非平稳时间序列的建模研究
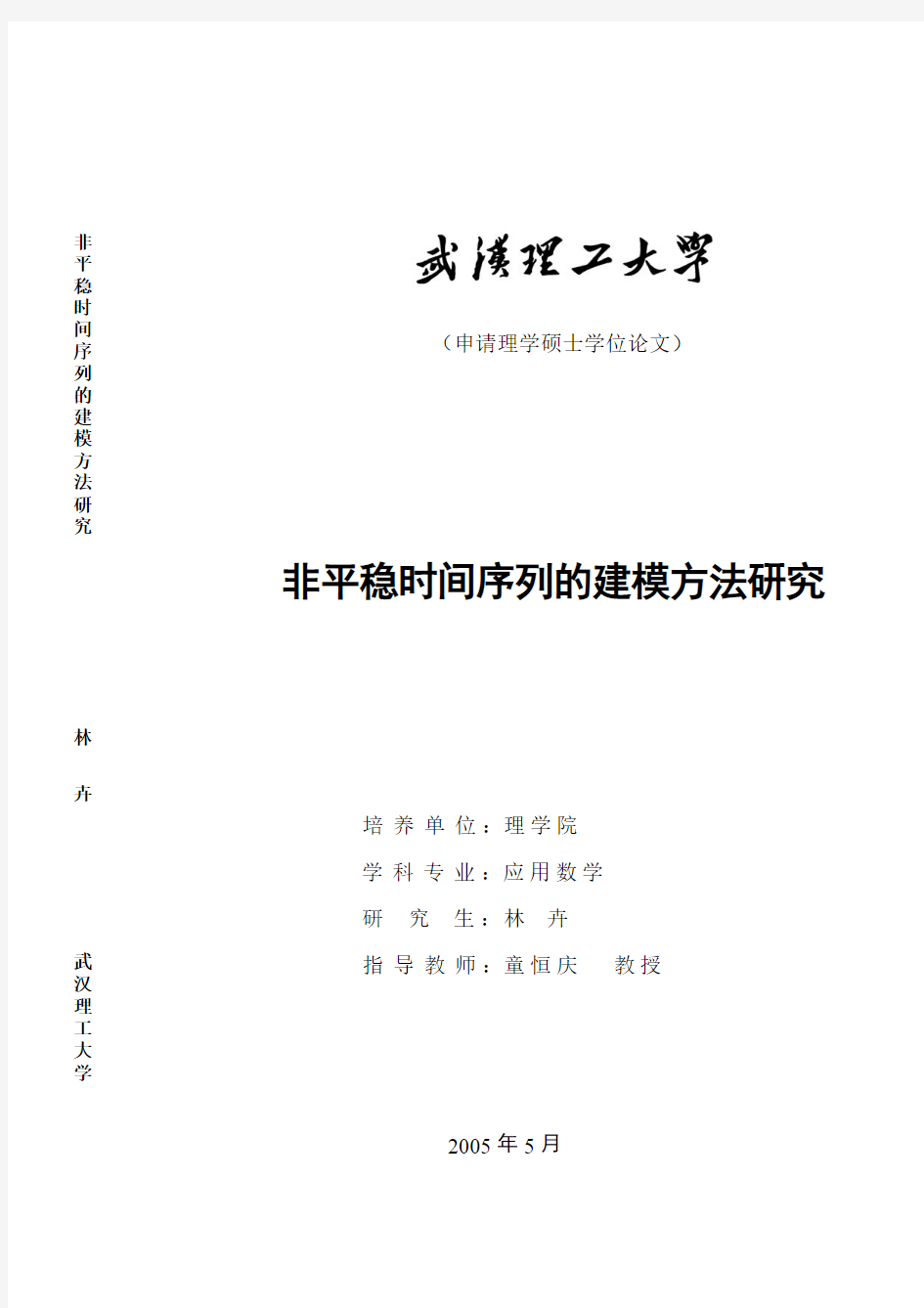
- 1、下载文档前请自行甄别文档内容的完整性,平台不提供额外的编辑、内容补充、找答案等附加服务。
- 2、"仅部分预览"的文档,不可在线预览部分如存在完整性等问题,可反馈申请退款(可完整预览的文档不适用该条件!)。
- 3、如文档侵犯您的权益,请联系客服反馈,我们会尽快为您处理(人工客服工作时间:9:00-18:30)。
(申请理学硕士学位论文)
非平稳时间序列的建模方法研究
培养单位 :理学院
学科专业 :应用数学
研 究 生 :林 卉 指导教师 :童恒庆 教授
2005年5月
非
平
稳
时
间
序
列
的
建
模
方
法
研
究
林
卉
武
汉
理
工
大
学
分类号密级
UDC 学校代码 10497
学位论文
题目非平稳时间序列的建模方法研究
英文The Research on the Method of the Modeling
题目of Nonstationary Time Series
研究生姓名林 卉
姓名童恒庆职称教授学位博士
指导教师
单位名称理学院邮编 430070 申请学位级别硕士学科专业名称应用数学
论文提交日期 2005年5月论文答辩日期 2005年6月学位授予单位武汉理工大学日期
答辩委员会主席评阅人
2005年5月
武汉理工大学硕士学位论文
中文摘要
时间序列分析是概率统计学中一个内容十分丰富的重要分支,近年来它在理论与应用两方面都得到了蓬勃发展。时间序列分析按时间序列的统计特性可以分为平稳时间序列和非平稳时间序列两类。在实际问题中,我们经常遇到的序列,特别是反映社会、经济现象的序列,大多数并不平稳,并且呈现出明显的趋势性或周期性,因此研究非平稳时间序列的建模具有很重要的现实意义。本文的重点是对非平稳时间序列的建模方法进行研究。
首先,本文介绍非平稳时间序列的一些传统的建模方法,主要对ARIMA模型法、季节性模型法、X-11法、回归方法、灰色模型法等方法加以研究分析。
然后,本文对非平稳时间序列的状态空间建模方法进行重点研究,主要有如下的研究工作:
(1)在建模方面,参照Kitagawa和顾岚教授关于时间序列状态空间建模思路,把非平稳时间序列趋势项、循环项和季节项这三项分别建立非平稳时间序列趋势项状态空间模型、非平稳时间序列循环项状态空间模型和非平稳时间序列季节项状态空间模型,最后建立非平稳时间序列的总体的状态空间模型。
(2)在状态估计方面,介绍卡尔曼滤波递推公式的理论依据,采用卡尔曼滤波与固定区间平滑融为一体的算法,对状态空间模型中的状态向量及其误差方差阵进行估计,并用Matlab编写出相应的程序。
(3)介绍改进的EM算法,对改进的EM算法的收敛性给出证明。
(4)在参数估计方面,提出采用卡尔曼滤波、固定区间平滑以及改进的EM 算法融为一体的方法进行估计。
(5)把传统的ARIMA建模方法和本文介绍的状态空间建模方法分别用于我国第三产业总值的预测中,比较两种模型的预测效果。用实例验证使用卡尔曼滤波与固定区间平滑相结合的算法优于单独使用卡尔曼滤波,以及卡尔曼滤波、卡尔曼滤波与固定区间平滑相结合的算法都具有算法稳定性。
关键词:非平稳时间序列,ARIMA模型,状态空间模型
. I
武汉理工大学硕士学位论文
Abstract
Time series analysis is one of important branch in probability and statistics. Recently, the theory and application of time series is developed quickly. According to the characteristic of statistics, time series is divided into two classes. One is stationary time series, the other is nonstationary time series. In daily time we usually observe time series which is almost nonstationary especially in the phenomena of society and economic. The nonstationary time series presents obvious tendency and periodicity. The research of the modeling of nonstationary time series is very important in practice. The study narrates mainly about the method of the modeling of nonstationary time series.
Firstly, some traditional research methods of the modeling of nonstationary time series are introduced in the study. For example, ARIMA model, season model, X-11 model, grey model and so on.
The emphasis of the study is the research of the state space modeling of nonstationary time series, it includes the five points:
(i) In modeling phase, according to the method of Kitagawa and Gulan on the state space modeling of nonstationary time series, time series is decomposed into four items which are trend item, cycle item, season item and random item. Then the study establishes the state space modeling of four items separatly which constitute the total state space modeling of nonstationary time series.
(ii) In the estimate of state vector, the study introduces the academic gist of the Kalman filtering and uses the arithmetic of the Kalman filtering and optimal fixed interval smoothing.
(iii) The study introduces the improved EM algorithm, and it proves the convergent property.
(iv) In the estimate of the parameter, the study uses the arithmetic of the Kalman filtering, optimal fixed interval smoothing and improved EM algorithm.
(v) The study uses the ARIMA model and state space model to forecast the total value of the three industry in our country. It compares the impact of the two model. And it testifies that the arithmetic of the Kalman filtering and optimal fixed interval smoothing is better than the Kalman filtering. And the Kalman filtering and the arithmetic of the Kalman filtering and optimal fixed interval smoothing are steady in arithmetic.
Keywords: nonstationary time series, ARIMA model, state space model
. II