetic engineering" can be translated as "_____".A. 基因工程B. 遗传工程C. 基因技术D. 遗传技术答案:B。
“genetic engineering”常见的翻译是“遗传工程”,A 选项“基因工程”不太准确,C 选项“基因技术”通常是“genetic technology”,D 选项“遗传技术”一般是“genetic technique”。
4. "Quantum mechanics" is usually translated to "_____".A. 量子力学B. 量子机械学C. 量子物理学D. 量子动力学答案:A。
“Quantum mechanics”准确的翻译是“量子力学”,B 选项“量子机械学”这种表述不常见,C 选项“量子物理学”是“Quantum Physics”,D 选项“量子动力学”是“Quantum Dynamics”。
高三英语艺术批评方法科学严谨单选题70题(答案解析)
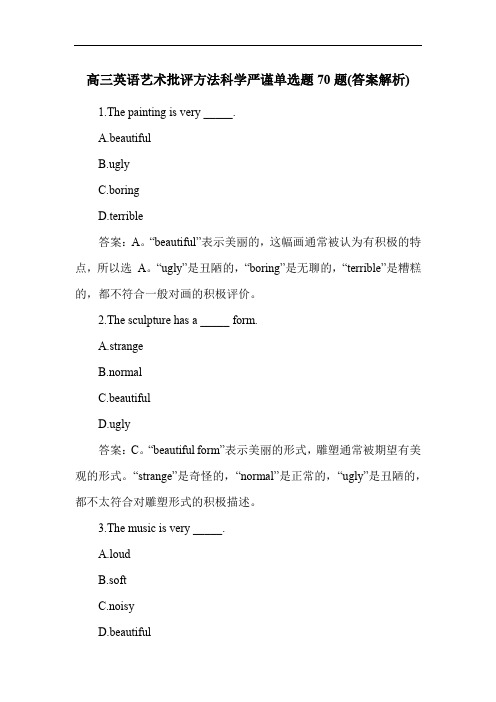
高三英语艺术批评方法科学严谨单选题70题(答案解析)1.The painting is very _____.A.beautifulB.uglyC.boringD.terrible答案:A。
“beautiful”表示美丽的,这幅画通常被认为有积极的特点,所以选A。
“ugly”是丑陋的,“boring”是无聊的,“terrible”是糟糕的,都不符合一般对画的积极评价。
2.The sculpture has a _____ form.A.strangeB.normalC.beautifulD.ugly答案:C。
“beautiful form”表示美丽的形式,雕塑通常被期望有美观的形式。
“strange”是奇怪的,“normal”是正常的,“ugly”是丑陋的,都不太符合对雕塑形式的积极描述。
3.The music is very _____.A.loudB.softC.noisyD.beautiful答案:D。
“beautiful”可以形容音乐好听。
“loud”是大声的,“soft”是轻柔的,“noisy”是嘈杂的,都不如“beautiful”能体现对音乐的积极评价。
4.The dance performance was _____.A.excitingB.boringC.terribleD.ugly答案:A。
“exciting”表示令人兴奋的,舞蹈表演通常希望给人带来积极的感受。
“boring”是无聊的,“terrible”是糟糕的,“ugly”一般不用于形容舞蹈表演。
5.The play had a _____ plot.A.boringB.interestingC.terribleD.ugly答案:B。
“interesting plot”表示有趣的情节,戏剧通常要有吸引人的情节。
“boring”是无聊的,“terrible”是糟糕的,“ugly”不用于形容情节。
6.The art exhibition was very _____.A.interestingB.boringD.ugly答案:A。
face recognition 考研英语阅读
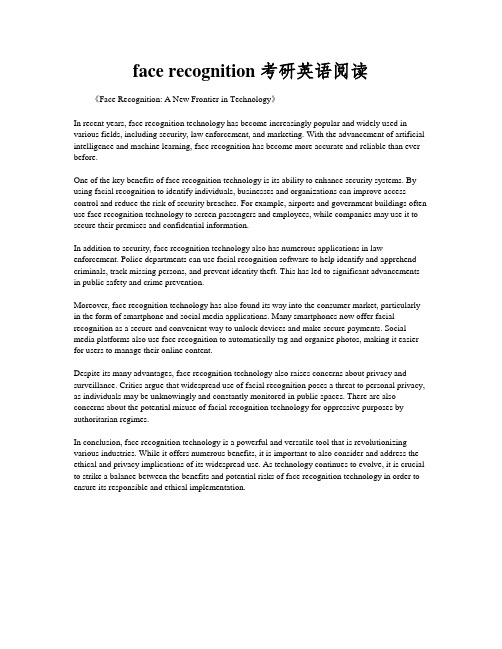
face recognition 考研英语阅读《Face Recognition: A New Frontier in Technology》In recent years, face recognition technology has become increasingly popular and widely used in various fields, including security, law enforcement, and marketing. With the advancement of artificial intelligence and machine learning, face recognition has become more accurate and reliable than ever before.One of the key benefits of face recognition technology is its ability to enhance security systems. By using facial recognition to identify individuals, businesses and organizations can improve access control and reduce the risk of security breaches. For example, airports and government buildings often use face recognition technology to screen passengers and employees, while companies may use it to secure their premises and confidential information.In addition to security, face recognition technology also has numerous applications in law enforcement. Police departments can use facial recognition software to help identify and apprehend criminals, track missing persons, and prevent identity theft. This has led to significant advancements in public safety and crime prevention.Moreover, face recognition technology has also found its way into the consumer market, particularly in the form of smartphone and social media applications. Many smartphones now offer facial recognition as a secure and convenient way to unlock devices and make secure payments. Social media platforms also use face recognition to automatically tag and organize photos, making it easier for users to manage their online content.Despite its many advantages, face recognition technology also raises concerns about privacy and surveillance. Critics argue that widespread use of facial recognition poses a threat to personal privacy, as individuals may be unknowingly and constantly monitored in public spaces. There are also concerns about the potential misuse of facial recognition technology for oppressive purposes by authoritarian regimes.In conclusion, face recognition technology is a powerful and versatile tool that is revolutionizing various industries. While it offers numerous benefits, it is important to also consider and address the ethical and privacy implications of its widespread use. As technology continues to evolve, it is crucial to strike a balance between the benefits and potential risks of face recognition technology in order to ensure its responsible and ethical implementation.。
高二英语科学探索技巧深化单选题45题
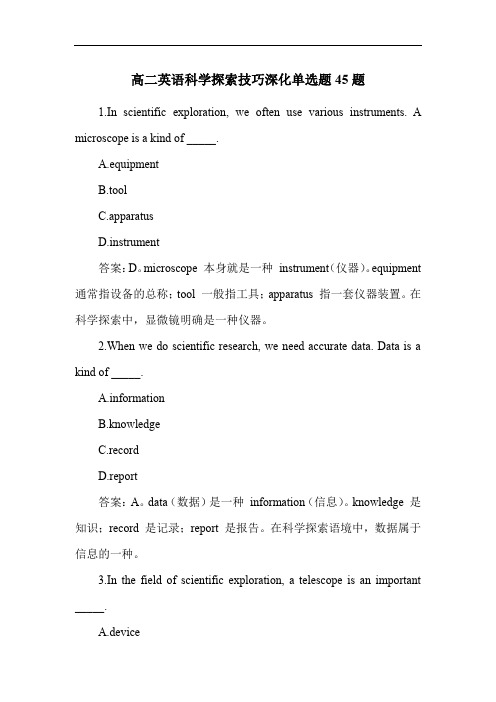
高二英语科学探索技巧深化单选题45题1.In scientific exploration, we often use various instruments. A microscope is a kind of _____.A.equipmentB.toolC.apparatusD.instrument答案:D。
microscope 本身就是一种instrument( 仪器)。
equipment 通常指设备的总称;tool 一般指工具;apparatus 指一套仪器装置。
在科学探索中,显微镜明确是一种仪器。
2.When we do scientific research, we need accurate data. Data is a kind of _____.rmationB.knowledgeC.recordD.report答案:A。
data( 数据)是一种information( 信息)。
knowledge 是知识;record 是记录;report 是报告。
在科学探索语境中,数据属于信息的一种。
3.In the field of scientific exploration, a telescope is an important _____.A.deviceB.implementC.applianceD.instrument答案:D。
telescope( 望远镜)是一种instrument( 仪器)。
device 一般指装置;implement 指工具、器具;appliance 通常指家用电器。
望远镜在科学探索中属于仪器。
4.Scientists use different chemicals in their experiments. A chemical is a kind of _____.A.substanceB.materialC.elementposition答案:A。
洗脸的英语作文怎么写
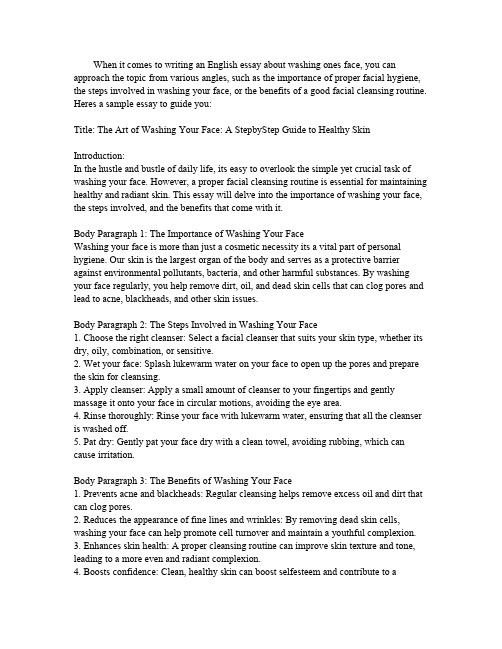
When it comes to writing an English essay about washing ones face,you can approach the topic from various angles,such as the importance of proper facial hygiene, the steps involved in washing your face,or the benefits of a good facial cleansing routine. Heres a sample essay to guide you:Title:The Art of Washing Your Face:A StepbyStep Guide to Healthy SkinIntroduction:In the hustle and bustle of daily life,its easy to overlook the simple yet crucial task of washing your face.However,a proper facial cleansing routine is essential for maintaining healthy and radiant skin.This essay will delve into the importance of washing your face, the steps involved,and the benefits that come with it.Body Paragraph1:The Importance of Washing Your FaceWashing your face is more than just a cosmetic necessity its a vital part of personal hygiene.Our skin is the largest organ of the body and serves as a protective barrier against environmental pollutants,bacteria,and other harmful substances.By washing your face regularly,you help remove dirt,oil,and dead skin cells that can clog pores and lead to acne,blackheads,and other skin issues.Body Paragraph2:The Steps Involved in Washing Your Face1.Choose the right cleanser:Select a facial cleanser that suits your skin type,whether its dry,oily,combination,or sensitive.2.Wet your face:Splash lukewarm water on your face to open up the pores and prepare the skin for cleansing.3.Apply cleanser:Apply a small amount of cleanser to your fingertips and gently massage it onto your face in circular motions,avoiding the eye area.4.Rinse thoroughly:Rinse your face with lukewarm water,ensuring that all the cleanser is washed off.5.Pat dry:Gently pat your face dry with a clean towel,avoiding rubbing,which can cause irritation.Body Paragraph3:The Benefits of Washing Your Face1.Prevents acne and blackheads:Regular cleansing helps remove excess oil and dirt that can clog pores.2.Reduces the appearance of fine lines and wrinkles:By removing dead skin cells, washing your face can help promote cell turnover and maintain a youthful complexion.3.Enhances skin health:A proper cleansing routine can improve skin texture and tone, leading to a more even and radiant complexion.4.Boosts confidence:Clean,healthy skin can boost selfesteem and contribute to apositive selfimage.Conclusion:In conclusion,washing your face is a fundamental aspect of skincare that should not be underestimated.By following a proper facial cleansing routine,you can enjoy the numerous benefits that come with clean,healthy skin.Remember,the key to a successful facial cleansing routine lies in consistency and using products that cater to your specific skin needs.So,make washing your face a daily habit and embrace the journey to healthier,more radiant skin.Remember to proofread your essay for grammar,punctuation,and spelling errors to ensure it is polished and professional.。
GaussianFace
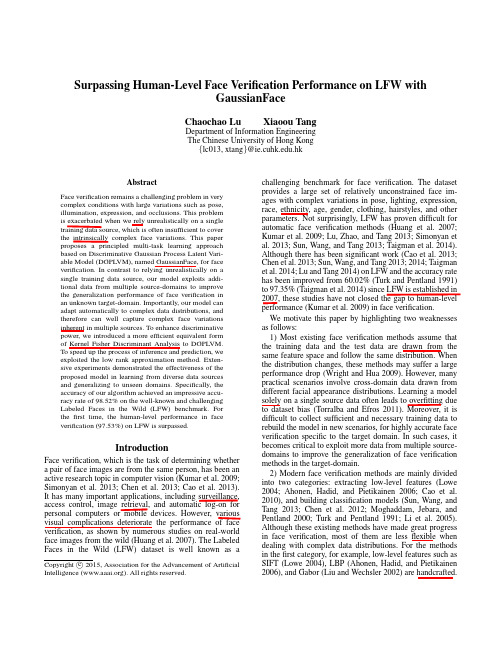
Surpassing Human-Level Face Verification Performance on LFW withGaussianFaceChaochao Lu Xiaoou TangDepartment of Information EngineeringThe Chinese University of Hong Kong{lc013,xtang}@.hkAbstractFace verification remains a challenging problem in verycomplex conditions with large variations such as pose,illumination,expression,and occlusions.This problemis exacerbated when we rely unrealistically on a singletraining data source,which is often insufficient to coverthe intrinsically complex face variations.This paperproposes a principled multi-task learning approachbased on Discriminative Gaussian Process Latent Vari-able Model(DGPLVM),named GaussianFace,for faceverification.In contrast to relying unrealistically on asingle training data source,our model exploits addi-tional data from multiple source-domains to improvethe generalization performance of face verification inan unknown target-domain.Importantly,our model canadapt automatically to complex data distributions,andtherefore can well capture complex face variationsinherent in multiple sources.To enhance discriminativepower,we introduced a more efficient equivalent formof Kernel Fisher Discriminant Analysis to DGPLVM.To speed up the process of inference and prediction,weexploited the low rank approximation method.Exten-sive experiments demonstrated the effectiveness of theproposed model in learning from diverse data sourcesand generalizing to unseen domains.Specifically,theaccuracy of our algorithm achieved an impressive accu-racy rate of98.52%on the well-known and challengingLabeled Faces in the Wild(LFW)benchmark.Forthefirst time,the human-level performance in faceverification(97.53%)on LFW is surpassed.IntroductionFace verification,which is the task of determining whether a pair of face images are from the same person,has been an active research topic in computer vision(Kumar et al.2009; Simonyan et al.2013;Chen et al.2013;Cao et al.2013). It has many important applications,including surveillance, access control,image retrieval,and automatic log-on for personal computers or mobile devices.However,various visual complications deteriorate the performance of face verification,as shown by numerous studies on real-world face images from the wild(Huang et al.2007).The Labeled Faces in the Wild(LFW)dataset is well known as a Copyright c 2015,Association for the Advancement of Artificial Intelligence().All rights reserved.challenging benchmark for face verification.The dataset provides a large set of relatively unconstrained face im-ages with complex variations in pose,lighting,expression, race,ethnicity,age,gender,clothing,hairstyles,and other parameters.Not surprisingly,LFW has proven difficult for automatic face verification methods(Huang et al.2007; Kumar et al.2009;Lu,Zhao,and Tang2013;Simonyan et al.2013;Sun,Wang,and Tang2013;Taigman et al.2014). Although there has been significant work(Cao et al.2013; Chen et al.2013;Sun,Wang,and Tang2013;2014;Taigman et al.2014;Lu and Tang2014)on LFW and the accuracy rate has been improved from60.02%(Turk and Pentland1991) to97.35%(Taigman et al.2014)since LFW is established in 2007,these studies have not closed the gap to human-level (Kumar et al.2009)in face verification.We motivate this paper by highlighting two weaknesses as follows:1)Most existing face verification methods assume that the training data and the test data are drawn from the same feature space and follow the same distribution.When the distribution changes,these methods may suffer a large performance drop(Wright and Hua2009).However,many practical scenarios involve cross-domain data drawn from different facial appearance distributions.Learning a model solely on a single source data often leads to overfitting due to dataset bias(Torralba and Efros2011).Moreover,it is difficult to collect sufficient and necessary training data to rebuild the model in new scenarios,for highly accurate face verification specific to the target domain.In such cases,it becomes critical to exploit more data from multiple source-domains to improve the generalization of face verification methods in the target-domain.2)Modern face verification methods are mainly divided into two categories:extracting low-level features(Lowe 2004;Ahonen,Hadid,and Pietikainen2006;Cao et al. 2010),and building classification models(Sun,Wang,and Tang2013;Chen et al.2012;Moghaddam,Jebara,and Pentland2000;Turk and Pentland1991;Li et al.2005). Although these existing methods have made great progress in face verification,most of them are lessflexible when dealing with complex data distributions.For the methods in thefirst category,for example,low-level features such as SIFT(Lowe2004),LBP(Ahonen,Hadid,and Pietikainen 2006),and Gabor(Liu and Wechsler2002)are handcrafted.Even for features learned from data(Cao et al.2010), the algorithm parameters(such as the depth of random projection tree,or the number of centers in k-means)also need to be specified by users.Similarly,for the methods in the second category,the architectures of deep networks in(Sun,Wang,and Tang2013;Taigman et al.2014;Sun, Wang,and Tang2014)(for example,the number of layers, the number of nodes in each layer,etc.),and the parameters of the models in(Li et al.2013;Berg and Belhumeur2012; Kumar et al.2009;Simonyan et al.2013)(for example,the number of Gaussians,the number of classifiers,etc.)must also be determined in advance.Since most existing methods require some assumptions to be made about the structures of the data,they cannot work well when the assumptions are not valid.Moreover,due to the existence of the assumptions, it is hard to capture the intrinsic structures of data using these methods.To this end,we propose the multi-task learning ap-proach based on Discriminative Gaussian Process Latent Variable Model(DGPLVM)(Urtasun and Darrell2007), named GaussianFace,for face verification.In order to take advantage of more data from multiple source-domains to improve the performance in the target-domain,we introduce the multi-task learning constraint to DGPLVM.Here,we investigate the asymmetric multi-task learning because we only focus on the performance improvement of the target task.Moreover,the GaussianFace model is a reformulation based on the Gaussian Processes(GPs)(Rasmussen and Williams2006),which is a non-parametric Bayesian kernel method.Therefore,our model also can adapt its complexity flexibly to the complex data distributions in the real-world, without any heuristics or manual tuning of parameters. Reformulating GPs for large-scale multi-task learning is non-trivial.To simplify calculations,we introduce a more efficient equivalent form of Kernel Fisher Discriminant Analysis(KFDA)to DGPLVM.Despite that the Gaussian-Face model can be optimized effectively using the Scaled Conjugate Gradient(SCG)technique,the inference is slow for large-scale data.We make use of GP approximations (Rasmussen and Williams2006)and low rank approxima-tion(Liu,He,and Chang2010)to speed up the process of inference and prediction,so as to scale our model to large-scale data.Our model can be applied to face verification in two different ways:as a binary classifier and as a feature extractor.In the former mode,given a pair of face images, we can directly compute the posterior likelihood for each class to make a prediction.In the latter mode,our model can automatically extract high-dimensional features for each pair of face images,and then feed them to a classifier to make thefinal decision.The main contributions of this paper are as follows: (1)We propose a novel GaussianFace model for face verification by virtue of the multi-task learning constraint to DGPLVM.Our model can adapt to complex distributions, avoid over-fitting,exploit discriminative information,and take advantage of multiple source-domains data.(2)We introduce a more efficient and dicriminative equivalent form of KFDA to DGPLVM.This equivalent form reformulates KFDA to the kernel version consistent with the covariance function in GPs,which greatly simplifies calculations.(3) We introduce the low rank approximation to speed up the process of inference and prediction.(4)Based on Gaus-sianFace model,we propose two different approaches for face verification:a binary classifier and a feature extractor.(5)We achieve superior performance on the challenging LFW benchmark(Huang et al.2007),with an accuracy rate of98.52%,beyond human-level performance reported in (Kumar et al.2009)for thefirst time.PreliminaryIn this section,we briefly review Gaussian Processes (GPs)for classification and clustering(Kim and Lee 2007),and Gaussian Process Latent Variable Model (GPLVM)(Lawrence2003).We recommend Rasmussen and Williams’s excellent monograph for further reading (Rasmussen and Williams2006).Gaussian Processes for Binary Classification Formally,for two-class classification,suppose that we have a training set D of N observations,D={(x i,y i)}N i=1, where the i-th input point x i∈R D and its corresponding output y i is binary,with y=1i for one class and y i=−1for the other.Let X be the N×D matrix,where the row vectors represent all n input points,and y be the column vector of all n outputs.We define a latent variable f i for each input point x i,and let f=[f1,...,f N] .A sigmoid functionπ(·)is imposed to squash the output of the latent function into[0,1],π(f i)=p(y i=1|f i).Assuming the data set is i.i.d,then the joint likelihood factorizes top(y|f)=Ni=1p(y i|f i)=Ni=1π(y i f i).(1) Moreover,the posterior distribution over latent functions isp(f|X,y)=p(y|f)p(f|X)p(y|X),(2)where p(f|X)is a zero-mean Gaussian Process prior over f with covariance K and K i,j=k(x i,x j).The hyper-parameters of K are denoted byθ.Since neither p(f|X,y)nor p(y|f)can be computed analytically,the Laplace method is utilized to approximate the posterior.The optimal value ofθcan be acquired by using the gradient method.Given any unseen test point x∗,the probability of its latent function f∗isf∗|X,y,x∗∼N(K∗K−1ˆf,K∗∗−K∗˜K−1K ∗),(3)where˜K=K+W−1,W=− log p(f|X,y)|f=ˆf, K∗=[k(x∗,x1),...,k(x∗,x N)],K∗∗=k(x∗,x∗)and ˆf=arg maxfp(f|X,y).Finally,we squash f∗tofind the probability of class membership as follows¯π(f∗)=π(f∗)p(f∗|X,y,x∗)d f∗.(4) Gaussian Processes for ClusteringThe principle of GP clustering is based on the key obser-vation that the variances of predictive values are smaller in dense areas and larger in sparse areas.The variances can beemployed as a good estimate of the support of a probability density function,where each separate support domain can be considered as a cluster.This observation can be explained from the variance function of any predictive data point x∗σ2(x∗)=K∗∗−K∗˜K−1K ∗.(5) If x∗is in a sparse region,then K∗˜K−1K ∗becomes small,which leads to large varianceσ2(x∗),and vice versa. Another good property of Equation(5)is that it does not depend on the labels,which means it can be applied to the unlabeled data.To perform clustering,the following dynamic system associated with Equation(5)can be written asF(x)=− σ2(x).(6) The theorem in(Kim and Lee2007)guarantees that almost all the trajectories approach one of the stable equilibrium points detected from Equation(6).After each data point finds its corresponding stable equilibrium point,we can employ a complete graph(Ben-Hur et al.2002;Kim and Lee2007)to assign cluster labels to data points with the stable equilibrium points.Obviously,the variance function in Equation(5)completely determines the performance of clustering.Gaussian Process Latent Variable ModelGPLVM has been extensively studied(Damianou and Lawrence2013;Lawrence2003).Formally,let Z= [z1,...,z N] denote the matrix whose rows represent cor-responding positions of X in latent space,where z i∈R d (d D).The GPLVM can be interpreted as a Gaussian process mapping from a low dimensional latent space to a high dimensional data set,where the locale of the points in latent space is determined by maximizing the Gaussian process likelihood with respect to Z.Given a covariance function for the Gaussian process,denoted by k(·,·),the likelihood of the data given the latent positions is as follows,p(X|Z,θ)=1(2π)ND|K|Dexp−12tr(K−1XX ),(7)where K i,j=k(z i,z j).Therefore,the posterior can be written asp(Z,θ|X)=1Z ap(X|Z,θ)p(Z)p(θ),(8)where Z a is a normalization constant,the uninformative priors overθ,and the simple spherical Gaussian priors over Z are introduced(Urtasun and Darrell2007).To obtain the optimalθand Z,we need to optimize the above likelihood (8)with respect toθand Z,respectively.GaussianFaceIn order to automatically learn discriminative features or covariance function,and to take advantage of source-domain data to improve the performance in face verification,we develop a principled GaussianFace model by including the multi-task learning constraint into Discriminative Gaussian Process Latent Variable Model(DGPLVM)(Urtasun and Darrell2007).DGPLVM ReformulationThe DGPLVM is an extension of GPLVM,where the discriminative prior is placed over the latent positions,rather than a simple spherical Gaussian prior.The DGPLVM uses the discriminative prior to encourage latent positions of the same class to be close and those of different classes to be far.Since face verification is a binary classification problem and the GPs mainly depend on the kernel function,it is natural to use Kernel Fisher Discriminant Analysis(KFDA) (Kim,Magnani,and Boyd2006)to model class structures in kernel spaces.For simplicity of inference in the followings, we introduce another equivalent formulation of KFDA to replace the one in(Urtasun and Darrell2007).KFDA is a kernelized version of linear discriminant analysis method.Itfinds the direction defined by a kernel in a feature space,onto which the projections of positive and negative classes are well separated by maximizing the ratio of the between-class variance to the within-class variance. Formally,let{z1,...,z N+}denote the positive class and {z N++1,...,z N}the negative class,where the numbers of positive and negative classes are N+and N−=N−N+, respectively.Let K be the kernel matrix.Therefore,in the feature space,the two sets{φK(z1),...,φK(z N+)}and {φK(z N++1),...,φK(z N)}represent the positive class and the negative class,respectively.The optimization criterion of KFDA is to maximize the ratio of the between-class variance to the within-class varianceJ(ω,K)=(w (µ+K−µ−K))2w (Σ+K+Σ−K+λI N)w,(9)whereλis a positive regularization parameter,µ+K= 1N+N+i=1φK(z i),µ−K=1N−Ni=N++1φK(z i),Σ+K=1N+N+i=1(φK(z i)−µ+K)(φK(z i)−µ+K) ,andΣ−K=1N−Ni=N++1(φK(z i)−µ−K)(φK(z i)−µ−K) .In this paper,however,we focus on the covariance func-tion rather than the latent positions.To simplify calculations, we represent Equation(9)with the kernel function,and let the kernel function have the same form as the covariance function.Therefore,it is natural to introduce a more efficient equivalent form of KFDA with certain assumptions as Kim et al.points out(Kim,Magnani,and Boyd2006),i.e., maximizing Equation(9)is equivalent to maximizing the following equationJ∗=1 a Ka−a KA(λIn+AKA)−1AKa,(10) where A=diagI N+−1N+1N+1N+√N+,I N−−1N−1N−1N−√N−, a=[1N+N+,−1N−N−],andλis a positive regularization parameter.Here,I N denotes the N×N identity matrix and1N denotes the length-N vector of all ones in R N. Therefore,the discriminative prior over the latent positions in DGPLVM can be written asp(Z)=1Z bexp−1σ2J∗,(11) where Z b is a normalization constant,andσ2represents a global scaling of the prior.The covariance matrix obtained by DGPLVM is discrim-inative and moreflexible than the one used in conventional GPs for classification(GPC),since they are learned based on a discriminative criterion,and more degrees of freedom are estimated than conventional kernel hyper-parameters. Multi-task Learning ConstraintFrom an asymmetric multi-task learning perspective,the tasks should be allowed to share common hyper-parameters of the covariance function.Moreover,from an information theory perspective,the information cost between target task and multiple source tasks should be minimized.A natural way to quantify the information cost is to use the mutual entropy,because it is the measure of the mutual dependence of two distributions.For multi-task learning,we extend the mutual entropy to multiple distributions as followsM=H(p t)−1SSi=1H(p t|p i),(12)where H(·)is the marginal entropy,H(·|·)is the conditional entropy,S is the number of source tasks,{p i}S i=1,and p t are the probability distributions of source tasks and target task, respectively.GaussianFace ModelIn this section,we describe our GaussianFace model in detail.Suppose we have S source-domain datasets {X1,...,X S}and a target-domain data X T.For each source-domain data or target-domain data X i,according to Equation(7),we write its marginal likelihoodp(X i|Z i,θ)=1(2π)ND|K|Dexp−12tr(K−1X i X i).(13)where Z i represents the domain-relevant latent space. For each source-domain data and target-domain data,their covariance functions K have the same form because they share the same hyper-parametersθ.In this paper,we use a widely used kernelK i,j=kθ(z i,z j)=θ0exp−12dm=1θm(z m i−z m j)2+θd+1+δzi,z jθd+2,(14)whereθ={θi}d+2i=0and d is the dimension of the datapoint.Then,from Equations(8),learning the DGPLVM is equivalent to optimizingp(Z i,θ|X i)=1Z ap(X i|Z i,θ)p(Z i)p(θ),(15)where p(X i|Z i,θ)and p(Z i)are respectively represented in(13)and(11).According to the multi-task learning constraint in Equation(12),we can attainM=H(p(Z T,θ|X T))−1SSi=1H(p(Z T,θ|X T)|p(Z i,θ|X i)).(16)From Equations(13),(15),and(16),we know that learningthe GaussianFace model amounts to minimizing the follow-ing marginal likelihoodL Model=−log p(Z T,θ|X T)−βM,(17)where the parameterβbalances the relative importancebetween the target-domain data and the multi-task learningconstraint.We can now optimize Equation(17)with respectto the hyper-parametersθand the latent positions Z i by theScaled Conjugate Gradient(SCG)technique.More detailedderivations can be found in the supplementary material.SpeedupIn the GaussianFace model,we need to invert the largematrix when doing inference and prediction.For largeproblems,both storing the matrix and solving the associatedlinear systems are computationally prohibitive.In this paper,we use the anchor graphs method(Liu,He,and Chang2010)to speed up this process.To put it simply,wefirstselect q(q n)anchors to cover a cloud of n data points,and form an n×q matrix Q,where Q i,j=kθ(z i,z j).z i and z j are from n latent data points and q anchors,respectively.Then the original kernel matrix K can beapproximated as K≈QQ .Using the Woodbury identity(Higham1996),computing the n×n matrix QQ can betransformed into computing the q×q matrix Q Q,whichis more efficient.Speedup on Inference When optimizing Equation(17),we need to invert the matrix(λI n+AKA).Duringinference,we take q k-means clustering centers as anchorsto form Q.Substituting K≈QQ into(λI n+AKA),andthen using the Woodbury identity,we get(λI n+AKA)−1≈(λI n+AQQ A)−1=λ−1I n−λ−1AQ(λI q+Q AAQ)−1Q A.Similarly,let K−1≈(K+τI)−1whereτa constant term,then we can getK−1≈(K+τI)−1≈τ−1I n−τ−1Q(τI q+Q Q)−1Q .Speedup on Prediction When we compute the predictivevarianceσ(z∗),we need to invert the matrix(K+W−1).At this time,we can use the method in Section to calculatethe accurate clustering centers that can be regarded as theing the Woodbury identity again,we obtain(K+W−1)−1≈W−WQ(I q+Q WQ)−1Q W,where(I q+Q WQ)is only a q×q matrix,and its inversematrix can be computed more efficiently.GaussianFace Model for Face VerificationIn this section,we describe two applications of the Gaus-sianFace model to face verification:as a binary classifier andas a feature extractor.Each face image isfirst normalized to150×120size by an affine transformation based onfivelandmarks(two eyes,nose,and two mouth corners).Theimage is then divided into overlapped patches of25×25AB⋯⋯⋯⋯⋯⋯S 1S P⋯GaussianFace Model As a Binary ClassifierSame Or DifferentImage pairMulti-scale AB⋯⋯⋯⋯⋯⋯GaussianFaceModel As a Feature ExtractorImage pair Joint Feature Vector⋯⋯⋯⋯⋯⋯⋯⋯⋯⋯⋯⋯⋯⋯High-dimensionalConcatenated Figure 1:Two verification.(a)GaussianFace model as a binary classifier.(b)GaussianFace model as a feature extractor.pixels with a stride of 2pixels.Each patch within the image is mapped to a vector by a certain descriptor,and the vectoris regarded as the feature of the patch,denoted by {x A p }Pp =1where P is the number of patches within the face image A .In this paper,the multi-scale LBP feature of each patch is extracted (Chen et al.2013).The difference is that the multi-scale LBP descriptors are extracted at the center of each patch instead of accurate landmarks.GaussianFace Model as a Binary ClassifierFor classification,our model can be regarded as an approach to learn a covariance function for GPC,as shown in Figure 1(a).Here,for a pair of face images A and B from the same (or different)person,let the similarity vector x i =[s 1,...,s p ,...,s P ] be the input data point of the GaussianFace model,where s p is thesimilarity of x A p and x Bp ,and its corresponding output is y i =1(or −1).With the learned hyper-parameters of covariance function from the training data,given any un-seen pair of face images,we first compute its similarity vector x ∗using the above method,then estimate its latent representation z ∗using the same method in (Urtasun and Darrell 2007)1,and finally predict whether the pair is from the same person through Equation (4).In this paper,we prescribe the sigmoid function π(·)to be the cumulative Gaussian distribution Φ(·),which can be solved analytically as ¯π∗=Φ¯f ∗(z ∗)/ 2∗ ,whereσ2(z ∗)=K ∗∗−K ∗˜K −1K ∗and ¯f ∗(z ∗)=K ∗K −1ˆf from Equation (3)(Rasmussen and Williams 2006).We call the method GaussianFace-BC .GaussianFace Model as a Feature ExtractorAs a feature extractor,our model can be regarded as an approach to automatically extract facial features,shown in Figure 1(b).Here,for a pair of face images A and B from the same (or different)person,we regard the joint feature1For convenience,readers can also refer to the supplementary material for the inference.vector x i =[(x A i ) ,(x B i ) ]as the input data point of the GaussianFace model,and its corresponding output is y i =1(or −1).To enhance the robustness of our approach,the flipped form of x i is also included;for example,x i =[(x B i ) ,(x A i ) ].After the hyper-parameters of covariance function are learnt from the training data,we first estimate the latent representations of the training data using the same method in (Urtasun and Darrell 2007),then can use the method in Section to group the latent data points into different clusters automatically.Suppose that we finally obtain C clusters.The centers of these clusters are denotedby {c i }C i =1,the variances of these clusters by {Σ2i }Ci =1,and their weights by {w i }C i =1where w i is the ratio of the number of latent data points from the i -th cluster to the number of all latent data points.Then we refer to each c i as the input of Equation (3),and we can obtain its correspondingprobability p i and variance σ2i .In fact,{c i }C i =1can be regarded as a codebook generated by our model.For any un-seen pair of face images,we also first compute its joint feature vector x ∗for each pair of patches,and estimate its latent representation z ∗.Then we compute its first-order and second-order statistics to the centers.Similarly,we regard z ∗as the input of Equation (3),and can also obtain its corresponding probability p ∗and variance σ2∗.The statistics and variance of z ∗are represented as its high-dimensional facial features,denotedby ˆz ∗=[∆11,∆21,∆31,∆41,...,∆1C ,∆2C ,∆3C ,∆4C ] ,where ∆1i =w i z ∗−c i Σi ,∆2i =w i z ∗−c iΣi 2,∆3i =log p ∗(1−p i )p i (1−p ∗),and ∆4i =σ2∗σ2i.We concatenate all of the new high-dimensional features from each pair of patches to form the final new high-dimensional feature for the pair of face images,and then compute the cosine similarity.The new high-dimensional facial features not only describe how the distribution of features of an un-seen face image differs from the distribution fitted to the features of all training images,but also encode the predictive information including the probabilities of label and uncertainty.We call this approach GaussianFace-FE .Experimental SettingsIn this section,we conduct experiments on face verification.We start by introducing the source-domain datasets and the target-domain dataset in all of our experiments (see Figure 2for examples).The source-domain datasets include four different types of datasets as follows:Multi-PIE (Gross et al.2010).This dataset contains face images from 337subjects under 15view points and 19illumination conditions in four recording sessions.These images are collected under controlled conditions.MORPH (Ricanek and Tesafaye 2006).The MORPH database contains 55,000images of more than 13,000people within the age ranges of 16to 77.There are an average of 4images per individual.Web Images 2.This dataset contains around 40,000facial2These two datasets are collected by our own from the Web.It is guaranteed that these two datasets are mutually exclusive with the LFW dataset.Figure2:Samples of the datasets in our experiments.From left to right:LFW,Multi-PIE,MORPH,Web Images,and Life Photos. images from3261subjects;that is,approximately10images for each person.The images were collected from the Web with significant variations in pose,expression,and illumina-tion conditions.Life Photos2.This dataset contains approximately5000 images of400subjects collected online.Each subject has roughly10images.If not otherwise specified,the target-domain dataset is the benchmark of face verification as follows:LFW(Huang et al.2007).This dataset contains13,233 uncontrolled face images of5749publicfigures with variety of pose,lighting,expression,race,ethnicity,age,gender, clothing,hairstyles,and other parameters.All of these images are collected from the Web.We use the LFW dataset as the target-domain dataset because it is well known as a challenging benchmark. Using it also allows us to compare directly with other existing face verification methods(Cao et al.2013;Berg and Belhumeur2012;Chen et al.2013;Simonyan et al.2013; Chen et al.2012).Besides,this dataset provides a large set of relatively unconstrained face images with complex variations as described above,and has proven difficult for automatic face verification methods(Huang et al.2007; Kumar et al.2009).In all the experiments conducted on LFW,we strictly follow the standard unrestricted protocol of LFW(Huang et al.2007).More precisely,during the training procedure,the four source-domain datasets are: Web Images,Multi-PIE,MORPH,and Life Photos,the target-domain dataset is the training set in View1of LFW, and the validation set is the test set in View1of LFW.At the test time,we follow the standard10-fold cross-validation protocol to test our model in View2of LFW.For each one of the four source-domain datasets,we ran-domly sample20,000pairs of matched images and20,000 pairs of mismatched images.The training partition and the testing partition in all of our experiments are mutually exclusive.In other words,there is no identity overlap among the two partitions.For the experiments below,“The Number of SD”means “the Number of Source-Domain datasets that are fed into the GaussianFace model for training”.By parity of reasoning,if“The Number of SD”is i,that means the first i source-domain datasets are used for model training. Therefore,if“The Number of SD”is0,models are trained with the training data from target-domain data only.model and other competing MTGP/GP methods as a binary classifier.(b)The accuracy rate(%)of the GaussianFace-FE model and other competing MTGP/GP methods as a feature extractor.(c) The relative improvement of each method as a binary classifier with the increasing number of SD,compared to their performance when the number of SD is0.(d)The relative improvement of each method as a feature extractor with the increasing number of SD, compared to their performance when the number of SD is0. Implementation details.Our model involves four impor-tant parameters:λin(10),σin(11),βin(17),and the number of anchors q3.Following the same setting in(Kim, Magnani,and Boyd2006),the regularization parameterλin(10)isfixed to10−8.σreflects the tradeoff between our method’s ability to discriminate(smallσ)and its ability to generalize(largeσ),andβbalances the relative importance between the target-domain data and the multi-task learning constraint.Therefore,the validation set(the test set in View 1of LFW)is used for selectingσandβ.Each time we use different number of source-domain datasets for training,the corresponding optimalσandβshould be selected on the validation set.Since our model is based on the kernel method with a large number of image pairs for training(20,000matched pairs and20,000mismatched pairs from each source-domain dataset),thus an important consideration is how to efficiently approximate the kernel matrix using a low-rank method in the limited space and time.We adopt the low rank method for kernel approximation.In our experiments,we take two steps to determine the number of anchor points. In thefirst step,the optimalσandβare selected on the validation set in each experiment.In the second step,we fixσandβ,and then tune the number of anchor points. We vary the number of anchor points to train our model on the training set,and test it on the validation set.We report the average accuracy for our model over10trials.After we consider the trade-off between memory and running time in practice,the number of anchor points with the best average accuracy is determined in each experiments.3The other parameters,such as the hyper-parameters in the kernel function,can be automatically learned from the data.。
2015下半年大学英语四级作文范文:浅谈整容
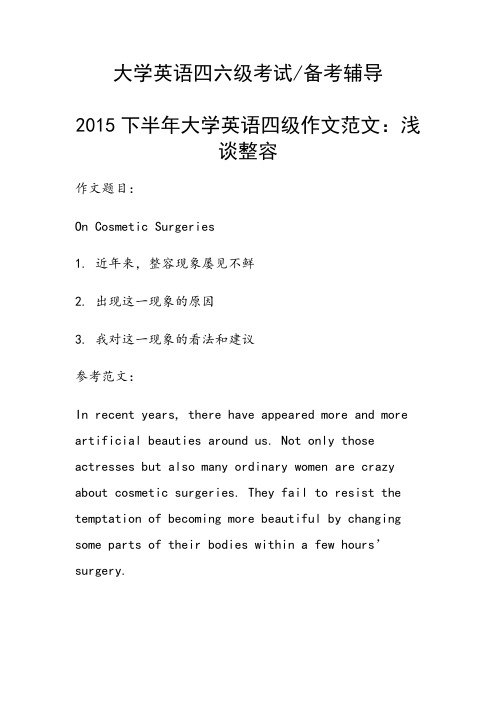
大学英语四六级考试/备考辅导2015下半年大学英语四级作文范文:浅谈整容作文题目:On Cosmetic Surgeries1. 近年来,整容现象屡见不鲜2. 出现这一现象的原因3. 我对这一现象的看法和建议参考范文:In recent years, there have appeared more and more artificial beauties around us. Not only those actresses but also many ordinary women are crazy about cosmetic surgeries. They fail to resist the temptation of becoming more beautiful by changing some parts of their bodies within a few hours’surgery.What are responsible for the prevalence of cosmetic surgeries? Firstly, thanks to the development of shaping techniques, the chances of successful surgeries have increased greatly, which makes more people begin to believe and accept cosmetic surgeries. Secondly, with the improvement of living standard, people have more money to satisfy their nature of pursuing beauty.From my point of view, cosmetic surgeries havetheir necessity of existence in modern society. They do help some people build confidence by giving them a beautiful face or figure. However, cosmetic surgeries cannot bring permanent beauty and might be dangerous. Besides, I think one’s inner beauty is more important. Therefore, I suggest that people should think twice before deciding to accept cosmetic surgeries.2015下半年大学英语四级作文范文:浅谈整容.doc [全文共630字] 编号:6137810。
《Teenage Life》Project and Video Time的学习模态ppt教学模板

3.The Highlights 特色 The different styles of facial make-up are the highlights and
they require distinctive techniques of painting.Exaggerated (夸张 的) designs are painted on each performer's face to symbolize a character's personality.
Section Ⅶ Project and Video Time的学习模态 ——用英语介绍中国(中国戏曲)
If you are in the night of life, don't forget to give you a ray of light — a ray of
light with hope, a ray of light with elf-confidence, a ray of light to face life smilingly, a ray of light to save your whole life.
•
1.根据近十年的高考全国卷真题,总 结出文 言文阅 读中文 本概括 分析题 这一题 型的特 点,每 一个选 项都由 论点和 论据的 形式组 成陈反 映。一 个王朝 成功的 经验和 失败的 教训往 往成为 后来的 政治家 和思想 家的素 材。他 们思考 和论证 往事的 得失, 以史为 鉴,为 当朝和 后代的 统治者 提供新 的治国 方针。
refined tunes. W:哇!昆曲被联合国教科文组织列入“世界文化遗产名录”了。 M:它以行腔婉转、曲调典雅而著称。
2014年3月高考英语考前突破 阅读理解能力 社会生活 自拍风潮影响面部整形
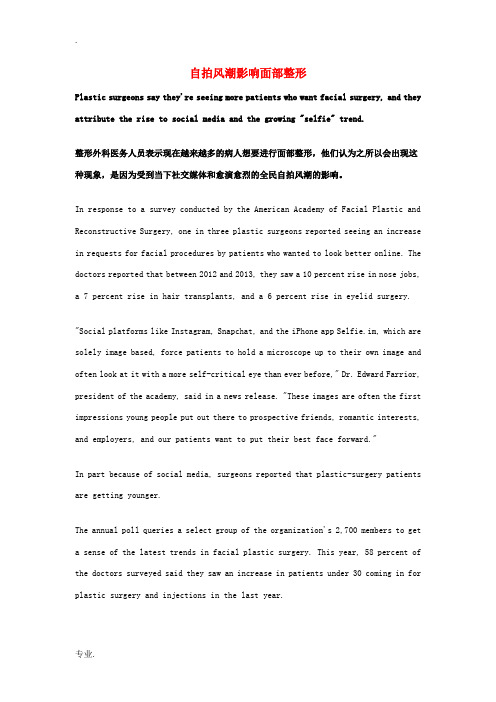
自拍风潮影响面部整形Plastic surgeons say they're seeing more patients who want facial surgery, and they attribute the rise to social media and the growing "selfie" trend.整形外科医务人员表示现在越来越多的病人想要进行面部整形,他们认为之所以会出现这种现象,是因为受到当下社交媒体和愈演愈烈的全民自拍风潮的影响。
In response to a survey conducted by the American Academy of Facial Plastic and Reconstructive Surgery, one in three plastic surgeons reported seeing an increase in requests for facial procedures by patients who wanted to look better online. The doctors reported that between 2012 and 2013, they saw a 10 percent rise in nose jobs, a 7 percent rise in hair transplants, and a 6 percent rise in eyelid surgery."Social platforms like Instagram, Snapchat, and the iPhone app Selfie.im, which are solely image based, force patients to hold a microscope up to their own image and often look at it with a more self-critical eye than ever before," Dr. Edward Farrior, president of the academy, said in a news release. "These images are often the first impressions young people put out there to prospective friends, romantic interests, and employers, and our patients want to put their best face forward."In part because of social media, surgeons reported that plastic-surgery patients are getting younger.The annual poll queries a select group of the organization's 2,700 members to get a sense of the latest trends in facial plastic surgery. This year, 58 percent of the doctors surveyed said they saw an increase in patients under 30 coming in for plastic surgery and injections in the last year.The study found that bullying is also a factor in young people deciding to get surgery, "but most surgeons surveyed report children and teens are undergoing plastic surgery as a result of being bullied (69 percent) rather than to prevent being bullied (31 percent)."Women are still plastic surgery's primary customers, accounting for 81 percent of all procedures and injections, but men are increasingly becoming more interested in plastic surgery. Whereas women more often ask for facelifts and eye lifts, men are more interested in keeping their hair and combating wrinkles.Meanwhile, in the under-35 category, the nose job remained the most popular elective surgical procedure for both genders, accounting for 90 percent of procedures in women and 86 percent in men.Have your selfies ever made you feel self-conscious about the way you look?。
2021届辽宁省辽南协作校(朝阳市)高三下学期4月第二次模拟考试(二模)英语试题(含答案)
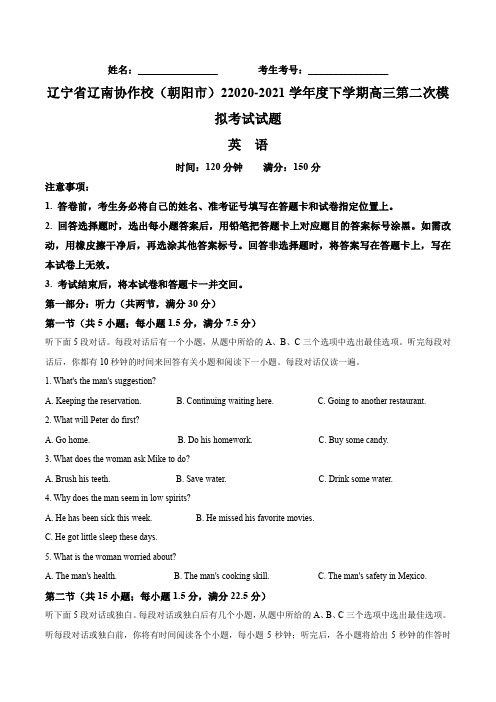
姓名:________________ 考生考号:________________辽宁省辽南协作校(朝阳市)22020-2021学年度下学期高三第二次模拟考试试题英语时间:120分钟满分:150分注意事项:1. 答卷前,考生务必将自己的姓名、准考证号填写在答题卡和试卷指定位置上。
2. 回答选择题时,选出每小题答案后,用铅笔把答题卡上对应题目的答案标号涂黑。
如需改动,用橡皮擦干净后,再选涂其他答案标号。
回答非选择题时,将答案写在答题卡上,写在本试卷上无效。
3. 考试结束后,将本试卷和答题卡一并交回。
第一部分:听力(共两节,满分30分)第一节(共5小题;每小题1.5分,满分7.5分)听下面5段对话。
每段对话后有一个小题,从题中所给的A、B、C三个选项中选出最佳选项。
听完每段对话后,你都有10秒钟的时间来回答有关小题和阅读下一小题。
每段对话仅读一遍。
1. What's the man's suggestion?A. Keeping the reservation.B. Continuing waiting here.C. Going to another restaurant.2. What will Peter do first?A. Go home.B. Do his homework.C. Buy some candy.3. What does the woman ask Mike to do?A. Brush his teeth.B. Save water.C. Drink some water.4. Why does the man seem in low spirits?A. He has been sick this week.B. He missed his favorite movies.C. He got little sleep these days.5. What is the woman worried about?A. The man's health.B. The man's cooking skill.C. The man's safety in Mexico.第二节(共15小题;每小题1.5分,满分22.5分)听下面5段对话或独白。
- 1、下载文档前请自行甄别文档内容的完整性,平台不提供额外的编辑、内容补充、找答案等附加服务。
- 2、"仅部分预览"的文档,不可在线预览部分如存在完整性等问题,可反馈申请退款(可完整预览的文档不适用该条件!)。
- 3、如文档侵犯您的权益,请联系客服反馈,我们会尽快为您处理(人工客服工作时间:9:00-18:30)。
1-4244-0991-8/07/$25.00/©2007 IEEE3554have:
u v 1 = f cos θ 0 − sin θ 0 f 0 f sin θ 0 cos θ ft ft tz x
x y
(1)
3-points method. First three points are found with the following properties:
A survey of techniques for Face Reconstruction
Ching Y. Suen 1, Arash Zaryabi Langaroudi2, Chunghua Feng1, Yuxing Mao 1
1
Centre for Pattern Recognition and Machine Intelligence (CENPARMI), Concordia University, Montreal, QC, Canada
2
Department of Computer Science and Software Engineering, Concordia University, Montreal, QC, Canada
Abstract— This paper presents a study on some approaches in face reconstruction and the methodology used. The results and drawbacks of each method are discussed. To construct the 3D face model there are some methods which select the features automatically or manually. First a method in textured 3D face reconstruction using two 2D images from any angle is discussed which does not need any particular database, but it has to define the feature points manually. Then we describe automatic 2D to 3D face reconstruction from a single frontal face image which needs the use of USF Human ID 3-D database. Afterwards we talk about a Model Based Face Reconstruction for Animation. We also describe briefly about 3D face modeling by fusing multiple 2D images which is fully automatic and via an EM approach which uses the shape and pose parameters. Finally we describe a Rapid Modeling of Animated Faces from Video method and 2D face reconstruction using a minimum set of feature points. Keywords: Face Reconstruction, feature point, texture generating, Pose estimation.
This work was supported in part by Bell Canada and the Natural Sciences and Engineering Research Council of Canada.
Fig. 1. System Overview [1]
A. Analytical 3D reconstruction algorithm for feature points By using orthogonal projections, the 3D Coordinates of the feature points are generated. If P=(x,y,z)T are 3D coordinates on the real face shape then its homogeneous coordinates will be P’=(x,y,z,1). Also Q=(u,v)T which are the corresponding coordinates of the image point in the camera are Q’=(u,v,1)T. By using camera pinhole model and assuming that the world coordinate system only rotates around the y-axis, we
of the database. In this paper we present a survey of some of the approaches to reconstruct the 3D face shape. This paper is organized as follows: First a method in textured 3D face reconstruction using two 2D images from any angle is discussed which does not need any particular database but it needs to define the feature points manually [1]. Then we describe an automatic 2D to 3D face reconstruction from a single frontal face image which needs the use of USF Human ID 3-D database [2]. Then we will talk about a Model Based Face Reconstruction for Animation [8], and briefly talk about 3D face modeling by fusing multiple 2D images which is fully automatic. Afterwards we discuss about a Rapid Modeling of Animated Faces based on the Video method [6] and 2D face reconstruction using a minimum set of feature points [4]. II. 3D FACE RECONSTRUCTION WITH TWO IMAGES AT ANY ANGLE USING 35 FEATURE POINTS [1] In this section we will describe a new approach for textured 3D face reconstruction based on two images taken at any angle. This technique uses 35 feature points corresponding to 2 images which are marked manually. First the analytical coordinates of the feature points are confirmed. Then by using Kriging interpolation method, missing vertices are produced. Finally the view-dependant or view-independent texture maps are generated.
y z 1
zA = zB
x c = (x A + x B )/2 z A ≠ zc
(2)
where f is the focal length of the camera and tx, ty, t z are the translation vector parameters in pinhole camera mode and is the angle of the image according to the camera. This technique assumes that the projection is orthogonal which requires the images of the face to be taken from a distance of two meters or more. We have two images with different angles 1 and 2. The feature points are marked on both images, so Q1 and Q2 show the same physical point on the real world (See Fig. 2). By solving equation 1 for x, y and z with 1 and 2, the 3D coordinates of the feature points will be obtained.
I. INTRODUCTION 3D modeling of human faces has applications ranging from 3D human face animation to video conferencing. The intuitive method to get face's 3D information is scanning it using a 3D scanner. In Laser Scanning for each pixel of the image, the range to the visible surface of the objects in the scene is known. Lee et al [23] digitized facial geometry through the use of scanning range sensors, but the approach based on 3D digitization and other methods are either cumbersome to operate or expensive to obtain. Cheaper and easier alternatives have been explored in the past two decades. It is a challenge to realize 3D face construction based on 2D face images. There are already numerous complicated techniques in this field. Various algorithms have been proposed for this problem, but the performances have been far from satisfactory. There are feature-based and appearance-based methods to construct a 3D face model. In the first method, a generic model in 3D is provided in advance, and a limited number of feature points are extracted either automatically or manually. Usually only the feature points with distinct characteristics can be located automatically. Feature points cooperate with the generic face information in the database to construct the face shape accurately. Note that the construction of an accurate face shape will be constrained by the characteristics